一、代码中的数据集可以点击以下链接进行下载
百度网盘提取码:lala
二、代码运行环境
Pytorch-gpu==1.7.1 Python==3.7
三、数据集处理的代码如下所示
import pandas as pd
import torch
def make_data():
data = pd.read_csv(r'dataset\dataset.csv')
X = data.Education.values.reshape(-1, 1)
Y = data.Income.values.reshape(-1, 1)
X = torch.from_numpy(X).type(torch.FloatTensor)
Y = torch.from_numpy(Y).type(torch.FloatTensor)
return X, Y
if __name__ == '__main__':
x, y = make_data()
print(x.shape)
print(x.type())
四、模型的构建代码如下所示
from torch import nn
class EIModel(nn.Module):
def __init__(self):
super(EIModel, self).__init__()
self.linear = nn.Linear(in_features=1, out_features=1)
def forward(self, inputs):
out = self.linear(inputs)
return out
if __name__ == '__main__':
model = EIModel()
print(model)
五、模型的训练代码如下所示
import torch
from data_loader import make_data
from model import EIModel
from torch import nn
from torch import optim
import tqdm
X, Y = make_data()
model = EIModel()
loss_fn = nn.MSELoss()
opt = optim.SGD(model.parameters(), lr=0.0001)
tqdm_range = tqdm.tqdm(range(5000), total=5000)
for epoch in tqdm_range:
for x, y in zip(X, Y):
y_pred = model(x)
loss = loss_fn(y_pred, y)
opt.zero_grad()
loss.backward()
opt.step()
torch.save(model.state_dict(), r'model_data\model.pth')
六、模型的预测代码如下所示
import torch
from model import EIModel
from data_loader import make_data
import matplotlib.pyplot as plt
X, Y = make_data()
model = EIModel()
model_state_dict = torch.load(r'model_data/model.pth')
model.load_state_dict(model_state_dict)
weight, bias = model.linear.weight, model.linear.bias
plt.scatter(X, Y)
plt.xlabel('Education')
plt.ylabel('Income')
plt.plot(X, model(X).detach().numpy(), c='red')
plt.savefig('result.jpg')
plt.show()
七、代码的运行结果如下所示
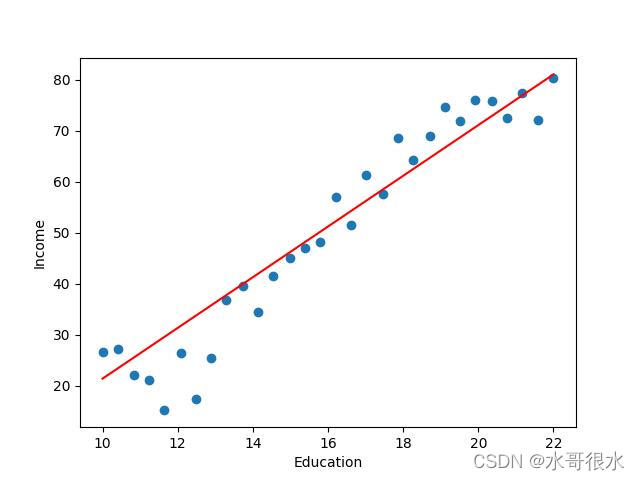
|