源码:models.py
1. 读取配置文件
- PyTorch-YOLOv3\config\yolov3.cfg
def __init__(self, config_path, img_size=416):
super(Darknet, self).__init__()
self.module_defs = parse_model_config(config_path)
2. 创建模型
self.hyperparams, self.module_list = create_modules(self.module_defs)
2.1. 读取参数
def create_modules(module_defs):
"""
Constructs module list of layer blocks from module configuration in module_defs
"""
hyperparams = module_defs.pop(0)
output_filters = [int(hyperparams["channels"])]
2.2. 搭建网络结构
按顺序一个模块一个模块的搭建;
module_list = nn.ModuleList()
for module_i, module_def in enumerate(module_defs):
modules = nn.Sequential()
2.2.1. 卷积层
PyTorch-YOLOv3\config\yolov3.cfg 中,一个[convolutional] 是一个数据组合:卷积 + batch normalize + ReLU变形体 ;
[convolutional]
batch_normalize=1
filters=32
size=1
stride=1
pad=1
activation=leaky
- 读取
convolutional 数据; - 做卷积;
- 做
batch_normalize ; - 激活函数,
ReLU 稍微做了点变形体:nn.LeakyReLU(0.1) ; - 最后三合一数据组成
modules 加到一个module_list 中;
if module_def["type"] == "convolutional":
bn = int(module_def["batch_normalize"])
filters = int(module_def["filters"])
kernel_size = int(module_def["size"])
pad = (kernel_size - 1) // 2
modules.add_module(
f"conv_{module_i}",
nn.Conv2d( # 做 2D的卷积
in_channels=output_filters[-1],
out_channels=filters,
kernel_size=kernel_size,
stride=int(module_def["stride"]),
padding=pad,
bias=not bn,
),
)
# batch_normalize
if bn:
modules.add_module(f"batch_norm_{module_i}", nn.BatchNorm2d(filters, momentum=0.9, eps=1e-5))
# 激活函数, ReLU稍微做了点变形体:nn.LeakyReLU(0.1);
if module_def["activation"] == "leaky":
modules.add_module(f"leaky_{module_i}", nn.LeakyReLU(0.1))
..............
..............
# Register module list and number of output filters
module_list.append(modules)
2.2.2. maxpool
V3版本中去掉了这个层;
2.2.3. 上采样upsample
只是定义了一个空层,定义了需要做上采样这件事;
elif module_def["type"] == "upsample":
upsample = Upsample(scale_factor=int(module_def["stride"]), mode="nearest")
modules.add_module(f"upsample_{module_i}", upsample)
2.2.4. route
- 拼接层;
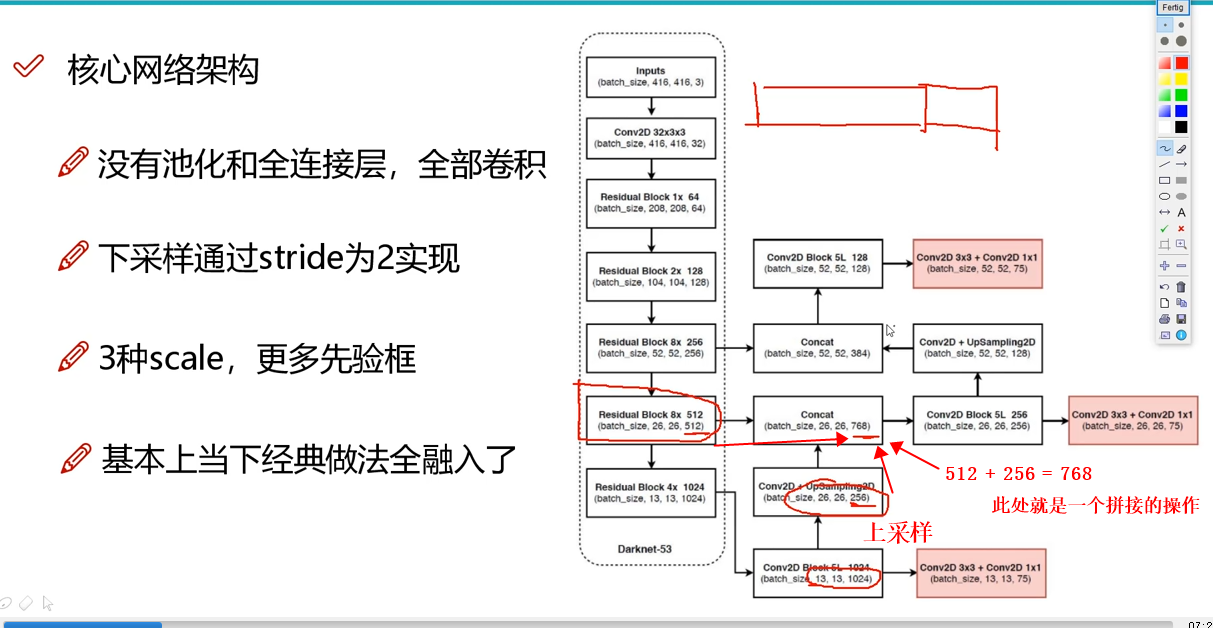 配置文件数据:
[route]
layers = -4
layers = -4 :跟前面第几层做拼接;
- 如:当前层
-1 跟前面第-4 层做拼接; 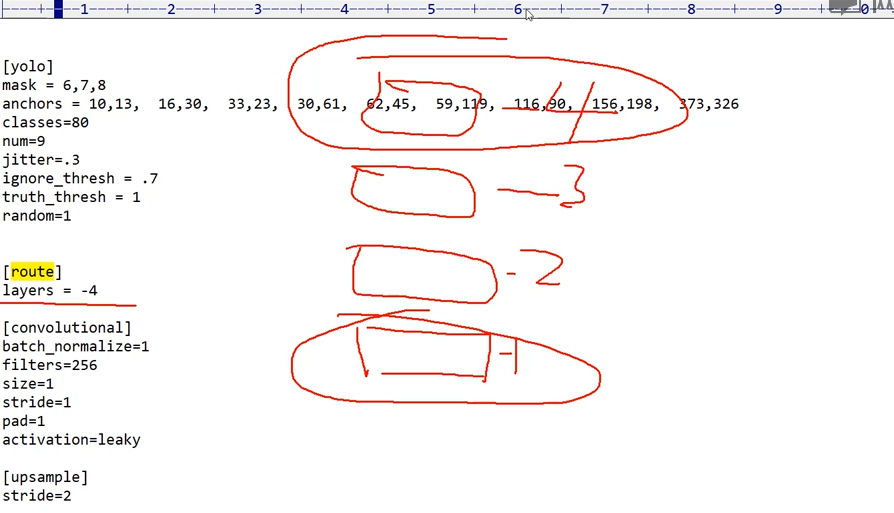 也只是定义了一个空层,定义了需要做这件事;
elif module_def["type"] == "route": # 输入1:26*26*256 输入2:26*26*128 输出:26*26*(256+128)
layers = [int(x) for x in module_def["layers"].split(",")]
filters = sum([output_filters[1:][i] for i in layers])
modules.add_module(f"route_{module_i}", EmptyLayer())
2.2.5. shortcut
- 残差链接层;
配置文件数据:
[shortcut]
from=-3
activation=linear
2.2.6. 核心:yolo层
如下图示,需要构建3个yolo层; 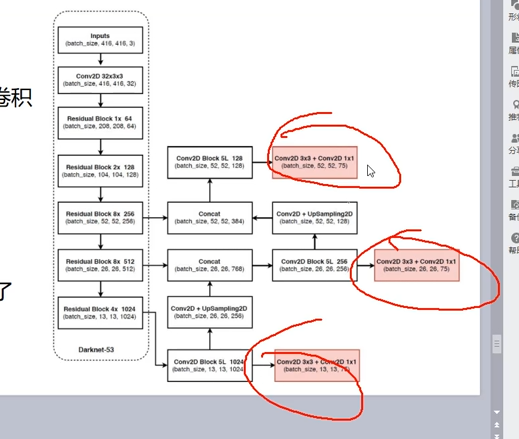
配置文件数据:
[yolo]
mask = 3,4,5
anchors = 10,13, 16,30, 33,23, 30,61, 62,45, 59,119, 116,90, 156,198, 373,326
classes=80
num=9
jitter=.3
ignore_thresh = .7
truth_thresh = 1
random=1
----
[yolo]
mask = 6,7,8
anchors = 10,13, 16,30, 33,23, 30,61, 62,45, 59,119, 116,90, 156,198, 373,326
classes=80
num=9
jitter=.3
ignore_thresh = .7
truth_thresh = 1
random=1
---
[yolo]
mask = 0,1,2
anchors = 10,13, 16,30, 33,23, 30,61, 62,45, 59,119, 116,90, 156,198, 373,326
classes=80
num=9
jitter=.3
ignore_thresh = .7
truth_thresh = 1
random=1
- 读取yolo层数据;
- 指定 先验框的id;
- 拿到3个先验框实际的大小;
- 获取类别,比如一共80个类别(猫、狗…)
- 构建 yolo层;
- 最后数据组成modules;
elif module_def["type"] == "yolo":
# 指定 先验框的id
anchor_idxs = [int(x) for x in module_def["mask"].split(",")]
# Extract anchors
# 拿到3个先验框实际的大小
anchors = [int(x) for x in module_def["anchors"].split(",")]
anchors = [(anchors[i], anchors[i + 1]) for i in range(0, len(anchors), 2)]
anchors = [anchors[i] for i in anchor_idxs]
# 类别,比如一共80个类别(猫、狗...)
num_classes = int(module_def["classes"])
img_size = int(hyperparams["height"])
# Define detection layer
# 构建 yolo层
yolo_layer = YOLOLayer(anchors, num_classes, img_size)
modules.add_module(f"yolo_{module_i}", yolo_layer)
# 构建 yolo层
yolo_layer = YOLOLayer(anchors, num_classes, img_size)
---
# 实现如下:
def __init__(self, anchors, num_classes, img_dim=416):
super(YOLOLayer, self).__init__()
self.anchors = anchors
# 先验框 大小
self.num_anchors = len(anchors)
# 先验框 数量
self.num_classes = num_classes
# 阈值
self.ignore_thres = 0.5
# 损失函数相关
self.mse_loss = nn.MSELoss()
self.bce_loss = nn.BCELoss()
self.obj_scale = 1
self.noobj_scale = 100
self.metrics = {}
self.img_dim = img_dim
self.grid_size = 0 # grid size
|