引言
在我之前的文章CNN到底每层学到了什么?中,我可视化了不同的层学到的特征表示,最近看论文看到了一些新东西,就打算再之前的基础上进一步加深理解模型学到的特征表示。如果下次有空,我会进一步探究剪枝模型的特征表示差异等等(目前只想到这个)。话不多说进入正题:
实验
本次使用了在自制的imagenet50上训练得到的vgg11,为啥用imagenet50呢,因为没卡,苦涩。先来看看不同层的各个卷积通道学到的特征表示吧。 先放原图: 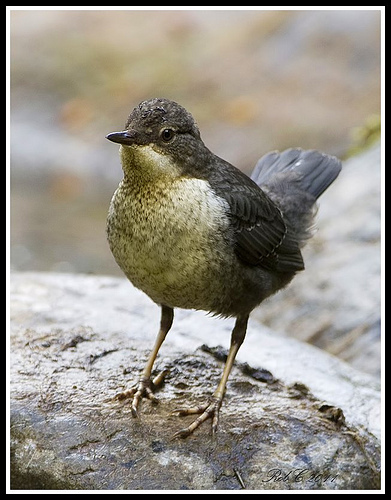 处理过后的阴间图片 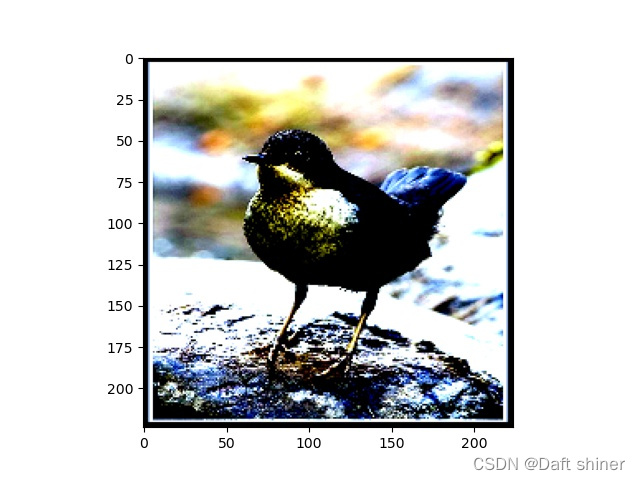 每层所有卷积核的特征表示,从左往右,从上往下卷积核number越大: 多图警告!!! 第一层: 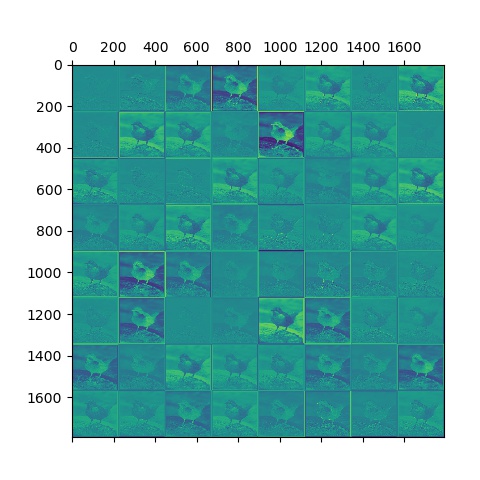 第二层: 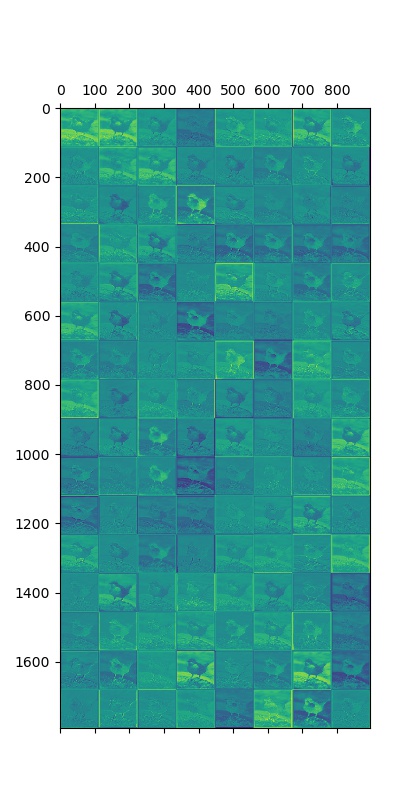 第三层: 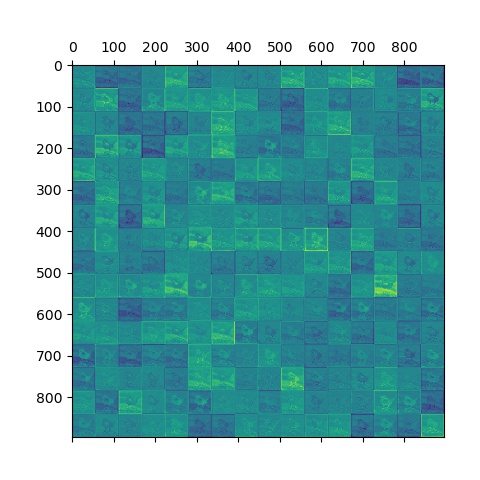 第四层: 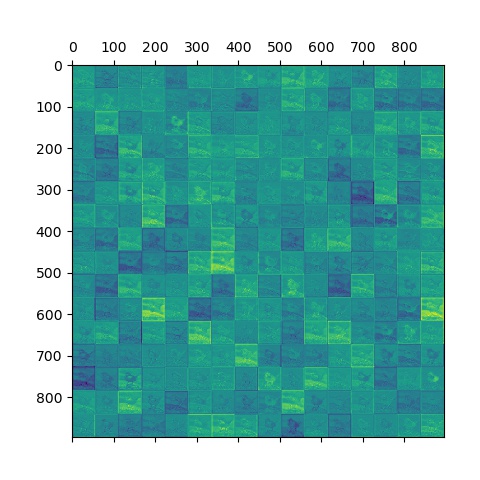 第五层: 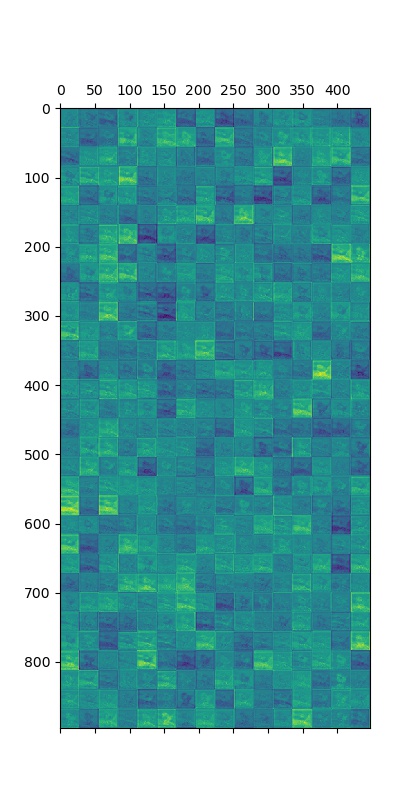 第六层: 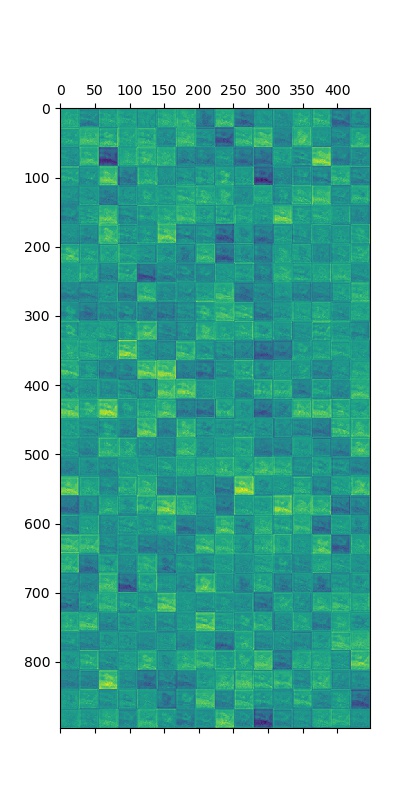 第七层: 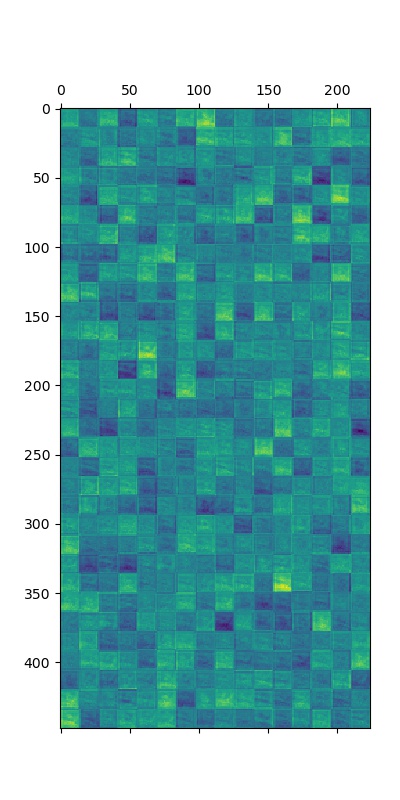 第八层: 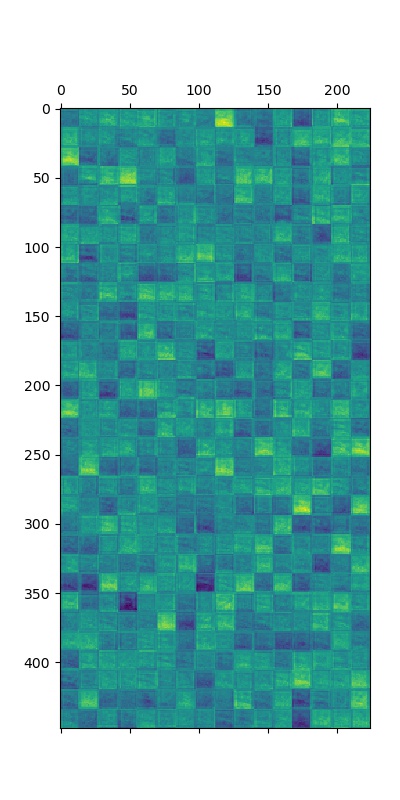 接下来我们使用CKA计算每两个卷积提取的特征的相似性,并画了下面这些图(颜色月森越相似),方便起见我没把横纵坐标可视化出来。 第一层: 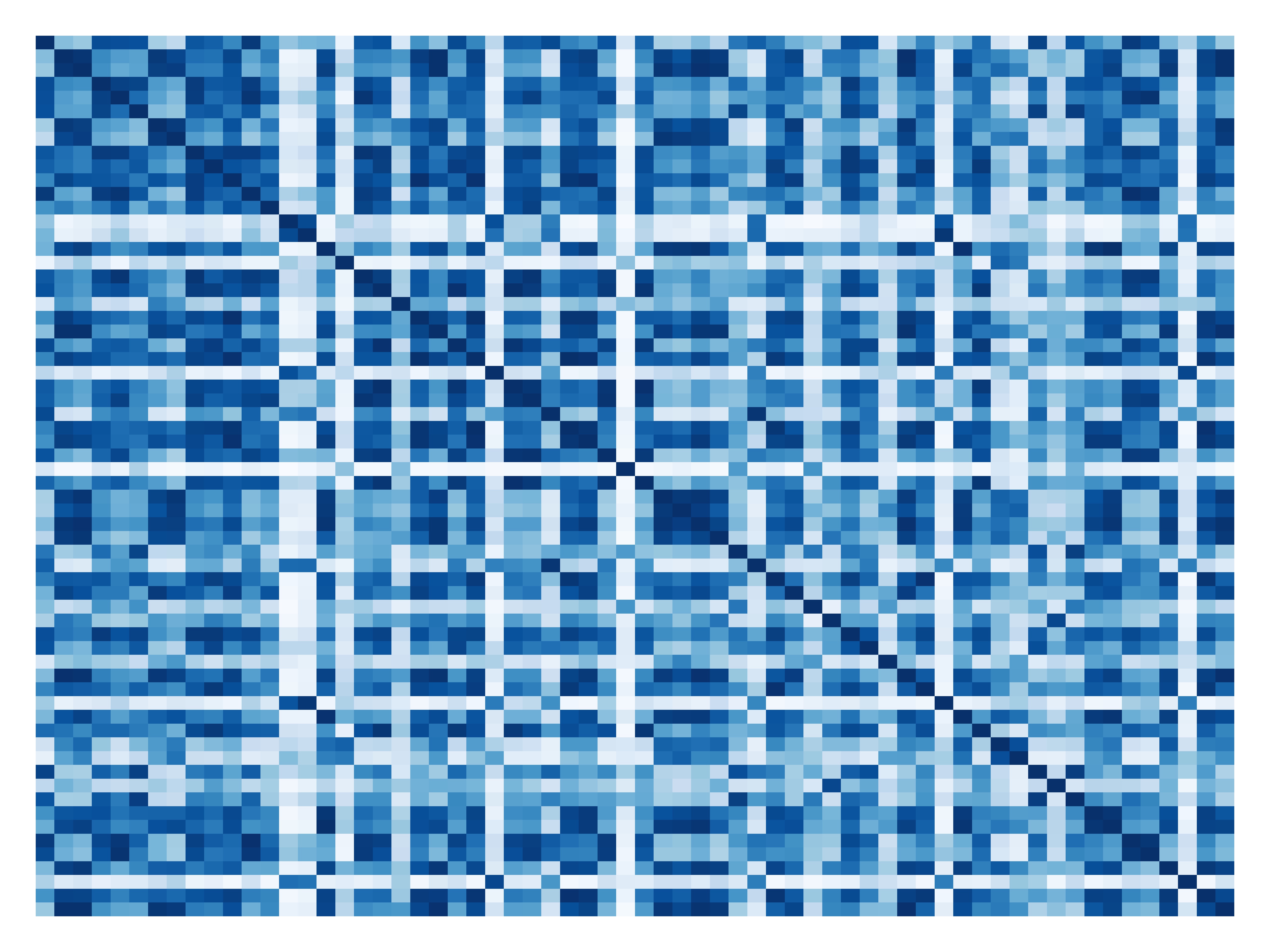 第二层: 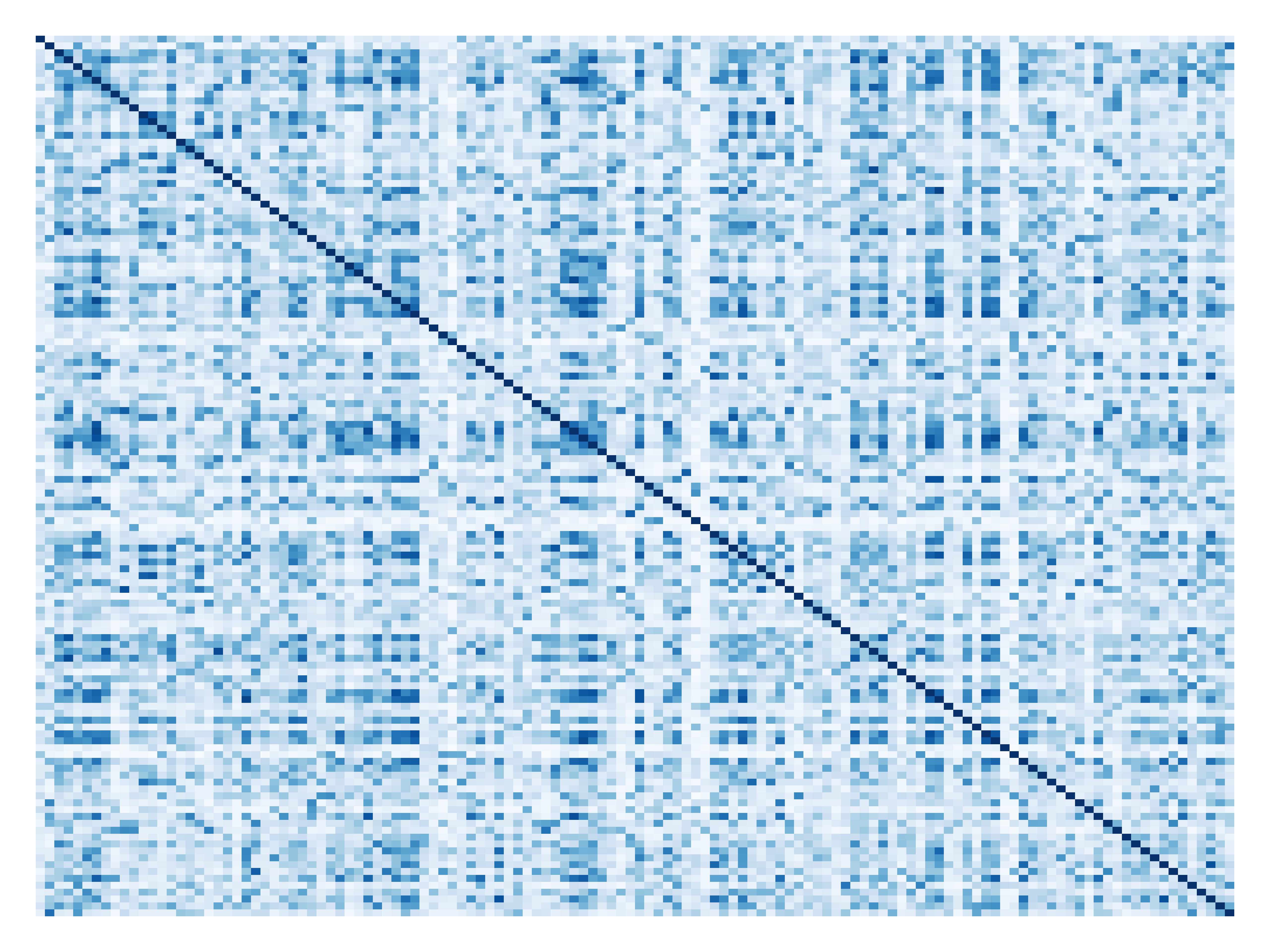 第三层: 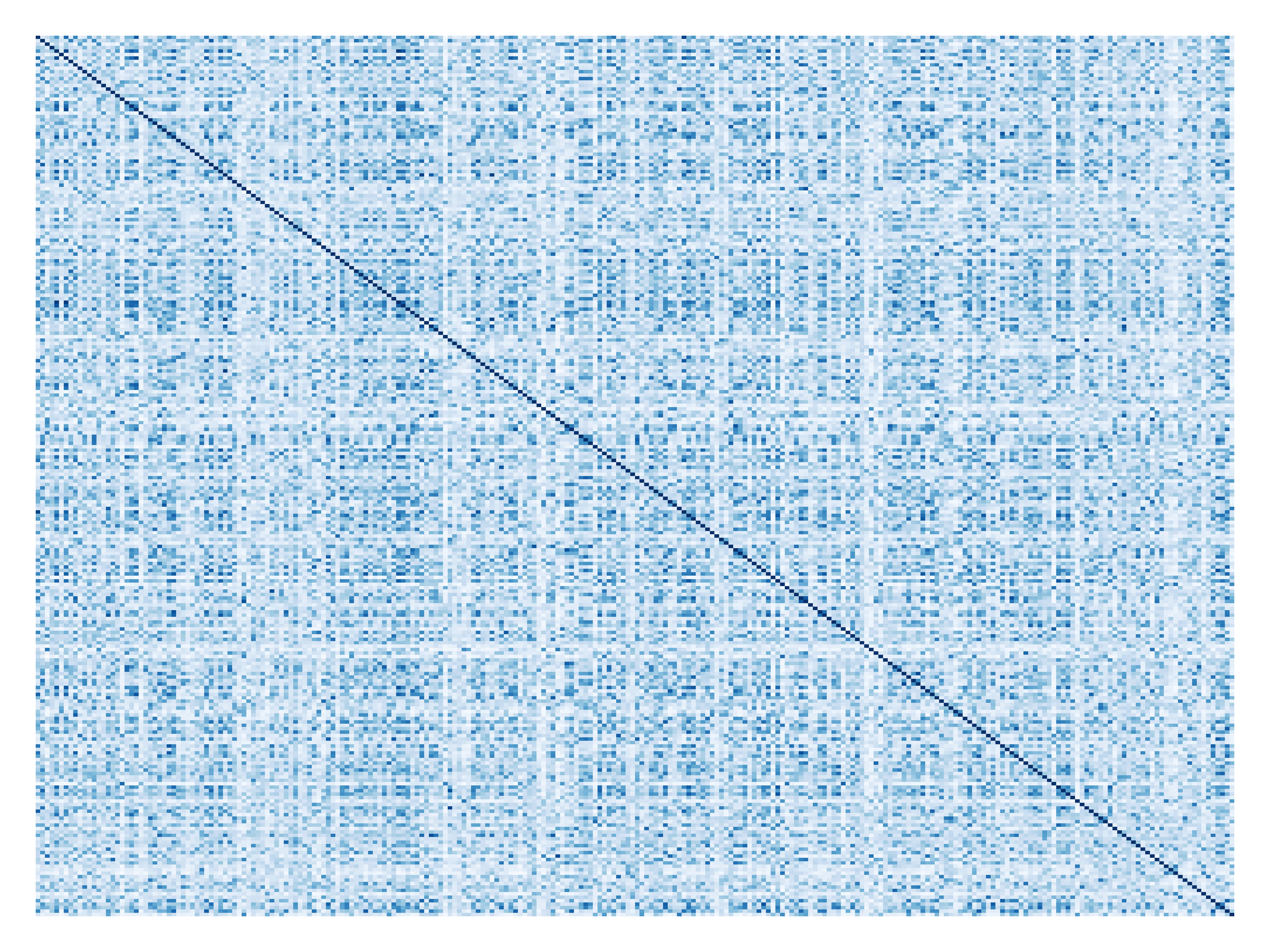 第四层: 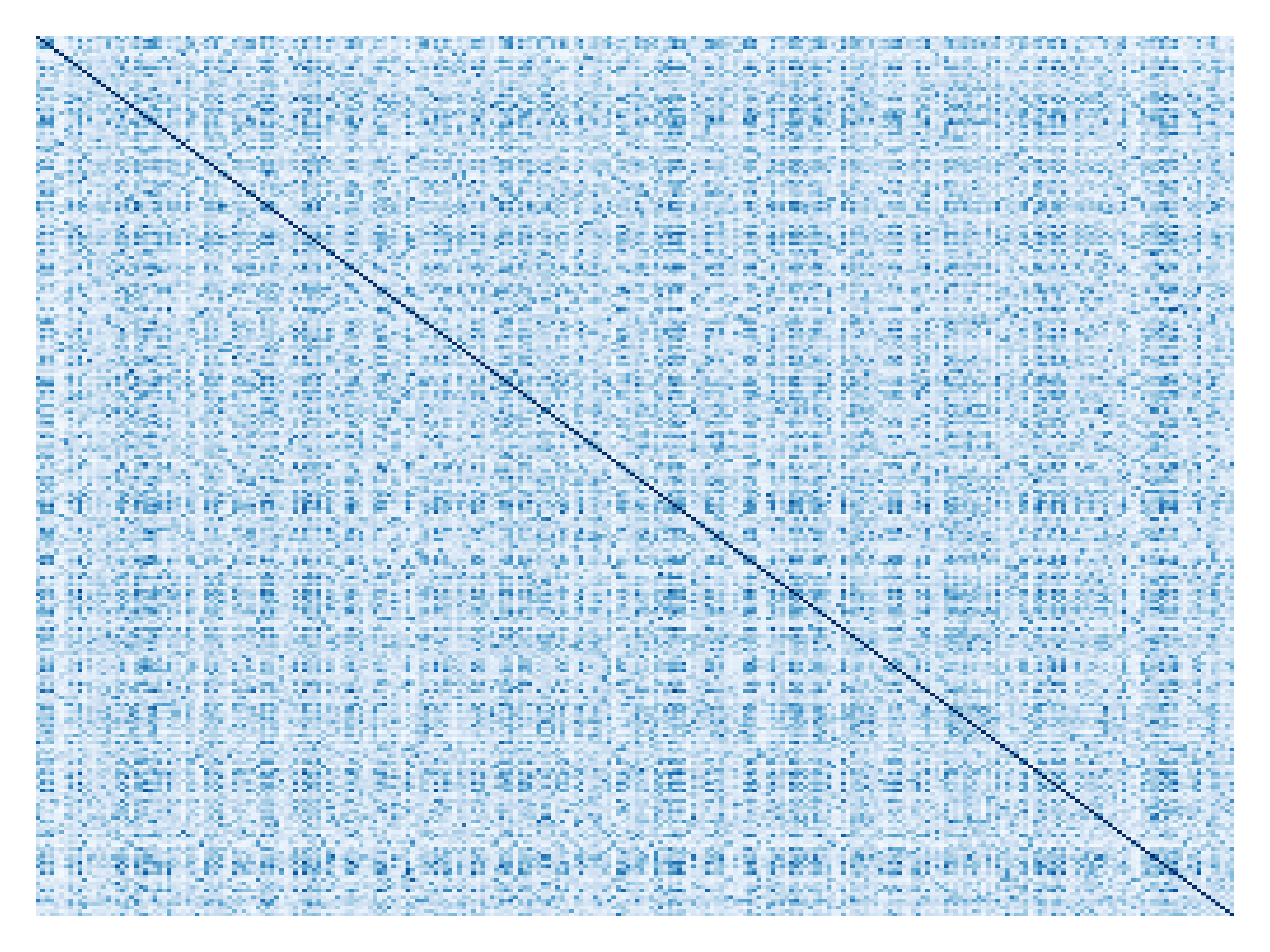 第五层: 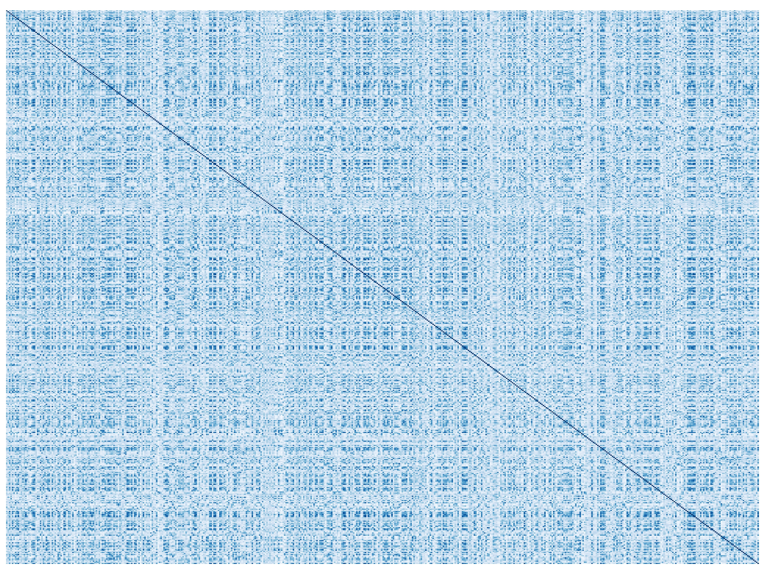 第六层: 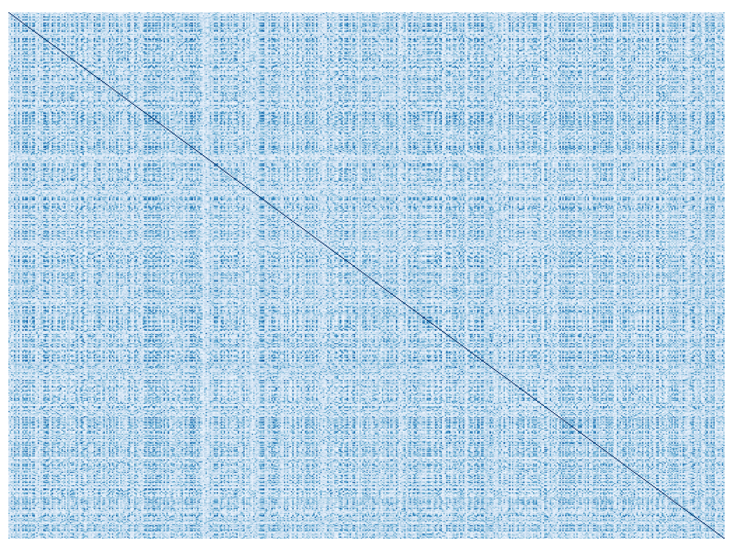 第七层: 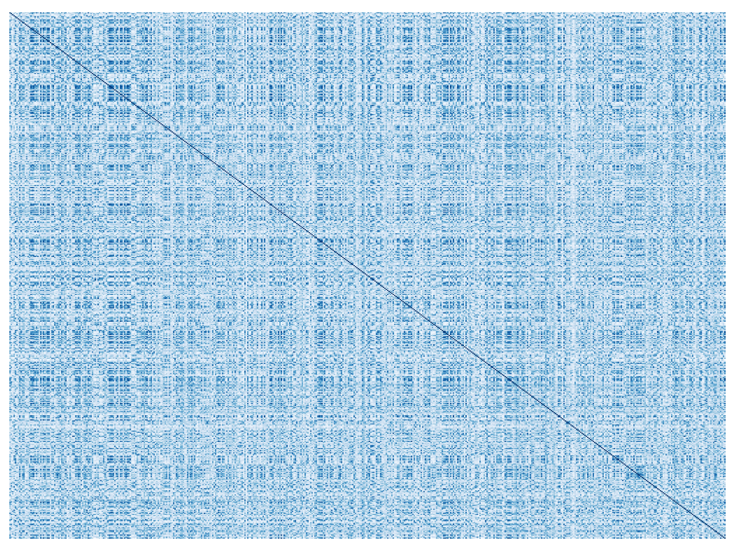 第八层: 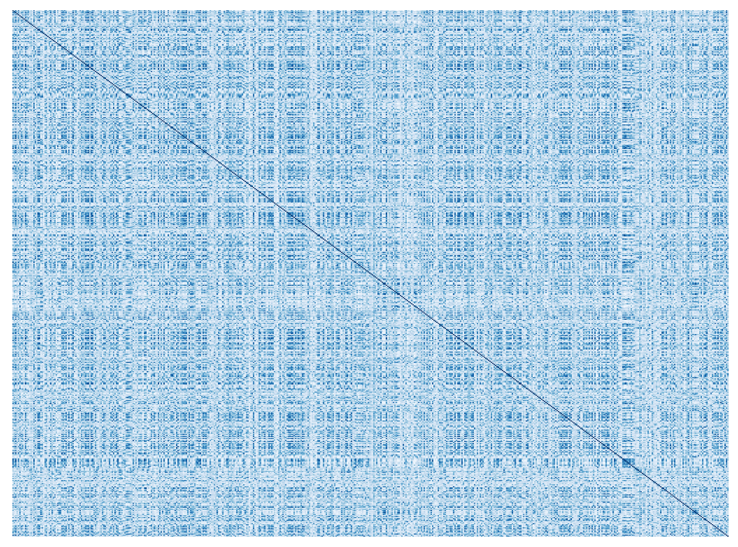 看的我一脸懵逼,没啥想法,看来CKA还是比较适合比较层间相似性。
源码
import os
import PIL
import numpy
import numpy as np
import torch
import torch.nn.functional as F
import matplotlib.pyplot as plt
import torch.nn as nn
import seaborn as sns
from args import args
from utils.Get_model import get_model
from utils.design_for_hook import get_inner_feature_for_vgg
def denormalize(tensor, mean, std):
if not tensor.ndimension() == 4:
raise TypeError('tensor should be 4D')
mean = torch.FloatTensor(mean).view(1, 3, 1, 1).expand_as(tensor).to(tensor.device)
std = torch.FloatTensor(std).view(1, 3, 1, 1).expand_as(tensor).to(tensor.device)
return tensor.mul(std).add(mean)
def normalize(tensor, mean, std):
if not tensor.ndimension() == 4:
raise TypeError('tensor should be 4D')
mean = torch.FloatTensor(mean).view(1, 3, 1, 1).expand_as(tensor).to(tensor.device)
std = torch.FloatTensor(std).view(1, 3, 1, 1).expand_as(tensor).to(tensor.device)
return tensor.sub(mean).div(std)
class Normalize(object):
def __init__(self, mean, std):
self.mean = mean
self.std = std
def __call__(self, tensor):
return self.do(tensor)
def do(self, tensor):
return normalize(tensor, self.mean, self.std)
def undo(self, tensor):
return denormalize(tensor, self.mean, self.std)
def __repr__(self):
return self.__class__.__name__ + '(mean={0}, std={1})'.format(self.mean, self.std)
def centering(K):
n = K.shape[0]
unit = np.ones([n, n])
I = np.eye(n)
H = I - unit / n
return np.dot(np.dot(H, K), H)
def linear_HSIC(X, Y):
L_X = np.dot(X, X.T)
L_Y = np.dot(Y, Y.T)
return np.sum(centering(L_X) * centering(L_Y))
def linear_CKA(X, Y):
hsic = linear_HSIC(X, Y)
var1 = np.sqrt(linear_HSIC(X, X))
var2 = np.sqrt(linear_HSIC(Y, Y))
return hsic / (var1 * var2)
def CKA_heatmap(inter_feature, layer):
layer_num = len(inter_feature)
print(layer_num)
CKA_matrix = torch.zeros((layer_num, layer_num))
for ll in range(layer_num):
for jj in range(layer_num):
if ll < jj:
CKA_matrix[ll, jj] = CKA_matrix[jj, ll] = linear_CKA(inter_feature[ll].cpu().numpy(),
inter_feature[jj].cpu().numpy())
CKA_matrix = CKA_matrix + torch.eye(layer_num)
plt.rc('font', family='Times New Roman', size=12)
ax = sns.heatmap(CKA_matrix.cpu().detach().numpy(), annot=False, cmap='Blues', cbar=False)
ax.set_xlabel('Conv')
ax.set_ylabel('Conv')
plt.axis('off')
plt.tight_layout()
plt.rcParams['savefig.dpi'] = 800
plt.rcParams['figure.dpi'] = 800
plt.xticks(rotation=0)
plt.savefig('imgs/heatmap%d.jpg' % layer)
def plot(feature, num, layer, con_num, row_num, total_num):
plt.rc('font', family='Times New Roman', size=12)
con = num % con_num
row = num // con_num
axes = plt.subplot2grid((con_num, row_num), (row, con), rowspan=1, colspan=1)
axes.matshow(feature.cpu().detach().numpy())
plt.rcParams['savefig.dpi'] = 800
plt.rcParams['figure.dpi'] = 800
plt.axis('off')
if num == total_num-1:
plt.tight_layout()
plt.savefig('imgs/conv in layer%d.jpg' % layer)
plt.show()
if __name__ == "__main__":
inter_feature = []
img_dir = '/public/MountData/dataset/ImageNet50/val/n01601694/'
img_name = 'ILSVRC2012_val_00043566.JPEG'
img_path = os.path.join(img_dir, img_name)
def hook(module, input, output):
inter_feature.append(output.clone().detach())
pil_img = PIL.Image.open(img_path)
normalizer = Normalize(mean=[0.485, 0.456, 0.406], std=[0.229, 0.224, 0.225])
torch_img = torch.from_numpy(np.asarray(pil_img)).permute(2, 0, 1).unsqueeze(0).float().div(255).cuda()
torch_img = F.upsample(torch_img, size=(224, 224), mode='bilinear', align_corners=False)
normed_torch_img = normalizer(torch_img)
criterion = nn.CrossEntropyLoss().cuda()
model = get_model(args)
model.eval()
model.cuda()
handle_list = get_inner_feature_for_vgg(model, hook, args.arch)
output = model(normed_torch_img)
for m in range(len(inter_feature)):
print('-' * 50)
print(m)
interfeature = inter_feature[m].squeeze()
dim0 = interfeature.shape[0]
h, w = interfeature.shape[1], interfeature.shape[2]
if dim0 == 64:
con_num = 8
row_num = 8
elif dim0 == 128:
con_num = 8
row_num = 16
elif dim0 == 256:
con_num = 16
row_num = 16
elif dim0 == 512:
con_num = 16
row_num = 32
else:
con_num = 100
row_num = 100
matrix = numpy.zeros((h * row_num, w * con_num))
print(matrix.shape)
for i in range(dim0):
con = i % con_num
row = i // con_num
feature = interfeature[i]
print(row*w, row*w+w, 111, con*h, con*h+h)
matrix[row*w:row*w+w, con*h:con*h+h] += feature.cpu().detach().numpy()
plt.matshow(matrix)
plt.savefig('imgs/conv%d.jpg' % m)
CKA_heatmap(interfeature, m)
plt.show()
这里模型的代码和hook的代码就不放了吧,反正也很简单,可以根据自己需求进行改动。
参考文献
|