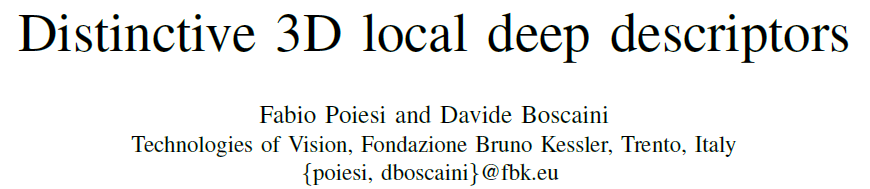 DIP[1]属于一种基于PointNet[2]网络的比较简洁的two-stage点云特征提取器,因此文章的重点并不在网络设计上面,而在输入数据local patches的准备上。因此生成正确的local patches以使用PointNet进行特征提取成为DIP的关键。
Dataset Preparation
按照DIP的格式要求,于作者提供的google drive中下载好3DMatch_train.zip与3DMatch_test.zip,解压后数据组织形式如下: ├── 3DMatch_train │ ├── 7-scenes-chess/ │ ├── 7-scenes-fire/ │ ├── … ├── 3DMatch_test │ ├── 7-scenes-redkitchen/ │ ├── sun3d-home_md-home_md_scan9_2012_sep_30/ │ ├── … 在训练集中,在每个scene下都在folder 01_data下保存有每个点云fragment的cloud_bin_.ply文件,其次是将每个fragment对齐至全局坐标系下的pose信息,存储于cloud_bin_.info.txt中。同时folder Correspondences中存储着这个scene下,所有对应pair的correspondences indices信息。(按照作者的说法,这里的correspondences信息均是在19年的3DSN[3]中计算得到的,这些信息在这里的主要作用主要是提供哪些fragment之间构成pair,而其中具体的correspondences indices并不使用,DIP选择重新计算这些indices) 在测试集中,每个scene下同样有folder 01_data保存*.ply,同时在folder 02_T中将gt transformation存储于*_*.pkl中。 接着便是最繁琐的对输入local patches的pre-computing部分,首先给出处理完后的数据组成: ├── 3DMatch_train_pre │ ├── correspondences/ │ ├── lrfs/ │ ├── patches_lrf/ │ ├── points_lrf/ │ ├── rotations_lrf/ ├── 3DMatch_test_pre │ ├── lrfs/ │ ├── points_lrf/ │ ├── patches_lrf/ │ ├── 7-scenes-redkitchen.hdf5 │ ├── … preprocess_3dmatch_correspondences_train/test.py 核心作用是利用folder Correspondences下保存的pair信息,以及gt transform信息,利用open3d[4]中的ICP API重新计算correspondence,并保存于3DMatch_train_pre/correspondences/scene_name.hdf5中。 preprocess_3dmatch_lrf_train/test.py 这一步主要在完成以下步骤,即corresponding local patches的预计算,即离线计算训练所需数据,加速网络训练过程。 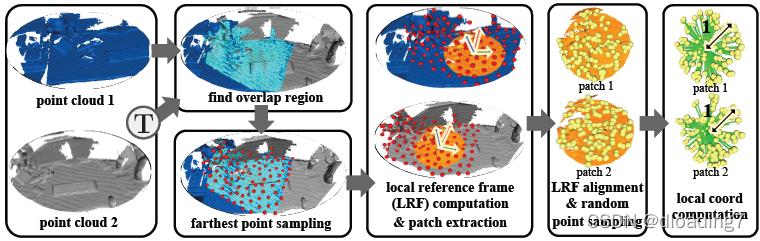 这里用pcd1、pcd2代表point cloud 1,point cloud 2. 按照图中的逻辑,overlap region的寻找已经由上一步:correspondences的计算中得到,这是由于只有处于overlap region中的点才有可能找到correspondences. 这里使用pcd1_corr以及pcd2_corr代表两点云中处于overlap region中的部分。接着对pcd1与pcd2进行voxel_size=0.01的down sampling,得到pcd1_down, pcd2_down. 接着对pcd1_corr与pcd2_corr进行FPS采样,分别采得256个centroids,再接下来以这些centriods为球心,在pcd1_down以及pcd2_down中构造点数为256的local patch,并用3DSN中的方法计算Local Reference Frame(LRF),将patch内的点x、y、z轴与LRF对齐以形成canonical representation.接着保存:
- local patches于folder patches_lrf中
- LRFs于folder lrfs中
- centroids于folder points_lrf中
- gt transform于folder rotations_lrf中
至此,数据pre-computing阶段已完成,接着便是对patch进行特征提取的步骤。
Feature Extractor
首先给出网络结构图: 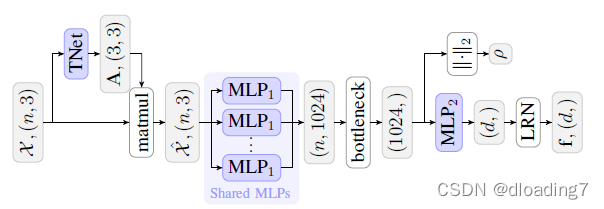 对于输入的local patch,即图中的
X
X
X,由以上数据准备过程中的已知信息可知,patch大小为(256, 3),即上图的n=256. 首先采用一个TNet产生一个transformation作用于patch
X
X
X上,这一步的目的与PointNet[2]中的TNet相同,即学习一个仿射变换以进一步消除旋转即平移不变性,得到
X
^
\hat X
X^. TNet部分的网络示意图如下: 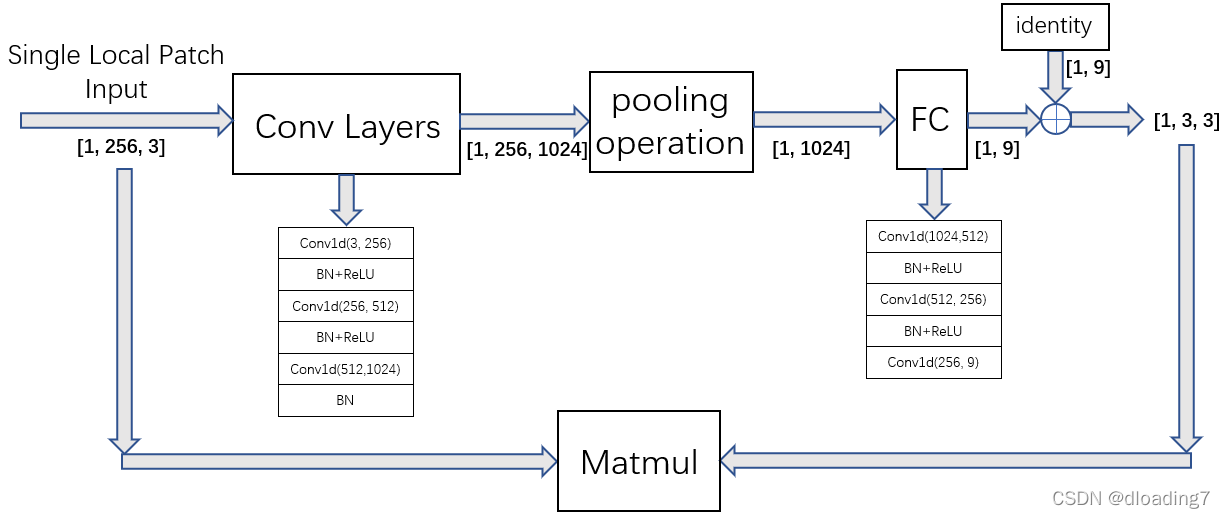 可以看到作用就是以patch自身作为输入产生一个transformation,并作用于自身。 接着便是比较常规的PointNet[2]-style特征提取网络,示意图如下: 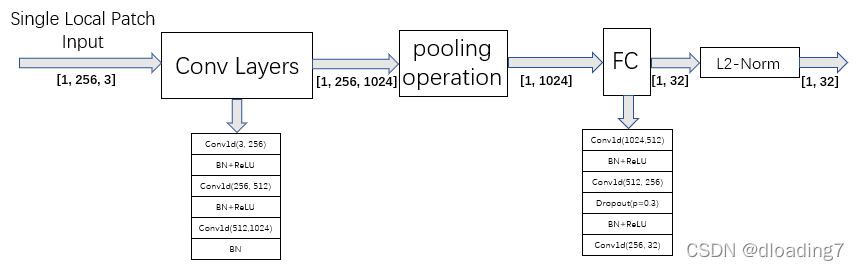 可以看到与TNet基本没有什么区别,无非是多了一个Dropout层,最终输出为对应local patches的32维descriptors.
Loss
网络整体架构流程如下: 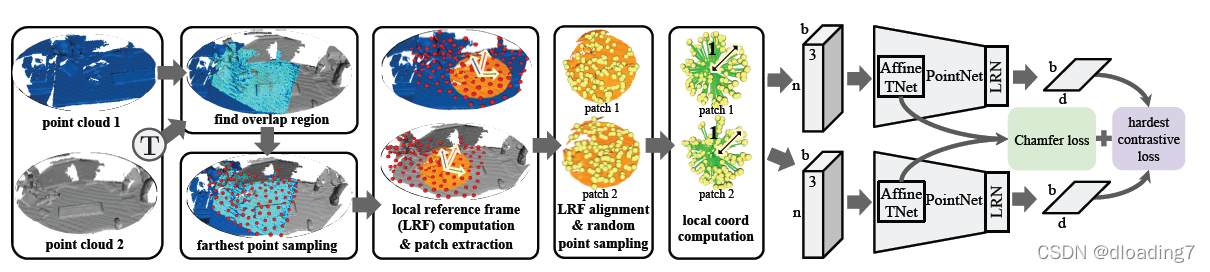 网络是一个Siamese network,输入为两个batch的patches,每个batch大小为(256, 256, 3). loss主要由两部分构成,Chamfer loss促使两个相同的经过仿射变换作用过的positive patch在欧氏空间上尽量接近。
X
X
X、
A
A
A为第一个patch与其仿射变换,
X
′
X'
X′、
A
′
A'
A′为第二个patch与其仿射变换: 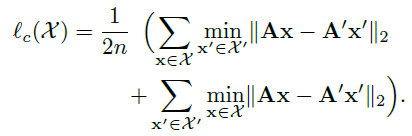 监督descriptor部分使用的是FCGF[5]中的Hardest Contrastive Loss: 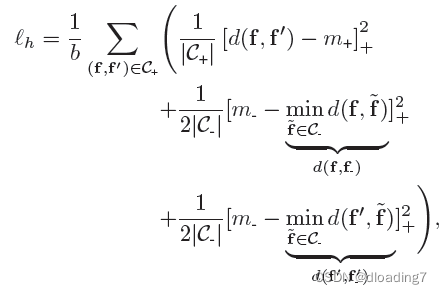 即在一个batch内,对每对postive pair
(
f
,
f
′
)
∈
C
+
(f,f') \in {C_ + }
(f,f′)∈C+?, 分别挖掘
f
f
f与
f
′
f'
f′的hardest negatives加以监督。 最终的loss由以上两个loss equally weighted组成。
Analysis
对于DIP,整篇文章最重要的部分,是指出在输入local patches经过PointNet[2]-style的特征提取网络时,经过max-pooling后得到的global vectors
Y
Y
Y在某种程度上反映了这个patch的informative程度。具体表现为vectors
Y
Y
Y的模长反映了patch内信息的”显著“程度: 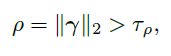
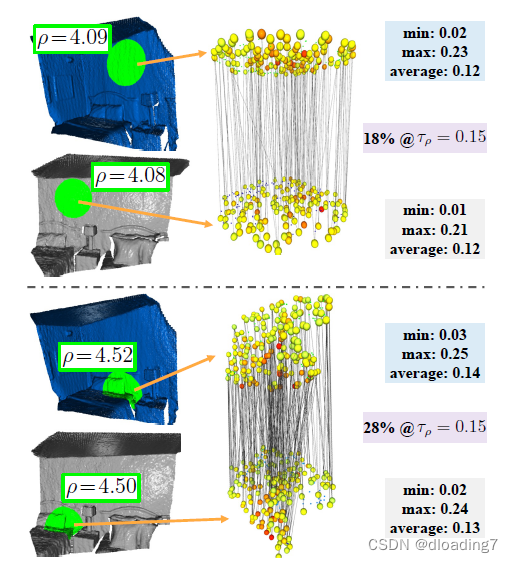 由上图可以看出,当patch位于平面区域flat surface即信息比较不显著的区域时,模长值较小;当patch位于一些结构性区域structured area时,模长值较大。基于以上观察,作者提出利用global vectors的模长,卡一个阈值,来去除掉不这些位于不”显著“区域patch所提取的特征,而只用patch位于”显著“区域处提取的特征去做匹配。 阈值的选取也比较简单,卡掉模长较小(后5%)的那些descriptors,而留下前95%的descriptors进行匹配。
Experiment
由于DIP只给了evaluate feature match recall(FMR)的script而没有evaluate registration recall(RR)的script,自己是可以实现的,但由于时间关系,这里就略过对RR的评估。只在一个数据集3DMatch评估FMR指标。 按照作者预设的参数,训练40个EPOCH,进行FMR的评估,结果如下: 首先是文章中FMR的结果: 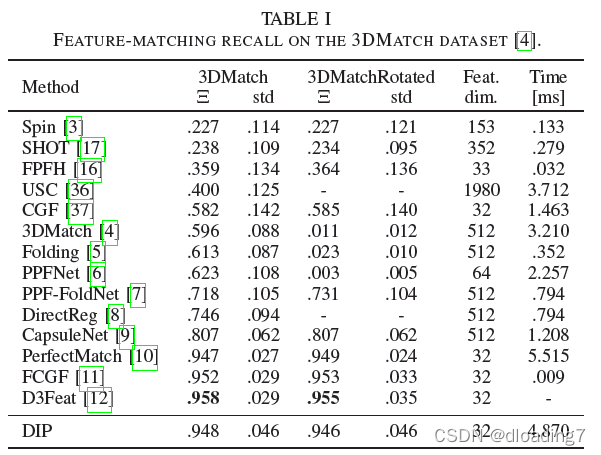 接着是分别测试作者提供的预训练权重final_chkpt.pth与自己训练得到的权重ckpt_e39_i9600_dim32.pth得到的FMR结果:
| 3DMatch | 3DMatch |
---|
sample | 5k | 5k | weight | final_chkpt.pth | ckpt_e39_i9600_dim32.pth | 7-scenes-redkitchen | .984 | .986 | sun3d-home_at-home_at_scan1_2013_jan_1 | .948 | .955 | sun3d-home_md-home_md_scan9_2012_sep_30 | .903 | .903 | sun3d-hotel_uc-scan3 | .991 | .991 | sun3d-hotel_umd-maryland_hotel1 | .971 | .971 | sun3d-hotel_umd-maryland_hotel3 | 1.0 | 1.0 | sun3d-mit_76_studyroom-76-1studyroom2 | .941 | .948 | sun3d-mit_lab_hj-lab_hj_tea_nov_2_2012_scan1_erika | .857 | .844 | avg | .949 | .950 |
可以看到FMR的复现结果与论文中的结果是基本一致的。
Generalization Ability
To do …
References
[1] Poiesi F, Boscaini D. Distinctive 3D local deep descriptors[C]//2020 25th International conference on pattern recognition (ICPR). IEEE, 2021: 5720-5727. [2] Qi C R, Su H, Mo K, et al. Pointnet: Deep learning on point sets for 3d classification and segmentation[C]//Proceedings of the IEEE conference on computer vision and pattern recognition. 2017: 652-660. [3] Gojcic Z, Zhou C, Wegner J D, et al. The perfect match: 3d point cloud matching with smoothed densities[C]//Proceedings of the IEEE/CVF Conference on Computer Vision and Pattern Recognition. 2019: 5545-5554. [4] Zhou Q Y, Park J, Koltun V. Open3D: A modern library for 3D data processing[J]. arXiv preprint arXiv:1801.09847, 2018. [5] Choy C, Park J, Koltun V. Fully convolutional geometric features[C]//Proceedings of the IEEE/CVF International Conference on Computer Vision. 2019: 8958-8966.
|