此篇为机器学习基础,如无Python基础,请移步Python入门系列学习:Python3基础系列博文索引.
1.基础
import numpy as np
scores = np.array([90, 100, 99, 98, 97, 98, 89, 100, 95, 96, 90])
scores_mean = np.mean(scores)
print("scores的平均值为:", scores_mean)
print("---------------------------------------------------------")
student_height = np.array([170, 172, 169, 168, 171, 180, 185])
student_height_median = np.median(student_height)
print("学生身高中值:", student_height_median)
print("---------------------------------------------------------")
from scipy import stats
scores1 = np.array([90, 100, 99, 98, 95, 95, 89, 100, 95, 96, 90])
scores1_mode = stats.mode(scores1)
print("分数众数为:", scores1_mode)
scores的平均值为: 95.63636363636364
---------------------------------------------------------
学生身高中值: 171.0
---------------------------------------------------------
分数众数为: ModeResult(mode=array([95]), count=array([3]))
import numpy as np
from scipy import stats
student_scores = np.array([99, 98, 97, 96, 99, 100, 97, 96, 90, 92, 91])
scores_mean = np.mean(student_scores)
scores_median = np.median(student_scores)
scores_mode = stats.mode(student_scores)
scores_std = np.std(student_scores)
print("分数均值:", scores_mean)
print("分数中位数:", scores_median)
print("分数众数:", scores_mode)
print("分数标准差:", scores_std)
print("---------------------------------------------------------")
scores_var = np.var(student_scores)
print("分数方差:", scores_var)
分数均值: 95.9090909090909
分数中位数: 97.0
分数众数: ModeResult(mode=array([96]), count=array([2]))
分数标准差: 3.2600765594620196
---------------------------------------------------------
分数方差: 10.62809917355372
2.直方图
import numpy as np
import matplotlib.pyplot as plt
student_scores = np.array([99, 98, 97, 96, 99, 100, 97, 96, 90, 92, 91])
scores_percentile100 = np.percentile(student_scores, 100)
scores_percentile95 = np.percentile(student_scores, 95)
scores_percentile85 = np.percentile(student_scores, 85)
scores_percentile80 = np.percentile(student_scores,80)
scores_percentile75 = np.percentile(student_scores, 75)
print("100%的学生分数是:", scores_percentile100)
print("95%的学生分数是:", scores_percentile95)
print("85%的学生分数是:", scores_percentile85)
print("80%的学生分数是:", scores_percentile80)
print("75%的学生分数是:", scores_percentile75)
print("------------------------------------------------------------------")
float_array1 = np.random.uniform(0.0, 5.0, 100)
print("100个介于0-5的随机浮点数的数组:\n", float_array1)
plt.hist(float_array1, 15)
plt.show()
print("------------------------------------------------------------------")
float_array2 = np.random.uniform(0.0, 10.0, 10000000)
plt.hist(float_array2, 10000)
plt.show()
100%的学生分数是: 100.0
95%的学生分数是: 99.5
85%的学生分数是: 99.0
80%的学生分数是: 99.0
75%的学生分数是: 98.5
------------------------------------------------------------------
100个介于0-5的随机浮点数的数组:
[3.55779127 1.0898295 2.88230074 2.91016949 2.70223263 0.5073136
1.91294576 4.02741398 1.77624827 2.62066579 1.88800846 1.82242203
0.47935634 1.02495598 0.64864446 0.80027518 0.65393513 1.32705114
2.95345842 0.45476184 1.77865828 0.3705677 0.20129932 3.97919018
4.80611527 2.75086995 2.29628742 2.86638662 2.98029015 1.11190308
3.82370503 4.33425818 2.66986368 2.15876732 3.77721441 3.62689171
4.07859732 0.82228595 0.39015825 0.34793828 2.58501242 0.29148589
2.86000172 2.62013179 1.79370237 0.16398119 2.94452524 3.72505094
1.84285272 1.3931879 2.81048161 0.48507558 0.88914312 3.47251292
2.72593175 4.45096033 1.78735771 2.56998716 4.16554642 1.73999731
1.66290151 0.10536236 3.81931982 1.26553089 2.263162 1.89819717
4.14928293 2.1153283 4.69587509 3.46279032 3.73848111 4.33138596
0.19568642 3.32156084 4.04812723 1.97195288 1.49315545 3.94315293
0.01906249 0.5646791 0.51415783 2.42077592 3.49763022 3.05110296
3.83902844 2.04113564 4.85806299 3.13167761 3.62689246 1.74706561
1.41927227 2.43478907 3.58531807 0.92286333 2.12511765 2.36192296
2.91134094 2.83906127 2.19326338 3.29235014]
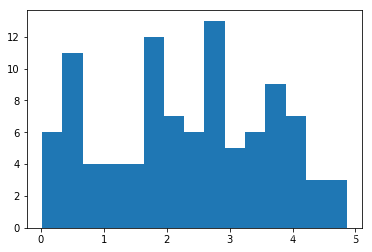 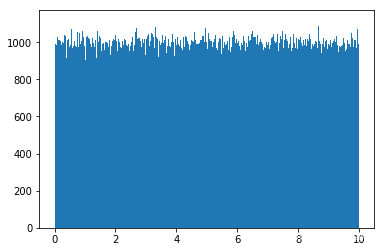
3.正态分布图
import numpy as np
import matplotlib.pyplot as plt
random_array1 = np.random.normal(5.0, 1.0, 10000000)
plt.hist(random_array1, 1000)
plt.show()
print("--------------------------------------------------------")
car_years = [5,7,8,7,2,17,2,9,4,11,12,9,6,7,8,10,15,16]
car_speed = [99,86,87,88,111,86,103,87,94,78,77,85,86,100,99,90,95,85]
plt.scatter(car_years, car_speed)
plt.show()
print("--------------------------------------------------------")
x_array = np.random.normal(5.0, 1.0, 1000000)
y_array = np.random.normal(10.0, 2.0, 1000000)
plt.scatter(x_array, y_array)
plt.show()
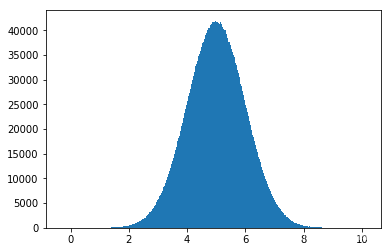 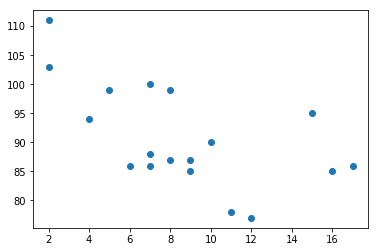 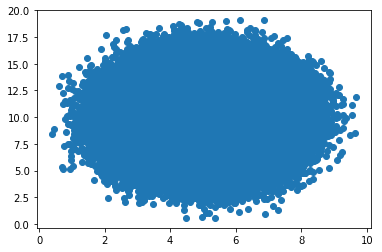
4.线性回归
import numpy as np
import matplotlib.pyplot as plt
from scipy import stats
car_years = [5,7,8,7,2,17,2,9,4,11,12,9,6]
car_speed = [99,86,87,88,111,86,103,87,94,78,77,85,86]
slope, intercept, r, p, std_err = stats.linregress(car_years, car_speed)
print("数据在线性回归中的拟合度:", r)
def linregress_func(x):
return slope * x + intercept
car_10_speed = linregress_func(10)
print("预测一辆拥有10年车龄的汽车速度:", car_10_speed)
linear_model = list(map(linregress_func, car_years))
plt.scatter(car_years, car_speed)
plt.plot(car_years, linear_model)
plt.show()
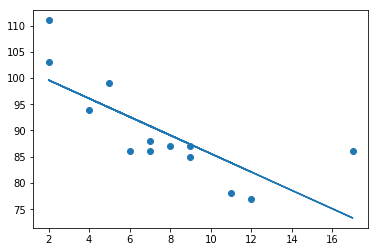
5.多项式回归
import matplotlib.pyplot as plt
import numpy as np
from sklearn.metrics import r2_score
through_time = [1,2,3,5,6,7,8,9,10,12,13,14,15,16,18,19,21,22]
car_speed = [100,90,80,60,60,55,60,65,70,70,75,76,78,79,90,99,99,100]
mymodel1 = np.poly1d(np.polyfit(through_time, car_speed, 3))
mymodel2 = np.poly1d(np.polyfit(through_time, car_speed, 4))
mymodel3 = np.poly1d(np.polyfit(through_time, car_speed, 5))
myline = np.linspace(1, 22, 100)
plt.scatter(through_time, car_speed)
plt.plot(myline, mymodel1(myline))
plt.title("mymodel1")
plt.xlabel("through_time")
plt.ylabel("car_speed")
plt.show()
plt.scatter(through_time, car_speed)
plt.plot(myline, mymodel2(myline))
plt.title("mymodel1")
plt.xlabel("through_time")
plt.title("mymodel2")
plt.show()
plt.scatter(through_time, car_speed)
plt.plot(myline, mymodel3(myline))
plt.title("mymodel1")
plt.xlabel("through_time")
plt.title("mymodel3")
plt.show()
print("model1拟合度:", r2_score(car_speed, mymodel1(through_time)))
print("model2拟合度:", r2_score(car_speed, mymodel2(through_time)))
print("model3拟合度:", r2_score(car_speed, mymodel3(through_time)))
car_speed1 = mymodel1(17)
car_speed2 = mymodel2(17)
car_speed3 = mymodel3(17)
print("model1预测17时的速度:", car_speed1)
print("model2预测17时的速度:", car_speed2)
print("model3预测17时的速度:", car_speed3)
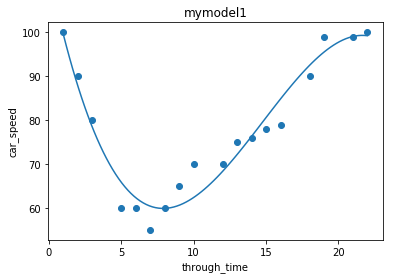 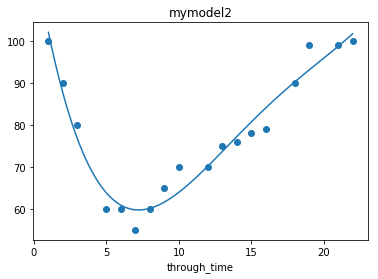 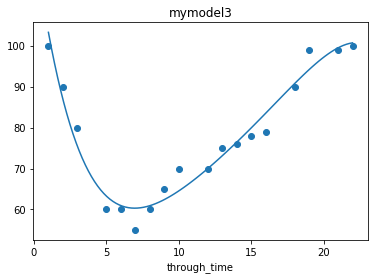
model1拟合度: 0.9432150416451026
model2拟合度: 0.9542030834699506
model3拟合度: 0.9568460139893514
model1预测17时的速度: 88.87331269697985
model2预测17时的速度: 87.21097471965412
model3预测17时的速度: 87.13740700265295
6.多元回归、缩放
import pandas as pd
import numpy as np
import matplotlib.pyplot as plt
from sklearn import linear_model
cars_data = pd.read_csv("cars.csv")
X = cars_data[["Weight", "Volume"]]
y = cars_data["CO2"]
regre = linear_model.LinearRegression()
regre.fit(X, y)
predictCO2 = regre.predict([[2300, 1300]])
print("重量2300kg,排量1300ccm汽车的CO2排放量:", predictCO2)
import pandas as pd
from sklearn import linear_model
from sklearn.preprocessing import StandardScaler
scale = StandardScaler()
cars_data = pd.read_csv("cars.csv")
X = cars_data[["Weight", "Volume"]]
scaledX = scale.fit_transform(X)
print("原始前10行数据:\n", X[:10])
print("----------------------------")
print("缩放后前10行数据:\n", scaledX[:10])
原始前10行数据:
Weight Volume
0 790 1000
1 1160 1200
2 929 1000
3 865 900
4 1140 1500
5 929 1000
6 1109 1400
7 1365 1500
8 1112 1500
9 1150 1600
----------------------------
缩放后前10行数据:
[[-2.10389253 -1.59336644]
[-0.55407235 -1.07190106]
[-1.52166278 -1.59336644]
[-1.78973979 -1.85409913]
[-0.63784641 -0.28970299]
[-1.52166278 -1.59336644]
[-0.76769621 -0.55043568]
[ 0.3046118 -0.28970299]
[-0.7551301 -0.28970299]
[-0.59595938 -0.0289703 ]]
7.训练/测试
import numpy as np
import matplotlib.pyplot as plt
from sklearn.metrics import r2_score
np.random.seed(2)
time_before_shopping = np.random.normal(3, 1, 1000)
money_speed = np.random.normal(150, 40, 1000) / time_before_shopping
plt.scatter(time_before_shopping, money_speed)
plt.title("Initial data")
plt.xlabel("time_before_shopping")
plt.ylabel("money_speed")
plt.show()
train_x = time_before_shopping[:800]
train_y = money_speed[:800]
test_x = time_before_shopping[800:]
test_y = money_speed[800:]
plt.scatter(train_x, train_y)
plt.title("Train data")
plt.xlabel("time_before_shopping")
plt.ylabel("money_speed")
plt.show()
plt.scatter(test_x, test_y)
plt.title("Test data")
plt.xlabel("time_before_shopping")
plt.ylabel("money_speed")
plt.show()
print("==============================================================")
model1 = np.poly1d(np.polyfit(train_x, train_y, 3))
model2 = np.poly1d(np.polyfit(train_x, train_y, 4))
model3 = np.poly1d(np.polyfit(train_x, train_y, 5))
myline = np.linspace(0, 7, 1000)
plt.scatter(train_x, train_y)
plt.plot(myline, model1(myline))
plt.title("Model1")
plt.xlabel("time_before_shopping")
plt.ylabel("money_speed")
plt.show()
plt.scatter(train_x, train_y)
plt.plot(myline, model2(myline))
plt.title("Model2")
plt.xlabel("time_before_shopping")
plt.ylabel("money_speed")
plt.show()
plt.scatter(train_x, train_y)
plt.plot(myline, model3(myline))
plt.title("Model3")
plt.xlabel("time_before_shopping")
plt.ylabel("money_speed")
plt.show()
print("==============================================================")
model_match1 = r2_score(train_y, model1(train_x))
model_match2 = r2_score(train_y, model2(train_x))
model_match3 = r2_score(train_y, model3(train_x))
print("model1中数据拟合度:", model_match1)
print("model2中数据拟合度:", model_match2)
print("model3中数据拟合度:", model_match3)
print("---------------------------------------------------------------")
test_match1 = r2_score(test_y, model1(test_x))
test_match2 = r2_score(test_y, model2(test_x))
test_match3 = r2_score(test_y, model3(test_x))
print("test_model1中数据拟合度:", test_match1)
print("test_model2中数据拟合度:", test_match2)
print("test_model3中数据拟合度:", test_match3)
print("---------------------------------------------------------------")
print("model1预测停留5分钟,花费:", model1(5))
print("model2预测停留5分钟,花费:", model2(5))
print("model3预测停留5分钟,花费:", model3(5))
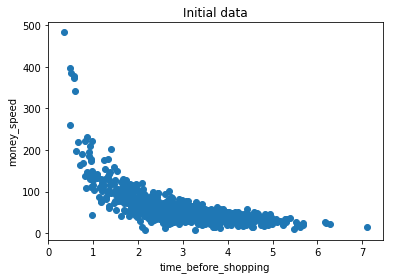 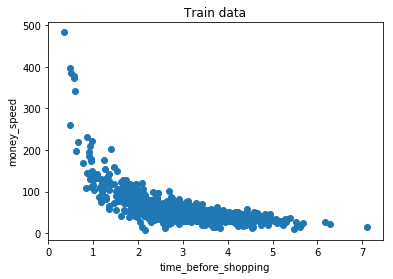 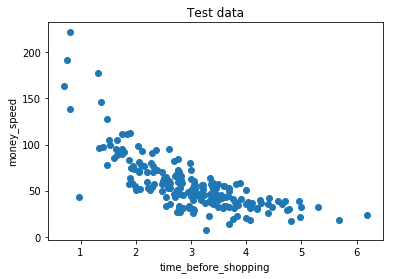 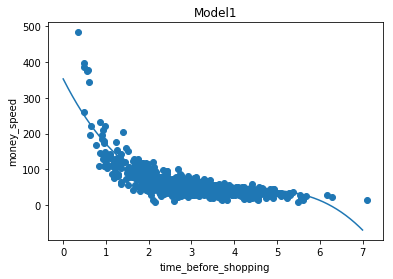 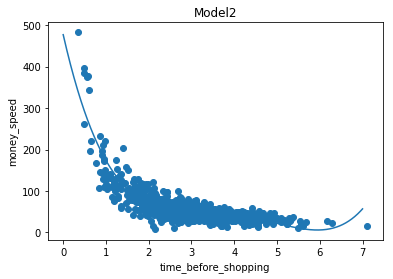 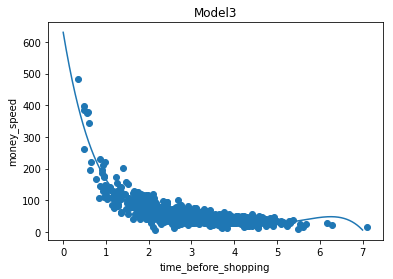
model1中数据拟合度: 0.7217325821712026
model2中数据拟合度: 0.7741924031491744
model3中数据拟合度: 0.8054110624472733
---------------------------------------------------------------
test_model1中数据拟合度: 0.6226812568805811
test_model2中数据拟合度: 0.5962872580670096
test_model3中数据拟合度: 0.5892020248634098
---------------------------------------------------------------
model1预测停留5分钟,花费: 40.538606323156614
model2预测停留5分钟,花费: 21.835783087172274
model3预测停留5分钟,花费: 27.73065855759296
|