参考以下英文教程:
OpenCV Face Recognition - PyImageSearch
一、所需代码安装
1.1、OpenCV
OpenCV Tutorials, Resources, and Guides | PyImageSearch
2、FaceNet
3、TensorFlow
4、Python
二、下载示例代码
地址:https://github.com/Ravi-Singh88/Face-Recognition-OpenCV-Facenet
OpenCV Face Recognition
$ tree --dirsfirst
.
├── dataset
│ ├── adrian [6 images]
│ ├── trisha [6 images]
│ └── unknown [6 images]
├── images
│ ├── adrian.jpg
│ ├── patrick_bateman.jpg
│ └── trisha_adrian.jpg
├── face_detection_model
│ ├── deploy.prototxt
│ └── res10_300x300_ssd_iter_140000.caffemodel
├── output
│ ├── embeddings.pickle
│ ├── le.pickle
│ └── recognizer.pickle
├── extract_embeddings.py
├── openface_nn4.small2.v1.t7
├── train_model.py
├── recognize.py
└── recognize_video.py
7 directories, 31 files
项目根目录有四个目录::
-
dataset/ ?: 人脸图像,按人名设置目录 -
images/ ?: 验证图片. -
face_detection_model/ ?: OpenCV中 pre-trained Caffe人脸检测模型,用于检测和定位图片中人脸 -
output/ ?: 输出的pickle files,包括:
总结根本下的五个文件:
三、Step #1: 从人脸数据集中提取并保存特征向量(embeddings)
extract_embeddings.py
3.1、加载包和参数
OpenCV Face Recognition
# import the necessary packages
from imutils import paths
import numpy as np
import argparse
import imutils
import pickle
import cv2
import os
# construct the argument parser and parse the arguments
ap = argparse.ArgumentParser()
ap.add_argument("-i", "--dataset", required=True,
help="path to input directory of faces + images")
ap.add_argument("-e", "--embeddings", required=True,
help="path to output serialized db of facial embeddings")
ap.add_argument("-d", "--detector", required=True,
help="path to OpenCV's deep learning face detector")
ap.add_argument("-m", "--embedding-model", required=True,
help="path to OpenCV's deep learning face embedding model")
ap.add_argument("-c", "--confidence", type=float, default=0.5,
help="minimum probability to filter weak detections")
args = vars(ap.parse_args())
说明:
1、需要安装包,OpenCV和?imutils?
$ pip install --upgrade imutils
2、参数说明
-
--dataset ?: 输入人脸数据集图片的路径 -
--embeddings ?: 提取特征向量输出文件. -
--detector ?: OpenCV?Caffe-based 人脸检测器路径,用于定位图片中的人脸. -
--embedding-model ?: OpenCV Torch 特征模型,用于提取128-D 人脸特征向量 -
--confidence ?: 选择阈值用于弱人脸检测器的过滤.
3.2、加载人脸检测器和特征提取器
OpenCV Face Recognition
# load our serialized face detector from disk
print("[INFO] loading face detector...")
protoPath = os.path.sep.join([args["detector"], "deploy.prototxt"])
modelPath = os.path.sep.join([args["detector"],
"res10_300x300_ssd_iter_140000.caffemodel"])
detector = cv2.dnn.readNetFromCaffe(protoPath, modelPath)
# load our serialized face embedding model from disk
print("[INFO] loading face recognizer...")
embedder = cv2.dnn.readNetFromTorch(args["embedding_model"])
?
-
detector ?: 采用基于Caffe模型的人脸检测器定位图片人脸? -
embedder ?: 采用基于Torch模型的提取器提取人脸特征facial embeddings.
代码中通过cv2.dnn的函数加载。
3.3、图片加载与处理
1、图片路径设置与初始化
OpenCV Face Recognition
# grab the paths to the input images in our dataset
print("[INFO] quantifying faces...")
imagePaths = list(paths.list_images(args["dataset"]))
# initialize our lists of extracted facial embeddings and
# corresponding people names
knownEmbeddings = []
knownNames = []
# initialize the total number of faces processed
total = 0
paths.list_images在imutils中。
循环图片
# loop over the image paths
for (i, imagePath) in enumerate(imagePaths):
# extract the person name from the image path
print("[INFO] processing image {}/{}".format(i + 1,
len(imagePaths)))
name = imagePath.split(os.path.sep)[-2]
# load the image, resize it to have a width of 600 pixels (while
# maintaining the aspect ratio), and then grab the image
# dimensions
image = cv2.imread(imagePath)
image = imutils.resize(image, width=600)
(h, w) = image.shape[:2]
?提取人名采用以下方式:
$ python
>>> from imutils import paths
>>> import os
>>> imagePaths = list(paths.list_images("dataset"))
>>> imagePath = imagePaths[0]
>>> imagePath
'dataset/adrian/00004.jpg'
>>> imagePath.split(os.path.sep)
['dataset', 'adrian', '00004.jpg']
>>> imagePath.split(os.path.sep)[-2]
'adrian'
>>>
?3.3 检测与定位人脸
# construct a blob from the image
imageBlob = cv2.dnn.blobFromImage(
cv2.resize(image, (300, 300)), 1.0, (300, 300),
(104.0, 177.0, 123.0), swapRB=False, crop=False)
# apply OpenCV's deep learning-based face detector to localize
# faces in the input image
detector.setInput(imageBlob)
detections = detector.forward()
参见:Deep learning: How OpenCV’s blobFromImage works.
# ensure at least one face was found
if len(detections) > 0:
# we're making the assumption that each image has only ONE
# face, so find the bounding box with the largest probability
i = np.argmax(detections[0, 0, :, 2])
confidence = detections[0, 0, i, 2]
# ensure that the detection with the largest probability also
# means our minimum probability test (thus helping filter out
# weak detections)
if confidence > args["confidence"]:
# compute the (x, y)-coordinates of the bounding box for
# the face
box = detections[0, 0, i, 3:7] * np.array([w, h, w, h])
(startX, startY, endX, endY) = box.astype("int")
# extract the face ROI and grab the ROI dimensions
face = image[startY:endY, startX:endX]
(fH, fW) = face.shape[:2]
# ensure the face width and height are sufficiently large
if fW < 20 or fH < 20:
continue
?
detections? 包含图片中检测到的人脸概率和位置
3.4 提取人脸特征(embeddings)
# construct a blob for the face ROI, then pass the blob
# through our face embedding model to obtain the 128-d
# quantification of the face
faceBlob = cv2.dnn.blobFromImage(face, 1.0 / 255,
(96, 96), (0, 0, 0), swapRB=True, crop=False)
embedder.setInput(faceBlob)
vec = embedder.forward()
# add the name of the person + corresponding face
# embedding to their respective lists
knownNames.append(name)
knownEmbeddings.append(vec.flatten())
total += 1
3.5 特征序列化
# dump the facial embeddings + names to disk
print("[INFO] serializing {} encodings...".format(total))
data = {"embeddings": knownEmbeddings, "names": knownNames}
f = open(args["embeddings"], "wb")
f.write(pickle.dumps(data))
f.close()
运行结果如下:
$ python extract_embeddings.py --dataset dataset \
--embeddings output/embeddings.pickle \
--detector face_detection_model \
--embedding-model openface_nn4.small2.v1.t7
[INFO] loading face detector...
[INFO] loading face recognizer...
[INFO] quantifying faces...
[INFO] processing image 1/18
[INFO] processing image 2/18
[INFO] processing image 3/18
[INFO] processing image 4/18
[INFO] processing image 5/18
[INFO] processing image 6/18
[INFO] processing image 7/18
[INFO] processing image 8/18
[INFO] processing image 9/18
[INFO] processing image 10/18
[INFO] processing image 11/18
[INFO] processing image 12/18
[INFO] processing image 13/18
[INFO] processing image 14/18
[INFO] processing image 15/18
[INFO] processing image 16/18
[INFO] processing image 17/18
[INFO] processing image 18/18
[INFO] serializing 18 encodings...
四、Step #2: 训练人脸识别模型Train face recognition model
Step#1中已经提取了每一个人脸的128-d embeddings,但是如何使用这些特征呢?答案是根据特征embeddings训练一个通用的机器学习模型(SVM, k-NN classifier, Random Forest, etc.)
通过k-NN方法识别人脸,参见:Face recognition with OpenCV, Python, and deep learning - PyImageSearch
本次采用SVM来训练。
train_model.py.
# import the necessary packages
from sklearn.preprocessing import LabelEncoder
from sklearn.svm import SVC
import argparse
import pickle
# construct the argument parser and parse the arguments
ap = argparse.ArgumentParser()
ap.add_argument("-e", "--embeddings", required=True,
help="path to serialized db of facial embeddings")
ap.add_argument("-r", "--recognizer", required=True,
help="path to output model trained to recognize faces")
ap.add_argument("-l", "--le", required=True,
help="path to output label encoder")
args = vars(ap.parse_args())
??scikit-learn是机器学习函数库,通过此库文件训练SVM模型,需要安装
$ pip install scikit-learn
train_model.py 参数说明
-
--embeddings ?: extract_embeddings.py中序列化提取到的特征文件。 -
--recognizer ?: 输出的基于SVM的识别模型。后面会用到。 -
--le ?: 输出的标签文件路径,在图片和视频中人脸识别要用到.
三个参数都是必须的。
4.1 加载特征向量文件和标签
# load the face embeddings
print("[INFO] loading face embeddings...")
data = pickle.loads(open(args["embeddings"], "rb").read())
# encode the labels
print("[INFO] encoding labels...")
le = LabelEncoder()
labels = le.fit_transform(data["names"])
LabelEncoder来自?scikit-learn。
4.2 训练SVM
# train the model used to accept the 128-d embeddings of the face and
# then produce the actual face recognition
print("[INFO] training model...")
recognizer = SVC(C=1.0, kernel="linear", probability=True)
recognizer.fit(data["embeddings"], labels)
此处用SVM,也可以用其他机器学习方法
4.3 输出训练模型
# write the actual face recognition model to disk
f = open(args["recognizer"], "wb")
f.write(pickle.dumps(recognizer))
f.close()
# write the label encoder to disk
f = open(args["le"], "wb")
f.write(pickle.dumps(le))
f.close()
训练结果是我们获得输出两个pickle文件,人脸识别模型文件和标签编码文件
执行结果:
$ python train_model.py --embeddings output/embeddings.pickle \
--recognizer output/recognizer.pickle \
--le output/le.pickle
[INFO] loading face embeddings...
[INFO] encoding labels...
[INFO] training model...
$ ls output/
embeddings.pickle le.pickle recognizer.pickle
五、Step #3: OpenCV人脸识别Recognize faces with OpenCV
recognize.py
5.1 参数说明
# import the necessary packages
import numpy as np
import argparse
import imutils
import pickle
import cv2
import os
# construct the argument parser and parse the arguments
ap = argparse.ArgumentParser()
ap.add_argument("-i", "--image", required=True,
help="path to input image")
ap.add_argument("-d", "--detector", required=True,
help="path to OpenCV's deep learning face detector")
ap.add_argument("-m", "--embedding-model", required=True,
help="path to OpenCV's deep learning face embedding model")
ap.add_argument("-r", "--recognizer", required=True,
help="path to model trained to recognize faces")
ap.add_argument("-l", "--le", required=True,
help="path to label encoder")
ap.add_argument("-c", "--confidence", type=float, default=0.5,
help="minimum probability to filter weak detections")
args = vars(ap.parse_args())
六个参数:
-
--image ?: 要识别的图片路径. -
--detector ?: OpenCV人脸检测器,用检测图片中的人脸ROIs -
--embedding-model ?: OpenCV 人脸特征提取器路径. -
--recognizer ?: 训练好的SVM人脸识别器模型路径. -
--le ?: 标签文件路径,人脸标签为人名?. -
--confidence ?: 置信度
六个参数包二个深度学习模型和一个SVM模型。
5.2 加载模型
# load our serialized face detector from disk
print("[INFO] loading face detector...")
protoPath = os.path.sep.join([args["detector"], "deploy.prototxt"])
modelPath = os.path.sep.join([args["detector"],
"res10_300x300_ssd_iter_140000.caffemodel"])
detector = cv2.dnn.readNetFromCaffe(protoPath, modelPath)
# load our serialized face embedding model from disk
print("[INFO] loading face recognizer...")
embedder = cv2.dnn.readNetFromTorch(args["embedding_model"])
# load the actual face recognition model along with the label encoder
recognizer = pickle.loads(open(args["recognizer"], "rb").read())
le = pickle.loads(open(args["le"], "rb").read())
-
detector ?: 预训练的Caffe DL模型,检测人脸.(OpenCV提供) -
embedder ?:预训练的Torch DL模型提取128-D face embeddings?人脸特征.(OpenCV提供) -
recognizer ?: Step #2中训练的SVM人脸识别模型。
?5.3 加载图片并识别
# load the image, resize it to have a width of 600 pixels (while
# maintaining the aspect ratio), and then grab the image dimensions
image = cv2.imread(args["image"])
image = imutils.resize(image, width=600)
(h, w) = image.shape[:2]
# construct a blob from the image
imageBlob = cv2.dnn.blobFromImage(
cv2.resize(image, (300, 300)), 1.0, (300, 300),
(104.0, 177.0, 123.0), swapRB=False, crop=False)
# apply OpenCV's deep learning-based face detector to localize
# faces in the input image
detector.setInput(imageBlob)
detections = detector.forward()
blob构建参见Here.
根据置信度提取图片中的人脸
# loop over the detections
for i in range(0, detections.shape[2]):
# extract the confidence (i.e., probability) associated with the
# prediction
confidence = detections[0, 0, i, 2]
# filter out weak detections
if confidence > args["confidence"]:
# compute the (x, y)-coordinates of the bounding box for the
# face
box = detections[0, 0, i, 3:7] * np.array([w, h, w, h])
(startX, startY, endX, endY) = box.astype("int")
# extract the face ROI
face = image[startY:endY, startX:endX]
(fH, fW) = face.shape[:2]
# ensure the face width and height are sufficiently large
if fW < 20 or fH < 20:
continue
识别
# construct a blob for the face ROI, then pass the blob
# through our face embedding model to obtain the 128-d
# quantification of the face
faceBlob = cv2.dnn.blobFromImage(face, 1.0 / 255, (96, 96),
(0, 0, 0), swapRB=True, crop=False)
embedder.setInput(faceBlob)
vec = embedder.forward()
# perform classification to recognize the face
preds = recognizer.predict_proba(vec)[0]
j = np.argmax(preds)
proba = preds[j]
name = le.classes_[j]
标签结果
# draw the bounding box of the face along with the associated
# probability
text = "{}: {:.2f}%".format(name, proba * 100)
y = startY - 10 if startY - 10 > 10 else startY + 10
cv2.rectangle(image, (startX, startY), (endX, endY),
(0, 0, 255), 2)
cv2.putText(image, text, (startX, y),
cv2.FONT_HERSHEY_SIMPLEX, 0.45, (0, 0, 255), 2)
# show the output image
cv2.imshow("Image", image)
cv2.waitKey(0)
5.4 执行
$ python recognize.py --detector face_detection_model \
--embedding-model openface_nn4.small2.v1.t7 \
--recognizer output/recognizer.pickle \
--le output/le.pickle \
--image images/adrian.jpg
[INFO] loading face detector...
[INFO] loading face recognizer...
六、视频流的人脸识别
recognize_video.py
# import the necessary packages
from imutils.video import VideoStream
from imutils.video import FPS
import numpy as np
import argparse
import imutils
import pickle
import time
import cv2
import os
# construct the argument parser and parse the arguments
ap = argparse.ArgumentParser()
ap.add_argument("-d", "--detector", required=True,
help="path to OpenCV's deep learning face detector")
ap.add_argument("-m", "--embedding-model", required=True,
help="path to OpenCV's deep learning face embedding model")
ap.add_argument("-r", "--recognizer", required=True,
help="path to model trained to recognize faces")
ap.add_argument("-l", "--le", required=True,
help="path to label encoder")
ap.add_argument("-c", "--confidence", type=float, default=0.5,
help="minimum probability to filter weak detections")
args = vars(ap.parse_args())
# load our serialized face detector from disk
print("[INFO] loading face detector...")
protoPath = os.path.sep.join([args["detector"], "deploy.prototxt"])
modelPath = os.path.sep.join([args["detector"],
"res10_300x300_ssd_iter_140000.caffemodel"])
detector = cv2.dnn.readNetFromCaffe(protoPath, modelPath)
# load our serialized face embedding model from disk
print("[INFO] loading face recognizer...")
embedder = cv2.dnn.readNetFromTorch(args["embedding_model"])
# load the actual face recognition model along with the label encoder
recognizer = pickle.loads(open(args["recognizer"], "rb").read())
le = pickle.loads(open(args["le"], "rb").read())
# initialize the video stream, then allow the camera sensor to warm up
print("[INFO] starting video stream...")
vs = VideoStream(src=0).start()
time.sleep(2.0)
# start the FPS throughput estimator
fps = FPS().start()
# loop over frames from the video file stream
while True:
# grab the frame from the threaded video stream
frame = vs.read()
# resize the frame to have a width of 600 pixels (while
# maintaining the aspect ratio), and then grab the image
# dimensions
frame = imutils.resize(frame, width=600)
(h, w) = frame.shape[:2]
# construct a blob from the image
imageBlob = cv2.dnn.blobFromImage(
cv2.resize(frame, (300, 300)), 1.0, (300, 300),
(104.0, 177.0, 123.0), swapRB=False, crop=False)
# apply OpenCV's deep learning-based face detector to localize
# faces in the input image
detector.setInput(imageBlob)
detections = detector.forward()
?
# loop over the detections
for i in range(0, detections.shape[2]):
# extract the confidence (i.e., probability) associated with
# the prediction
confidence = detections[0, 0, i, 2]
# filter out weak detections
if confidence > args["confidence"]:
# compute the (x, y)-coordinates of the bounding box for
# the face
box = detections[0, 0, i, 3:7] * np.array([w, h, w, h])
(startX, startY, endX, endY) = box.astype("int")
# extract the face ROI
face = frame[startY:endY, startX:endX]
(fH, fW) = face.shape[:2]
# ensure the face width and height are sufficiently large
if fW < 20 or fH < 20:
continue
# construct a blob for the face ROI, then pass the blob
# through our face embedding model to obtain the 128-d
# quantification of the face
faceBlob = cv2.dnn.blobFromImage(face, 1.0 / 255,
(96, 96), (0, 0, 0), swapRB=True, crop=False)
embedder.setInput(faceBlob)
vec = embedder.forward()
# perform classification to recognize the face
preds = recognizer.predict_proba(vec)[0]
j = np.argmax(preds)
proba = preds[j]
name = le.classes_[j]
# draw the bounding box of the face along with the
# associated probability
text = "{}: {:.2f}%".format(name, proba * 100)
y = startY - 10 if startY - 10 > 10 else startY + 10
cv2.rectangle(frame, (startX, startY), (endX, endY),
(0, 0, 255), 2)
cv2.putText(frame, text, (startX, y),
cv2.FONT_HERSHEY_SIMPLEX, 0.45, (0, 0, 255), 2)
# update the FPS counter
fps.update()
# show the output frame
cv2.imshow("Frame", frame)
key = cv2.waitKey(1) & 0xFF
# if the `q` key was pressed, break from the loop
if key == ord("q"):
break
# stop the timer and display FPS information
fps.stop()
print("[INFO] elasped time: {:.2f}".format(fps.elapsed()))
print("[INFO] approx. FPS: {:.2f}".format(fps.fps()))
# do a bit of cleanup
cv2.destroyAllWindows()
vs.stop()
执行
$ python recognize_video.py --detector face_detection_model \
--embedding-model openface_nn4.small2.v1.t7 \
--recognizer output/recognizer.pickle \
--le output/le.pickle
[INFO] loading face detector...
[INFO] loading face recognizer...
[INFO] starting video stream...
[INFO] elasped time: 12.52
[INFO] approx. FPS: 16.13
七、不足与提高识别精度的方法
7.1、更多的数据
gather more data — there are no “computer vision tricks” that will save you from the data gathering process.(更多的数据)。
Invest in your data and you’ll have a better OpenCV face recognition pipeline.?In general, I would recommend a?minimum of 10-20 faces per person
7.2、人脸对齐
参见?Face Alignment with OpenCV and Python.,OpenFace project.
人脸对齐包括(1)识别图片中人脸的几何机构。(2)通过平移、旋转、缩放实现正则对齐。
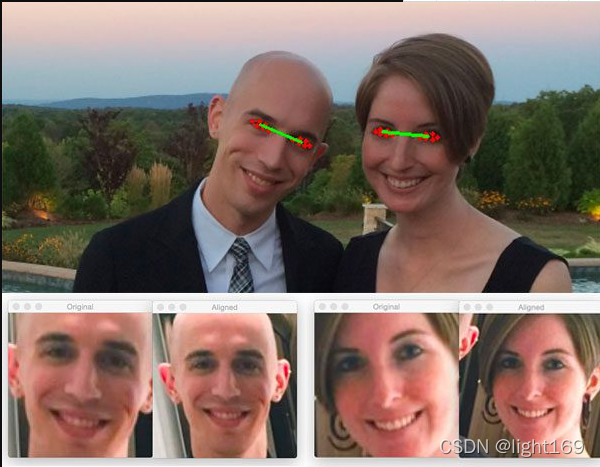
?
人脸对齐步骤:
- 在图片中检测人脸,提取ROI?(based on the bounding box coordinates).
- 根据facial landmark detection?提取双眼坐标.
- 沿双眼中点计算每只眼睛的中心矩.
- 根据中心矩和坐标对人脸仿射变换到固定大小和维度
如果数据集中的图片进行了人脸对齐,那么在输出时应该已经完成以下工作:
- 人脸居中.
- 根据双眼水平基线进行旋转,即双眼的y坐标一致
- 已经进行缩放,人脸大小基本一致.
?人脸对齐参见??Face Alignment with OpenCV and Python?专门说明。
7.3 参数调整
在SVM模型中,C参数是避免错误分类的严格程度,C越大越严格,越小错误分类会多。
OpenFace GitHub中建议用C=1
7.4?使用dlib’s embedding 模型 (不使用k-NN进行人脸识别)
参见?dlib’s face recognition model,?dlib’s face embeddings识别率更好,特别是小数据集。
并且dlib’s face embeddings模型更少依赖人脸是否对齐和是否采用更加强大的人脸检测的深度学习方法。
参见my original face recognition tutorial
八、其他人脸识别方法
8.1?siamese networks.
- Can be successfully trained with very little data
- Learn a similarity score between two images (i.e., how similar two faces are)
- Are the cornerstone of modern face recognition systems
参见
- Building image pairs for siamese networks with Python
- Siamese networks with Keras, TensorFlow, and Deep Learning
- Comparing images for similarity using siamese networks, Keras, and TensorFlow
- Contrastive Loss for Siamese Networks with Keras and TensorFlow
8.2?non-deep learning-based face recognition methods
These methods are less accurate than their deep learning-based face recognition counterparts, but tend to be much more computationally efficient and will run faster on embedded systems.
|