1. 图示思路
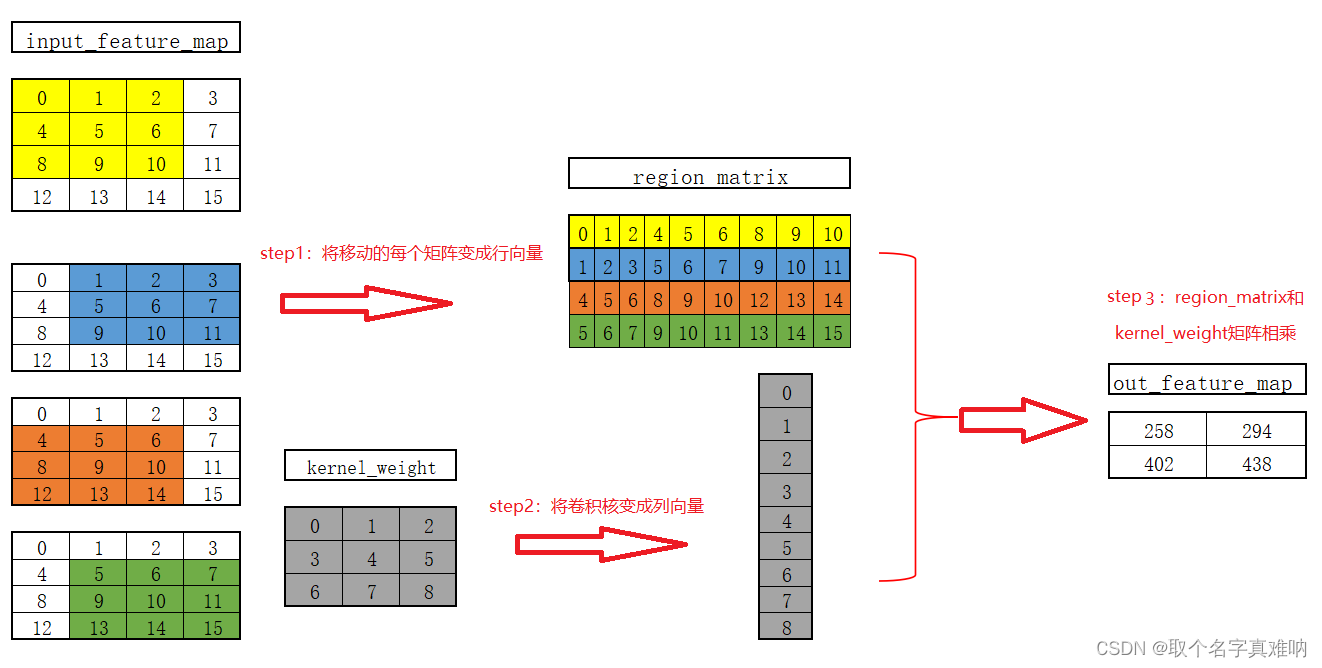
2. 代码
def matrix_multiplication_for_conv2d(input, kernel, stride=1, padding=0, bias=0):
import torch
from torch import nn
from torch.nn import functional as F
import math
input_feature_map = torch.arange(16, dtype=torch.float).reshape((1, 1, 4, 4))
in_channel = 1
out_channel = 1
kernel_size = 3
kernel_weight = torch.arange(9, dtype=torch.float).reshape(1, 1, 3, 3)
my_conv2d = nn.Conv2d(in_channels=in_channel, out_channels=out_channel, kernel_size=kernel_size, bias=False)
my_conv2d.weight = nn.Parameter(kernel_weight, requires_grad=True)
output_feature_map = my_conv2d(input_feature_map)
functional_output_feature_map = F.conv2d(input_feature_map, kernel_weight)
print(f"input_feature_map={input_feature_map}")
print(f"input_feature_map.shape={input_feature_map.shape}")
print(f"my_conv2d.weight={my_conv2d.weight}")
print(f"my_conv2d.weight.shape={my_conv2d.weight.shape}")
print(f"output_feature_map={output_feature_map}")
print(f"output_feature_map.shape={output_feature_map.shape}")
print(f"functional_output_feature_map={functional_output_feature_map}")
print(f"functional_output_feature_map.shape={functional_output_feature_map.shape}")
my_input_matrix = torch.arange(16, dtype=torch.float).reshape(4, 4)
my_kernel = torch.arange(9, dtype=torch.float).reshape(3, 3)
def matrix_multiplication_for_conv2d(input, kernel, stride=1, padding=0, bias=0):
if padding > 0:
input = F.pad(input, (padding, padding, padding, padding))
input_h, input_w = input.shape
kernel_h, kernel_w = kernel.shape
output_h = math.floor((input_h - kernel_h) / stride + 1)
output_w = math.floor((input_w - kernel_w) / stride + 1)
output_matrix = torch.zeros(output_h, output_w)
for i in range(0, input_h - kernel_h + 1, stride):
for j in range(0, input_w - kernel_w + 1, stride):
region = input[i:i + kernel_h, j: j + kernel_w]
output_matrix[int(i / stride), int(j / stride)] = torch.sum(region * kernel) + bias
return output_matrix
my_output = matrix_multiplication_for_conv2d(my_input_matrix, my_kernel)
print(f"my_output={my_output}")
print(f"判断自定义函数得到的结果是否跟pytorch计算结果一致\n{torch.isclose(my_output, torch.squeeze(functional_output_feature_map))}")
my_input_new = torch.randn(5, 5)
my_kernel_weight = torch.randn(3, 3)
my_kernel_bias = torch.randn(1)
my_ouput_new = torch.squeeze(F.conv2d(my_input_new.reshape(1, 1, 5, 5), weight=my_kernel_weight.reshape(1, 1, 3, 3),
bias=my_kernel_bias, stride=2, padding=2))
my_ouput_fun = matrix_multiplication_for_conv2d(my_input_new, my_kernel_weight, stride=2, padding=2,
bias=my_kernel_bias)
print(f"my_ouput_new={my_ouput_new}")
print(f"my_ouput_fun={my_ouput_fun}")
print(torch.isclose(my_ouput_new, my_ouput_fun))
def matrix_multiplication_for_flatten(input, kernel, stride=1, padding=0, bias=0):
if padding > 0:
input = F.pad(input, (padding, padding, padding, padding))
input_h, input_w = input.shape
kernel_h, kernel_w = kernel.shape
output_h = math.floor((input_h - kernel_h) / stride + 1)
output_w = math.floor((input_w - kernel_w) / stride + 1)
output_matrix = torch.zeros(output_h, output_w)
kernel_vector = kernel.reshape((kernel.numel(), 1))
region_matrix = torch.zeros(output_matrix.numel(), kernel.numel())
row_index = 0
for i in range(0, input_h - kernel_h + 1, stride):
for j in range(0, input_w - kernel_w + 1, stride):
region = input[i:i + kernel_h, j: j + kernel_w]
region_vector = torch.flatten(region)
region_matrix[row_index] = region_vector
row_index += 1
output_matrix = region_matrix @ kernel_vector
output_matrix = output_matrix.reshape((output_h,output_w))
return output_matrix
output_flatten = matrix_multiplication_for_flatten(my_input_matrix, my_kernel)
print(f"output_flatten={output_flatten}")
print(f"output_flatten.shape={output_flatten.shape}")
print(f"my_output={my_output}")
print(f"判断矩阵是否一致:\n"
f"output_flatten,my_output)\n{torch.isclose(output_flatten,my_output)}")
input_feature_map=tensor([[[[ 0., 1., 2., 3.],
[ 4., 5., 6., 7.],
[ 8., 9., 10., 11.],
[12., 13., 14., 15.]]]])
input_feature_map.shape=torch.Size([1, 1, 4, 4])
my_conv2d.weight=Parameter containing:
tensor([[[[0., 1., 2.],
[3., 4., 5.],
[6., 7., 8.]]]], requires_grad=True)
my_conv2d.weight.shape=torch.Size([1, 1, 3, 3])
output_feature_map=tensor([[[[258., 294.],
[402., 438.]]]], grad_fn=<ThnnConv2DBackward>)
output_feature_map.shape=torch.Size([1, 1, 2, 2])
functional_output_feature_map=tensor([[[[258., 294.],
[402., 438.]]]])
functional_output_feature_map.shape=torch.Size([1, 1, 2, 2])
my_output=tensor([[258., 294.],
[402., 438.]])
判断自定义函数得到的结果是否跟pytorch计算结果一致
tensor([[True, True],
[True, True]])
my_ouput_new=tensor([[ 3.2830e-01, 2.7485e+00, 1.9194e+00, 2.8474e+00],
[-1.1993e+00, -2.2748e+00, 7.3436e+00, -2.1672e+00],
[ 7.4937e-01, 6.2679e+00, 1.3324e+00, -4.9498e-03],
[ 1.6167e-01, 1.0183e+00, -6.7516e-01, 6.6955e-01]])
my_ouput_fun=tensor([[ 3.2830e-01, 2.7485e+00, 1.9194e+00, 2.8474e+00],
[-1.1993e+00, -2.2748e+00, 7.3436e+00, -2.1672e+00],
[ 7.4937e-01, 6.2679e+00, 1.3324e+00, -4.9498e-03],
[ 1.6167e-01, 1.0183e+00, -6.7516e-01, 6.6955e-01]])
tensor([[True, True, True, True],
[True, True, True, True],
[True, True, True, True],
[True, True, True, True]])
output_flatten=tensor([[258., 294.],
[402., 438.]])
output_flatten.shape=torch.Size([2, 2])
my_output=tensor([[258., 294.],
[402., 438.]])
判断矩阵是否一致:
output_flatten,my_output)
tensor([[True, True],
[True, True]])
3. torch.nn.unfold
通过滑动来实现卷积中的“卷” ;不用实现积 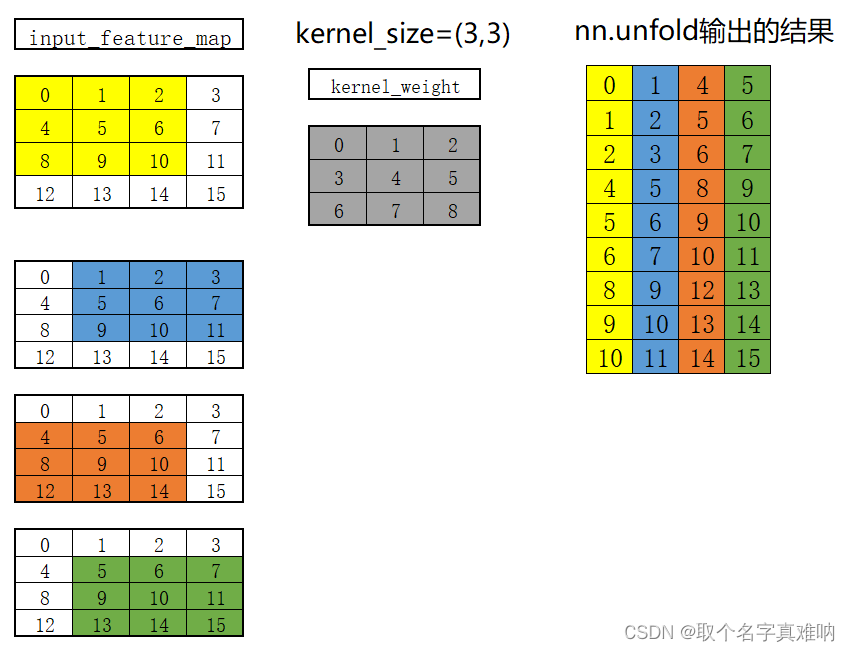
import torch
from torch import nn
from torch.nn import functional as F
import math
def matrix_multiplication_for_flatten(input, kernel, stride=1, padding=0, bias=0):
if padding > 0:
input = F.pad(input, (padding, padding, padding, padding))
input_h, input_w = input.shape
kernel_h, kernel_w = kernel.shape
output_h = math.floor((input_h - kernel_h) / stride + 1)
output_w = math.floor((input_w - kernel_w) / stride + 1)
output_matrix = torch.zeros(output_h, output_w)
kernel_vector = kernel.reshape((kernel.numel(), 1))
region_matrix = torch.zeros(output_matrix.numel(), kernel.numel())
row_index = 0
for i in range(0, input_h - kernel_h + 1, stride):
for j in range(0, input_w - kernel_w + 1, stride):
region = input[i:i + kernel_h, j: j + kernel_w]
region_vector = torch.flatten(region)
region_matrix[row_index] = region_vector
row_index += 1
return region_matrix.T
input1 = torch.arange(16,dtype=torch.float).reshape((4,4))
kernel1 = torch.arange(9,dtype=torch.float).reshape((3,3))
output1 = matrix_multiplication_for_flatten(input1,kernel1)
print(f"input1={input1}")
print(f"kernel1={kernel1}")
print(f"output1={output1}")
my_unfold = nn.Unfold(kernel_size=(3,3))
my_input = input1.unsqueeze(0).unsqueeze(0)
my_ouput_unfold = my_unfold(my_input).squeeze()
print(f"my_input={my_input.shape}")
print(f"my_ouput_unfold={my_ouput_unfold}")
print(f"my_ouput_unfold.shape={my_ouput_unfold.shape}")
print(f"my_input={my_input}")
print(f"my_input.shape={my_input.shape}")
print(f"kernel_size=(3,3)")
func_unfold = F.unfold(my_input,kernel_size=(3,3))
print(f"func_unfold={func_unfold}")
print(f"func_unfold.shape={func_unfold.shape}")
input1=tensor(
[[ 0., 1., 2., 3.],
[ 4., 5., 6., 7.],
[ 8., 9., 10., 11.],
[12., 13., 14., 15.]])
kernel1=tensor(
[[0., 1., 2.],
[3., 4., 5.],
[6., 7., 8.]])
output1=tensor(
[[ 0., 1., 4., 5.],
[ 1., 2., 5., 6.],
[ 2., 3., 6., 7.],
[ 4., 5., 8., 9.],
[ 5., 6., 9., 10.],
[ 6., 7., 10., 11.],
[ 8., 9., 12., 13.],
[ 9., 10., 13., 14.],
[10., 11., 14., 15.]])
my_input=torch.Size([1, 1, 4, 4])
my_ouput_unfold=tensor(
[[ 0., 1., 4., 5.],
[ 1., 2., 5., 6.],
[ 2., 3., 6., 7.],
[ 4., 5., 8., 9.],
[ 5., 6., 9., 10.],
[ 6., 7., 10., 11.],
[ 8., 9., 12., 13.],
[ 9., 10., 13., 14.],
[10., 11., 14., 15.]])
my_ouput_unfold.shape=torch.Size([9, 4])
my_input=tensor(
[[[[ 0., 1., 2., 3.],
[ 4., 5., 6., 7.],
[ 8., 9., 10., 11.],
[12., 13., 14., 15.]]]])
my_input.shape=torch.Size([1, 1, 4, 4])
kernel_size=(3,3)
func_unfold=tensor(
[[[ 0., 1., 4., 5.],
[ 1., 2., 5., 6.],
[ 2., 3., 6., 7.],
[ 4., 5., 8., 9.],
[ 5., 6., 9., 10.],
[ 6., 7., 10., 11.],
[ 8., 9., 12., 13.],
[ 9., 10., 13., 14.],
[10., 11., 14., 15.]]])
func_unfold.shape=torch.Size([1, 9, 4])
|