在上一篇中介绍了seq2seq for translation中decoder with attention的维度变化,这篇将介绍另一个pytorch教程chatbot中 attention decoder的部分
模型架构为传统的seq2seq模型 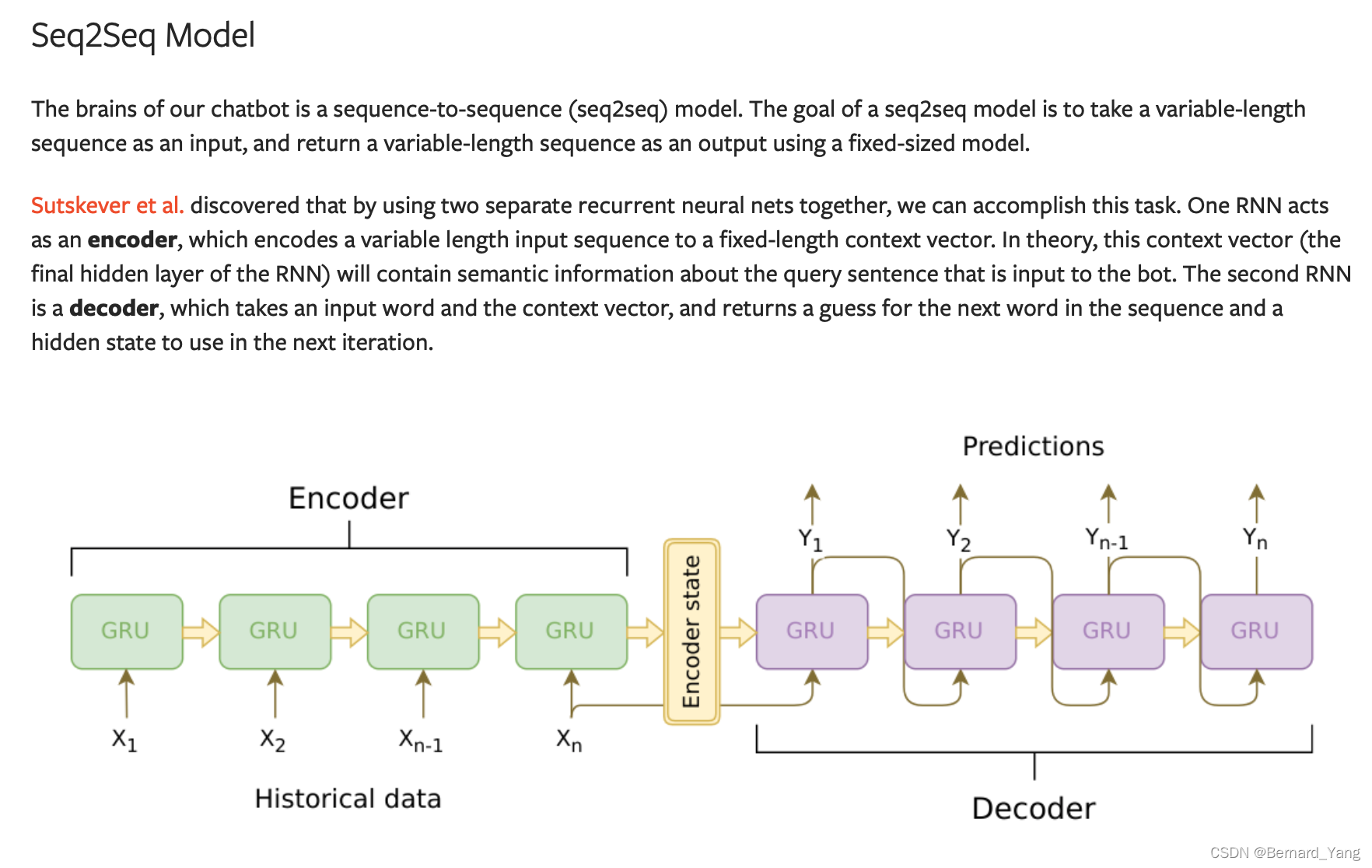 encoder负责将变长的句子转变为固定长度的vector,包括了句子的语义 decoder将input word和encoder输出的向量作为输入,每次输出一个output预测这个时间步的输出word,以及一个hidden作为下一次时间步的输入。
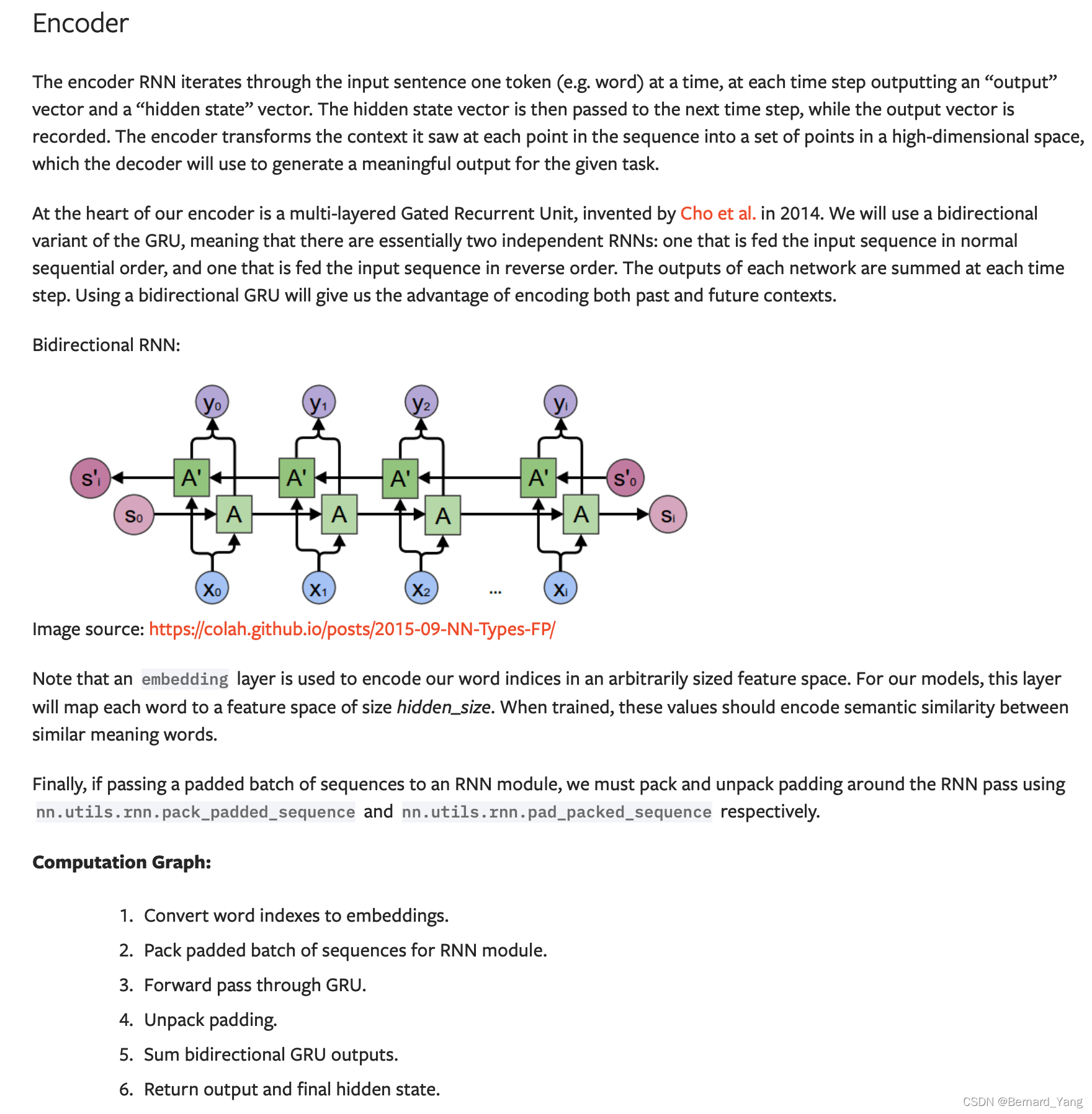
关于encoder的输入输出维度: 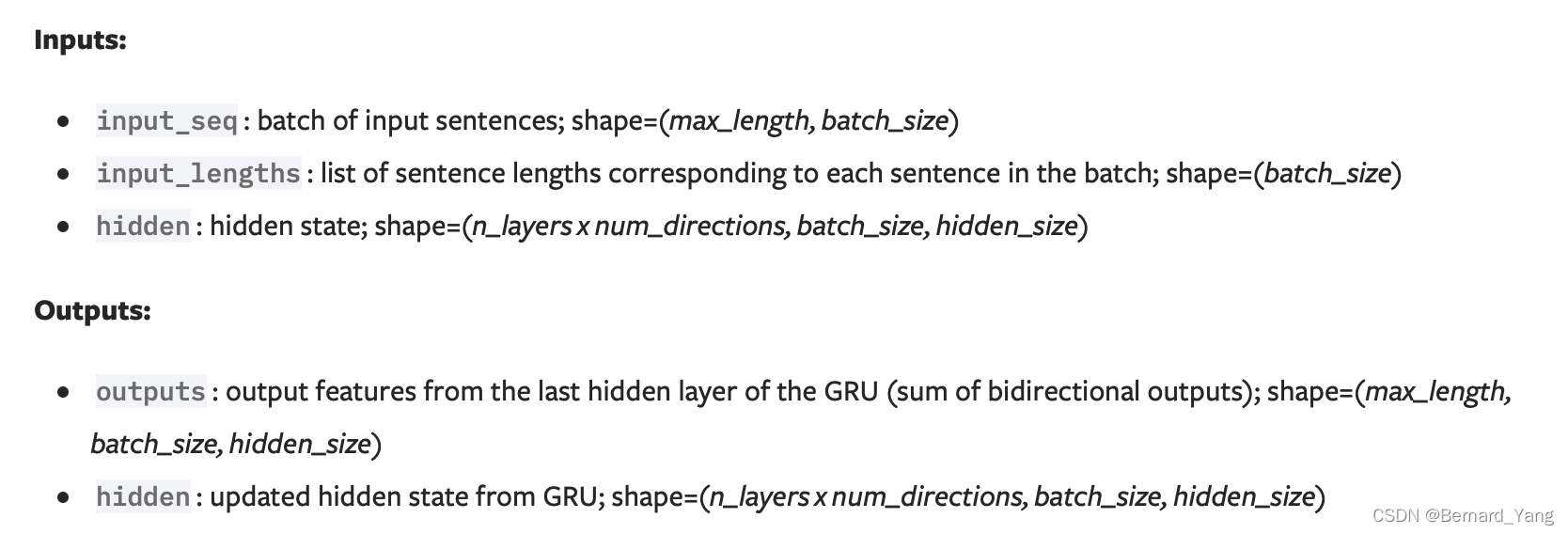
class EncoderRNN(nn.Module):
def __init__(self, hidden_size, embedding, n_layers=1, dropout=0):
super(EncoderRNN, self).__init__()
self.n_layers = n_layers
self.hidden_size = hidden_size
self.embedding = embedding
# Initialize GRU; the input_size and hidden_size params are both set to 'hidden_size'
# because our input size is a word embedding with number of features == hidden_size
self.gru = nn.GRU(hidden_size, hidden_size, n_layers,
dropout=(0 if n_layers == 1 else dropout), bidirectional=True)
def forward(self, input_seq, input_lengths, hidden=None):
# Convert word indexes to embeddings
embedded = self.embedding(input_seq)
# Pack padded batch of sequences for RNN module
packed = nn.utils.rnn.pack_padded_sequence(embedded, input_lengths)
# Forward pass through GRU
outputs, hidden = self.gru(packed, hidden)
# Unpack padding
outputs, _ = nn.utils.rnn.pad_packed_sequence(outputs)
# Sum bidirectional GRU outputs
outputs = outputs[:, :, :self.hidden_size] + outputs[:, : ,self.hidden_size:]
# Return output and final hidden state
return outputs, hidden
例如输入的input _seq维度: torch.Size([10, 5])代表最大句子长度为10,batch为5 lengths: tensor([10, 9, 9, 8, 8])代表了五个句子对应的长度
输出: encoder_outputs torch.Size([10, 64, 500]) encoder_hidden torch.Size([4, 64, 500]) nlayers=2,direction=2 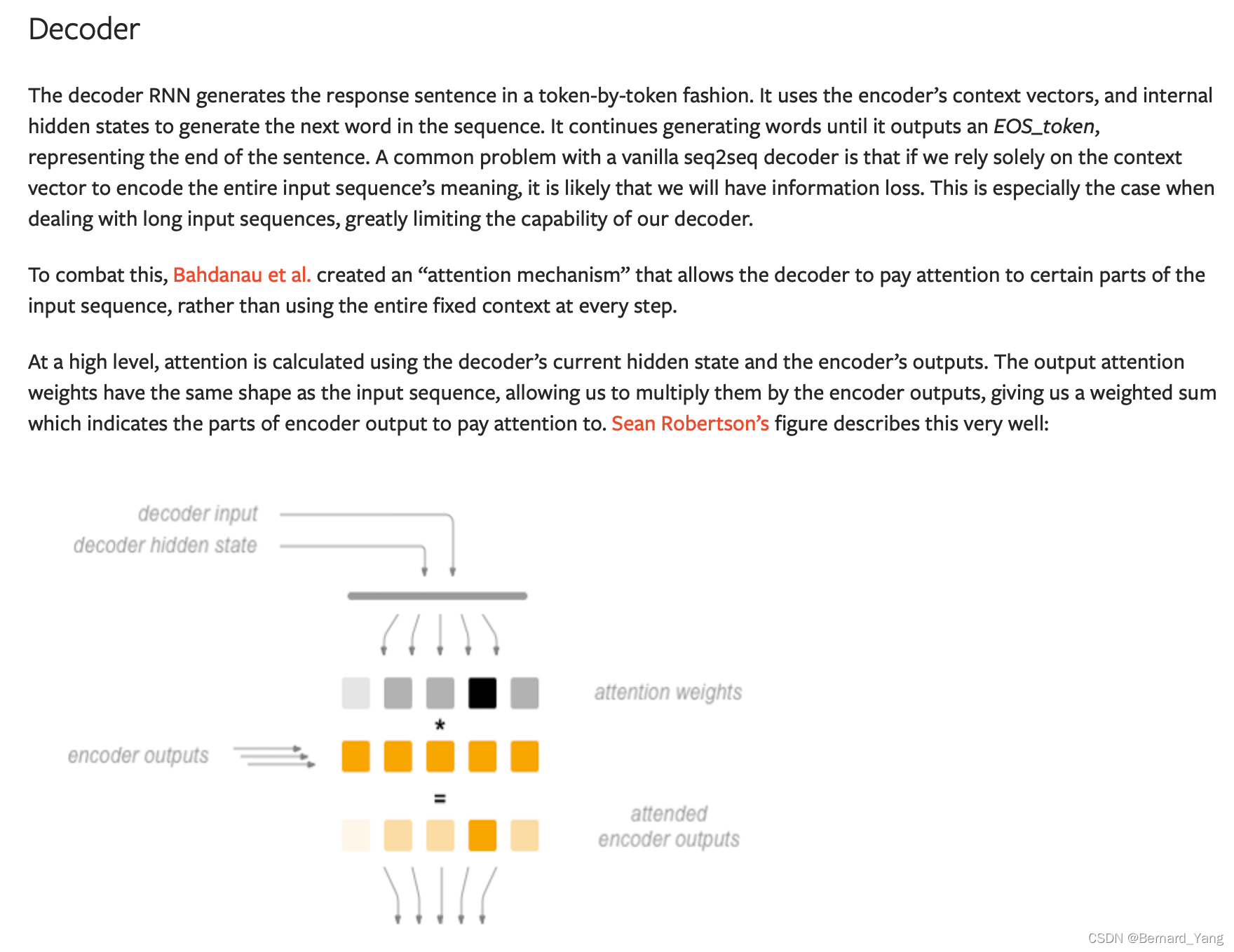
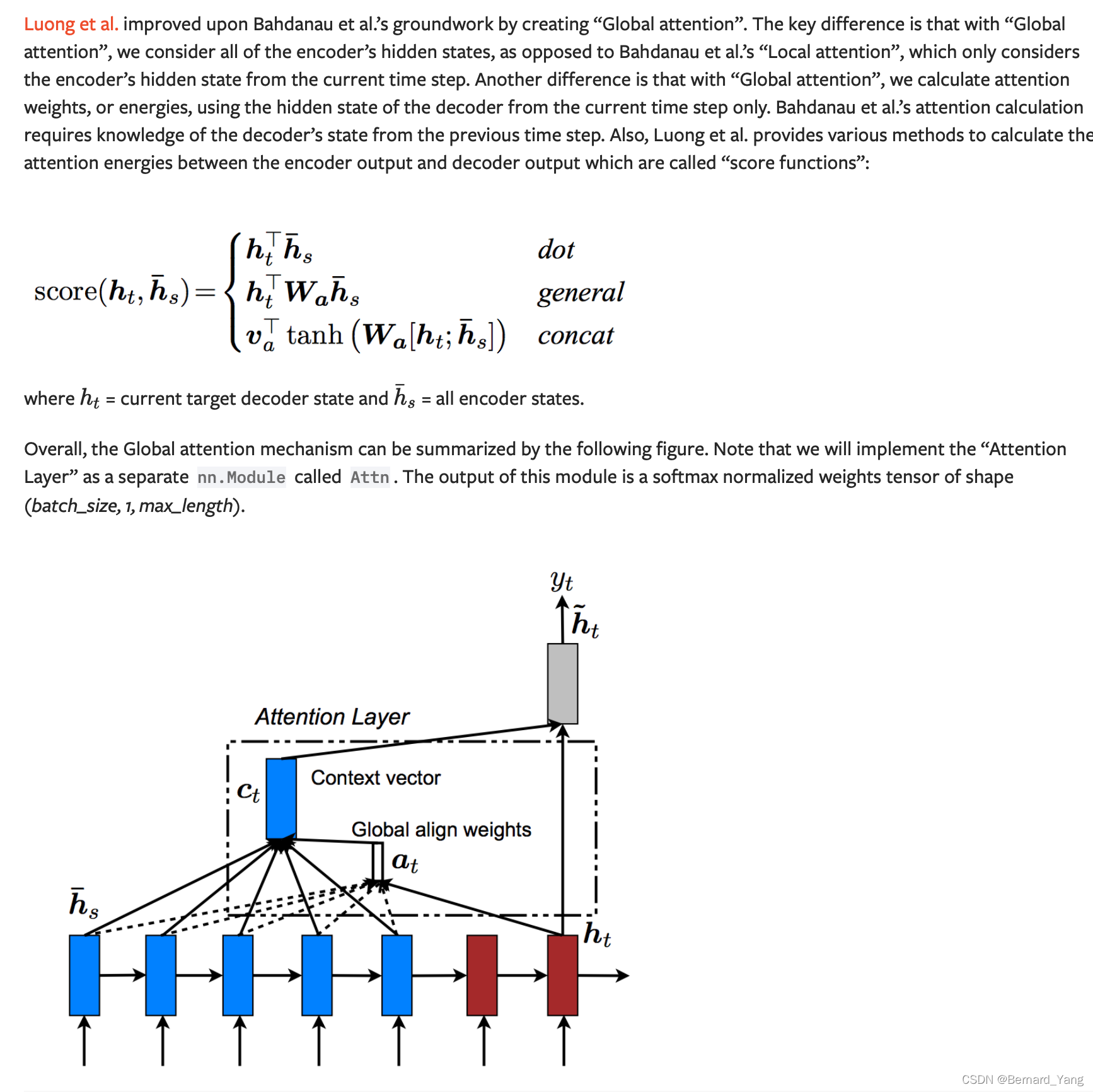
# Luong attention layer
class Attn(nn.Module):
def __init__(self, method, hidden_size):
super(Attn, self).__init__()
self.method = method
if self.method not in ['dot', 'general', 'concat']:
raise ValueError(self.method, "is not an appropriate attention method.")
self.hidden_size = hidden_size
if self.method == 'general':
self.attn = nn.Linear(self.hidden_size, hidden_size)
elif self.method == 'concat':
self.attn = nn.Linear(self.hidden_size * 2, hidden_size)
self.v = nn.Parameter(torch.FloatTensor(hidden_size))
def dot_score(self, hidden, encoder_output):
return torch.sum(hidden * encoder_output, dim=2)
def general_score(self, hidden, encoder_output):
energy = self.attn(encoder_output)
return torch.sum(hidden * energy, dim=2)
def concat_score(self, hidden, encoder_output):
energy = self.attn(torch.cat((hidden.expand(encoder_output.size(0), -1, -1), encoder_output), 2)).tanh()
return torch.sum(self.v * energy, dim=2)
def forward(self, hidden, encoder_outputs):
# Calculate the attention weights (energies) based on the given method
if self.method == 'general':
attn_energies = self.general_score(hidden, encoder_outputs)
elif self.method == 'concat':
attn_energies = self.concat_score(hidden, encoder_outputs)
elif self.method == 'dot':
attn_energies = self.dot_score(hidden, encoder_outputs)
# Transpose max_length and batch_size dimensions
attn_energies = attn_energies.t()
# Return the softmax normalized probability scores (with added dimension)
return F.softmax(attn_energies, dim=1).unsqueeze(1)
Attn返回的vector的维度为(batch_size, 1, max_length).
Computation Graph:
Get embedding of current input word. Forward through unidirectional GRU. Calculate attention weights from the current GRU output from (2). Multiply attention weights to encoder outputs to get new “weighted sum” context vector. Concatenate weighted context vector and GRU output using Luong eq. 5. Predict next word using Luong eq. 6 (without softmax). Return output and final hidden state.
代码中concat_output在Luong et al.中的公示表示 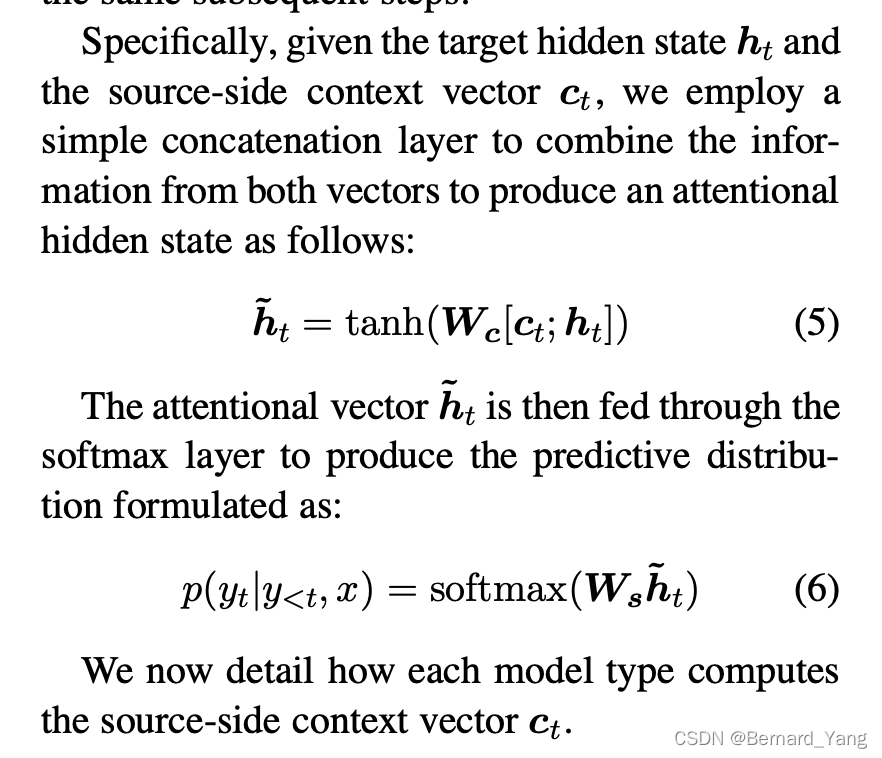 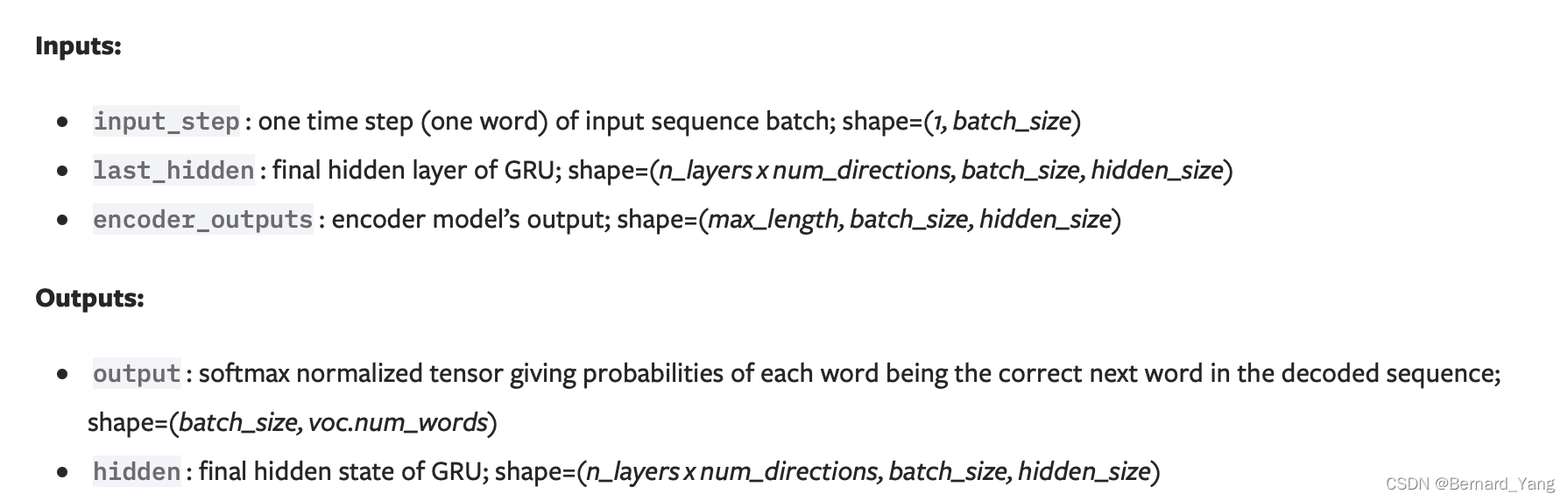
decoder输入的input_step维度为[1,batch] encoder_outputs torch.Size([max_length, batch, hidden])
经过embedding: embedded torch.Size([1, batch, hidden])
经过Gru: rnn_output torch.Size([1, batch, hidden]) hidden torch.Size([2, batch, hidden]) 因为layers * directions=2
经过attn: attn_weights torch.Size([batch, 1, max_length])
经过bmm: context torch.Size([batch, 1, hidden])
concat_input torch.Size([batch, 2*hidden]) concat_output torch.Size([batch, hidden])
经过out: output torch.Size([batch, hidden])
class LuongAttnDecoderRNN(nn.Module):
def __init__(self, attn_model, embedding, hidden_size, output_size, n_layers=1, dropout=0.1):
super(LuongAttnDecoderRNN, self).__init__()
# Keep for reference
self.attn_model = attn_model
self.hidden_size = hidden_size
self.output_size = output_size
self.n_layers = n_layers
self.dropout = dropout
# Define layers
self.embedding = embedding
self.embedding_dropout = nn.Dropout(dropout)
self.gru = nn.GRU(hidden_size, hidden_size, n_layers, dropout=(0 if n_layers == 1 else dropout))
self.concat = nn.Linear(hidden_size * 2, hidden_size)
self.out = nn.Linear(hidden_size, output_size)
self.attn = Attn(attn_model, hidden_size)
def forward(self, input_step, last_hidden, encoder_outputs):
# Note: we run this one step (word) at a time
# Get embedding of current input word
#torch.Size([1, batch, hidden])
embedded = self.embedding(input_step)
embedded = self.embedding_dropout(embedded)
# Forward through unidirectional GRU
#rnn_output torch.Size([1, batch, hidden])
#hidden torch.Size([2, batch, hidden]) 因为layers=2
rnn_output, hidden = self.gru(embedded, last_hidden)
# Calculate attention weights from the current GRU output
#attn_weights torch.Size([batch, 1, max_length])
attn_weights = self.attn(rnn_output, encoder_outputs)
# Multiply attention weights to encoder outputs to get new "weighted sum" context vector
#context torch.Size([batch, 1, hidden])
context = attn_weights.bmm(encoder_outputs.transpose(0, 1))
# Concatenate weighted context vector and GRU output using Luong eq. 5
rnn_output = rnn_output.squeeze(0)
context = context.squeeze(1)
#concat_input torch.Size([batch, 2*hidden])
#concat_output torch.Size([batch, hidden])
concat_input = torch.cat((rnn_output, context), 1)
concat_output = torch.tanh(self.concat(concat_input))
# Predict next word using Luong eq. 6
output = self.out(concat_output)
output = F.softmax(output, dim=1)
# Return output and final hidden state
#output torch.Size([batch, hidden])
#hidden torch.Size([2, batch, hidden])
return output, hidden
|