现在有的东西 数据集: 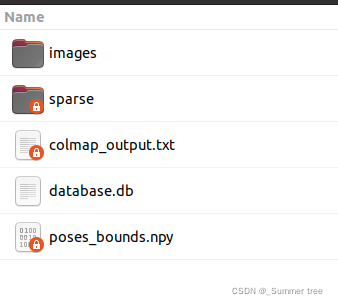 和yen给出测试数据集进行对比 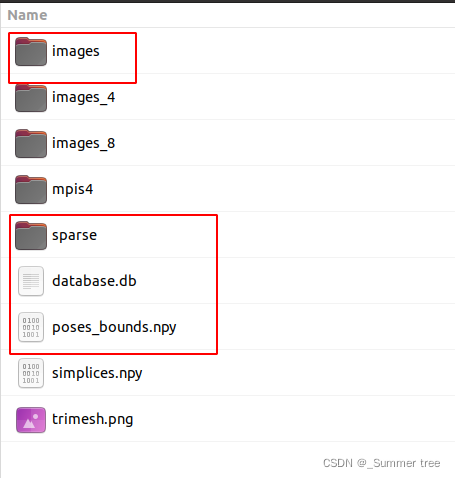 圈出来的文件是有的,不确定其他没有的文件影不影响运行 先试一下再说。
在yen上运行自己的数据集
yen 是这么说的 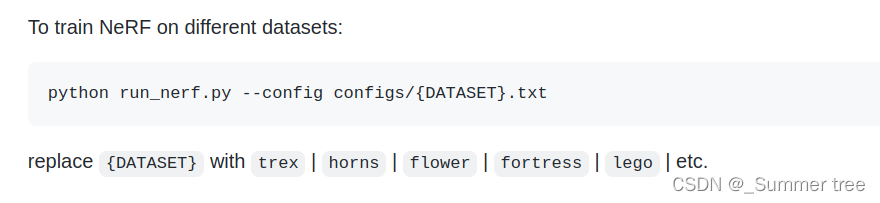 也就是说,yen为每个数据集都准备了对应的config文件。 fern的config文件内容如下:
expname = fern_test
basedir = ./logs
datadir = ./data/nerf_llff_data/fern
dataset_type = llff
factor = 8
llffhold = 8
N_rand = 1024
N_samples = 64
N_importance = 64
use_viewdirs = True
raw_noise_std = 1e0
我们依样画葫芦去创建 自己数据集的config文件
expname = desk1_test
basedir = ./logs
datadir = ./data/img_desk1
dataset_type = llff
factor = 8
llffhold = 8
N_rand = 1024
N_samples = 64
N_importance = 64
use_viewdirs = True
raw_noise_std = 1e0
我们尝试命令python run_nerf.py --config configs/desk1.txt 报错:subprocess.CalledProcessError: Command 'mogrify -resize 12.5% -format png *.jpg' returned non-zero exit status 127. 、 全部输出如下:
Minifying 8 ./data/img_desk1
mogrify -resize 12.5% -format png *.jpg
/bin/sh: 1: mogrify: not found
Traceback (most recent call last):
File "run_nerf.py", line 878, in <module>
train()
File "run_nerf.py", line 544, in train
spherify=args.spherify)
File "/home/joselyn/workspace/NeRF/nerf-pytorch-master/load_llff.py", line 246, in load_llff_data
poses, bds, imgs = _load_data(basedir, factor=factor)
File "/home/joselyn/workspace/NeRF/nerf-pytorch-master/load_llff.py", line 76, in _load_data
_minify(basedir, factors=[factor])
File "/home/joselyn/workspace/NeRF/nerf-pytorch-master/load_llff.py", line 51, in _minify
check_output(args, shell=True)
File "/home/joselyn/.conda/envs/nerfPytorch1/lib/python3.7/subprocess.py", line 411, in check_output
**kwargs).stdout
File "/home/joselyn/.conda/envs/nerfPytorch1/lib/python3.7/subprocess.py", line 512, in run
output=stdout, stderr=stderr)
subprocess.CalledProcessError: Command 'mogrify -resize 12.5% -format png *.jpg' returned non-zero exit status 127.
尝修改config 内容如下:
expname = desk1_test
basedir = ./logs
datadir = ./data/img_desk1
dataset_type = blenderhttps://blog.csdn.net/NGUever15/article/details/123815762?spm=1001.2014.3001.5502
no_batching = True
use_viewdirs = True
white_bkgd = True
lrate_decay = 500
N_samples = 64
N_importance = 128
N_rand = 1024
precrop_iters = 500
precrop_frac = 0.5
half_res = True
报错:
Traceback (most recent call last):
File "run_nerf.py", line 878, in <module>
train()
File "run_nerf.py", line 570, in train
images, poses, render_poses, hwf, i_split = load_blender_data(args.datadir, args.half_res, args.testskip)
File "/home/joselyn/workspace/NeRF/nerf-pytorch-master/load_blender.py", line 41, in load_blender_data
with open(os.path.join(basedir, 'transforms_{}.json'.format(s)), 'r') as fp:
FileNotFoundError: [Errno 2] No such file or directory: './data/img_desk1/transforms_train.json'
显然这几个json文件我确实没有。 也没有在项目给出的config文件中找到其他数据类型。
解决subprocess.CalledProcessError: Command 'mogrify -resize 12.5% -format png *.jpg' returned non-zero exit status 127.
查看了了多个LLFF类型的数据集的config,发现以下部分是他们共有的。
factor = 8
llffhold = 8
N_rand = 1024
N_samples = 64
N_importance = 64
use_viewdirs = True
raw_noise_std = 1
于是将我们的数据集 的config 修改为了 llff 的数据类型后 再次进行 运行尝试 python run_nerf.py --config configs/desk1.txt 。 离谱的是,这次并没有报错。 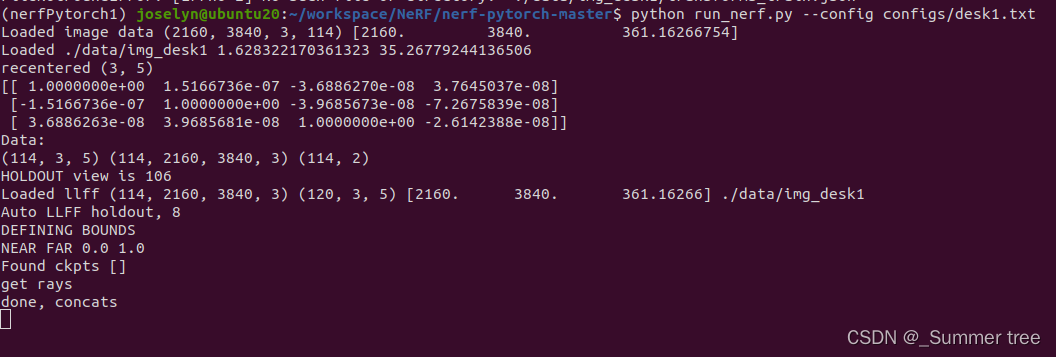
运行自己的数据集
现在程序还是运行中,我们趁这个时间,在看看源码中,关于数据集加载的部分。
噢,报错了~ shuffle rays 结束后,报错:RuntimeError: CUDA out of memory. Tried to allocate 10.57 GiB (GPU 0; 10.76 GiB total capacity; 4.56 MiB already allocated; 9.47 GiB free; 6.00 MiB reserved in total by PyTorch) If reserved memory is >> allocated memory try setting max_split_size_mb to avoid fragmentation. See documentation for Memory Management and PYTORCH_CUDA_ALLOC_CONF
在跑了一下fern数据集。前期输出如下: 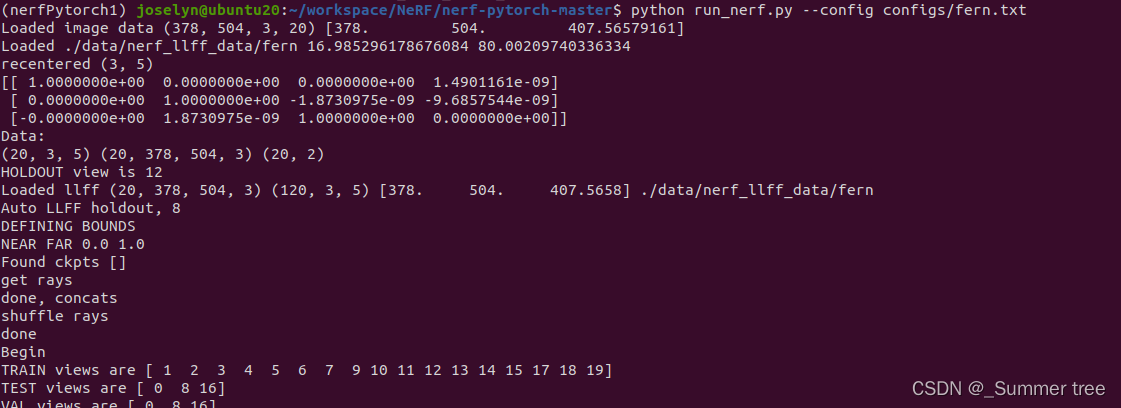 显然,我的数据集,图像数量比fern多(114 vs 20),图片分辨率大很多(2160 x 3840 vs 378 x 504)。 【思考】是否要降低数据集的分别率。
- 查看一下,数据集来源的 工作中,采用的什么分别率。 发现文章没有交代图像分别率。
- 查看fern数据的原始图像分辨率。 4032 x 3024, 那为什么训练的时候用的是 378 x 504 呢。 这里发现,我们的config中有个参数 factor,这个应该就是图像分辨率的放缩比例。
- 再看我们数据集desk1的原始分辨率。3840*2160,为什么我们的数据在训练过程的放缩操作会失效呢。
下面接着阅读源码找答案。
源码- run_nerf.py- Load data
源码中这部分功能的实现如下:
if args.dataset_type == 'llff':
images, poses, bds, render_poses, i_test = load_llff_data(args.datadir, args.factor,
recenter=True, bd_factor=.75,
spherify=args.spherify)
hwf = poses[0,:3,-1]
poses = poses[:,:3,:4]
print('Loaded llff', images.shape, render_poses.shape, hwf, args.datadir)
if not isinstance(i_test, list):
i_test = [i_test]
if args.llffhold > 0:
print('Auto LLFF holdout,', args.llffhold)
i_test = np.arange(images.shape[0])[::args.llffhold]
i_val = i_test
i_train = np.array([i for i in np.arange(int(images.shape[0])) if
(i not in i_test and i not in i_val)])
print('DEFINING BOUNDS')
if args.no_ndc:
near = np.ndarray.min(bds) * .9
far = np.ndarray.max(bds) * 1.
else:
near = 0.
far = 1.
print('NEAR FAR', near, far)
下面具体来看load_llff_data() 这个函数
这个函数是在load_llff.py 中实现的。函数内容如下:
def load_llff_data(basedir, factor=8, recenter=True, bd_factor=.75, spherify=False, path_zflat=False):
poses, bds, imgs = _load_data(basedir, factor=factor)
print('Loaded', basedir, bds.min(), bds.max())
return images, poses, bds, render_poses, i_test
函数_load_data() 如下:
def _load_data(basedir, factor=None, width=None, height=None, load_imgs=True):
poses_arr = np.load(os.path.join(basedir, 'poses_bounds.npy'))
poses = poses_arr[:, :-2].reshape([-1, 3, 5]).transpose([1,2,0])
bds = poses_arr[:, -2:].transpose([1,0])
img0 = [os.path.join(basedir, 'images', f) for f in sorted(os.listdir(os.path.join(basedir, 'images'))) \
if f.endswith('JPG') or f.endswith('jpg') or f.endswith('png')][0]
sh = imageio.imread(img0).shape
print('sh = ',sh)
sfx = ''
if factor is not None:
sfx = '_{}'.format(factor)
_minify(basedir, factors=[factor])
factor = factor
elif height is not None:
factor = sh[0] / float(height)
width = int(sh[1] / factor)
_minify(basedir, resolutions=[[height, width]])
sfx = '_{}x{}'.format(width, height)
elif width is not None:
factor = sh[1] / float(width)
height = int(sh[0] / factor)
_minify(basedir, resolutions=[[height, width]])
sfx = '_{}x{}'.format(width, height)
else:
factor = 1
print('factor = 1')
imgdir = os.path.join(basedir, 'images' + sfx)
if not os.path.exists(imgdir):
print( imgdir, 'does not exist, returning' )
return
imgfiles = [os.path.join(imgdir, f) for f in sorted(os.listdir(imgdir)) if f.endswith('JPG') or f.endswith('jpg') or f.endswith('png')]
if poses.shape[-1] != len(imgfiles):
print( 'Mismatch between imgs {} and poses {} !!!!'.format(len(imgfiles), poses.shape[-1]) )
return
sh = imageio.imread(imgfiles[0]).shape
poses[:2, 4, :] = np.array(sh[:2]).reshape([2, 1])
poses[2, 4, :] = poses[2, 4, :] * 1./factor
if not load_imgs:
return poses, bds
下面进一步看_minify() 函数
def _minify(basedir, factors=[], resolutions=[]):
needtoload = False
for r in factors:
imgdir = os.path.join(basedir, 'images_{}'.format(r))
if not os.path.exists(imgdir):
needtoload = True
for r in resolutions:
imgdir = os.path.join(basedir, 'images_{}x{}'.format(r[1], r[0]))
if not os.path.exists(imgdir):
needtoload = True
if not needtoload:
return
from shutil import copy
from subprocess import check_output
imgdir = os.path.join(basedir, 'images')
imgs = [os.path.join(imgdir, f) for f in sorted(os.listdir(imgdir))]
imgs = [f for f in imgs if any([f.endswith(ex) for ex in ['JPG', 'jpg', 'png', 'jpeg', 'PNG']])]
imgdir_orig = imgdir
wd = os.getcwd()
for r in factors + resolutions:
if isinstance(r, int):
name = 'images_{}'.format(r)
resizearg = '{}%'.format(100./r)
else:
name = 'images_{}x{}'.format(r[1], r[0])
resizearg = '{}x{}'.format(r[1], r[0])
imgdir = os.path.join(basedir, name)
if os.path.exists(imgdir):
continue
print('Minifying', r, basedir)
os.makedirs(imgdir)
check_output('cp {}/* {}'.format(imgdir_orig, imgdir), shell=True)
ext = imgs[0].split('.')[-1]
args = ' '.join(['mogrify', '-resize', resizearg, '-format', 'png', '*.{}'.format(ext)])
print(args)
os.chdir(imgdir)
check_output(args, shell=True)
os.chdir(wd)
if ext != 'png':
check_output('rm {}/*.{}'.format(imgdir, ext), shell=True)
print('Removed duplicates')
print('Done')
? 还是没有看出来他是在哪里 修改的图片的分辨率。
测试fern能够生成降分辨率的数据集。
先把fern目录下 images_8的数据集给移除掉。 运行python run_nerf.py --config configs/fern.txt 运行输出如下“:报错subprocess.CalledProcessError: Command 'mogrify -resize 12.5% -format png *.JPG' returned non-zero exit status 127.
sh = (3024, 4032, 3)
Minifying 8 ./data/nerf_llff_data/fern
mogrify -resize 12.5% -format png *.JPG
/bin/sh: 1: mogrify: not found
Traceback (most recent call last):
File "run_nerf.py", line 878, in <module>
train()
File "run_nerf.py", line 544, in train
spherify=args.spherify)
File "/home/joselyn/workspace/NeRF/nerf-pytorch-master/load_llff.py", line 258, in load_llff_data
poses, bds, imgs = _load_data(basedir, factor=factor)
File "/home/joselyn/workspace/NeRF/nerf-pytorch-master/load_llff.py", line 81, in _load_data
_minify(basedir, factors=[factor])
File "/home/joselyn/workspace/NeRF/nerf-pytorch-master/load_llff.py", line 53, in _minify
check_output(args, shell=True)
File "/home/joselyn/.conda/envs/nerfPytorch1/lib/python3.7/subprocess.py", line 411, in check_output
**kwargs).stdout
File "/home/joselyn/.conda/envs/nerfPytorch1/lib/python3.7/subprocess.py", line 512, in run
output=stdout, stderr=stderr)
subprocess.CalledProcessError: Command 'mogrify -resize 12.5% -format png *.JPG' returned non-zero exit status 127.
这个报错 ,和我们第一次运行python run_nerf.py --config configs/desk1.txt 的运行报错是一样的 原来check_output(args, shell=True) 这句话是执行resize 分辨率的关键,但是他出错了。
尝试在终端 该目录下,手动执行mogrify -resize 12.5% -format png *.JPG 提示需要安装一些包
sudo apt install imagemagick-6.q16
sudo apt install imagemagick-6.q16hdri
sudo apt install graphicsmagick-imagemagick-compat
安装完成后,在终端再次尝试运行mogrify -resize 12.5% -format png *.JPG ,这能够成功地生成对应的png对象,但是文件夹中还保留了原来 JPG图片。 下面尝试在程序中去执行这个操作。 【成功】因为代码里面有删除非png格式的文件
if ext != 'png':
check_output('rm {}/*.{}'.format(imgdir, ext), shell=True)
print('Removed duplicates')
print('Done')
再次尝试运行自己的数据
【ok】程序跑起来。 运行成功了。 但定性的来看,结果似乎非常不好。
|