GhostModule
论文地址: https://arxiv.org/abs/1911.11907 论文名称:GhostNet 如下图,作者在实验过程中将ResNet50的第一个残差组的feature map进行可视化,发现里面有三对feature map(如下图中的红绿蓝三对feature map)它们极其相似,作者认为这些feature map对之间是冗余的(相关的)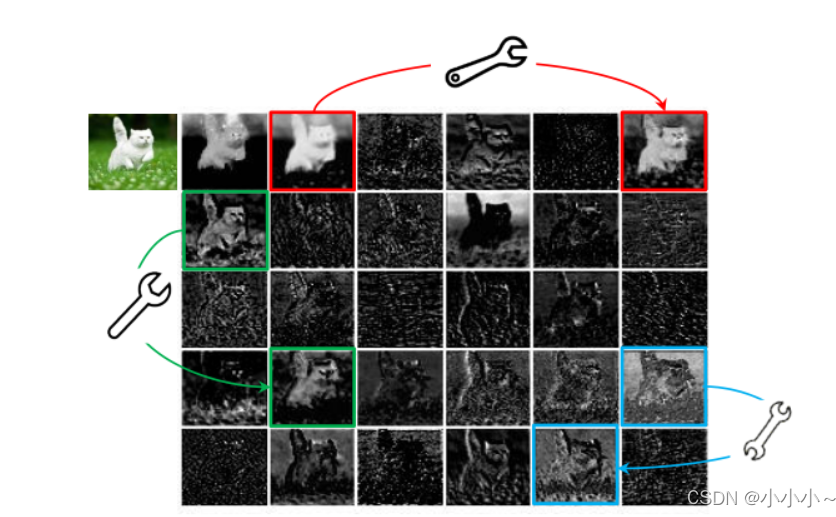 作者考虑到这些feature map层中的冗余信息可能是一个成功模型的重要组成部分,正是因为这些冗余信息才能保证输入数据的全面理解,所以作者在设计轻量化模型的时候并没有试图去除这些冗余feature map,而是尝试使用更低成本的计算量来获取这些冗余feature map。 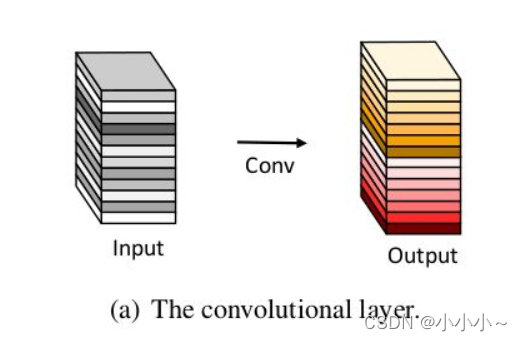 上图为一个普通卷积。一部分是Intrinic,而另外一部分是可以由 intrinsic 通过cheap operations来生成的,因为本来就是由intrinsic feature maps 生成的,所以肯定会有很多的冗余信息,所以称其为 ghost feature maps。 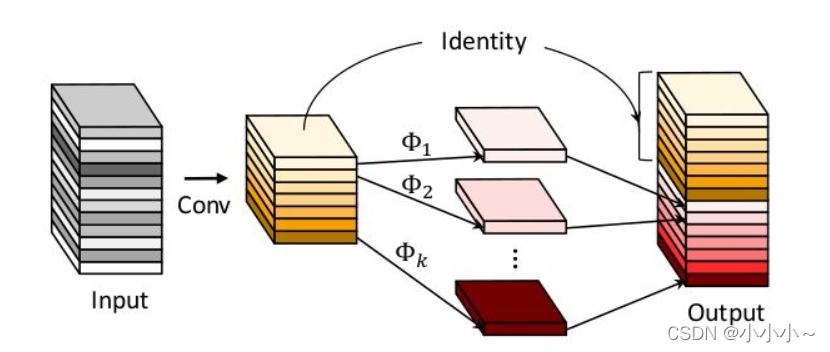 Ghost Module: 第一步: 少量卷积(比如正常用32个卷积核,这里就用16个,从而减少一半的计算量) 第二步:cheap operations,如图中的
Φ
Φ
Φ表示,
Φ
Φ
Φ是诸如3x3 或 5x5的卷积,并且是逐个特征图的进行卷积(Depth-wise convolutional)。
相关代码:
class GhostModule(nn.Module):
def __init__(self, inp, oup, kernel_size=1, ratio=2, dw_size=3, stride=1, relu=True):
super(GhostModule, self).__init__()
self.oup = oup
init_channels = math.ceil(oup / ratio)
new_channels = init_channels*(ratio-1)
self.primary_conv = nn.Sequential(
nn.Conv2d(inp, init_channels, kernel_size, stride, kernel_size//2, bias=False),
nn.BatchNorm2d(init_channels),
nn.ReLU(inplace=True) if relu else nn.Sequential(),
)
self.cheap_operation = nn.Sequential(
nn.Conv2d(init_channels, new_channels, dw_size, 1, dw_size//2, groups=init_channels, bias=False),
nn.BatchNorm2d(new_channels),
nn.ReLU(inplace=True) if relu else nn.Sequential(),
)
def forward(self, x):
x1 = self.primary_conv(x)
x2 = self.cheap_operation(x1)
out = torch.cat([x1,x2], dim=1)
return out[:,:self.oup,:,:]
|