中文文本纠错任务是一项NLP基础任务,其输入是一个可能含有语法错误的中文句子,输出是一个正确的中文句子。语法错误类型很多,有多字、少字、错别字等,目前最常见的错误类型是错别字。
1. SoftMaskedBert4CSC
-
论文 【ACL 2020】《Spelling Error Correction with Soft-Masked BERT》https://arxiv.org/abs/2005.07421 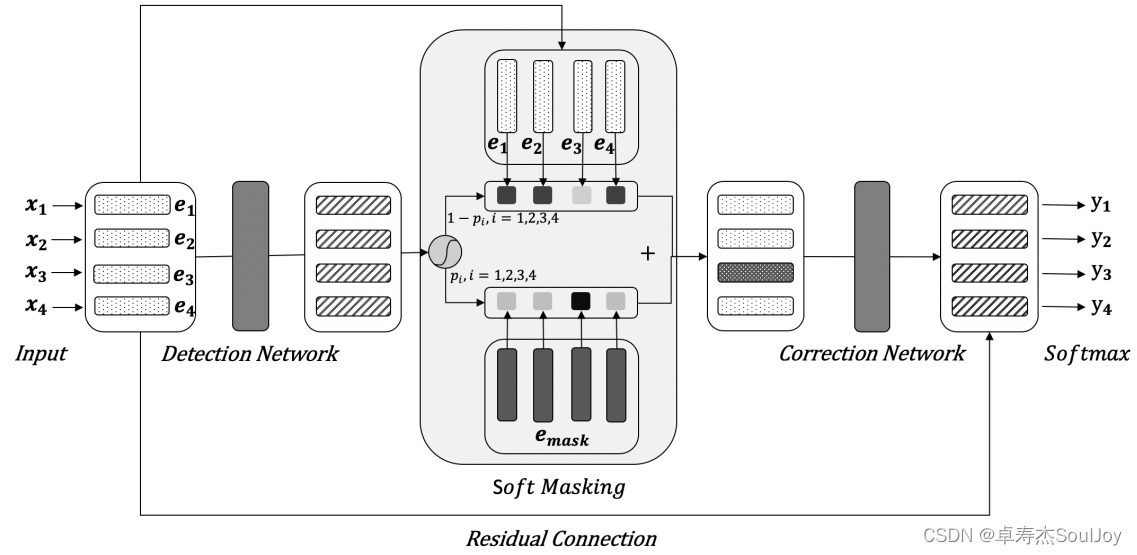 -
Detection 首先,模型的输入是n个中文字符X=(x1,x2,… ,xn)经过embeddings得到的E = (e1,e2,…,en),该embeding是word embeding+position embeding+segment embeding,经过Bi-GRU得到各个字符错误的概率G = (g1,g2,…,gn),其中g在0-1之间,越靠近1表示该字符错误的概率越大,其损失函数为: 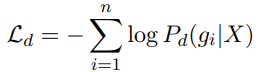 -
soft-masked 作者认为只hard-mask了15%字符的Bert不完全具备纠错的能力(至于为啥不具备,作者也没讲清楚,我觉得这里有些牵强),所以作者提出了soft-mask,大致的思路就是利用Detection输出的得分来引导Bert输入的mask,使得得分高(错误概率高)的地方更大概率被mask,公式如下:  -
Correction Correction的输入是经过soft-masked的embeding,输出的是生成的字符,损失函数是: 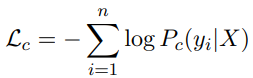
- Loss
模型没有分阶段训练,而是直接end-to-end,使用Bert的per-trained模型,损失函数由Detection和Correction线性组合,如下: 
2. MacBert4CSC
MacBert 可以参阅 :https://blog.csdn.net/u011239443/article/details/121820752?spm=1001.2014.3001.5502
MacBert4CSC:https://github.com/shibing624/pycorrector/blob/master/pycorrector/macbert/README.md
本项目是 MacBERT 改变网络结构的中文文本纠错模型,可支持 BERT 类模型为 backbone。
在通常 BERT 模型上进行了魔改,追加了一个全连接层作为错误检测即 detection, 与 SoftMaskedBERT 模型不同点在于,本项目中的 MacBERT 中,只是利用 detection 层和 correction 层的 loss 加权得到最终的 loss。不像 SoftmaskedBERT 中需要利用 detection 层的置信概率来作为 correction 的输入权重。 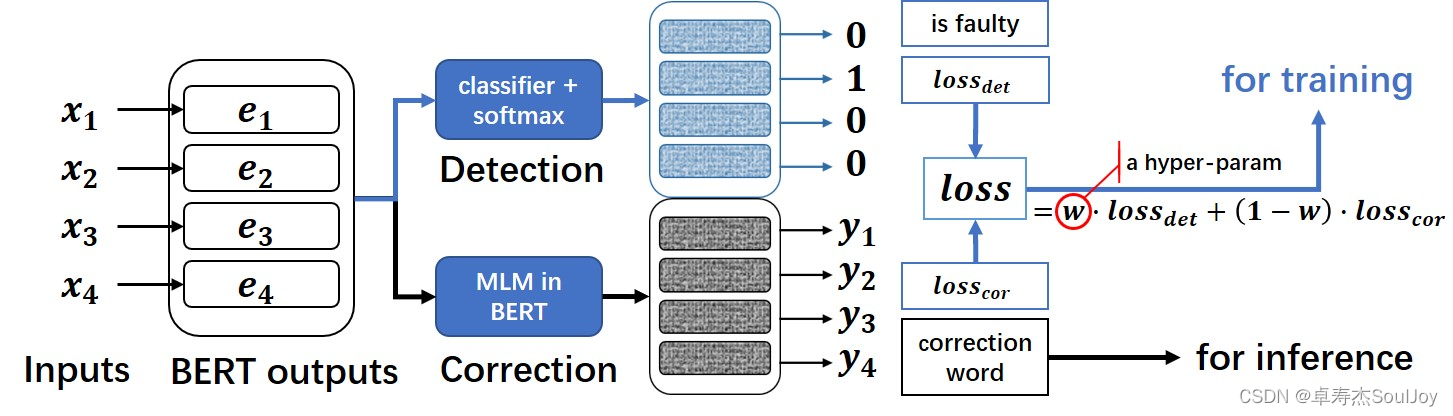
3. ErnieCSC
Ernie参阅:https://blog.csdn.net/u011239443/article/details/121820752?spm=1001.2014.3001.5502
ErnieCSC PaddleNLP模型库实现了百度在ACL 2021上提出结合拼音特征的Softmask策略的中文错别字纠错的下游任务网络,并提供预训练模型,模型结构如下:
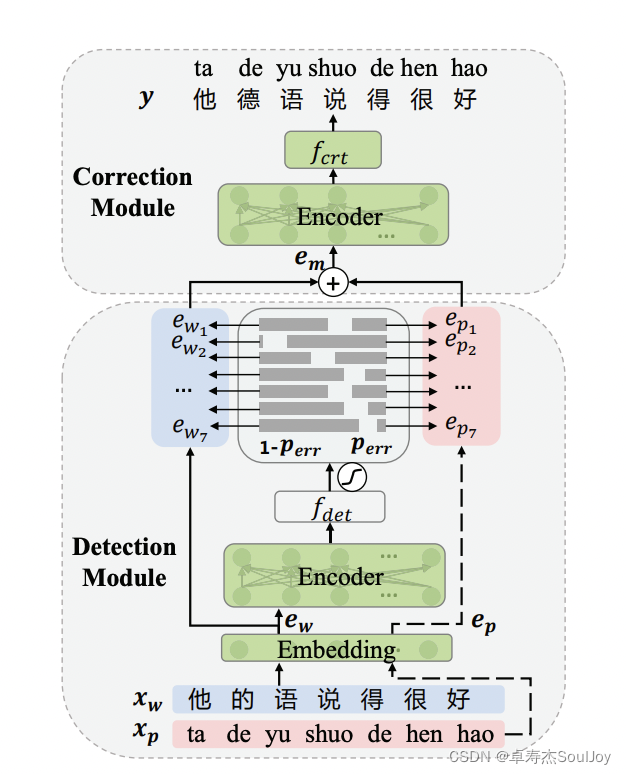 PyTorch实现版本:https://github.com/orangetwo/ernie-csc
|