- 从零开始
0、下载数据集到内存 1、初始化模型参数 2、定义softmax 3、定义模型 4、定义损失函数 5、计算分类精度,即正确的数量 6、训练
from tkinter.tix import Y_REGION
from turtle import update
from pyparsing import nums
import torch
from IPython import display
from d2l import torch as d2l
batch_size = 256
train_iter, test_iter = d2l.load_data_fashion_mnist(batch_size)
num_inputs = 784
num_outputs = 10
W = torch.normal(0, 0.01, size=(num_inputs, num_outputs), requires_grad=True)
b = torch.zeros(num_outputs, requires_grad=True)
def softmax(X):
X_exp = torch.exp(X)
partition = X_exp.sum(1, keepdim=True)
return X_exp / partition
X = torch.normal(0, 1, (2, 5))
X_prob = softmax(X)
print(X_prob, X_prob.sum(1))
def net(X):
return softmax(torch.matmul(X.reshape((-1, W.shape[0])), W) + b)
def cross_entropy(y_hat, y):
return -torch.log(y_hat[range(len(y_hat)), y])
y = torch.tensor([0, 2])
y_hat = torch.tensor([[0.1, 0.3, 0.6], [0.3, 0.3, 0.5]])
print(y_hat[[0, 1], y])
print('examples cross_entropy:',cross_entropy(y_hat, y) )
def accuracy(y_hat, y):
if len(y_hat.shape) > 1 and y_hat.shape[1] > 1:
y_hat = y_hat.argmax(axis=1)
cmp = y_hat.type(y.dtype) == y
return float(cmp.type(y.dtype).sum())
print('examples accuracy rate:', accuracy(y_hat, y) / len(y))
class Accumulator:
"""在n个变量上累加"""
def __init__(self, n):
self.data = [0.0] * n
def add(self, *args):
self.data = [a + float(b) for a, b in zip(self.data, args)]
def reset(self):
self.data = [0.0] * len(self.data)
def __getitem__(self, idx):
return self.data[idx]
def evaluate_accuracy(net, data_iter):
if isinstance(net, torch.nn.Module):
net.eval()
metric = Accumulator(2)
with torch.no_grad():
for X, y in data_iter:
metric.add(accuracy(net(X), y), y.numel())
return metric[0] / metric[1]
print('test accuracy rate:', evaluate_accuracy(net, test_iter))
def train_epoch_ch3(net, train_iter, loss, updater):
if isinstance(net, torch.nn.Module):
net.train()
metric = Accumulator(3)
for X, y in train_iter:
y_hat = net(X)
l = loss(y_hat, y)
if isinstance(updater, torch.optim.Optimizer):
updater.zero_grad()
l.mean().backward()
updater.step()
else:
l.sum().backward()
updater(X.shape[0])
metric.add(float(l.sum()), accuracy(y_hat, y), y.numel())
return metric[0] / metric[2], metric[1] / metric[2]
def train_ch3(net, train_iter, test_iter, loss, num_epochs, updater):
"""训练模型(定义见第3章)"""
d2l.plt.ion()
animator = d2l.Animator(xlabel='epoch', xlim=[1, num_epochs], ylim=[0.3, 0.9],
legend=['train loss', 'train acc', 'test acc'])
for epoch in range(num_epochs):
train_metrics = train_epoch_ch3(net, train_iter, loss, updater)
test_acc = evaluate_accuracy(net, test_iter)
animator.add(epoch + 1, train_metrics + (test_acc,))
d2l.plt.pause(0.2)
d2l.plt.ioff()
d2l.plt.show()
train_loss, train_acc = train_metrics
assert train_loss < 0.5, train_loss
assert train_acc <= 1 and train_acc > 0.7, train_acc
assert test_acc <= 1 and test_acc > 0.7, test_acc
lr = 0.1
def updater(batch_size):
return d2l.sgd([W, b], lr, batch_size)
num_epochs = 10
train_ch3(net, train_iter, test_iter, cross_entropy, num_epochs, updater)
def predict_ch3(net, test_iter, n=6):
"""预测标签(定义见第3章)"""
for X, y in test_iter:
break
trues = d2l.get_fashion_mnist_labels(y)
preds = d2l.get_fashion_mnist_labels(net(X).argmax(axis=1))
titles = [true +'\n' + pred for true, pred in zip(trues, preds)]
d2l.show_images(
X[0:n].reshape((n, 28, 28)), 1, n, titles=titles[0:n])
d2l.plt.show()
predict_ch3(net, test_iter)
运行结果: 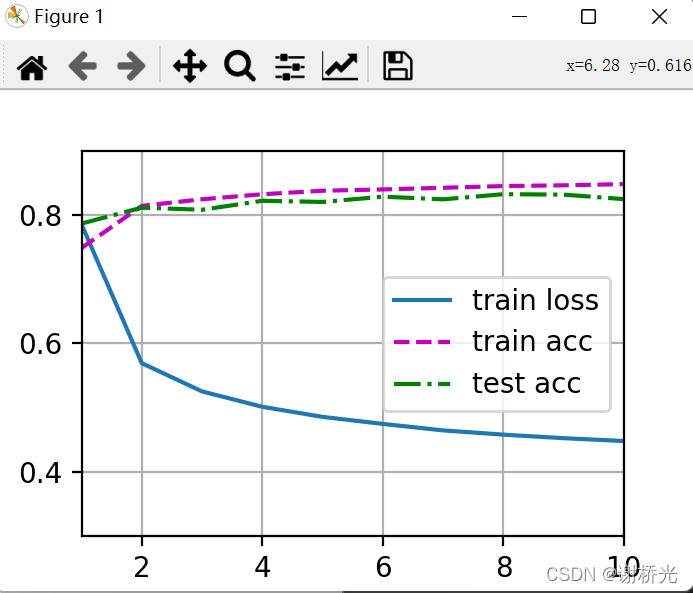 test acc差不多0.83的准确率  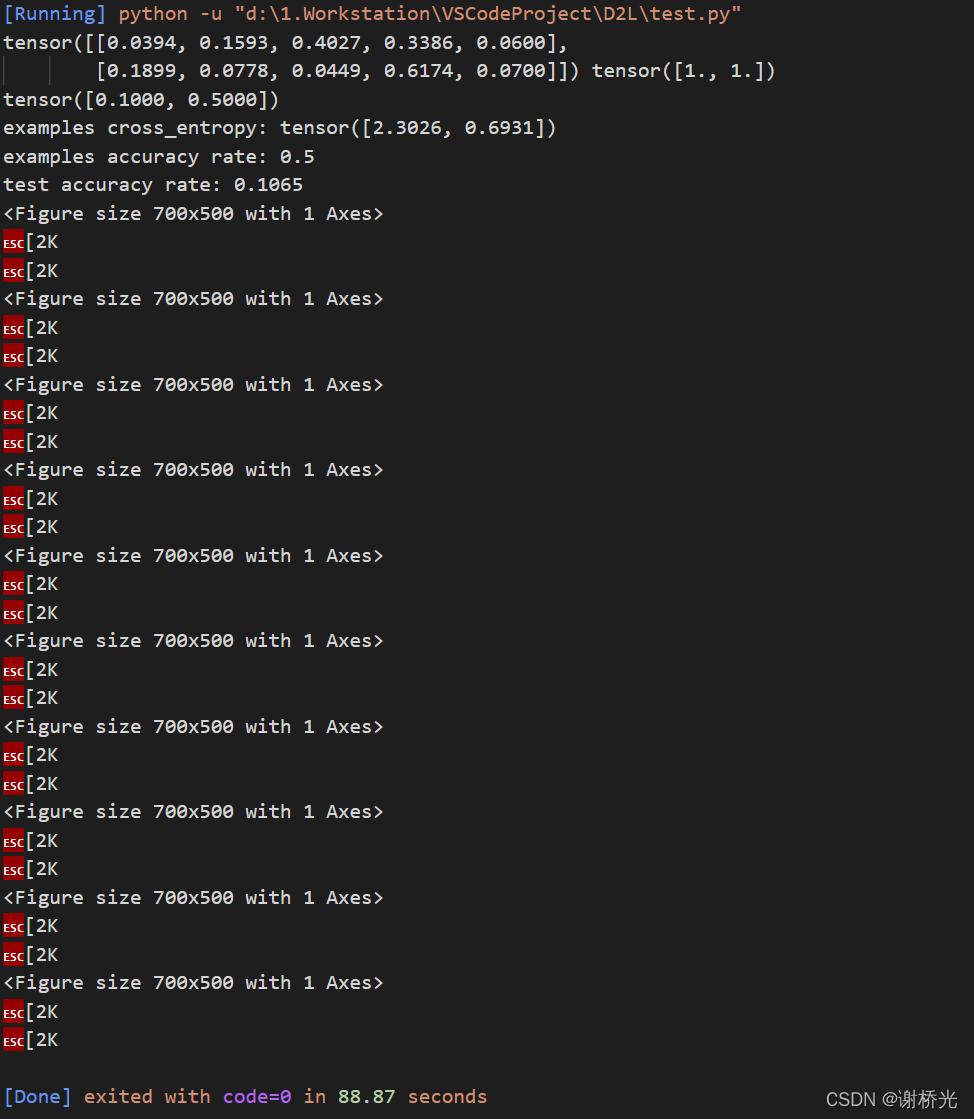 画图代码参考Python matplotlib.pyplot 绘制动态图
- 简洁实现
1、数据迭代器 2、定义网络模型 3、初始化参数 4、定义损失函数,中有softmax了 5、优化算法:更新参数的方法 6、训练
import torch
from torch import nn
from d2l import torch as d2l
batch_size = 256
train_iter, test_iter = d2l.load_data_fashion_mnist(batch_size)
net = nn.Sequential(nn.Flatten(), nn.Linear(784, 10))
def init_weights(m):
if type(m) == nn.Linear:
nn.init.normal_(m.weight, std=0.01)
net.apply(init_weights)
loss = nn.CrossEntropyLoss(reduction='none')
trainer = torch.optim.SGD(net.parameters(), lr = 0.1)
num_epochs = 10
d2l.train_ch3(net, train_iter, test_iter, loss, num_epochs, trainer)
运行结果: 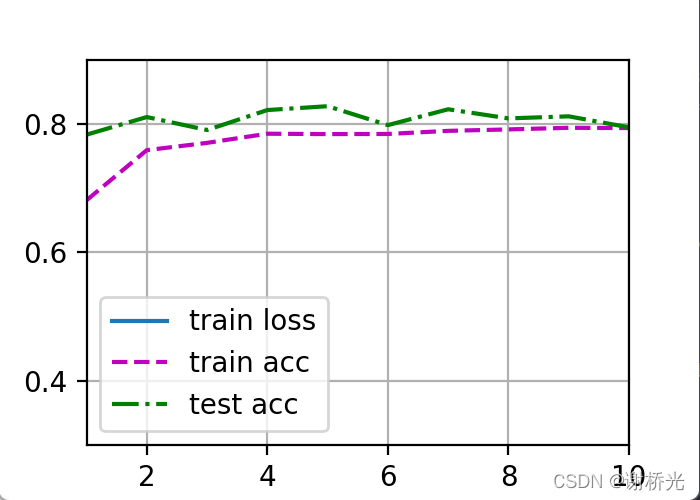 没有看到loss线,看了下评论区: 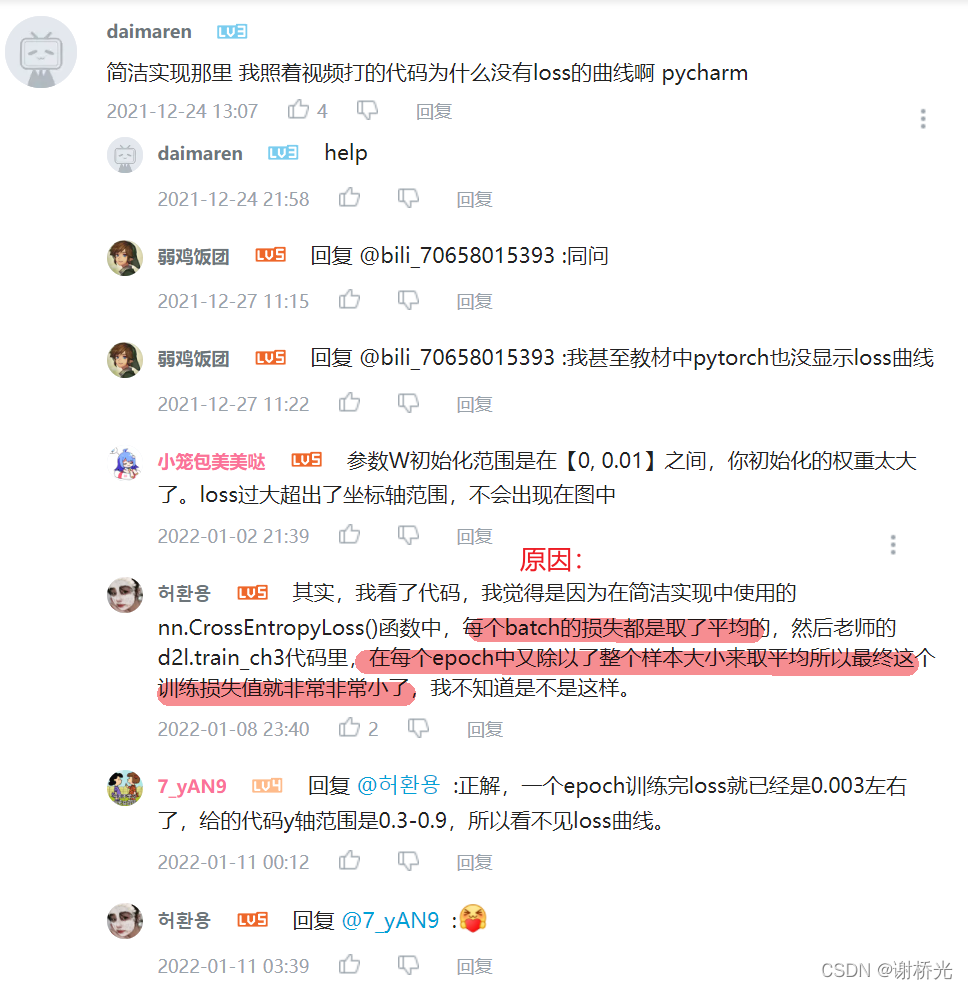 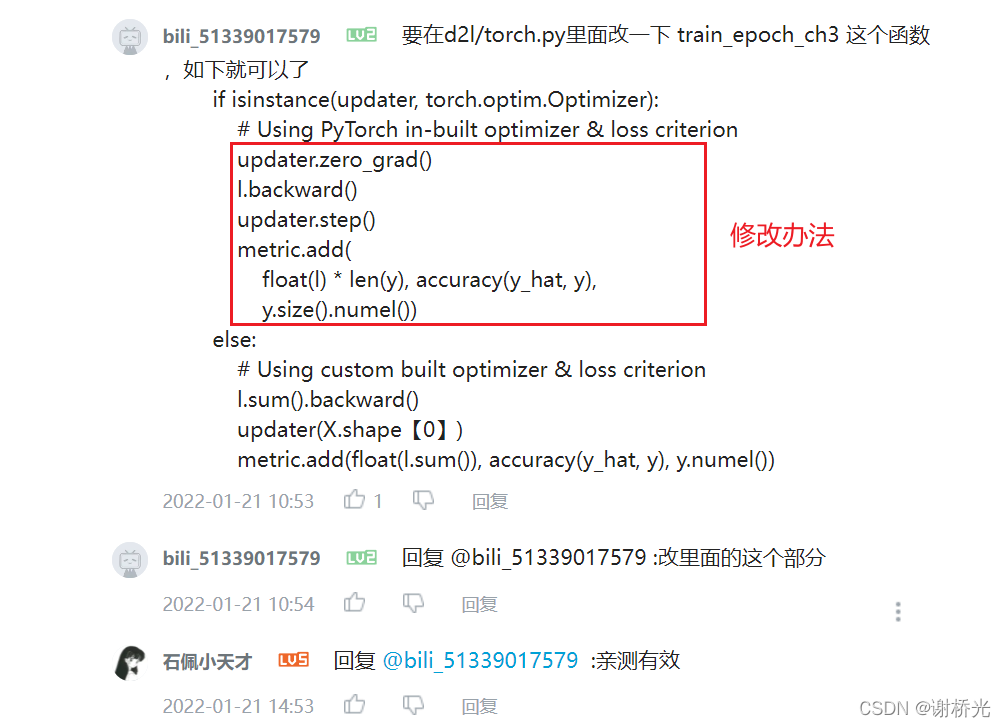 试了不行,然后将torch.py中train_epoch_ch3函数中的对应部分改成从零开始时写的部分。 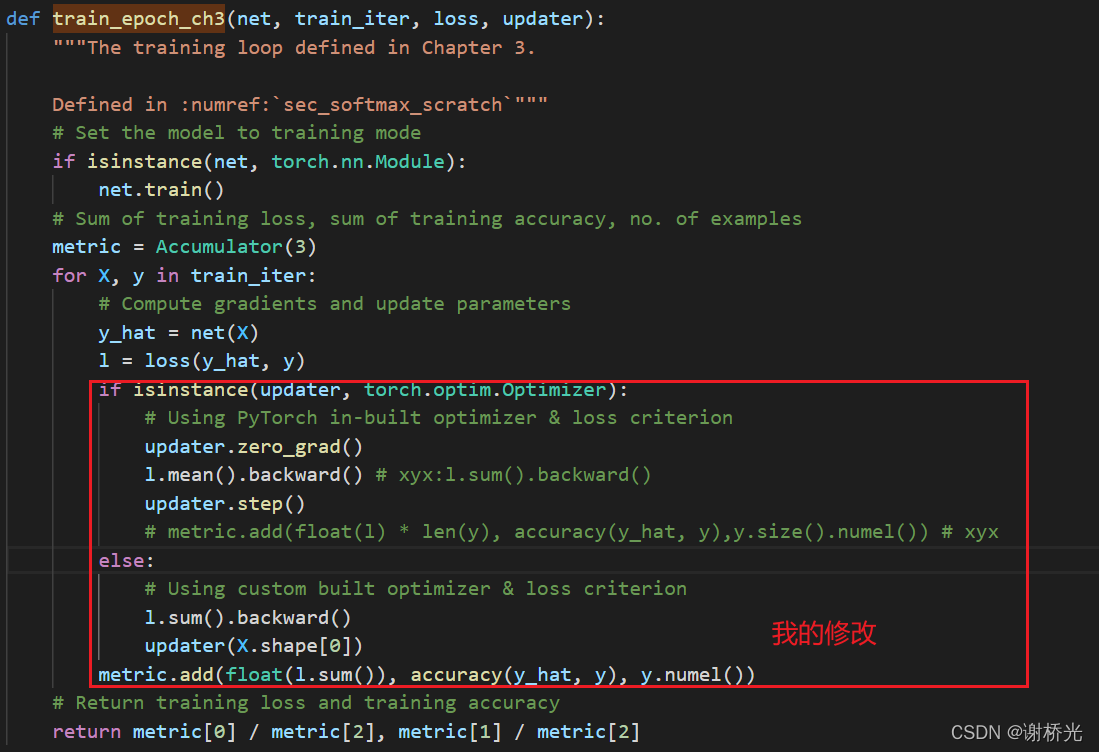 有了: 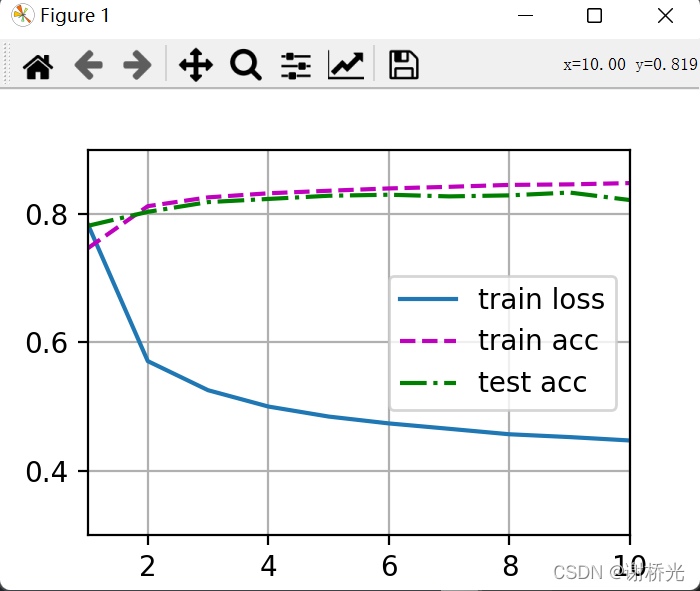
|