1.WordCell原理图
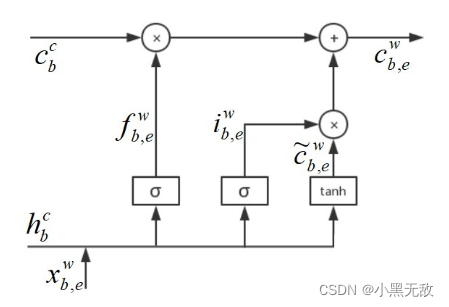
2.WordCell门控单元原始公式:
i
b
,
e
w
=
σ
(
W
i
,
e
x
b
,
e
w
+
W
i
,
b
h
b
,
c
+
b
i
)
i_{b,e}^{w} = \sigma(W_{i,e}x_{b,e}^{w} + W_{i,b}h_{b,c} + b_{i})
ib,ew?=σ(Wi,e?xb,ew?+Wi,b?hb,c?+bi?)
f
b
,
e
w
=
σ
(
W
f
,
e
x
b
,
e
w
+
W
f
,
b
h
b
,
c
+
b
f
)
f_{b,e}^{w} = \sigma(W_{f,e}x_{b,e}^{w} + W_{f,b}h_{b,c} + b_{f})
fb,ew?=σ(Wf,e?xb,ew?+Wf,b?hb,c?+bf?)
c
~
b
,
e
w
=
t
a
n
h
(
W
f
,
e
x
b
,
e
w
+
W
f
,
b
h
b
,
c
+
b
f
)
\widetilde{c}_{b,e}^{w} = tanh(W_{f,e}x_{b,e}^{w} + W_{f,b}h_{b,c} + b_{f})
c
b,ew?=tanh(Wf,e?xb,ew?+Wf,b?hb,c?+bf?)
维度分析:
W
x
x
W_{xx}
Wxx?.shape:[hidden_size,hidden_size]
x
b
,
e
w
x_{b,e}^{w}
xb,ew?.shape:[hidden_size,1](已经经过了变化,从input_size变成hidden_size)
3.WordCell门控单元简化公式:
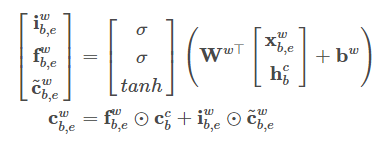 维度分析:
W
W
T
W^{W^{T}}
WWT与原始公式
[
W
i
,
e
,
W
i
,
b
;
W
f
,
e
,
W
f
,
b
;
W
f
,
e
,
W
f
,
b
]
[W_{i,e}, W_{i,b};W_{f,e},W_{f,b};W_{f,e},W_{f,b}]
[Wi,e?,Wi,b?;Wf,e?,Wf,b?;Wf,e?,Wf,b?]
x
b
,
e
w
x_{b,e}^{w}
xb,ew?.shape:[hidden_size,1]
h
b
c
h_{b}^{c}
hbc?.shape:[hidden_size,1]
W
W
T
W^{W^{T}}
WWT.shape:[3 x hidden_size,2 x hidden_size]
4.代码实现
import torch
from torch import nn
import torch.autograd as autograd
from torch.autograd import Variable
from torch.nn import functional,init
import numpy as np
class WordLSTMCell(nn.Module):
def __init__(self,input_size,hidden_size,use_bias = True):
super(WordLSTMCell,self).__init__()
self.input_size = input_size
self.hidden_size = hidden_size
self.use_bias = use_bias
self.weight_ih = nn.Parameter(
torch.FloatTensor(input_size,3 * hidden_size)
)
self.weight_hh = nn.Parameter(
torch.FloatTensor(hidden_size,3 * hidden_size)
)
if use_bias:
self.bias = nn.Parameter(torch.FloatTensor(3 * hidden_size))
else:
self.register_parameter('bias',None)
self.reset_parameters()
def reset_parameters(self):
init.orthogonal_(self.weight_ih.data)
weight_hh_data = torch.eye(self.hidden_size)
weight_hh_data = weight_hh_data.repeat(1,3)
with torch.no_grad():
self.weight_hh.set_(weight_hh_data)
if self.use_bias:
init.constant_(self.bias.data,val = 0)
def forward(self,input_,hx):
h_0,c_0 = hx
batch_size = h_0.size(0)
bias_batch = self.bias.unsqueeze(0).expand(batch_size,*self.bias.size())
wh_b = torch.addmm(bias_batch,h_0,self.weight_hh)
wi = torch.mm(input_,self.weight_ih)
f,i,g = torch.split(wh_b + wi,split_size_or_sections = self.hidden_size,dim = 1)
c_l = torch.sigmoid(f) * c_0 + torch.sigmoid(i) * torch.tanh(g)
return c_l
num_words = 5
word_emb_dim = 100
hidden_size = 100
input_ = torch.randn([num_words,word_emb_dim])
h_0 = torch.randn([1,hidden_size])
c_0 = torch.randn([1,hidden_size])
hx = (h_0,c_0)
word_lstm_cell = WordLSTMCell(word_emb_dim,hidden_size)
print(word_lstm_cell(input_,hx).shape)
|