A Novel Transformer based Semantic Segmentation Scheme for Fine-Resolution Remote Sensing Images(语义分割任务) 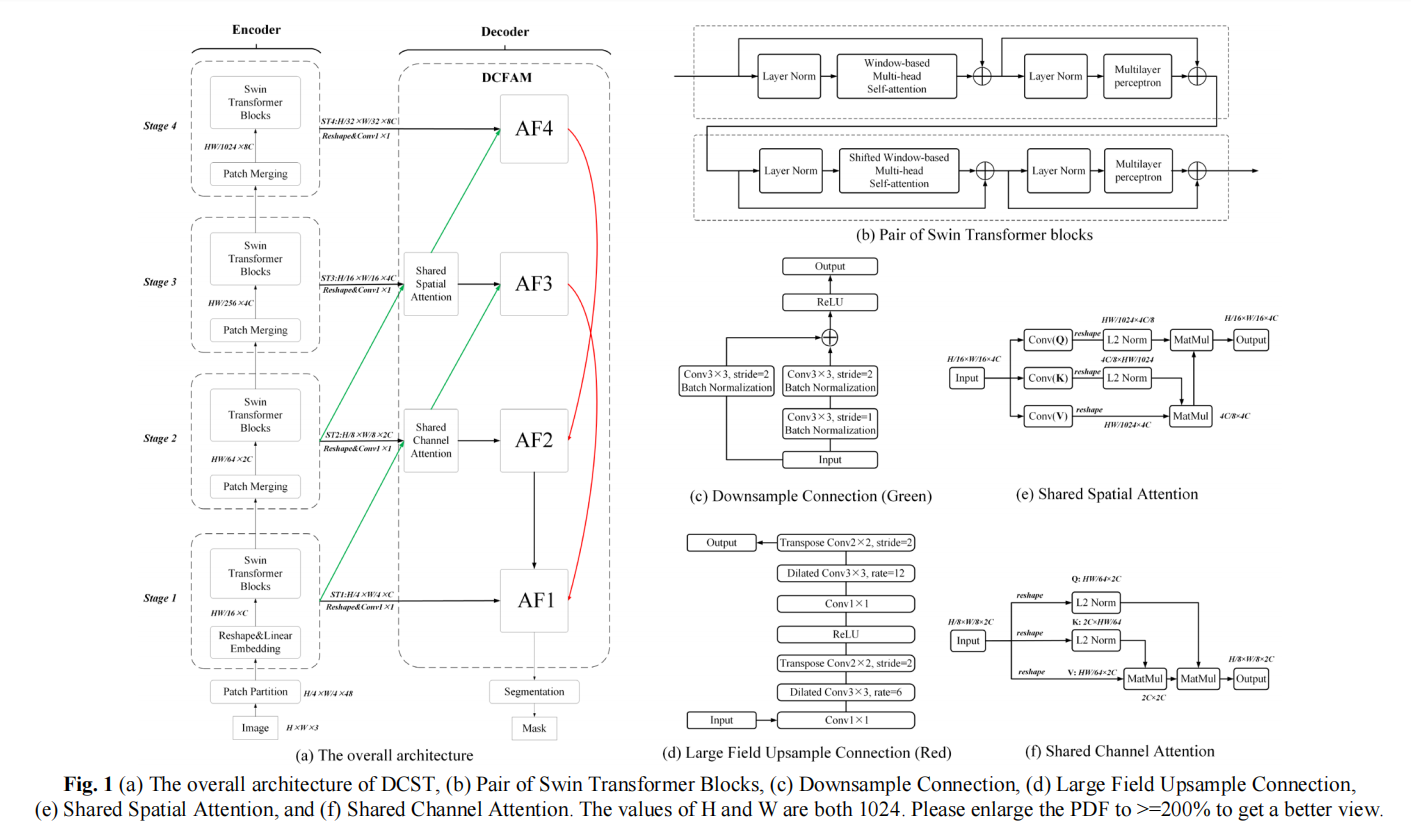 Self-Supervised Learning with Swin Transformers(模型简称:MoBY,使用了对比学习) 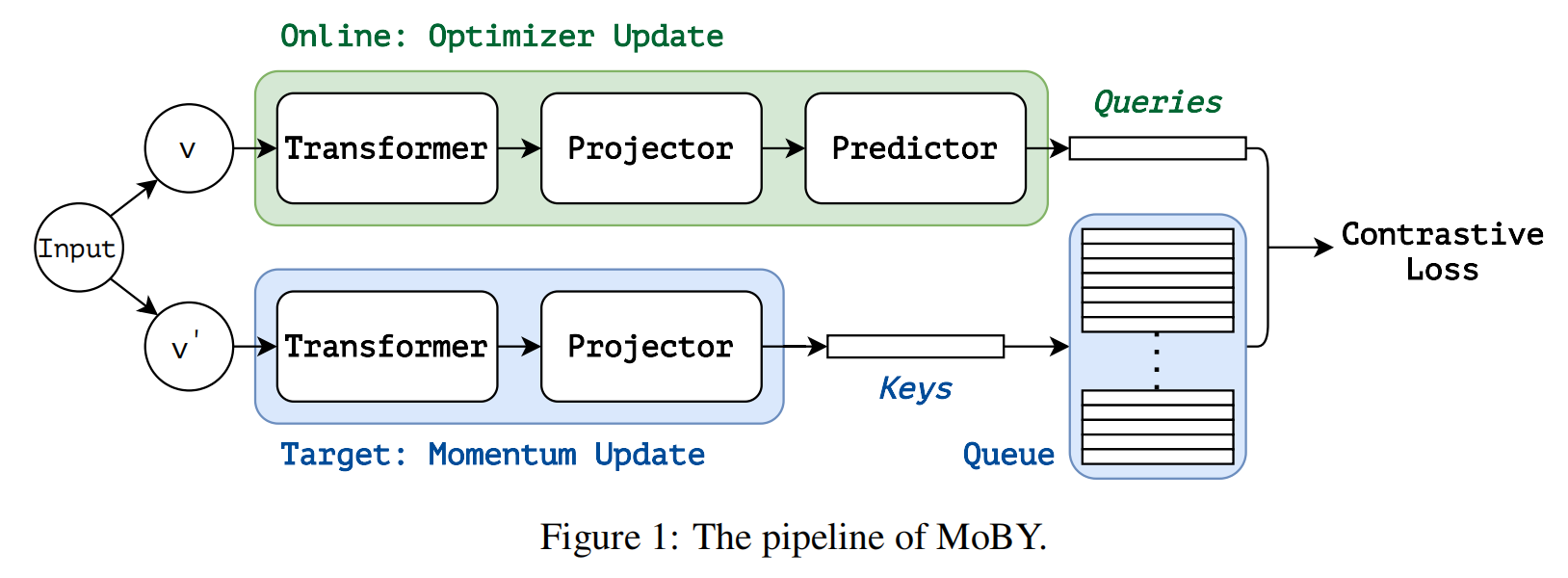 Swin-Unet: Unet-like Pure Transformer for Medical Image Segmentation(医疗图像语义分割)
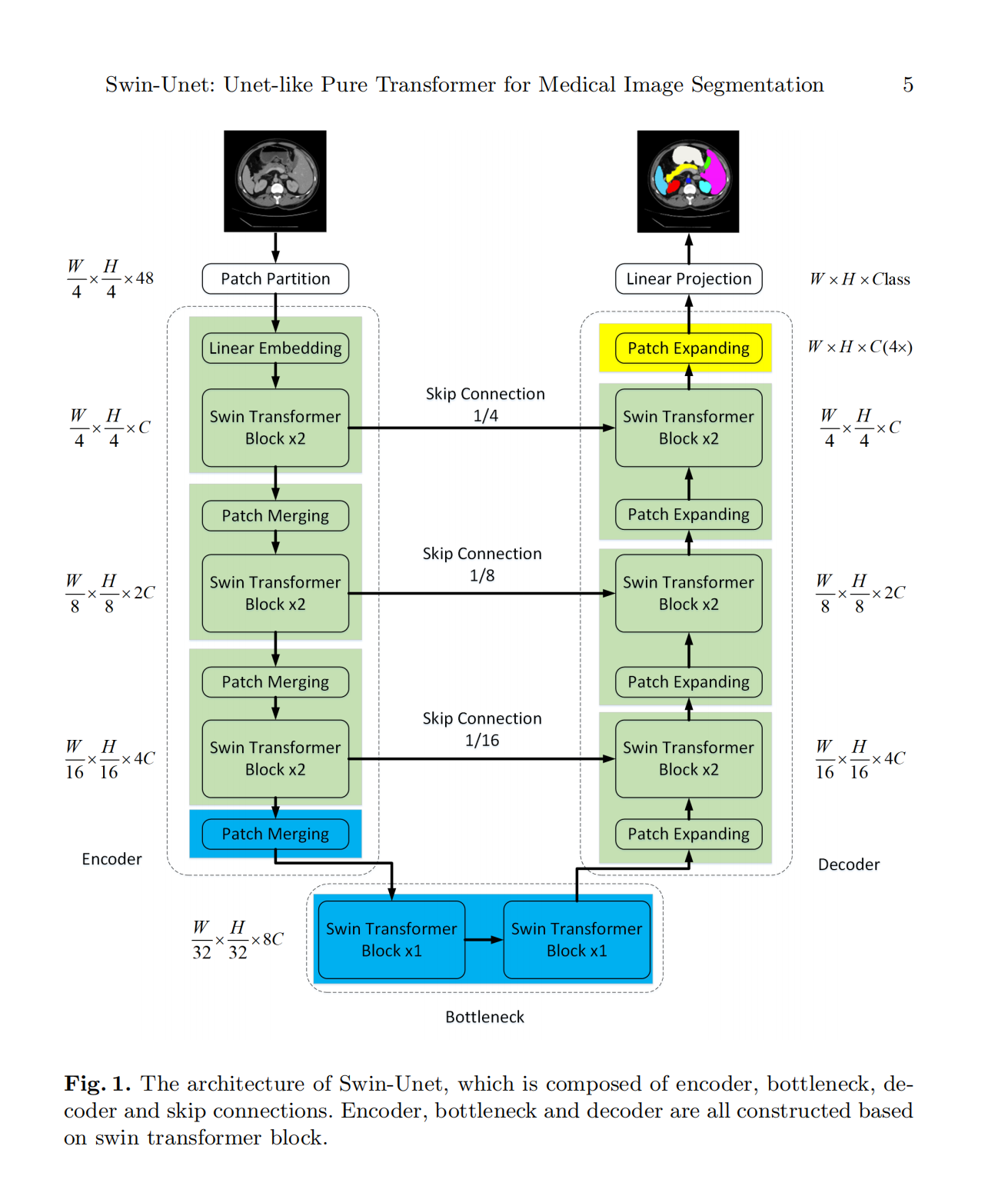 Rethinking Training from Scratch for Object Detection(看不懂)
Demystifying Local Vision Transformer: Sparse Connectivity, Weight Sharing, and Dynamic Weight 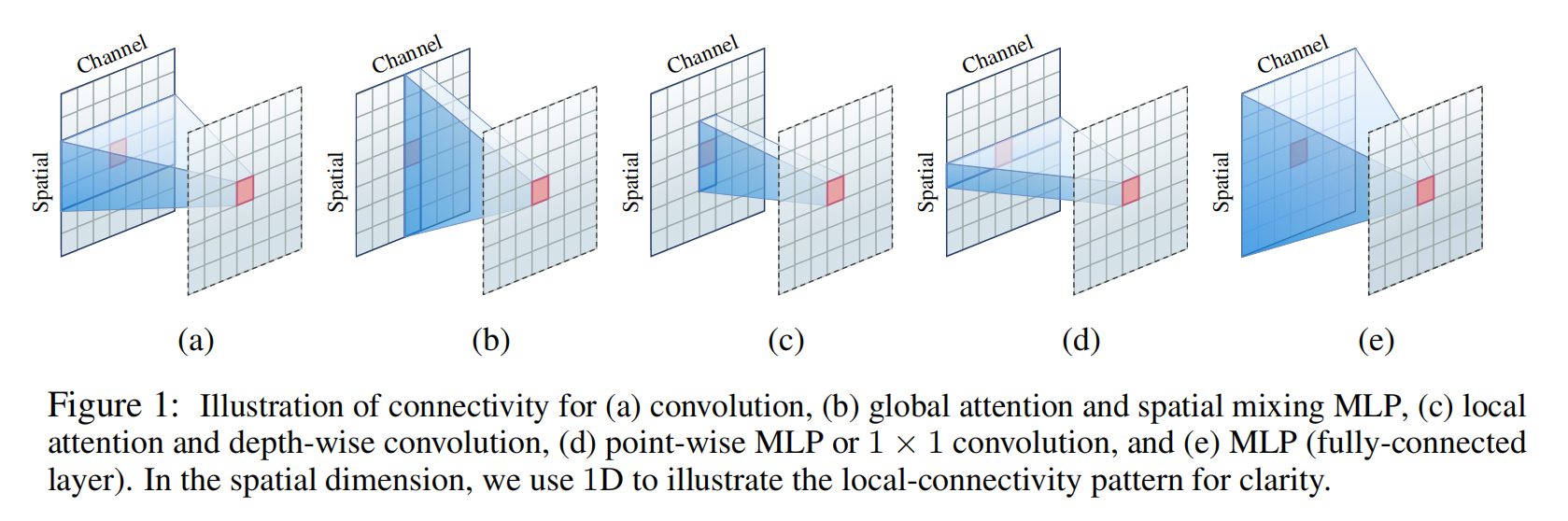 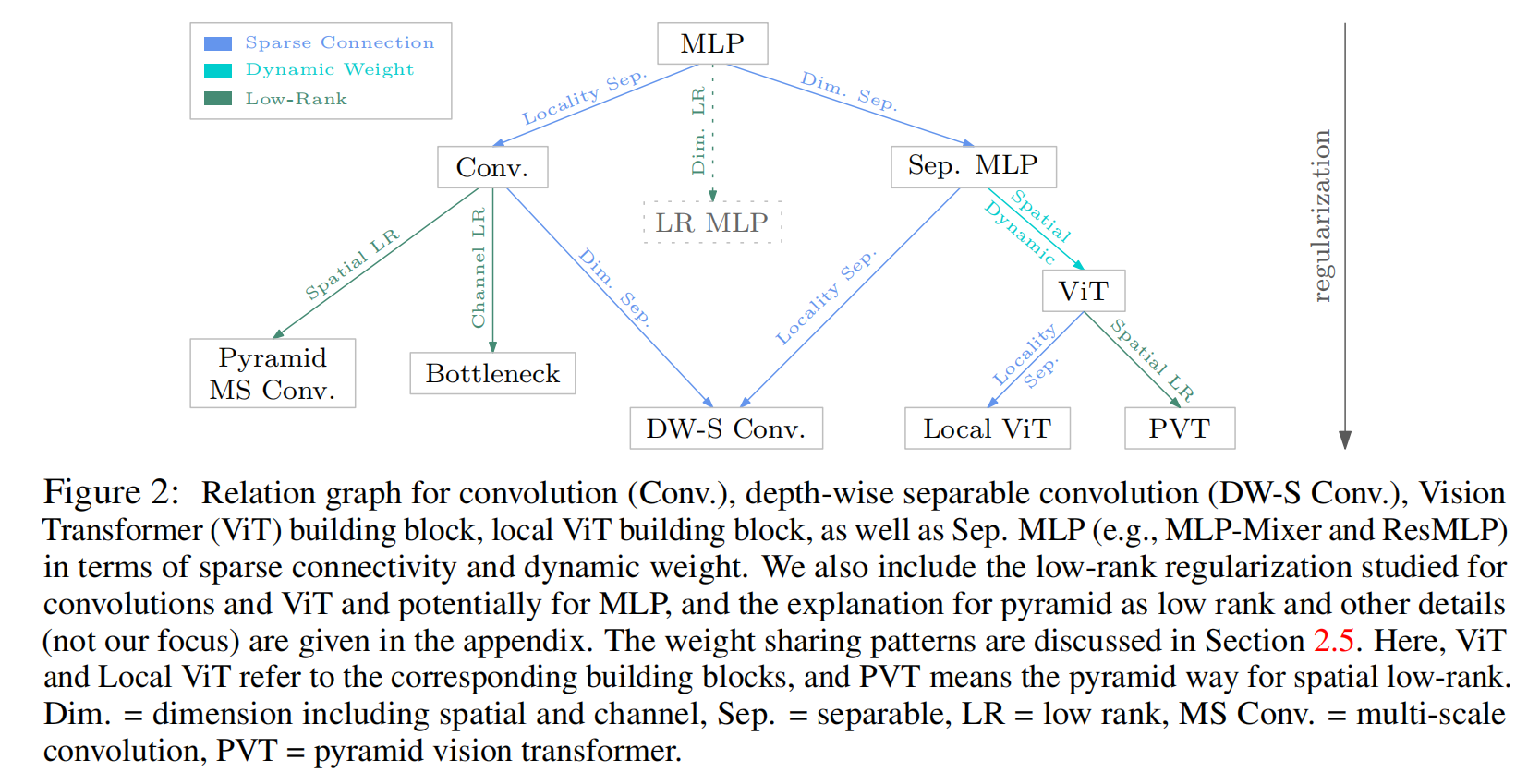 DS-TransUNet: Dual Swin Transformer U-Net for Medical Image Segmentation(医疗图像的语义分割) 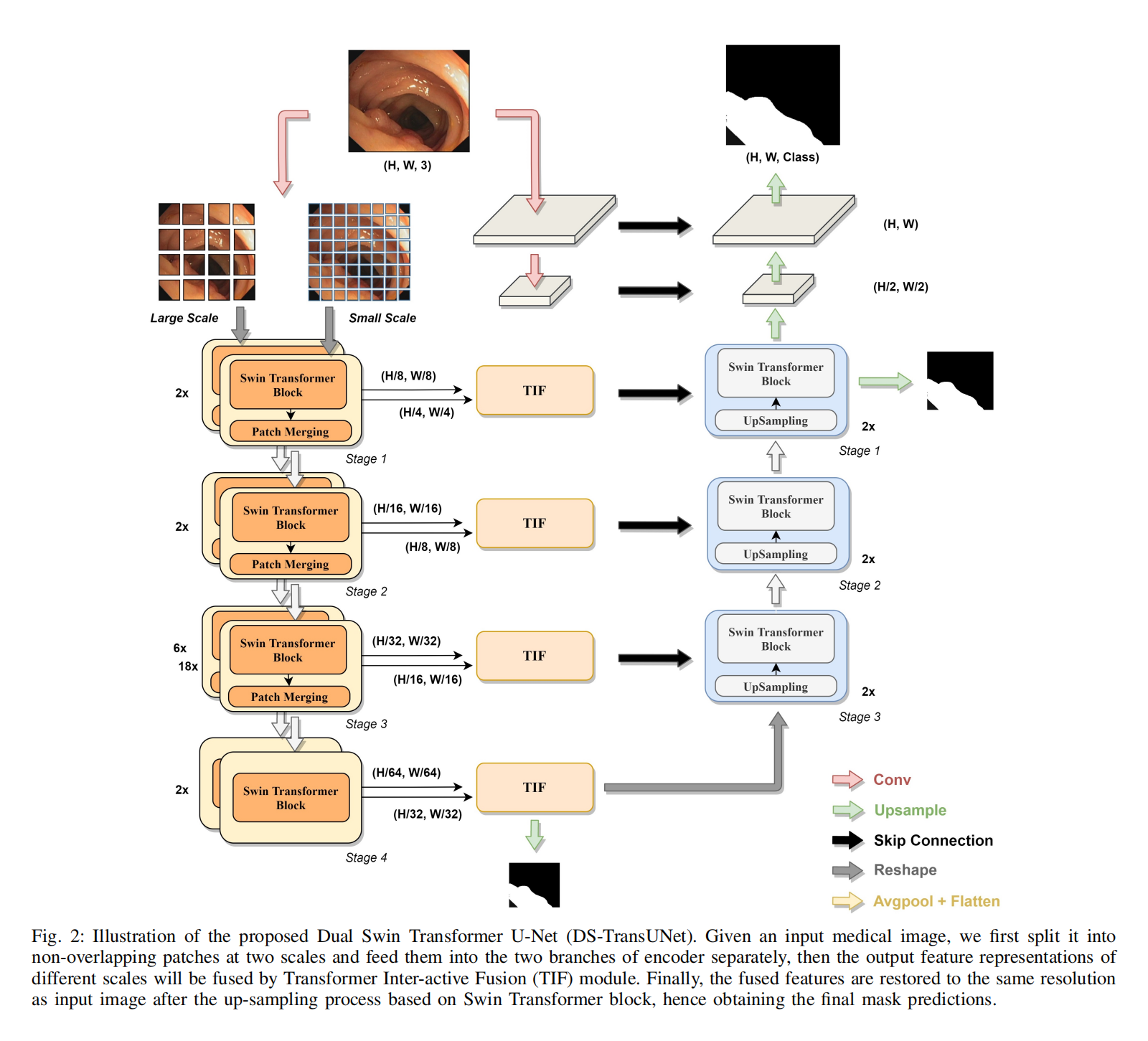 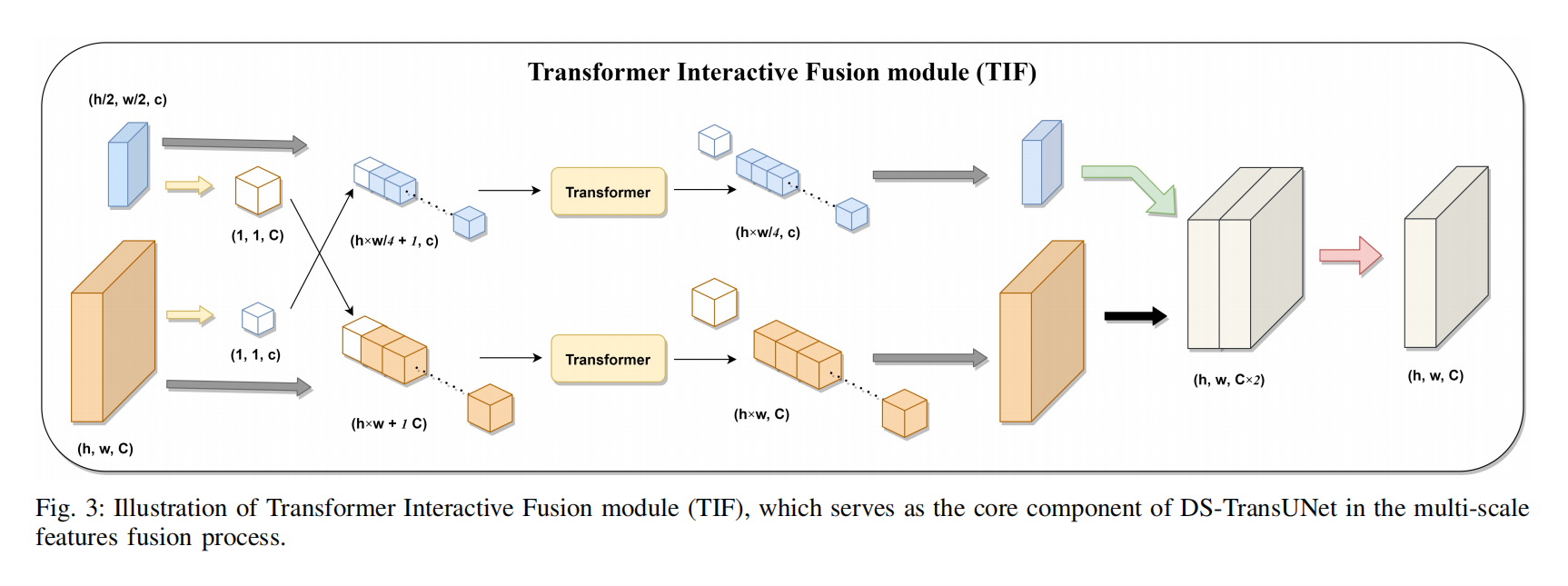 Long-Short Temporal Contrastive Learning of Video Transformers 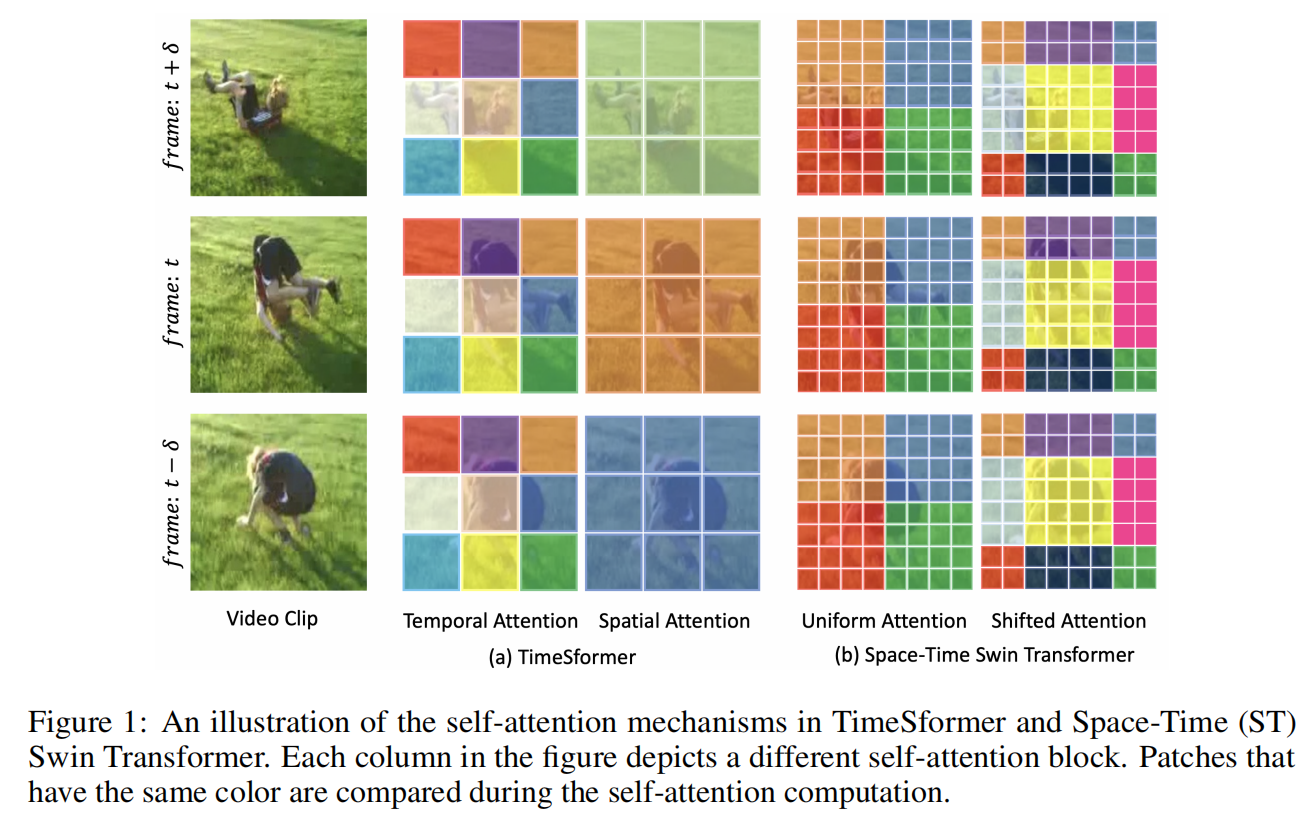 Video Swin Transformer 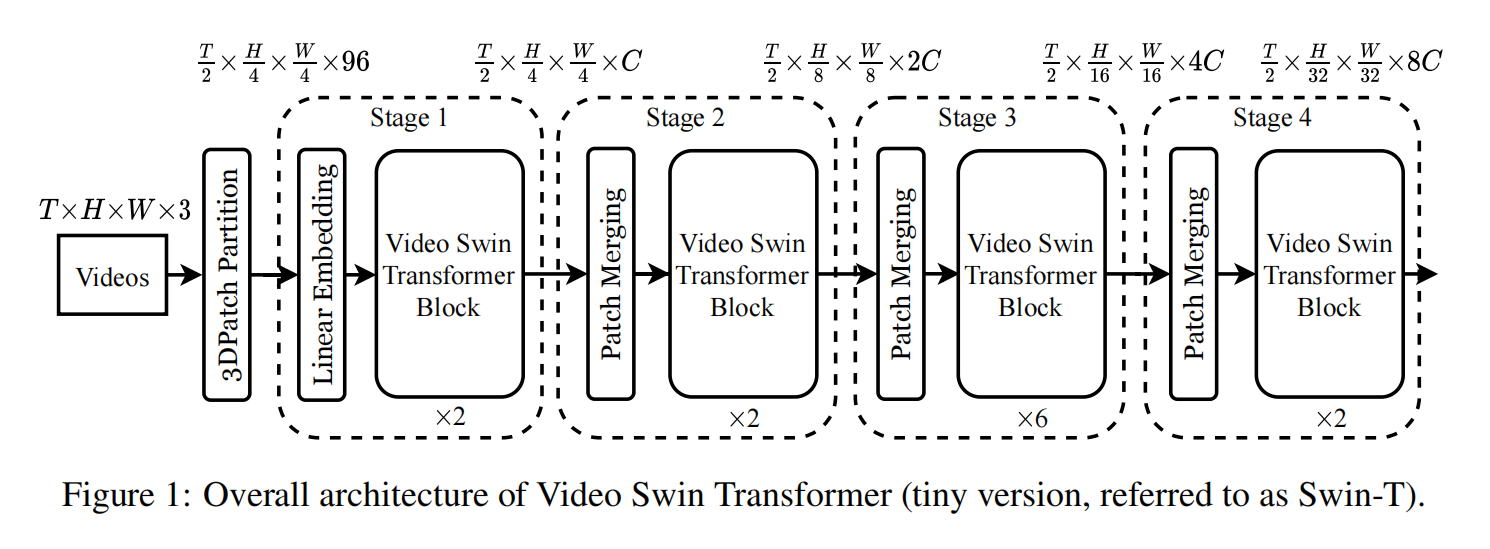 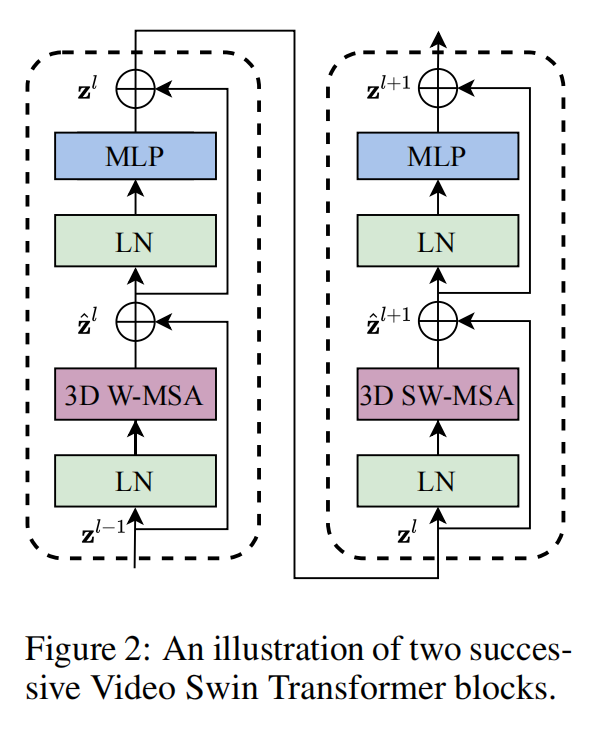 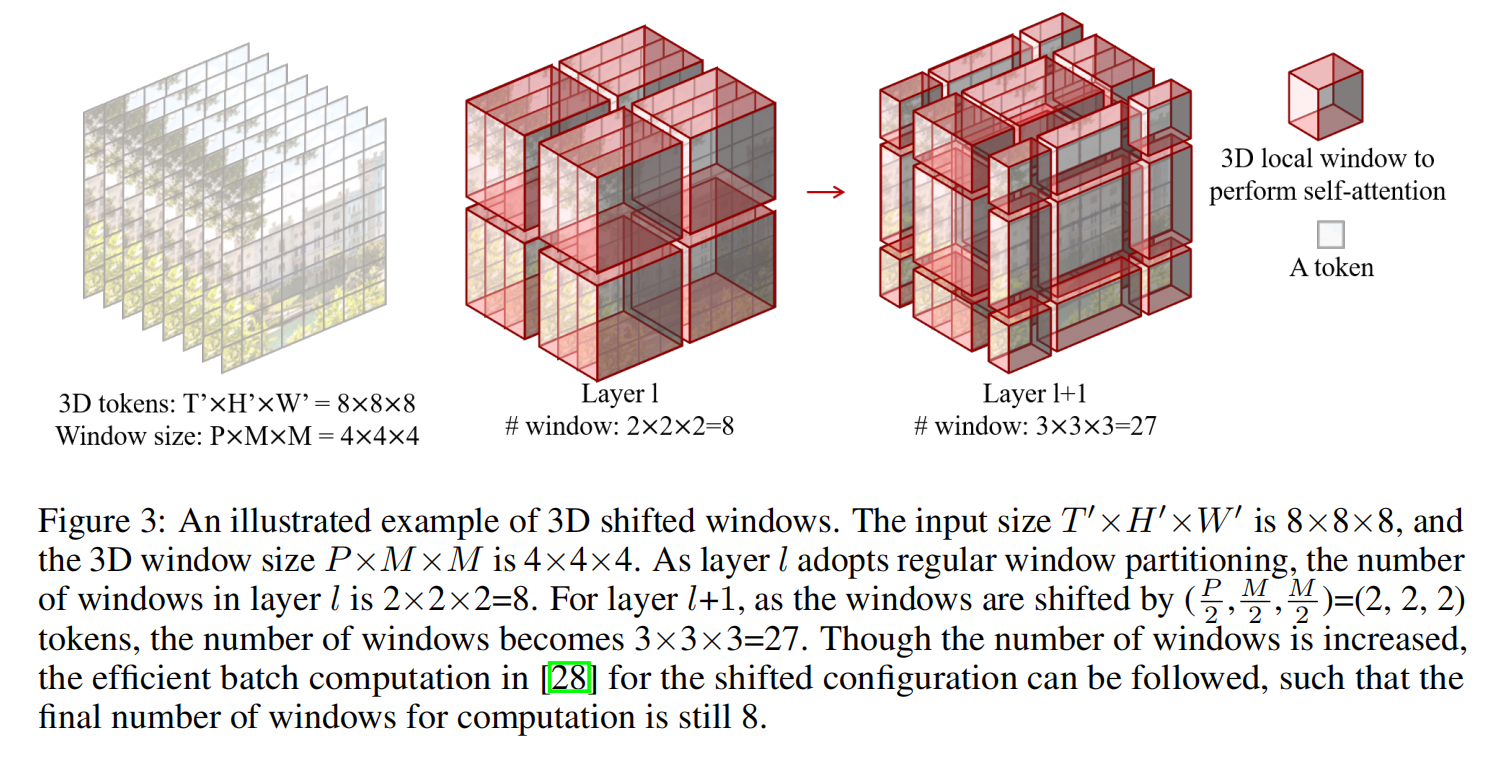 PVTv2: Improved Baselines with Pyramid Vision Transformer(Pyramid:金字塔) 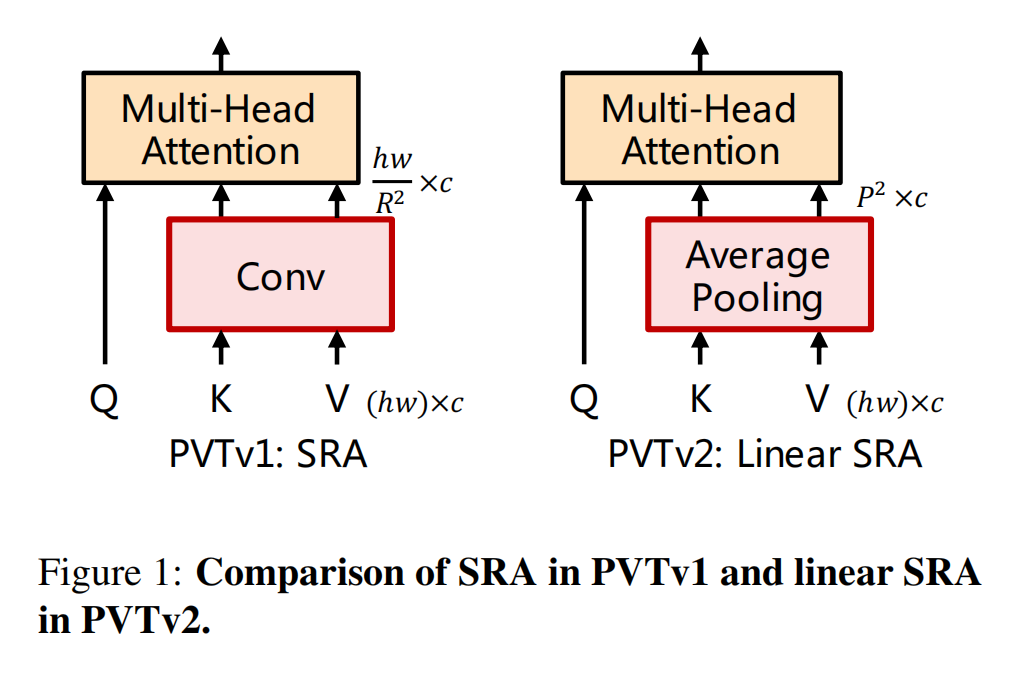 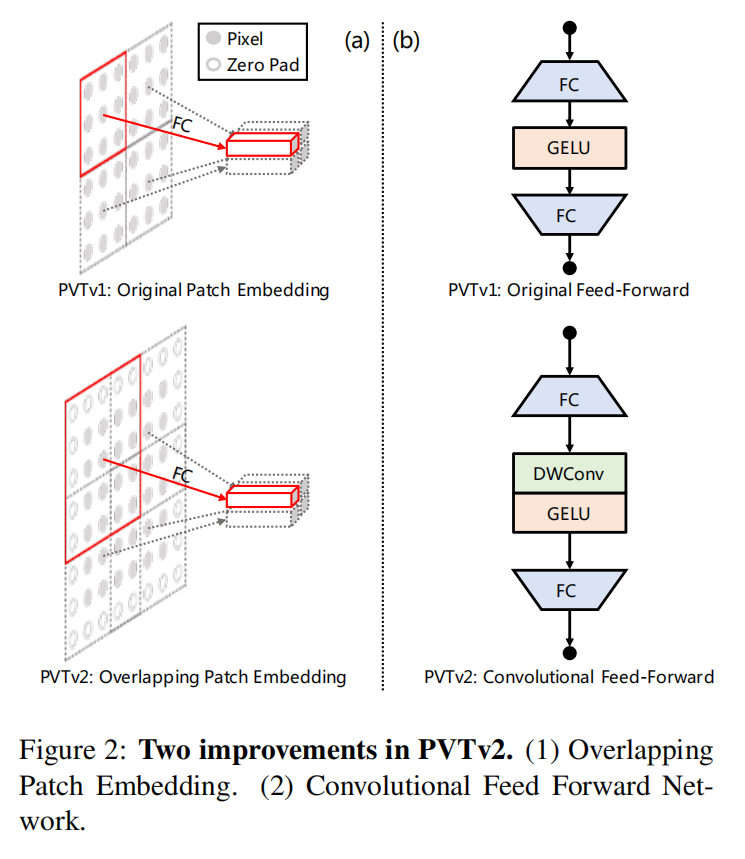 CSWin Transformer: A General Vision Transformer Backbone with Cross-Shaped Windows 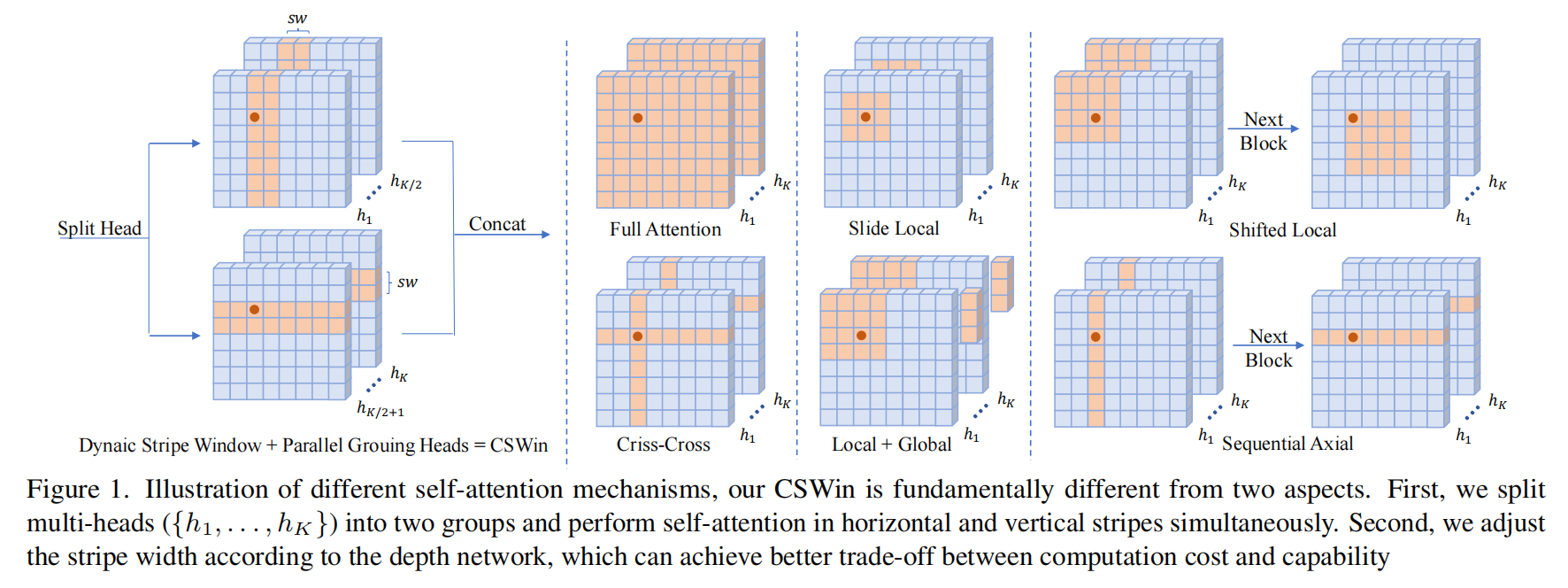 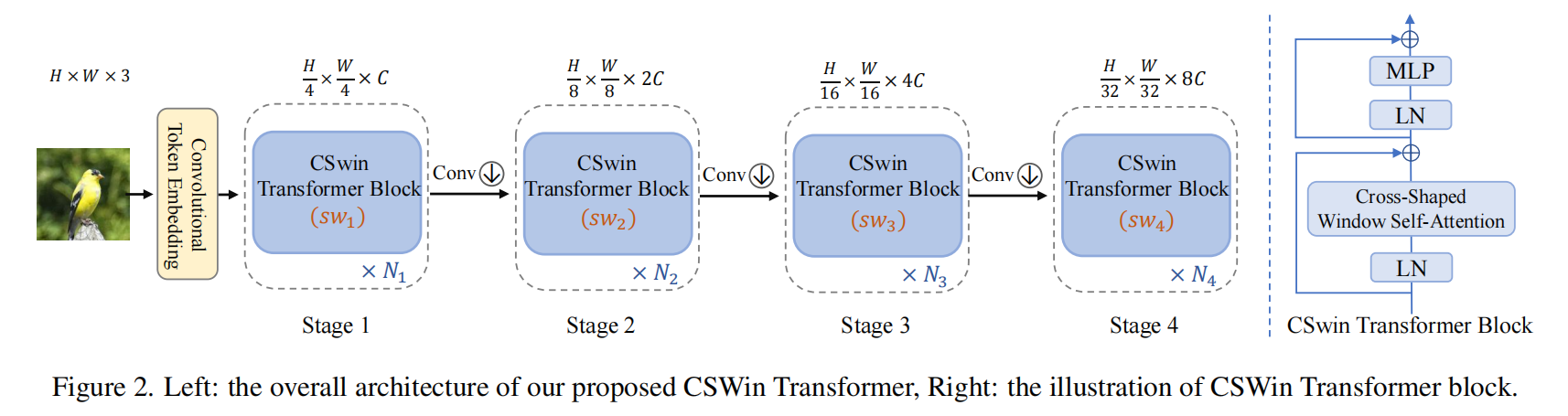 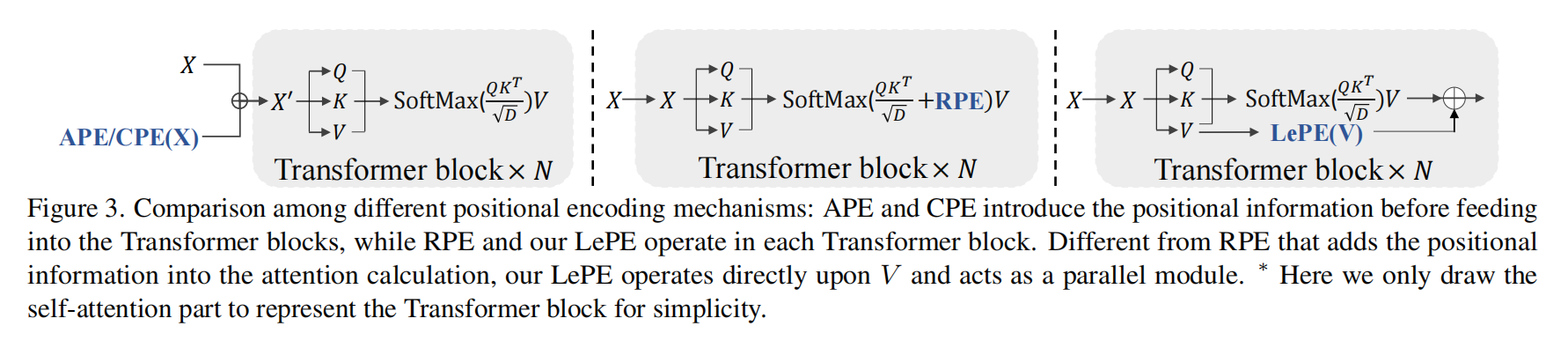 What Makes for Hierarchical Vision Transformer? 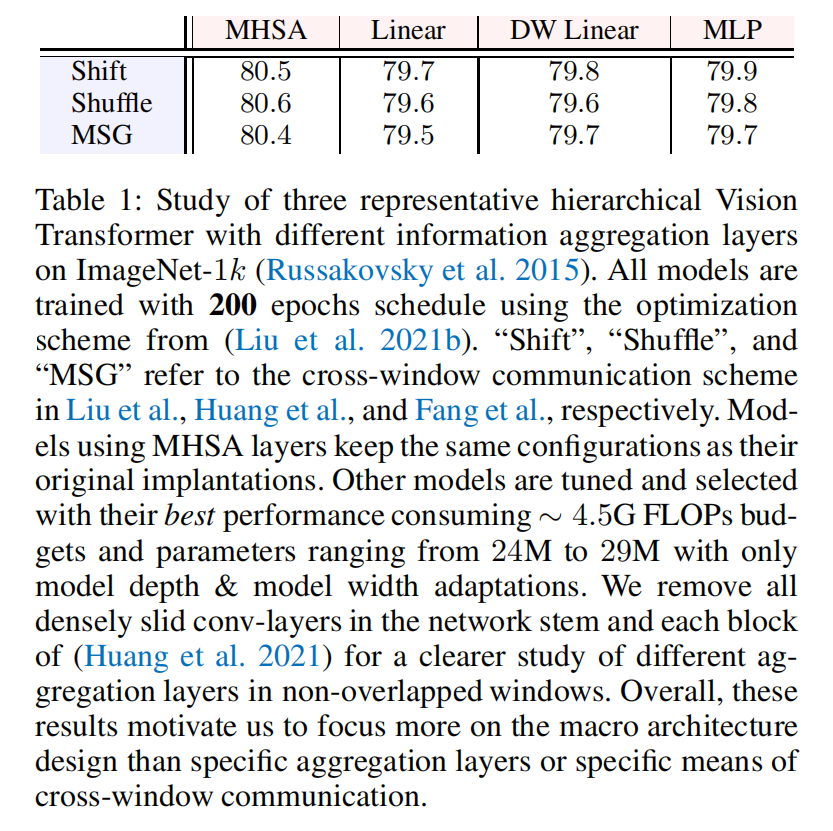
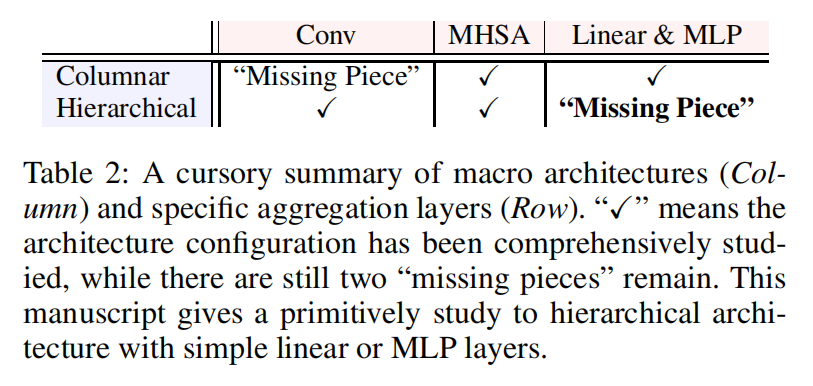
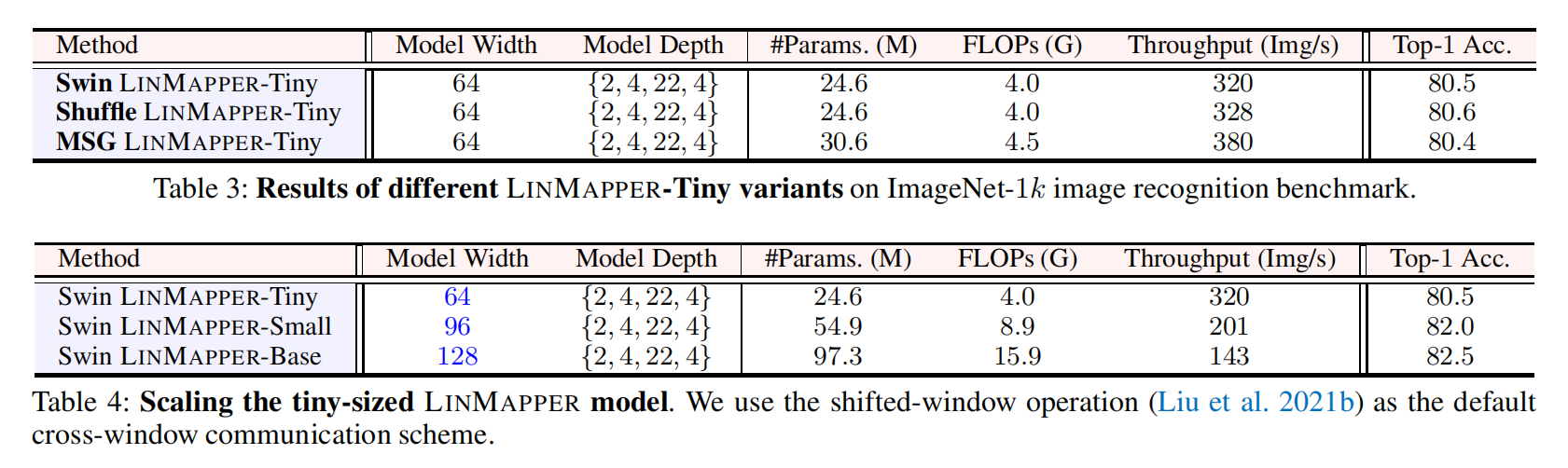
CYCLEMLP: A MLP-LIKE ARCHITECTURE FOR DENSE PREDICTION 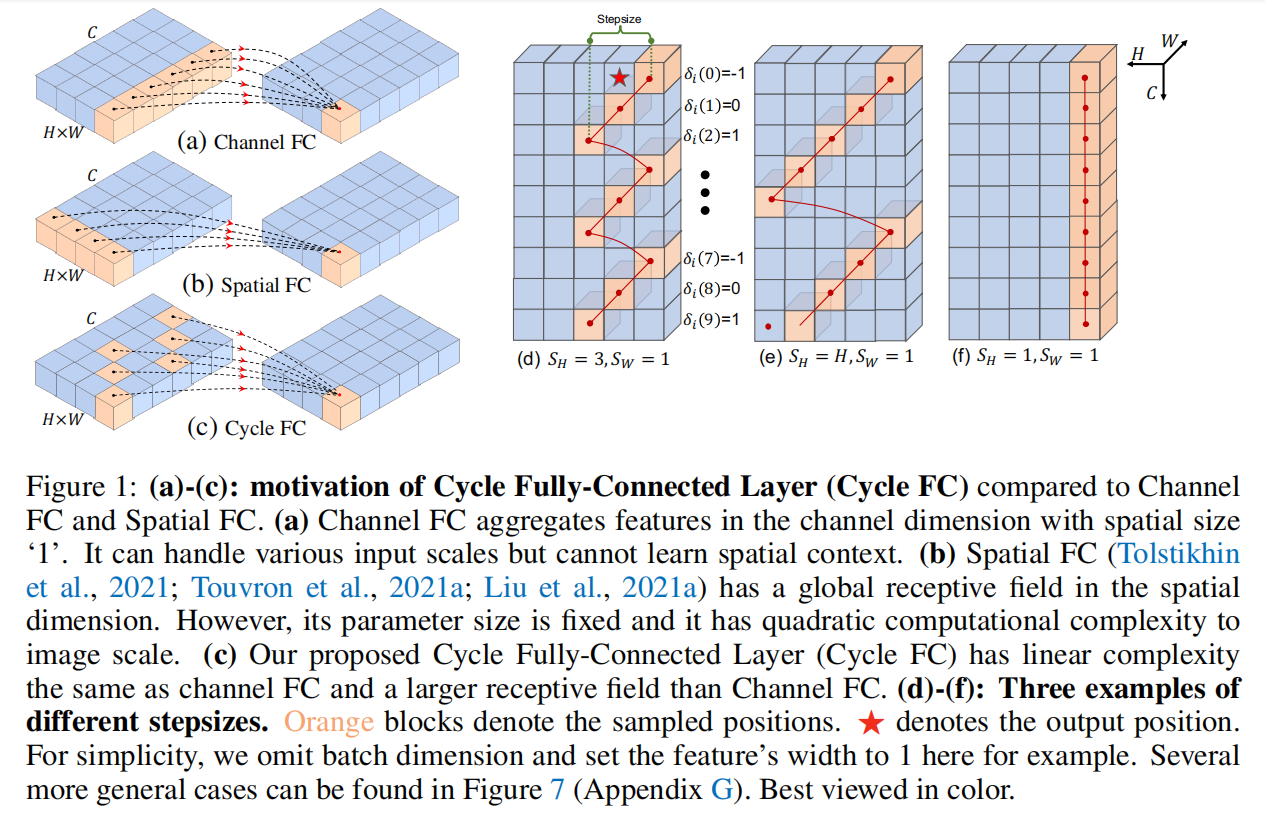 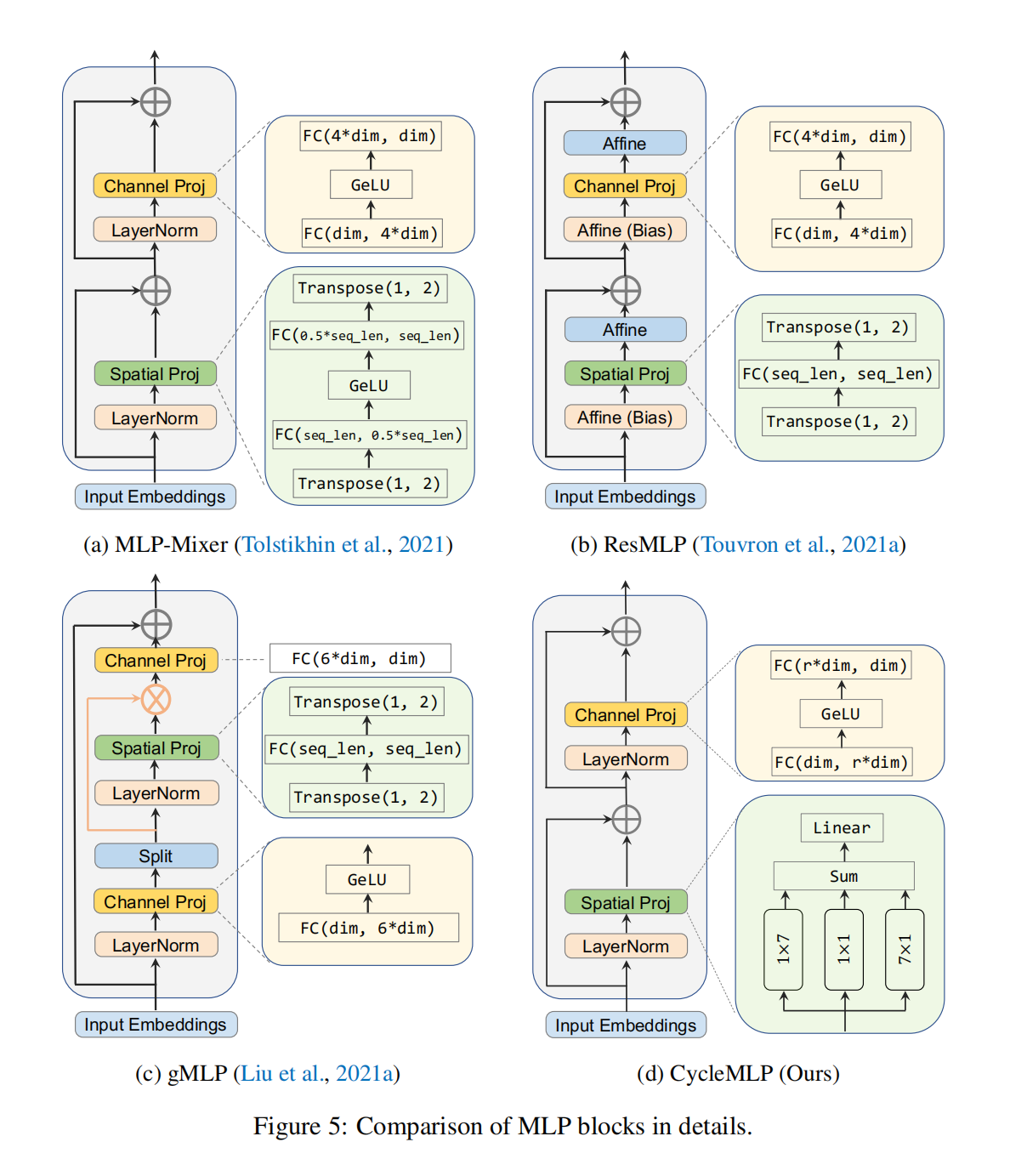 Congested Crowd Instance Localization with Dilated Convolutional Swin Transformer 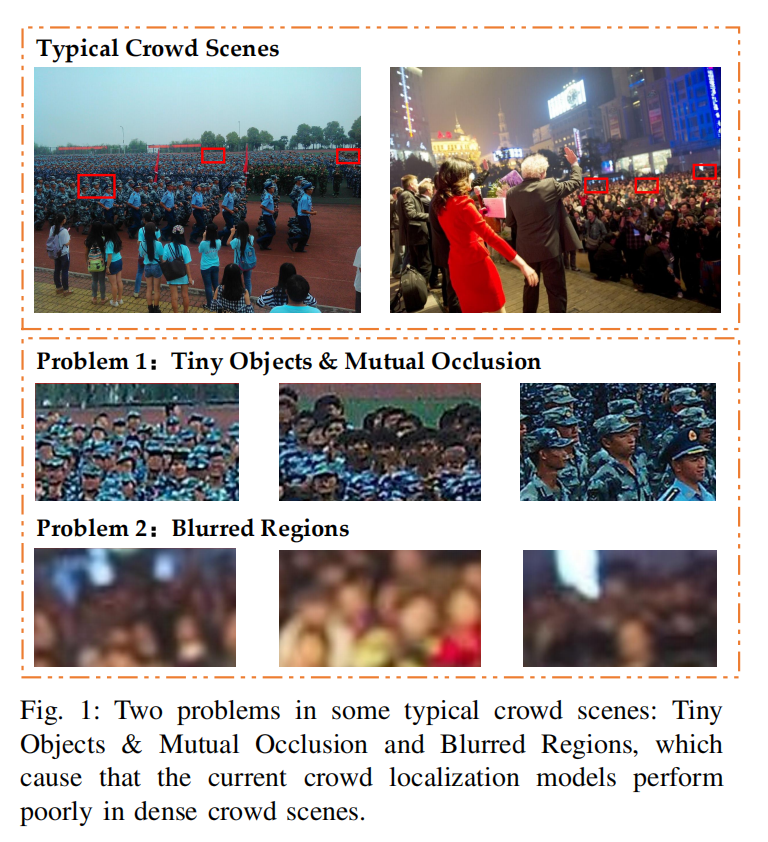 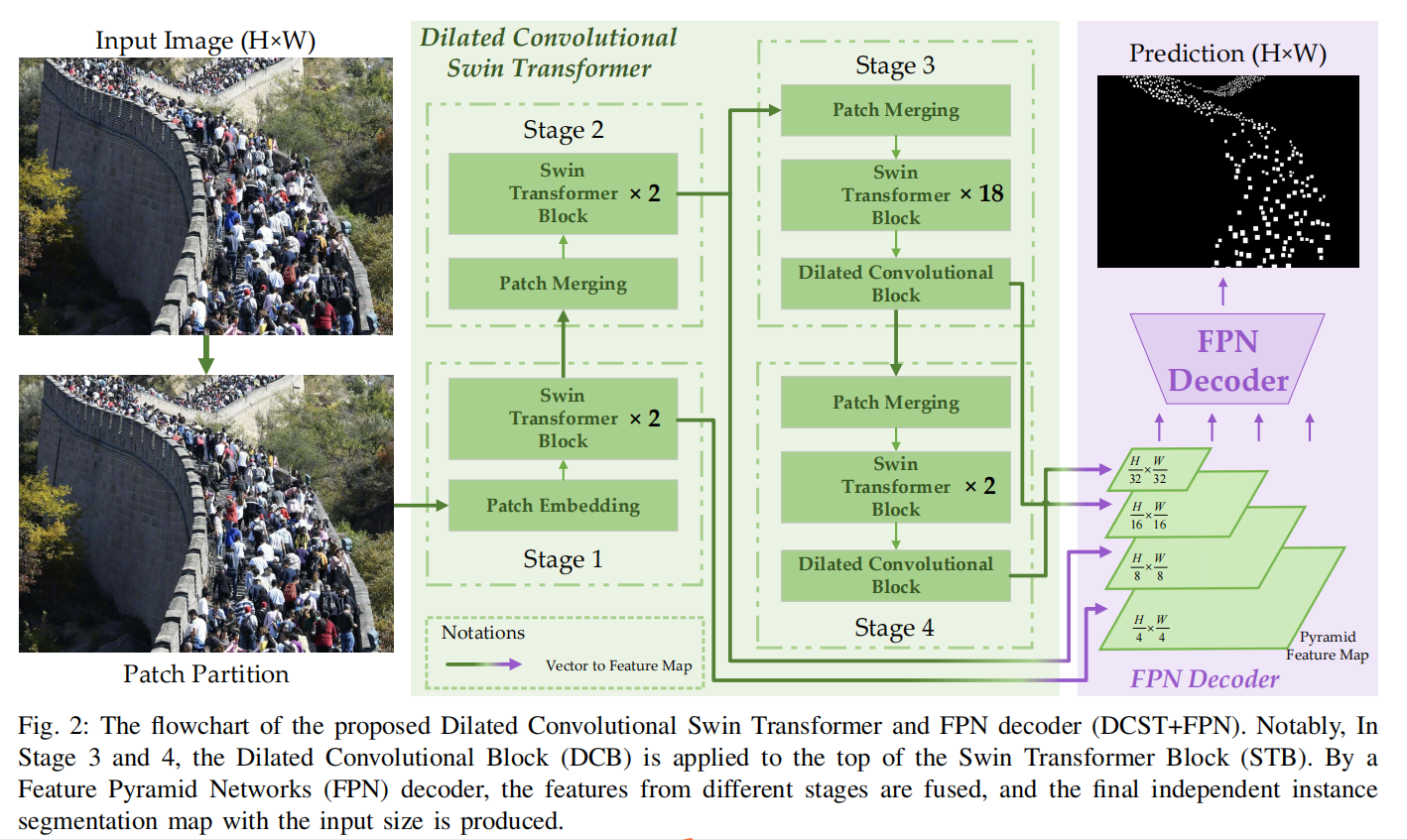 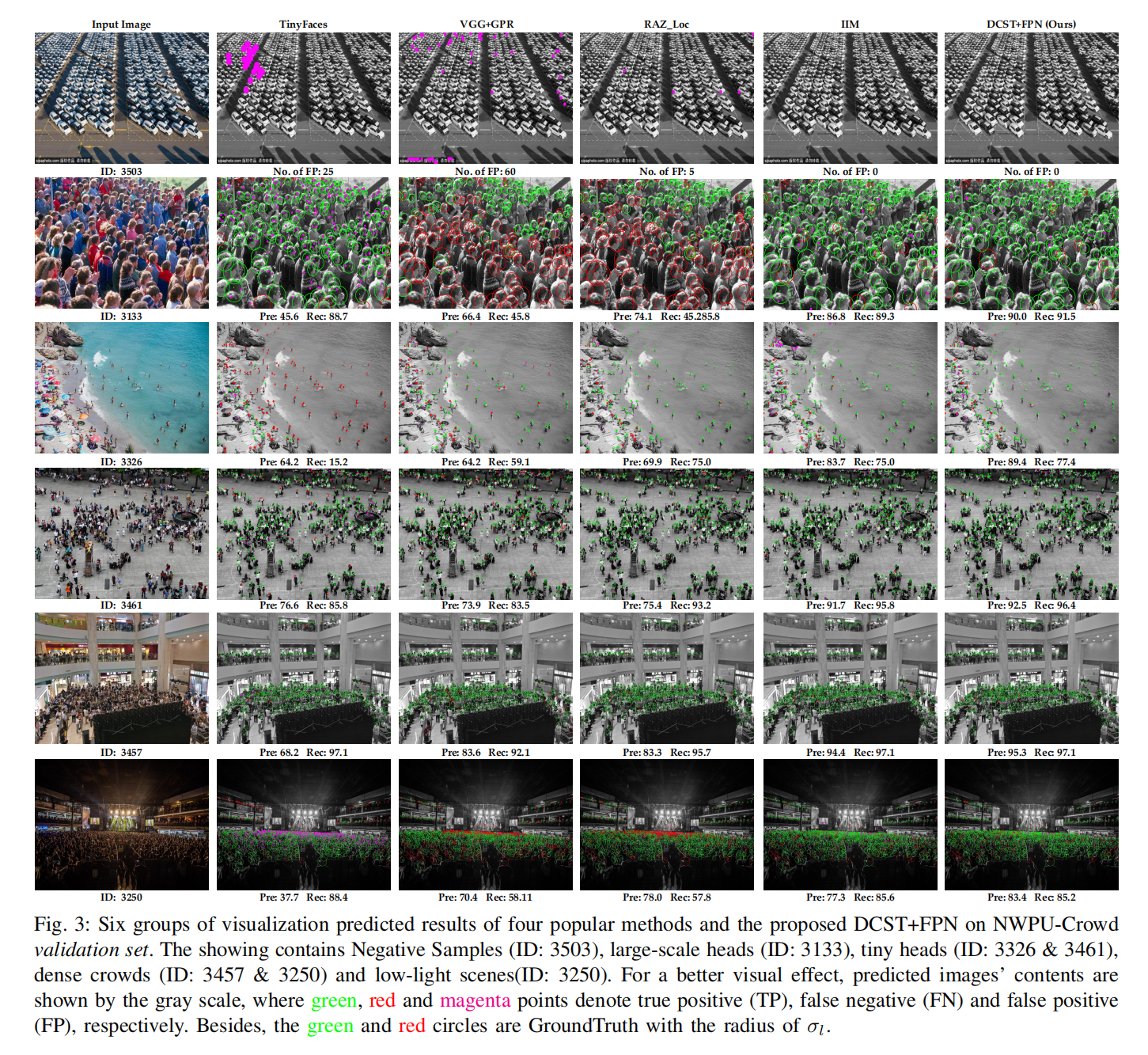
ConvNets vs. Transformers: Whose Visual Representations are More Transferable?
Vision transformers have attracted much attention from computer vision researchers as they are not restricted to the spatial inductive bias of ConvNets. However, although Transformer-based backbones have achieved much progress on ImageNet classification, it is still unclear whether the learned representations are as transferable as or even more transferable than ConvNets’ features. To address this point, we systematically investigate the transfer learning ability of ConvNets and vision transformers in 15 single-task and multi-task performance evaluations. Given the strong correlation between the performance of pretrained models and transfer learning, we include 2 residual ConvNets (i.e., R-101×3 and R-152×4) and 3 Transformer based visual backbones (i.e., ViT-B, ViT-L and Swin-B), which have close error rates on ImageNet, that indicate similar transfer learning performance on downstream datasets. We observe consistent advantages of Transformer-based backbones on 13 downstream tasks (out of 15), including but not limited to fine-grained classification, scene recognition (classification, segmentation and depth estimation), open-domain classification, face recognition, etc. More specifically, we find that two ViT models heavily rely on whole network fine-tuning to achieve performance gains while Swin Transformer does not have such a requirement. Moreover, vision transformers behave more robustly in multi-task learning, i.e., bringing more improvements when managing mutually beneficial tasks and reducing performance losses when tackling irrelevant tasks. We hope our discoveries can facilitate the exploration and exploitation of vision transformers in the future. 视觉变压器因其不局限于卷积神经网络的空间感应偏置而受到计算机视觉研究者的广泛关注。然而,尽管基于transformer的主干网在ImageNet分类方面取得了很大的进展,但我们仍然不清楚学习后的表示是否和卷积网络的特征一样可转移,甚至比卷积网络的特征更可转移。为了解决这一问题,我们在15个单任务和多任务性能评估中系统地研究了卷积神经网络和视觉变压器的迁移学习能力。考虑到预训练模型的性能与迁移学习之间的强相关性,我们包括2个残差ConvNets (R-101×3和R-152×4)和3个基于Transformer的视觉主干(vi - b、vi - l和swi - b),它们在ImageNet上的错误率接近,表明在下游数据集上的迁移学习性能类似。我们观察到基于transformer的骨干在13个下游任务(15个任务中)上具有一致的优势,包括但不限于细粒度分类、场景识别(分类、分割和深度估计)、开放域分类、人脸识别等。更具体地说,我们发现两个ViT模型严重依赖于整个网络的微调来实现性能增益,而Swin Transformer没有这样的需求。此外,视觉变压器在多任务学习中表现得更加稳健,即在管理互惠任务时带来更多的改进,在处理无关任务时减少性能损失。我们希望我们的发现可以促进未来视觉变压器的探索和开发。 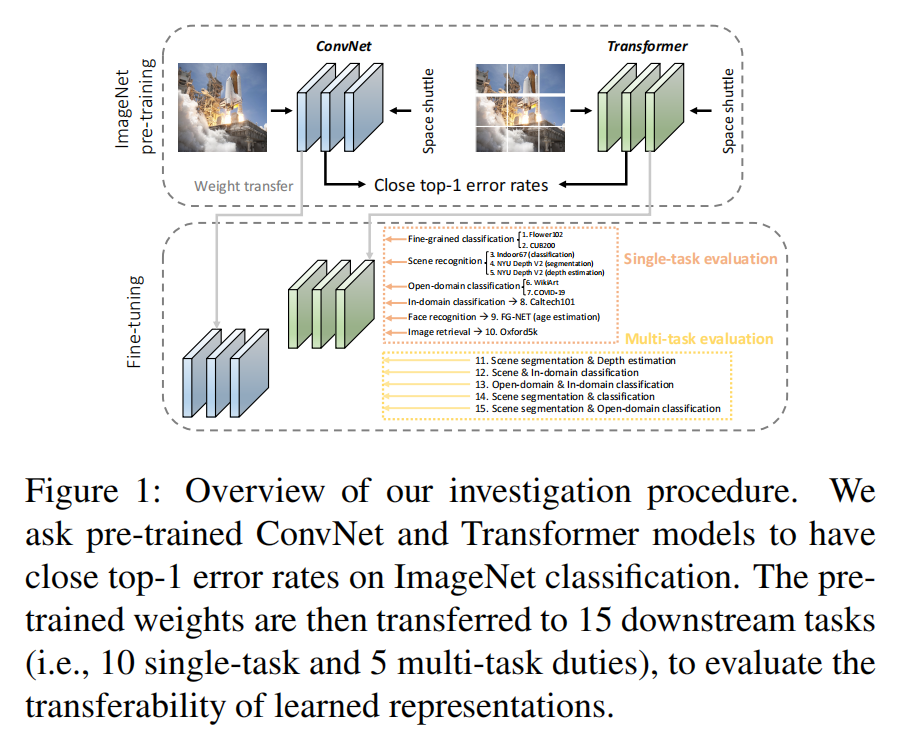 SwinIR: Image Restoration Using Swin Transformer(重点是残差链接) 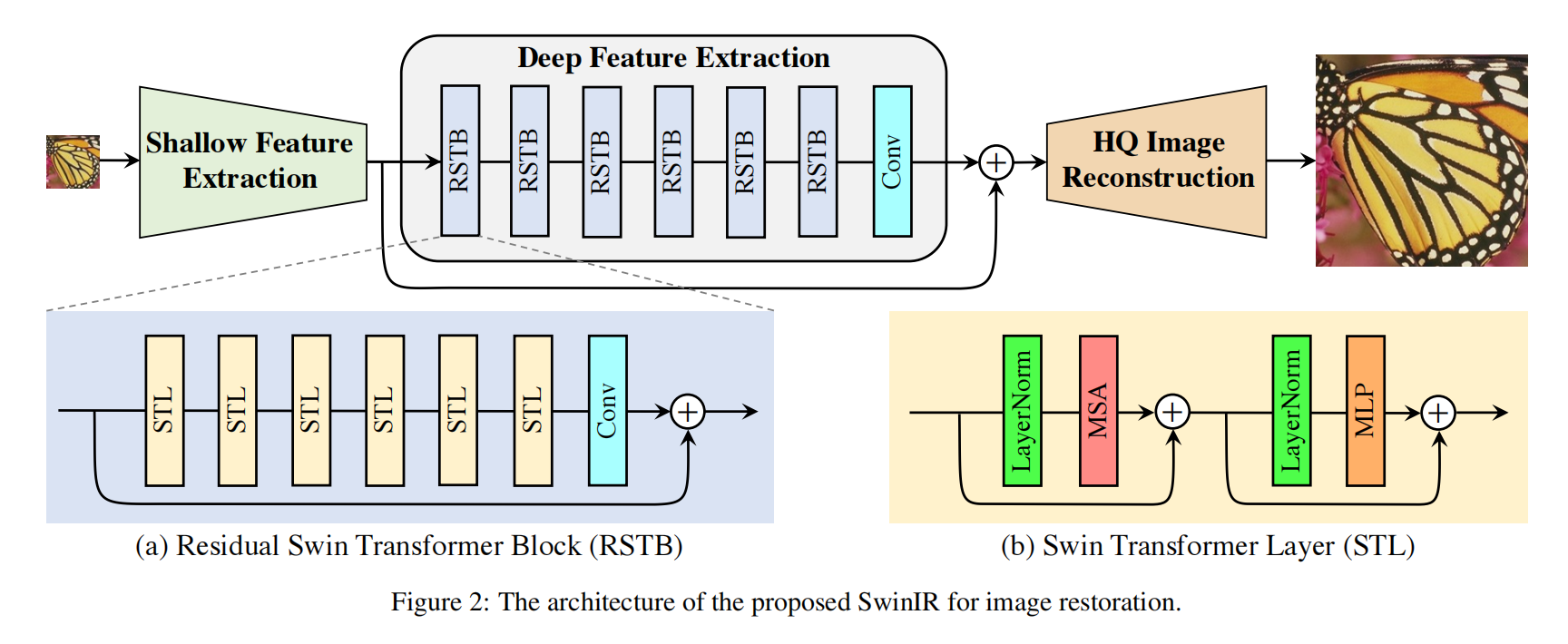 Sparse MLP for Image Recognition: Is Self-Attention Really Necessary?
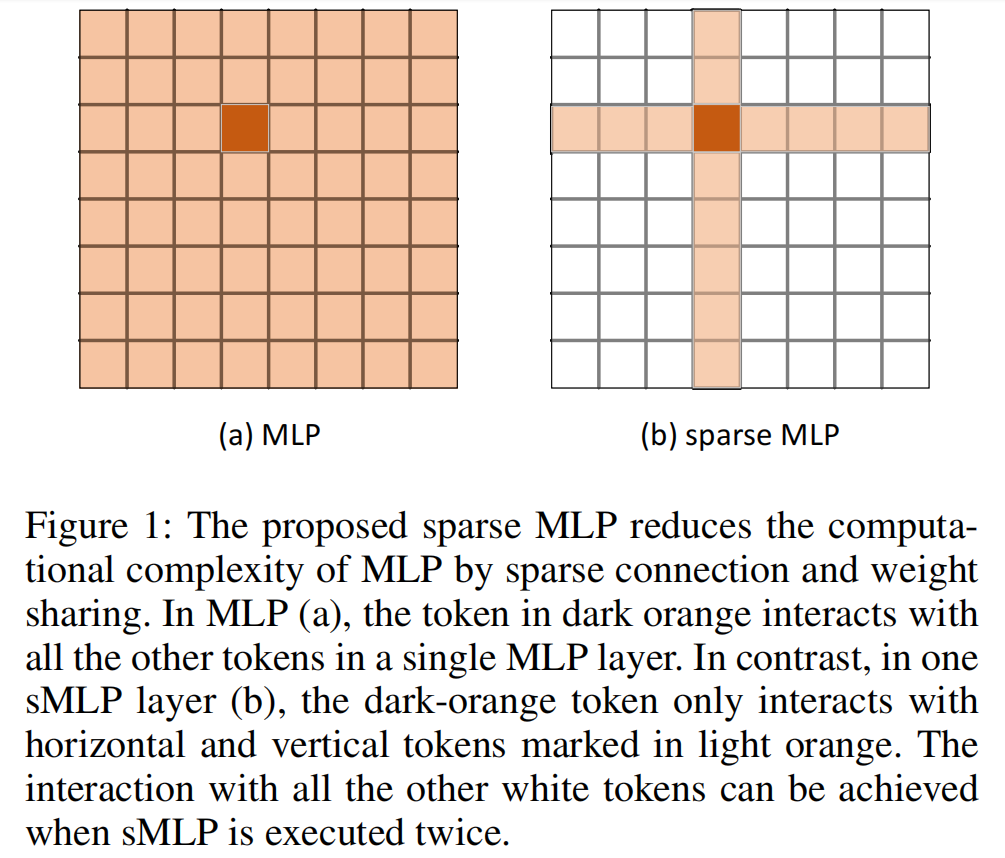 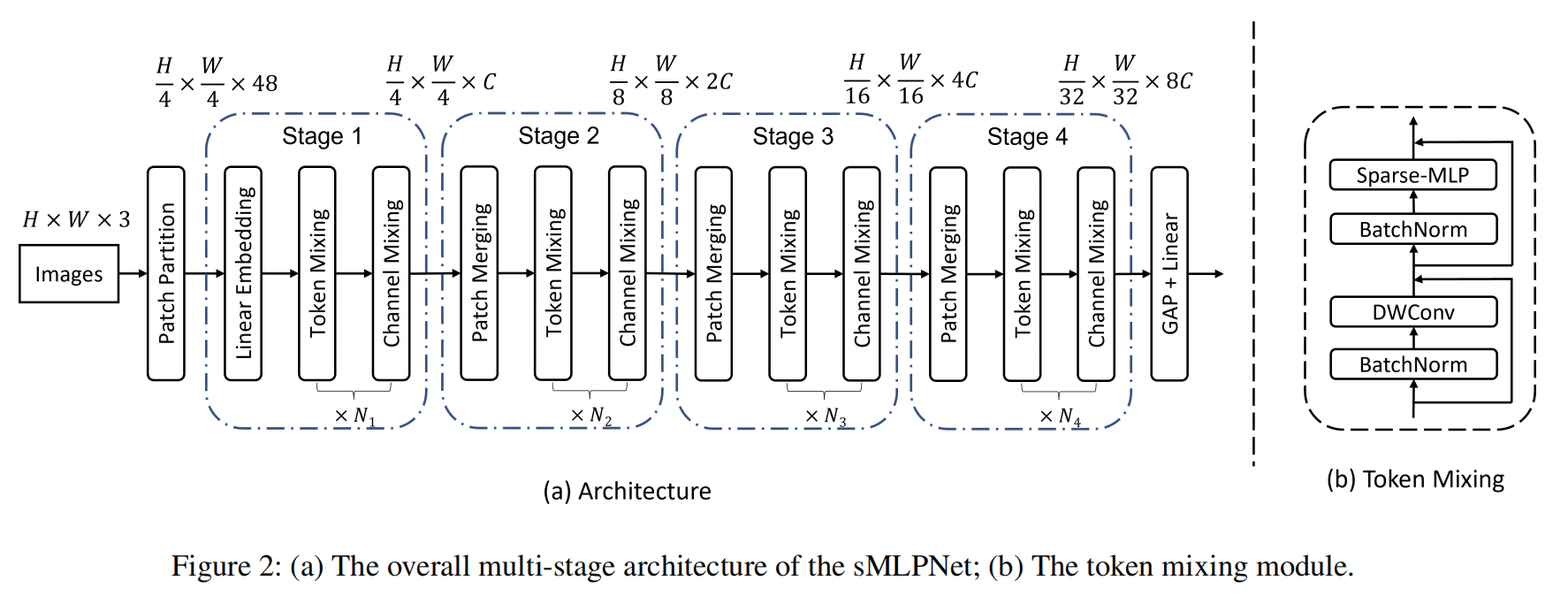 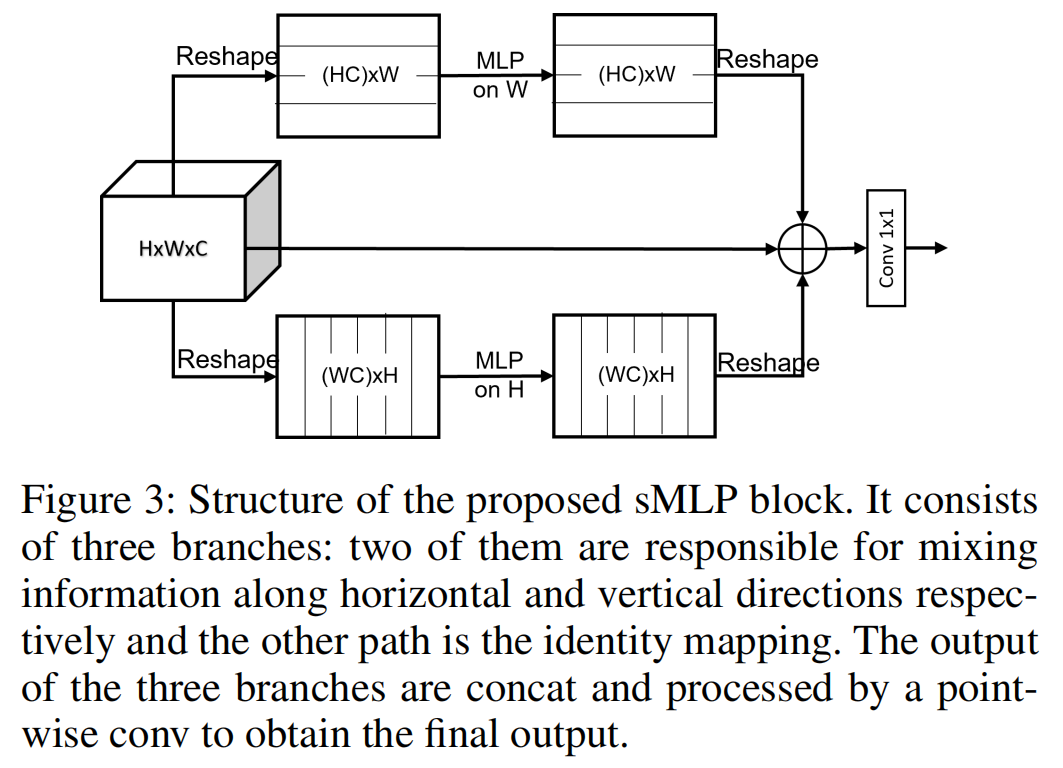 Semi-Supervised Wide-Angle Portraits Correction by Multi-Scale Transformer 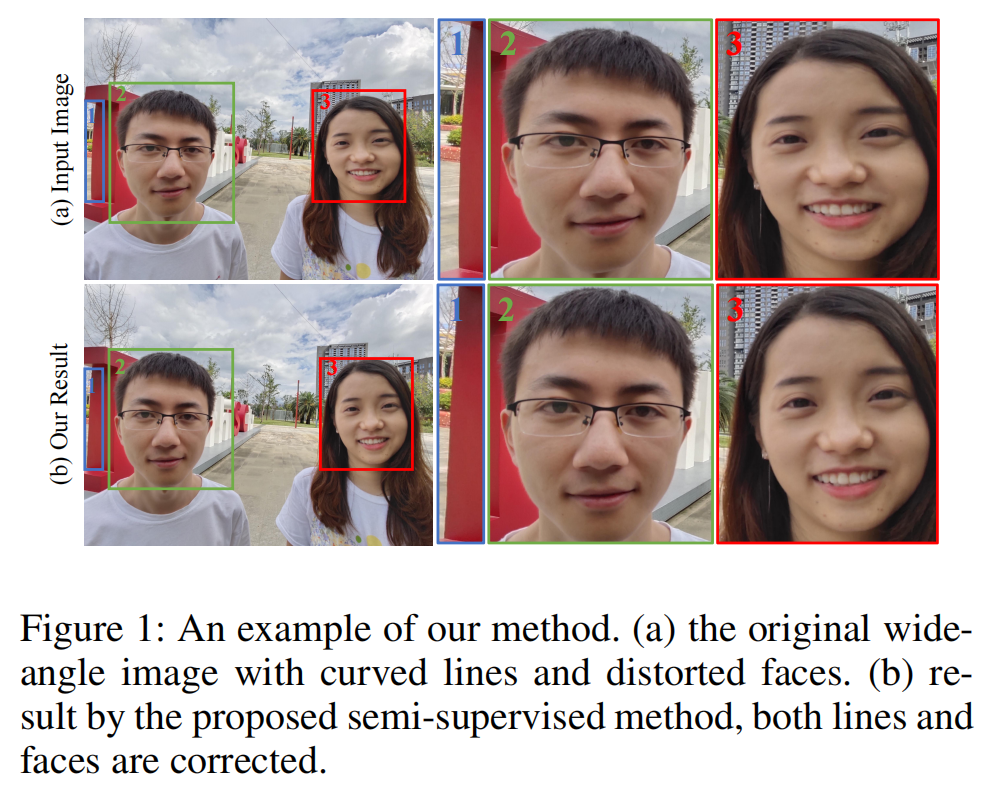 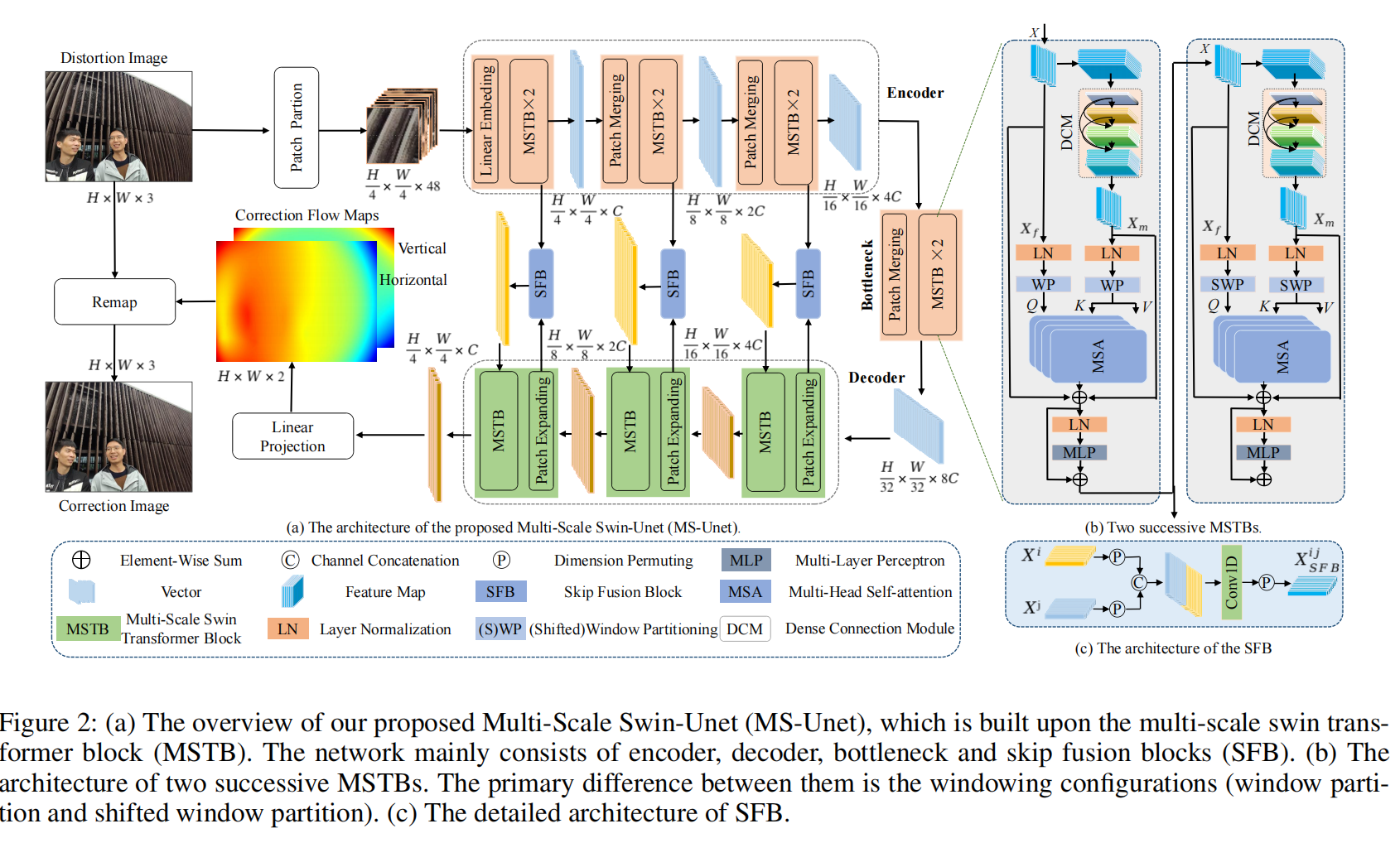 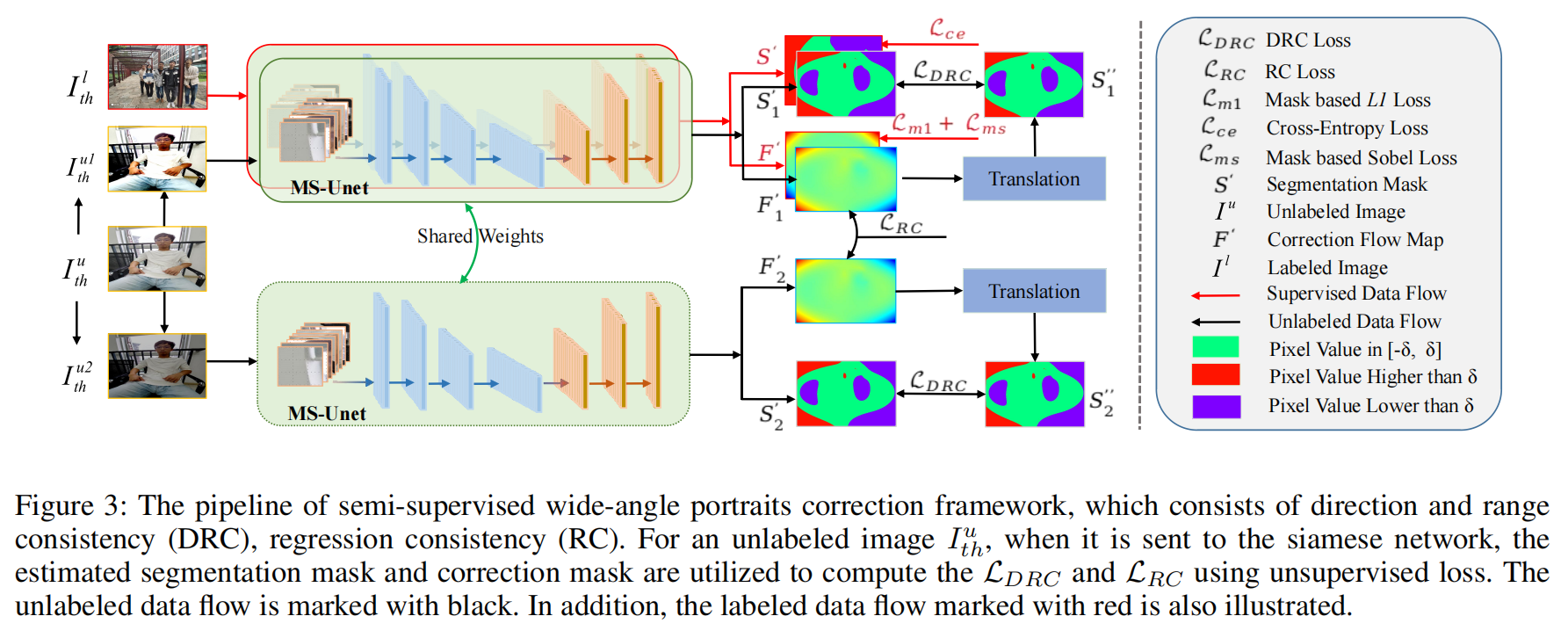 3rd Place Scheme on Instance Segmentation Track of ICCV 2021 VIPriors Challenges 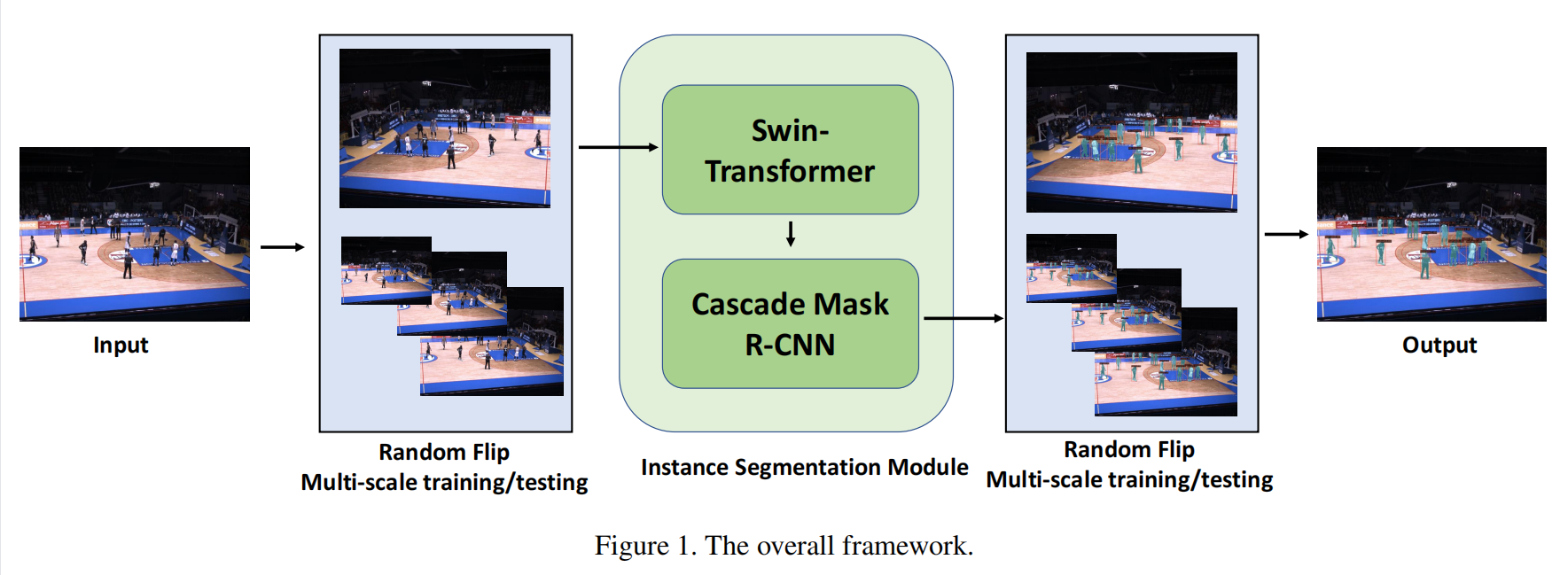 VIDT: AN EFFICIENT AND EFFECTIVE FULLY TRANSFORMER-BASED OBJECT DETECTOR 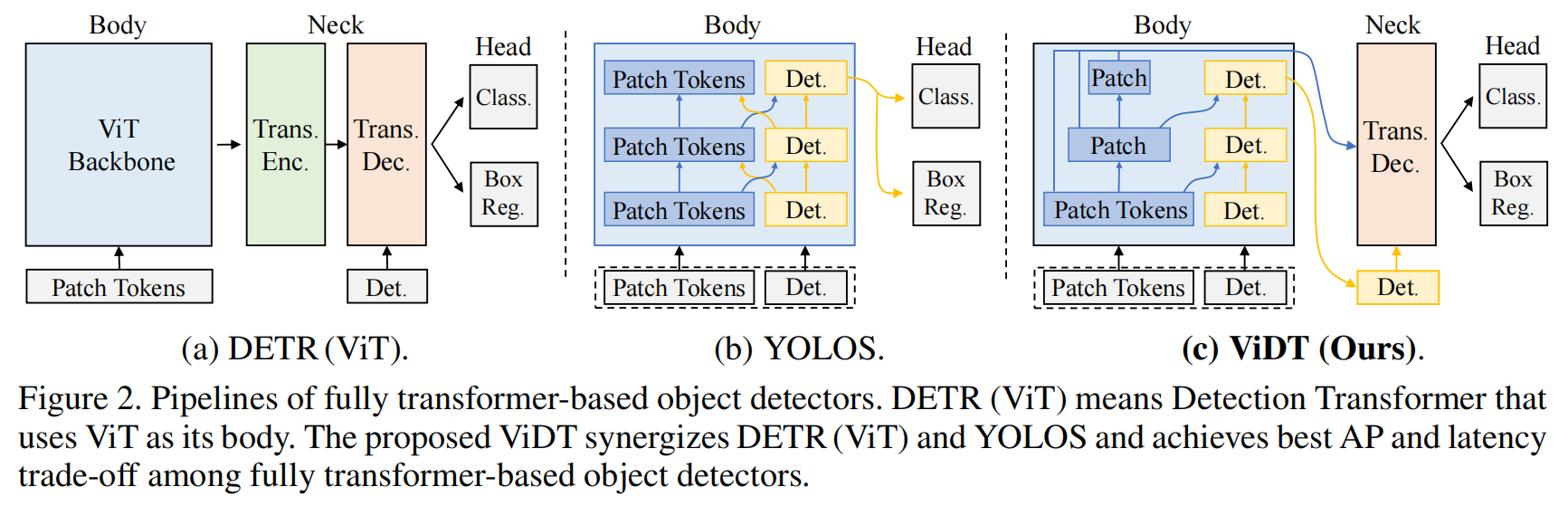 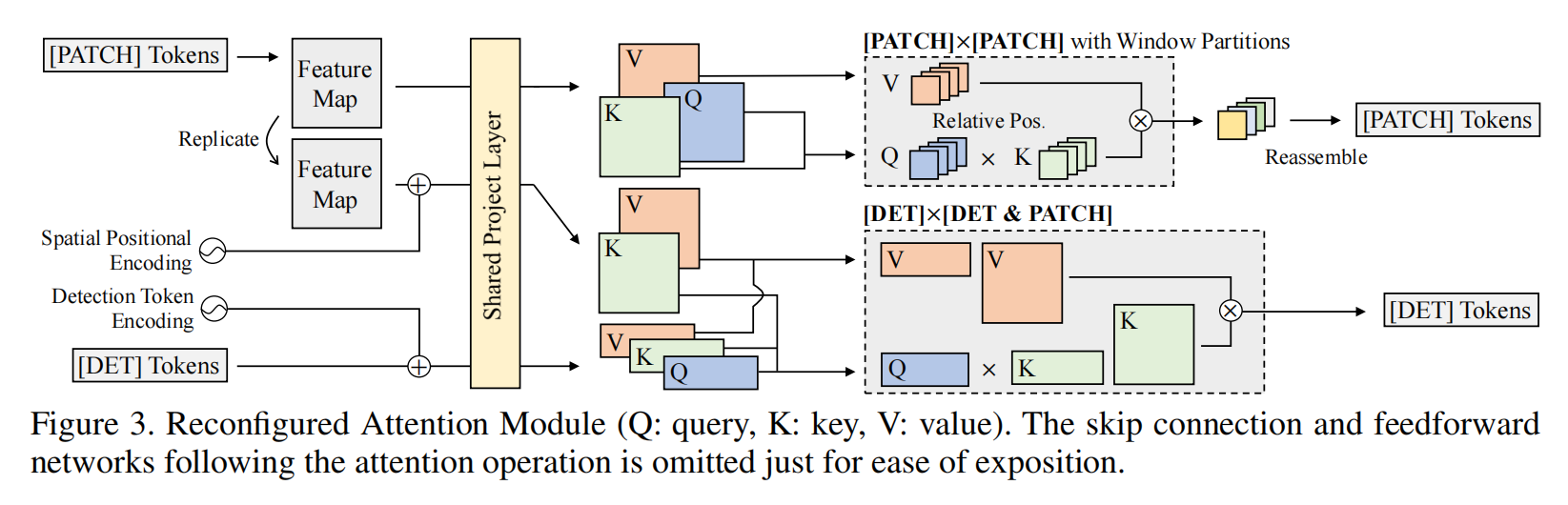 Satellite Image Semantic Segmentation(卫星图像语义分割)(手稿) COVID-19 Detection in Chest X-ray Images Using Swin Transformer and Transformer in Transformer 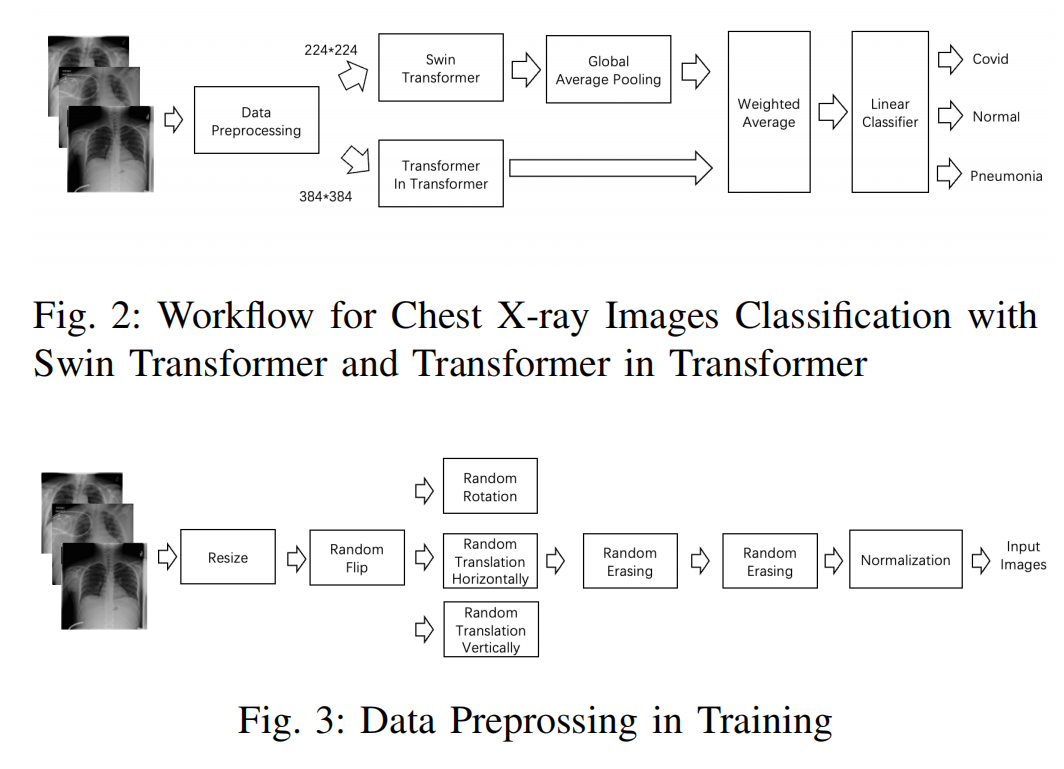 HRFormer: High-Resolution Transformer for Dense Prediction 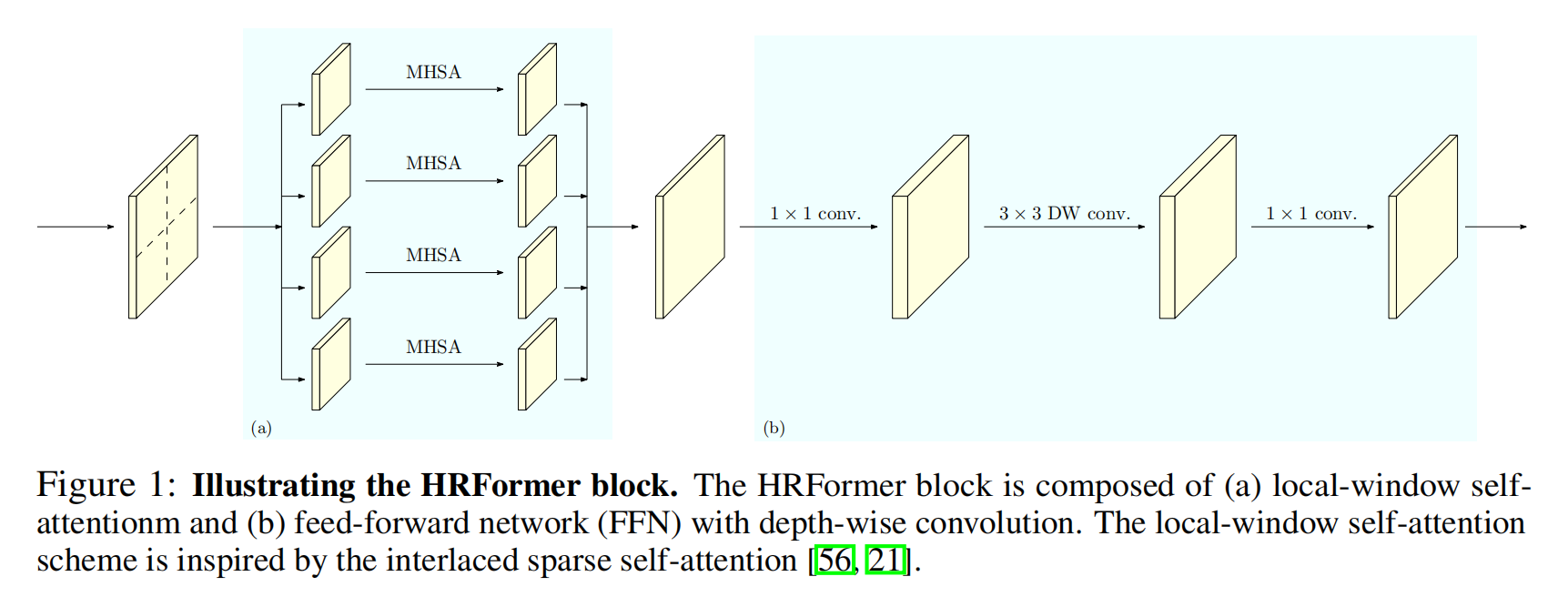 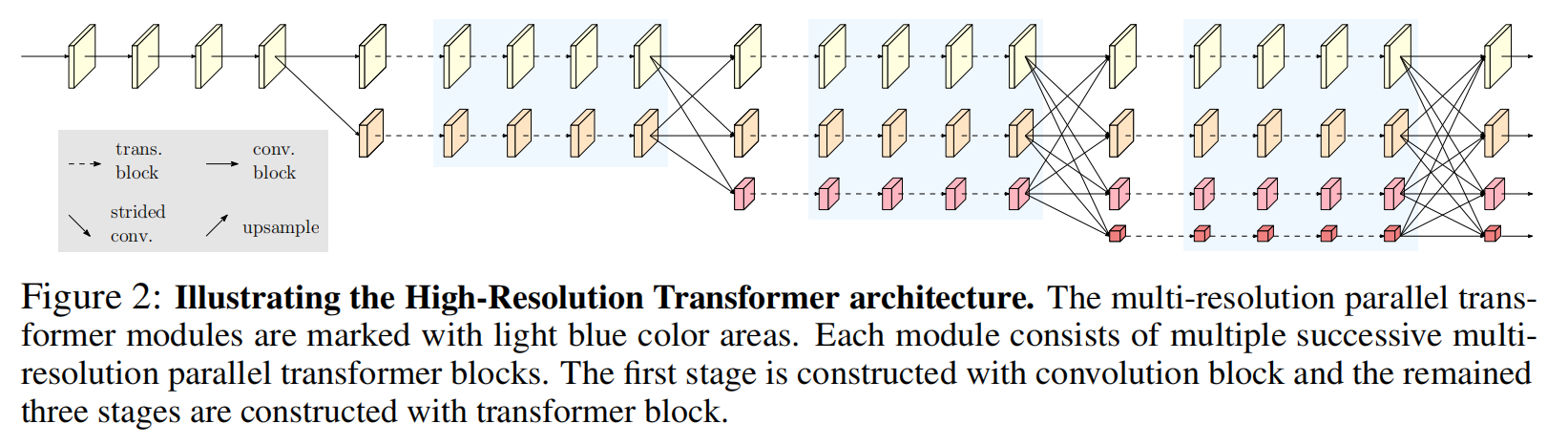 Vis-TOP: Visual Transformer Overlay Processor 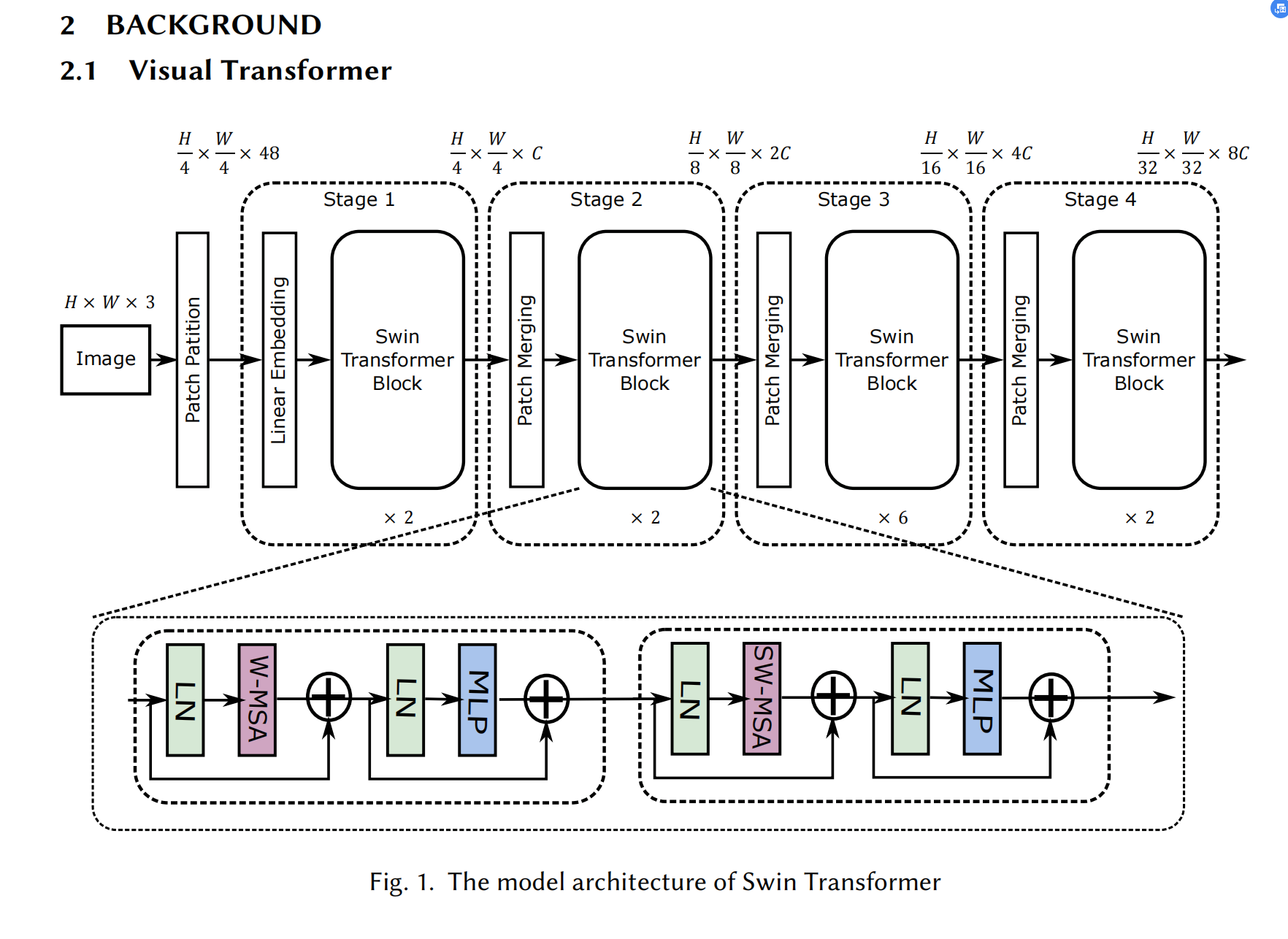 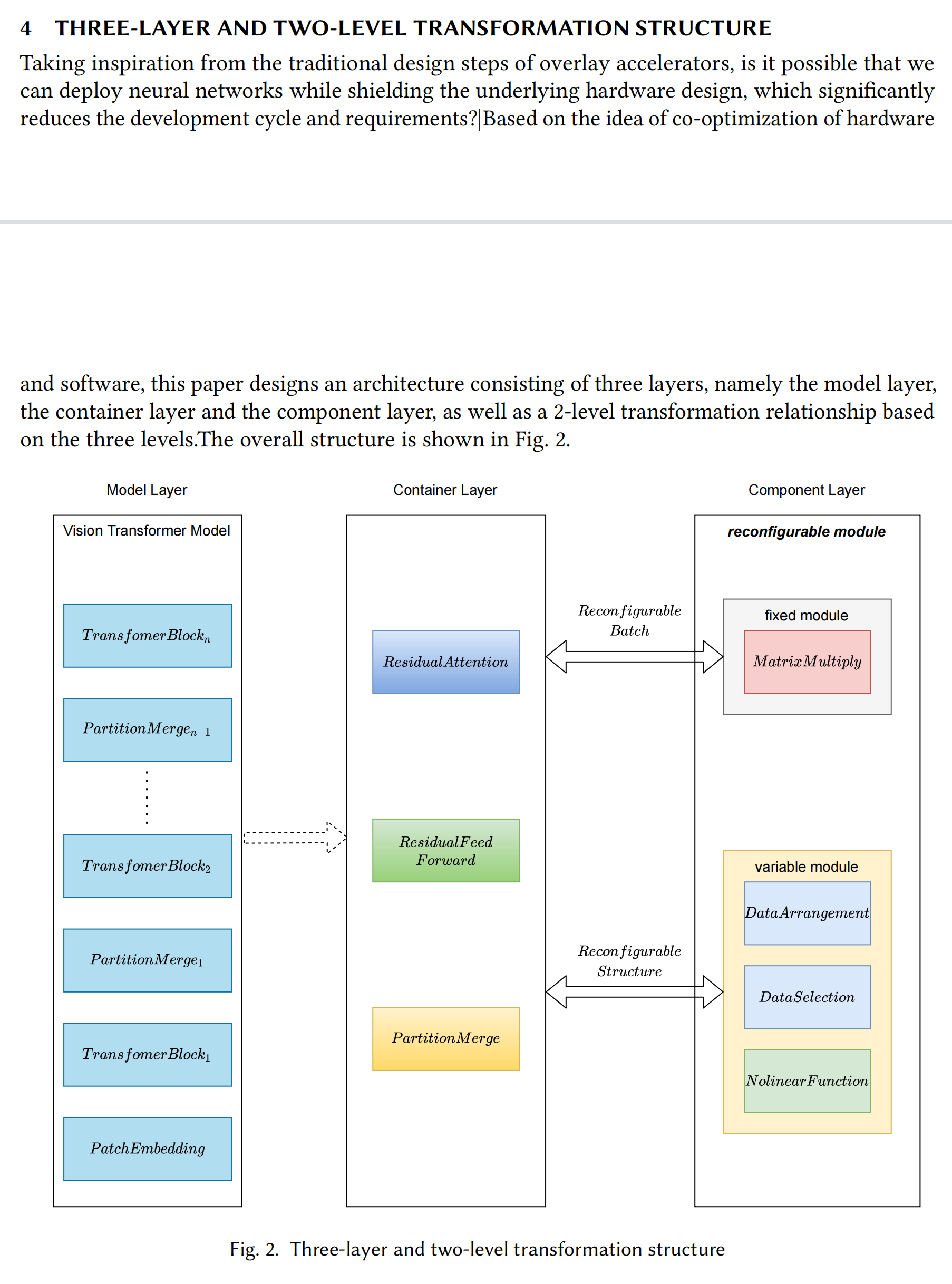 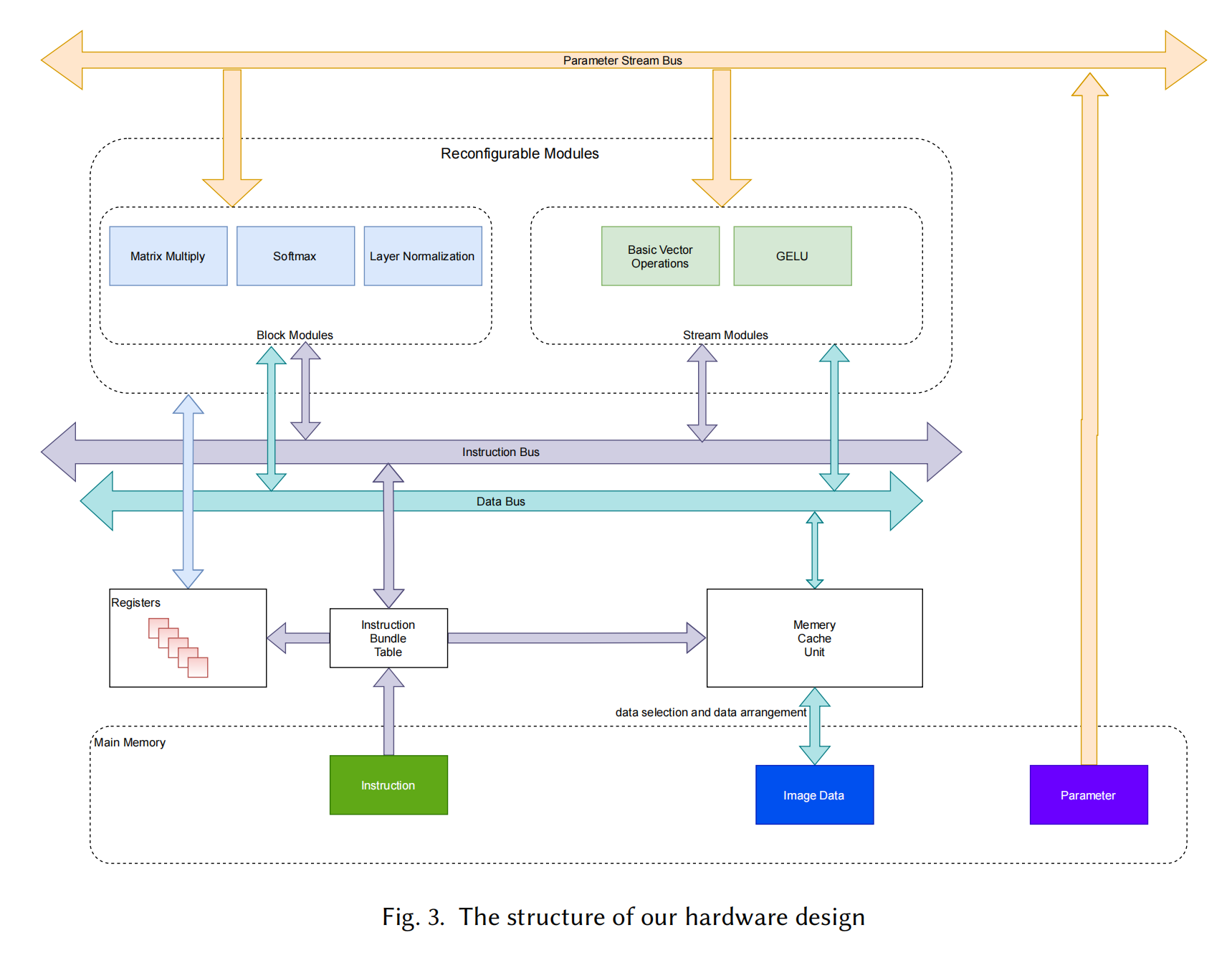 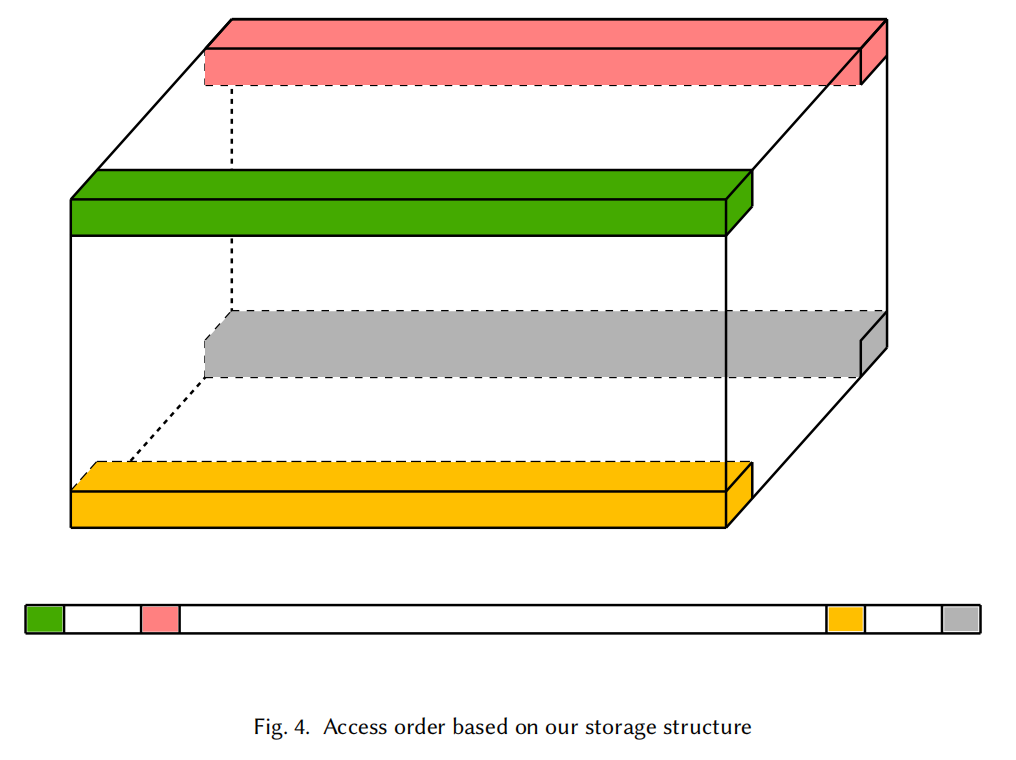 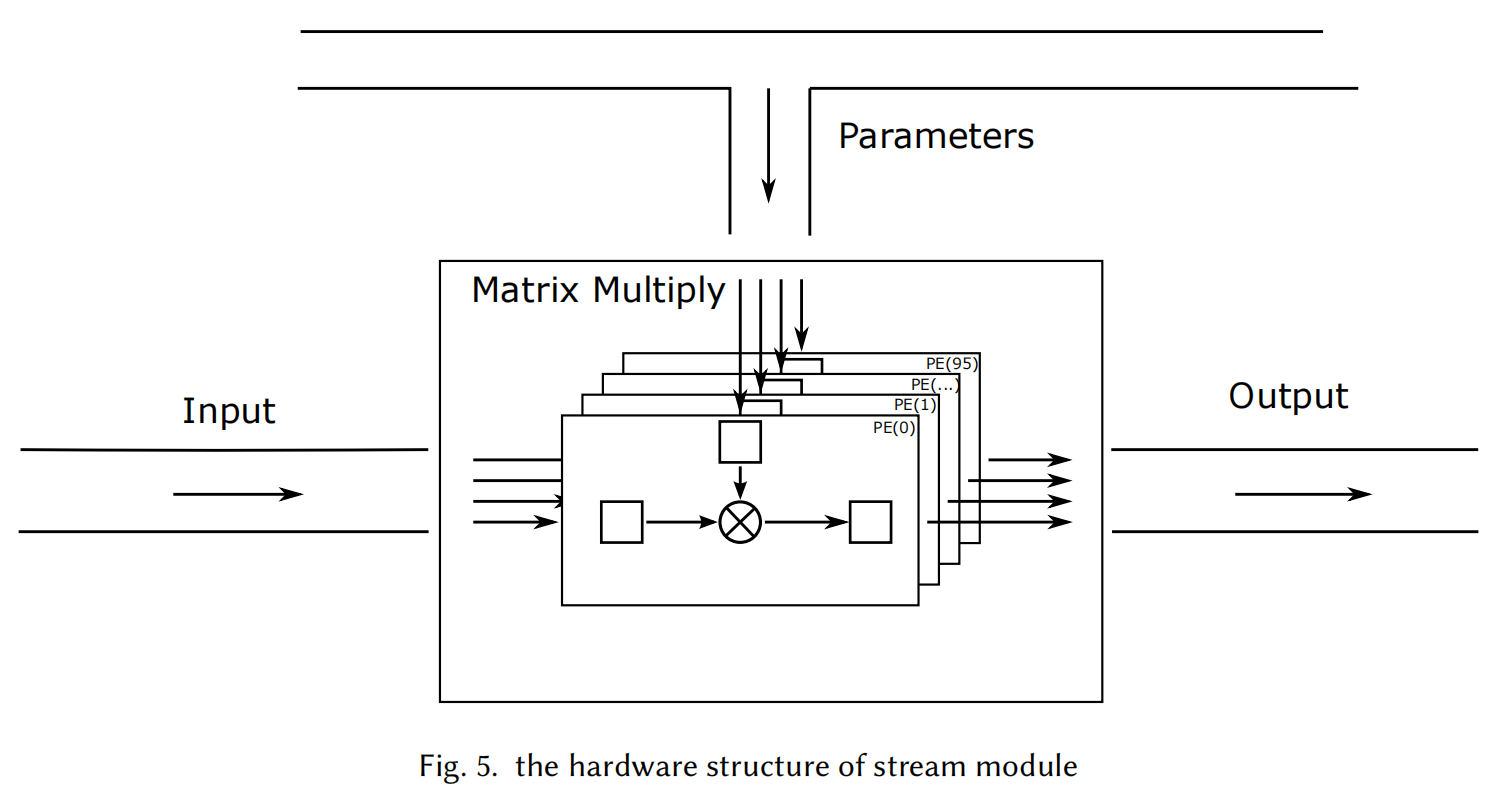 Hepatic vessel segmentation based on 3D swin-transformer with inductive biased multi-head self-attention 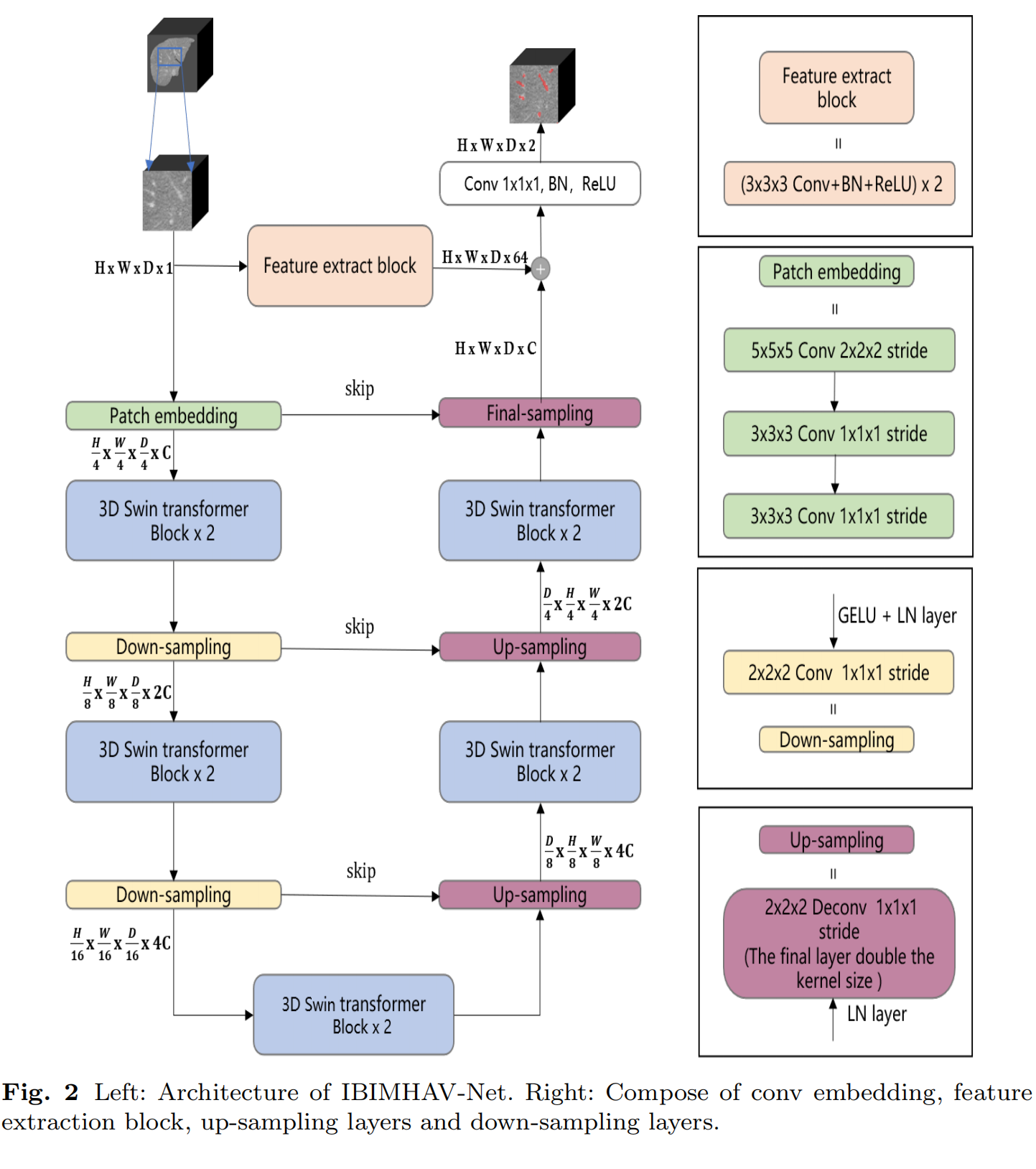 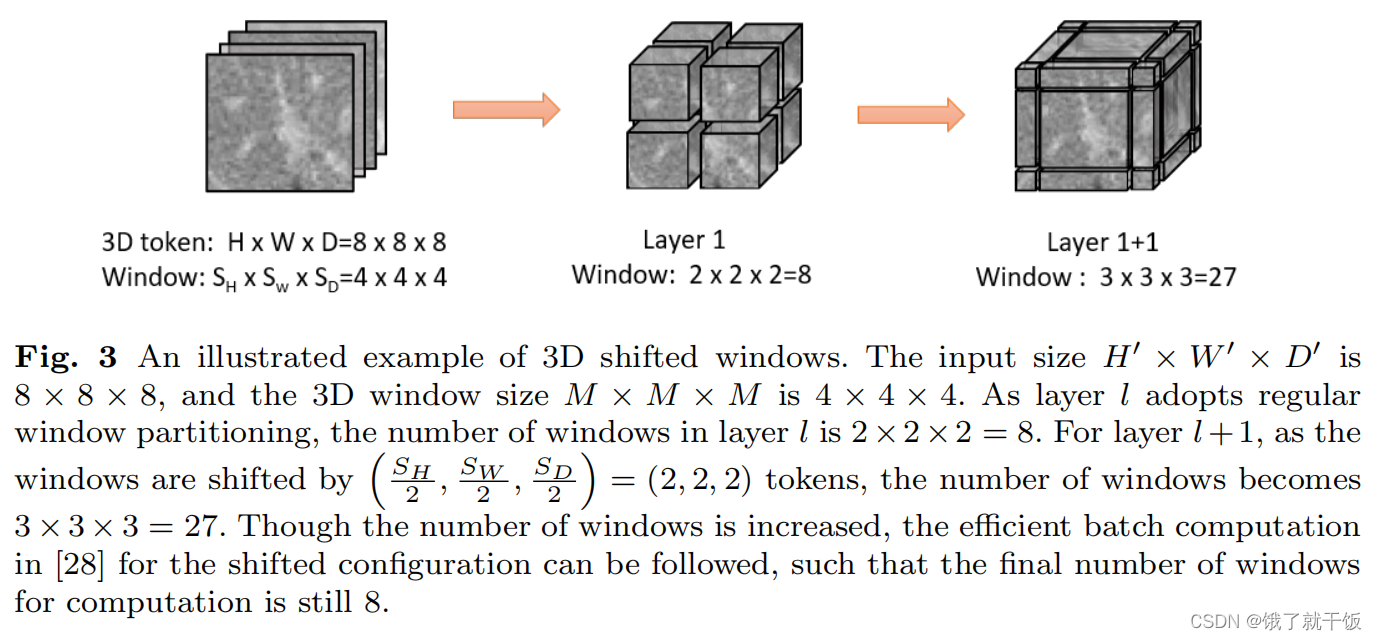 Transformer-based Image Compression(图像压缩) 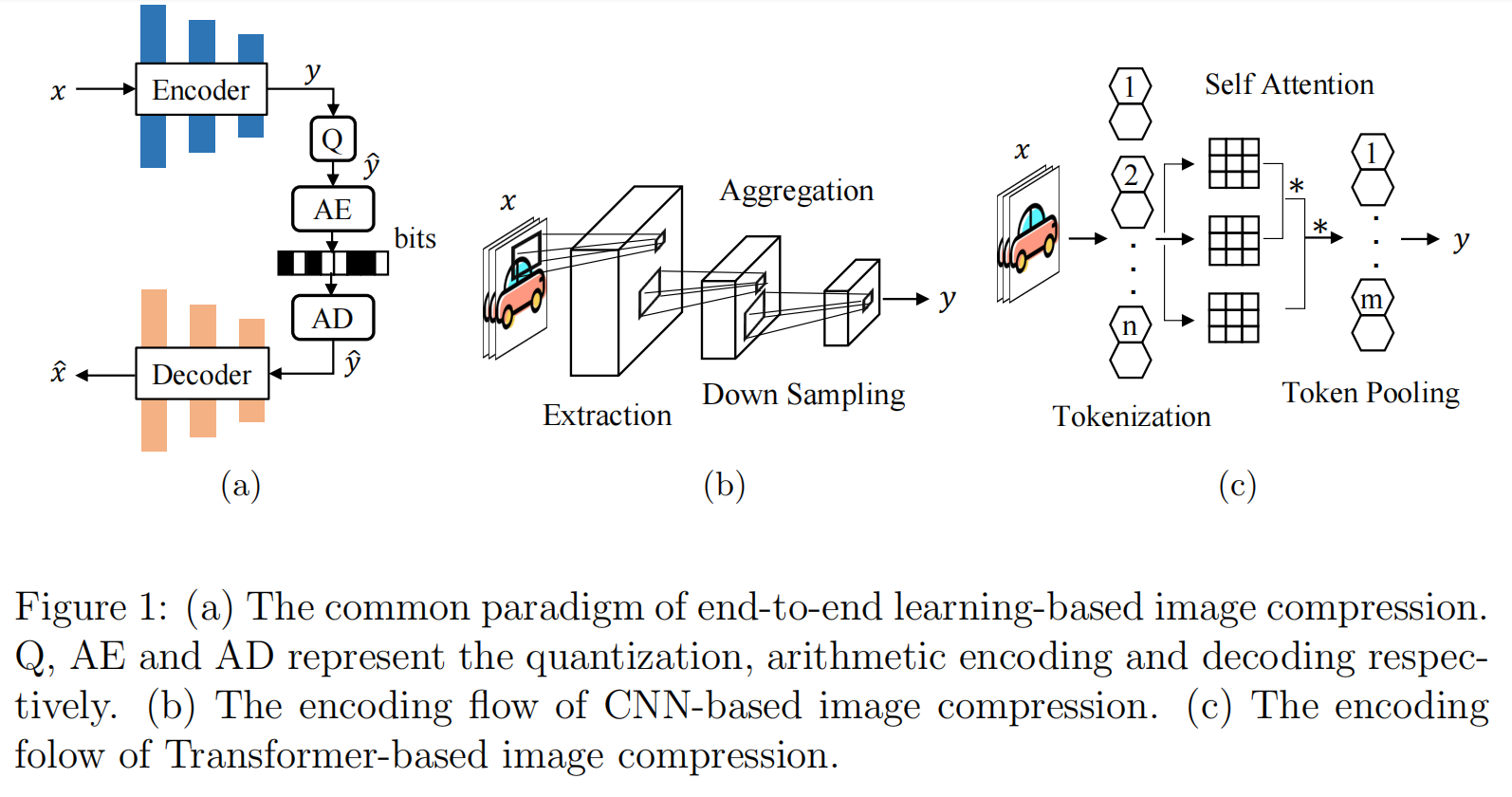 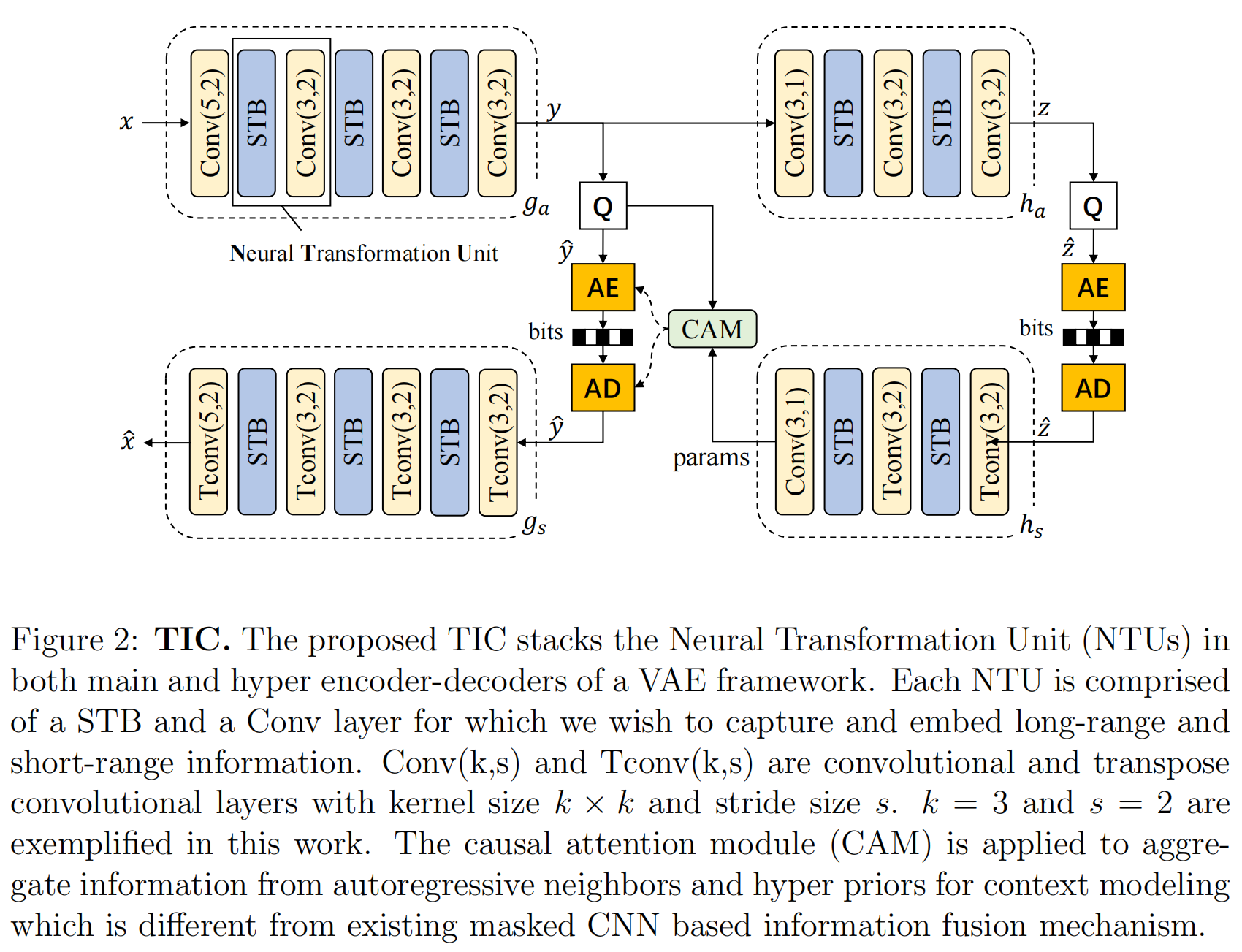 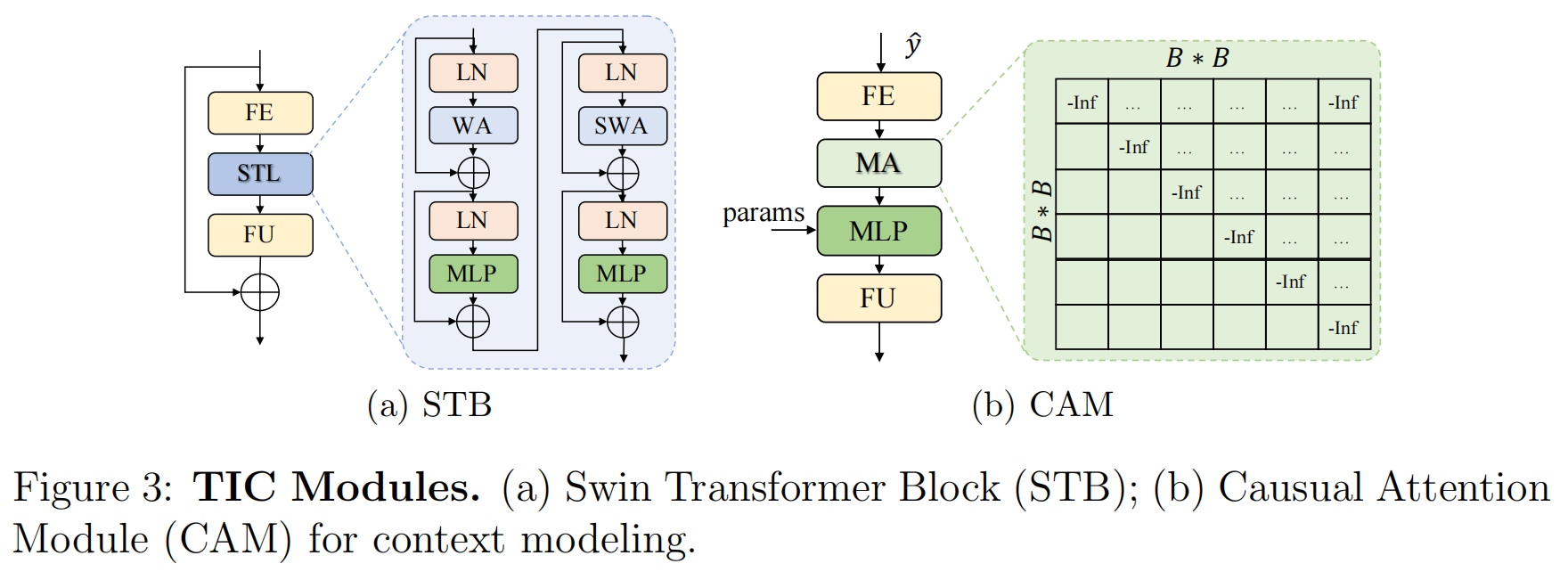 Swin Transformer V2: Scaling Up Capacity and Resolution 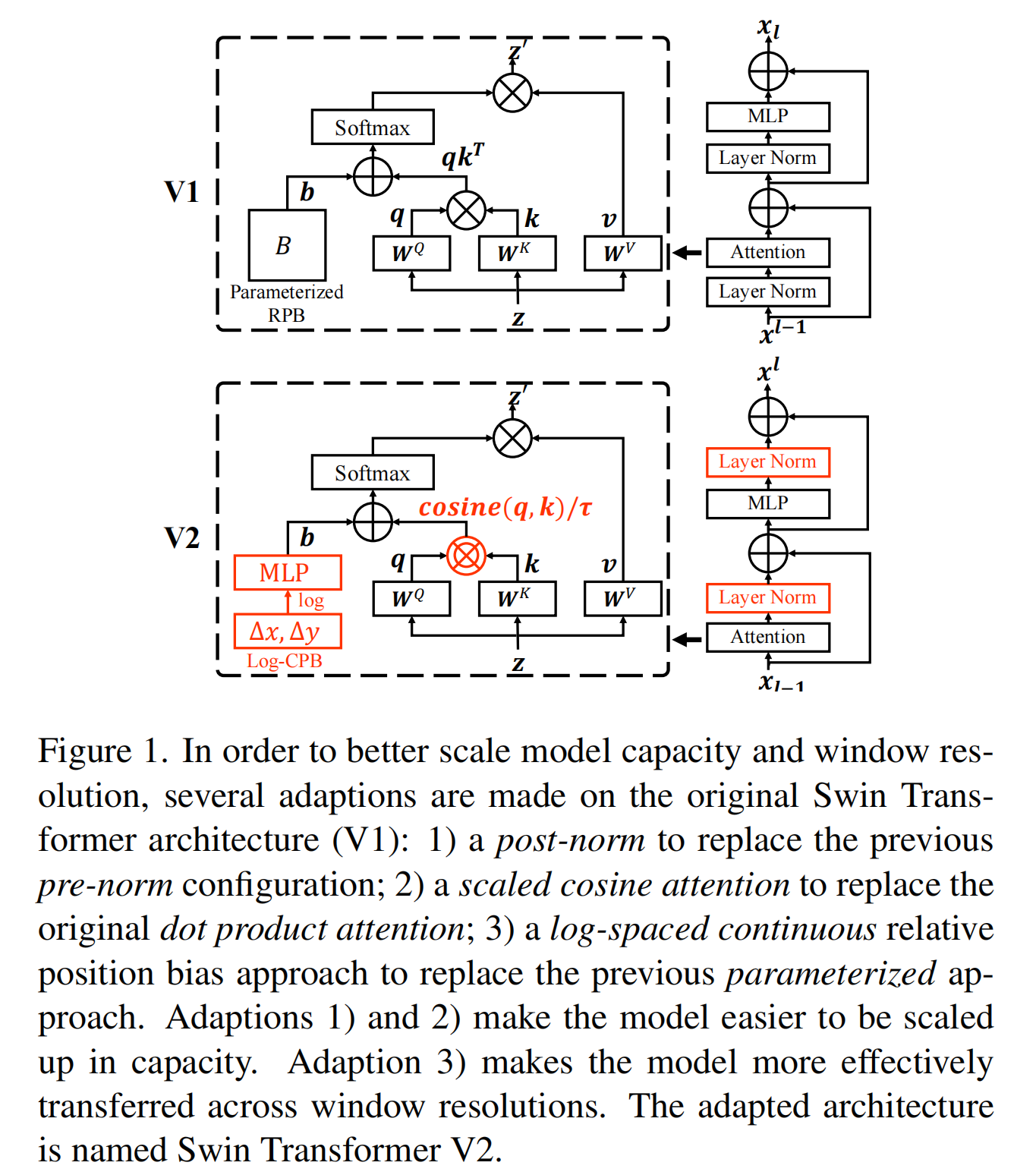 Vision Transformer with Deformable Attention 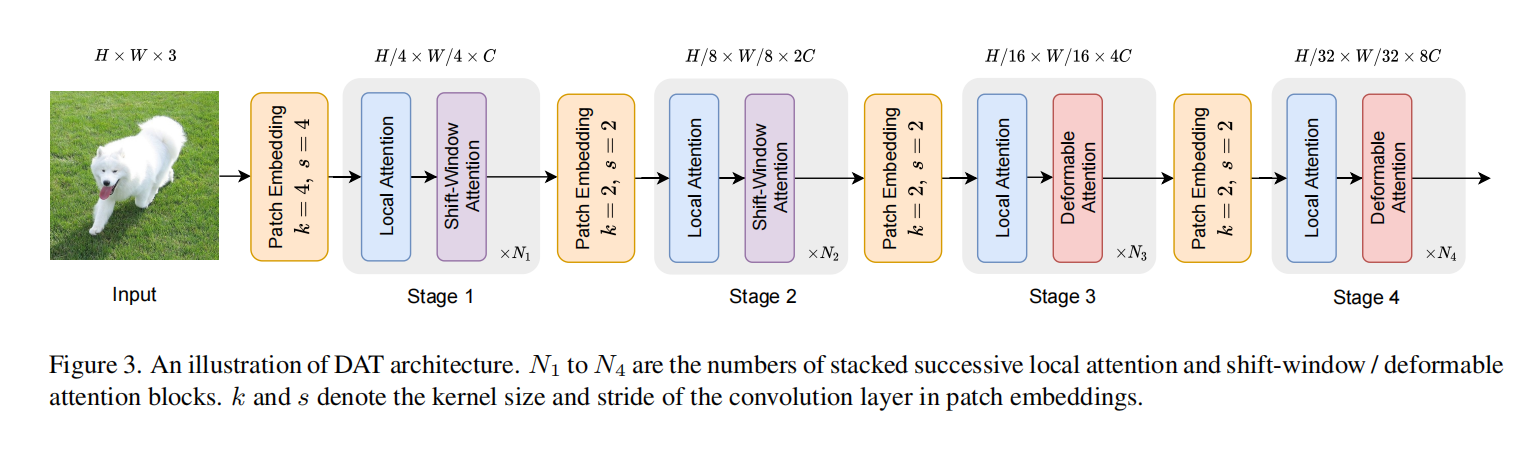 Swin UNETR: Swin Transformers for Semantic Segmentation of Brain Tumors in MRI Images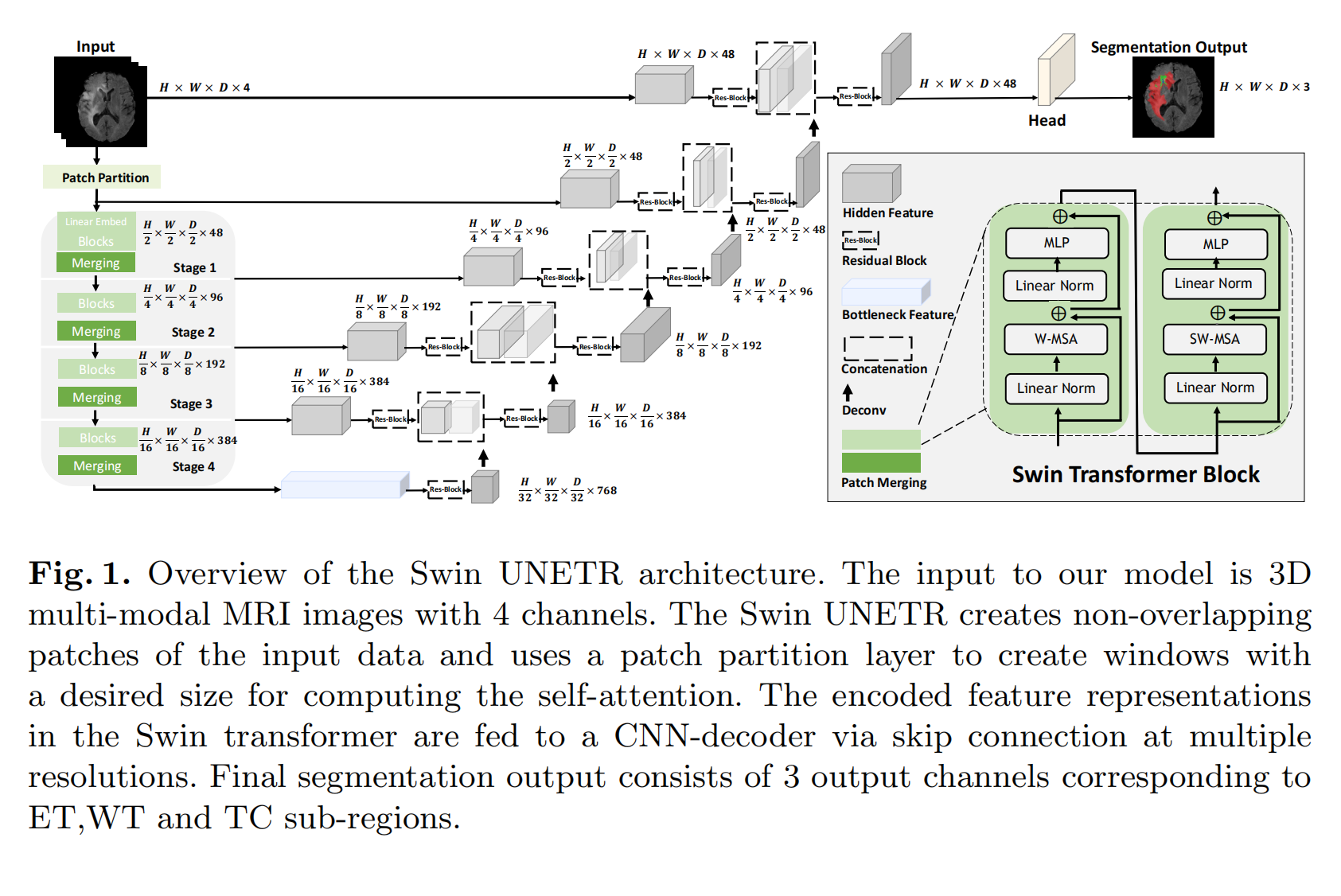
SWIN-POSE: SWIN TRANSFORMER BASED HUMAN POSE ESTIMATION
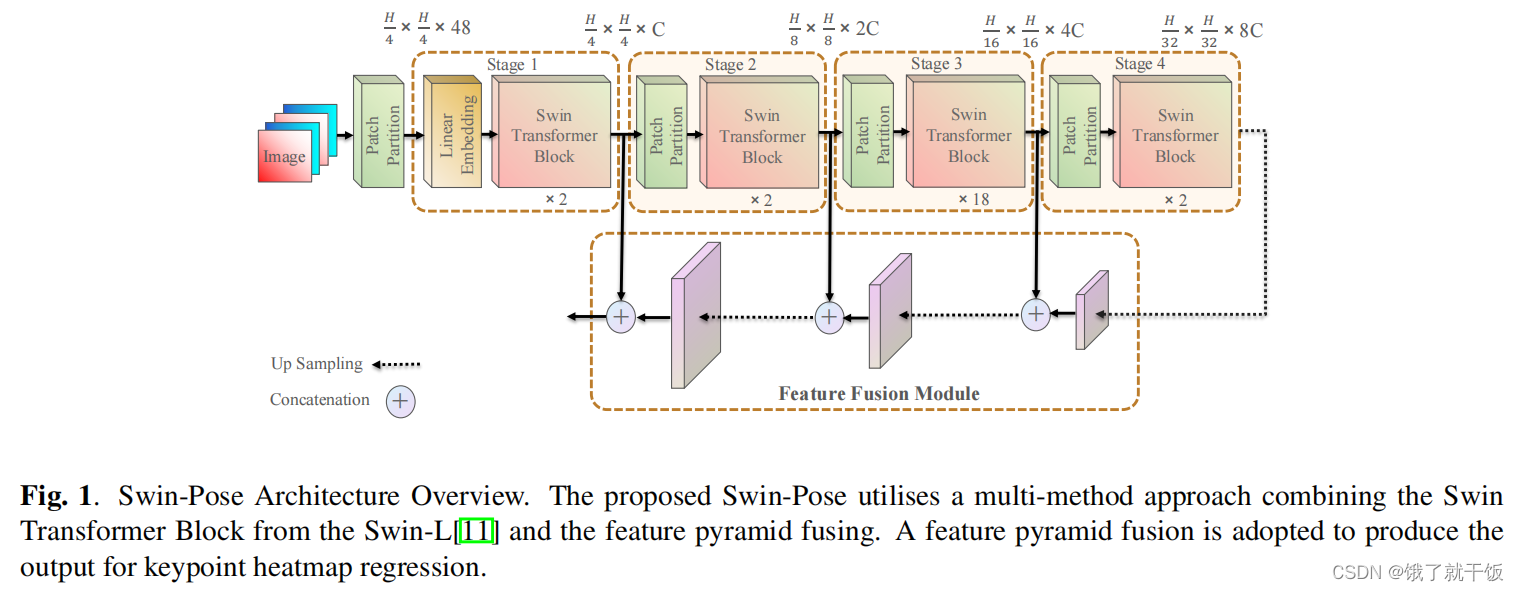 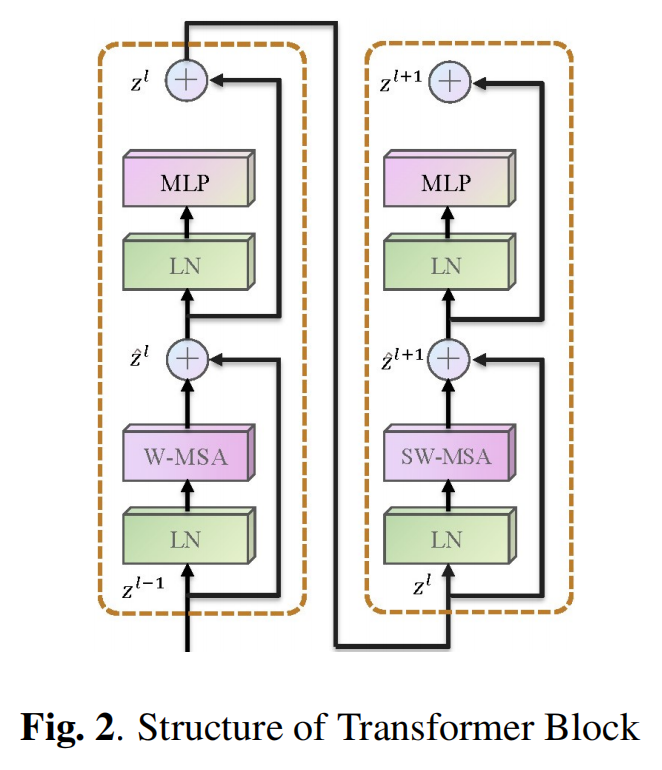 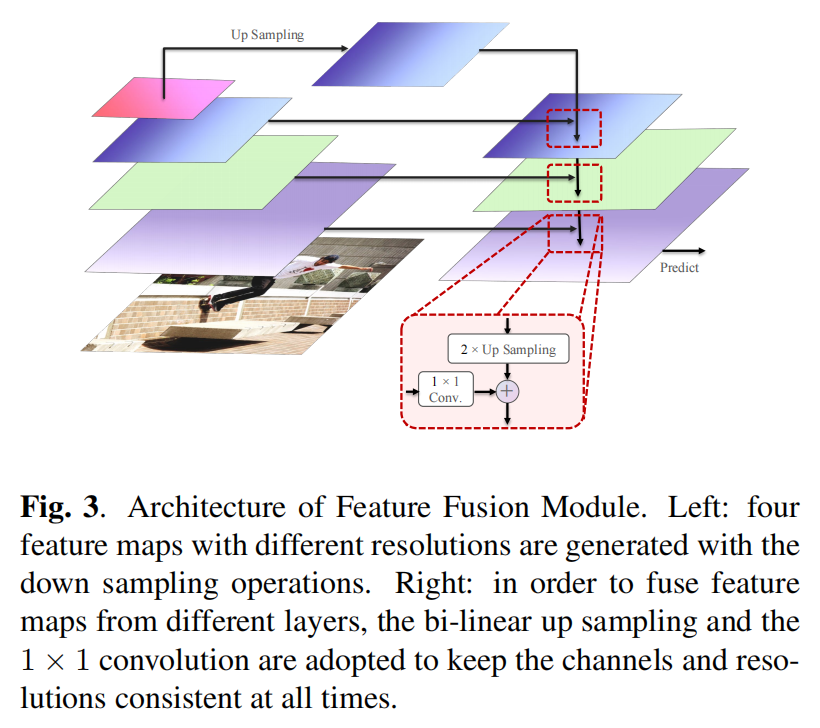 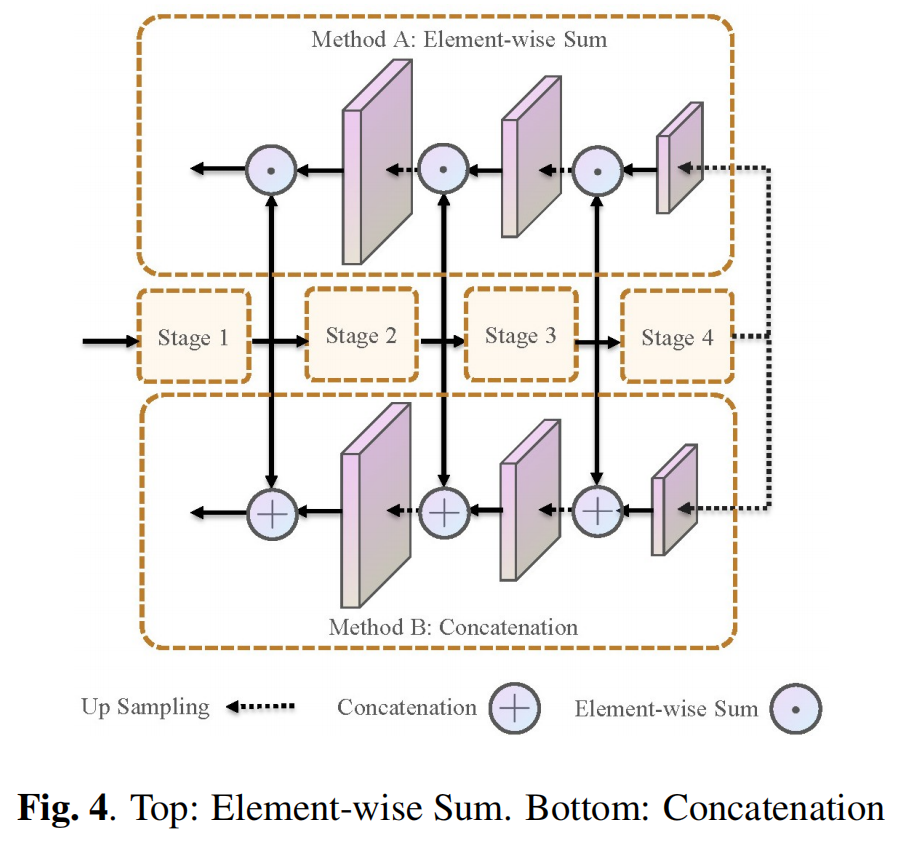
|