主要是对博主之前的博客做一些归纳整理和补充。
一. 数据集方面
1. 关于深度学习数据集的介绍可以参见此博客
Tensorflow知识点总结(一)_竹叶青lvye的博客-CSDN博客
2.mnist数据集获取的三种方式见博客
Tensorflow知识点总结(二)_竹叶青lvye的博客-CSDN博客
?3.对于一些深度学习框架,已经包含了常用的数据集,如下博客的最后有相关代码获取
Ubuntu配置TensorRT及验证_竹叶青lvye的博客-CSDN博客
?获取示例代码如下:
import torch
import torchvision
train_data = torchvision.datasets.CIFAR10("./data",train=True,transform=torchvision.transforms.ToTensor,download=True)
print("load ok")
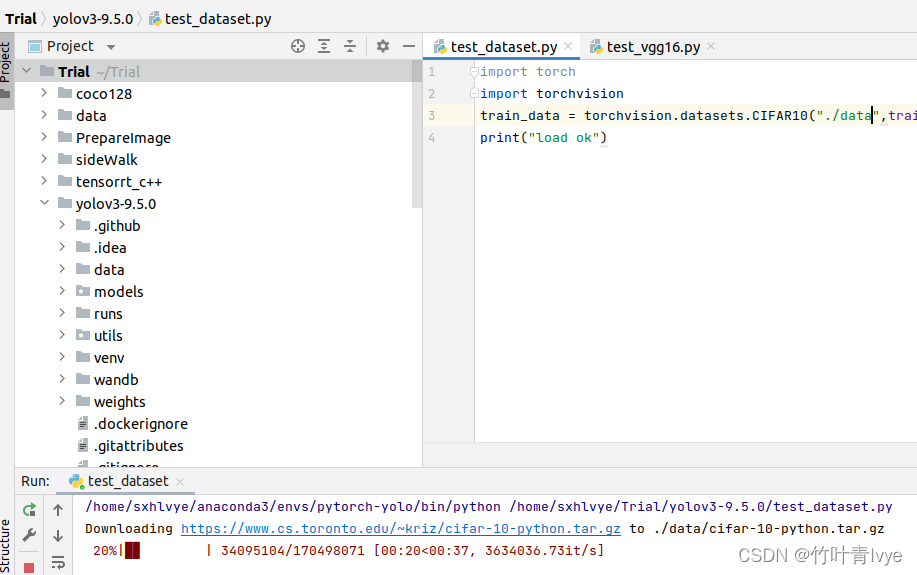
?
?官网上有对pytorch的自带数据集进行介绍
?Datasets — Torchvision 0.12 documentation
官网上对keras自带数据集的介绍
常用数据集 Datasets - Keras 中文文档第
github上一些例子常用的数据集
第1个:
mirrors / shekkizh / FCN.tensorflow · GitCode用的是ADEChallengeData2016数据集
第2个:
GitHub - lsh1994/keras-segmentation: Get started with Semantic Segmentation based on Keras, including FCN32/FCN8/SegNet/U-Net
?用的是CamVid 数据集
第3个:
mirrors / divamgupta / image-segmentation-keras · GitCode数据集很丰富
第4个:
mirrors / balancap / ssd-tensorflow · GitCode 用的是Pascal VOC datasets (2007 and 2012)
第5个:
第Releases · ultralytics/yolov3 · GitHubCOCO数据集
博主此前已经有相关博客介绍了此资源
ultralytics/yolov3训练预测自己数据集的配置过程_竹叶青lvye的博客-CSDN博客_ultralytics yolov3
最近发现其已经更新到yolov5了,后期会重点研究,并部署到树莓派上
二.模型方面
第一个:
FCN.py · master · mirrors / shekkizh / FCN.tensorflow · GitCode用的是imagenet-vgg-verydeep-19.mat作为预训练模型,其前置网络模型是vgg19网络结构
?之前的博客中用其训练和预测了自己的数据集
第二个
GitHub - lsh1994/keras-segmentation: Get started with Semantic Segmentation based on Keras, including FCN32/FCN8/SegNet/U-Net
里的segnet用的是vgg16_weights_tf_dim_ordering_tf_kernels_notop.h5预训练模型参数
#加载vgg16的预训练权重
Vgg_streamlined.load_weights("premodel/vgg16_weights_tf_dim_ordering_tf_kernels_notop.h5")
之前的博客中用其训练和预测了自己的数据集
第三个
文件 · master · mirrors / divamgupta / image-segmentation-keras · GitCode
也用到了vgg16_weights_tf_dim_ordering_tf_kernels_notop.h5预训练模型
之前的博客中用其训练和预测了自己的数据集
第四个:
mirrors / balancap / ssd-tensorflow · GitCode 预训练模型是vgg_16.ckpt
之前的博客中用其训练和预测了自己的数据集
第五个:
Releases · ultralytics/yolov3 · GitHub 用的是自己提前训练好的yolov3.pt(在COCO数据集上)
之前的博客中用其训练和预测了自己的数据集,代码中用到了如下语句
model.load_state_dict(state_dict, strict=False) # load
?可以知道其预训练模式保存的只是模型的参数
Pytorch踩坑记:赋值、浅拷贝、深拷贝三者的区别以及model.state_dict()和model.load_state_dict()的坑点_白马金羁侠少年的博客-CSDN博客_model.state_dict()
PyTorch:存储和恢复模型并查看参数,load_state_dict(),state_。。。 - 百度文库
Pytorch:模型的保存与加载 torch.save()、torch.load()、torch.nn.Module.load_state_dict()_宁静致远*的博客-CSDN博客_load_state_dict
三.速度性能比较
第一个实验:
ultralytics/yolov3训练预测自己数据集的配置过程_竹叶青lvye的博客-CSDN博客_ultralytics yolov3
拿上面博客中的网络做实验,比较有预训练模型和没有之间的性能差异(在同样的参数设定,迭代步数下)
有预训练模型:

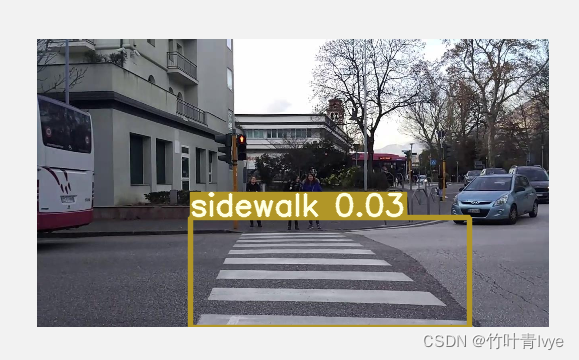
?
无预训练模型
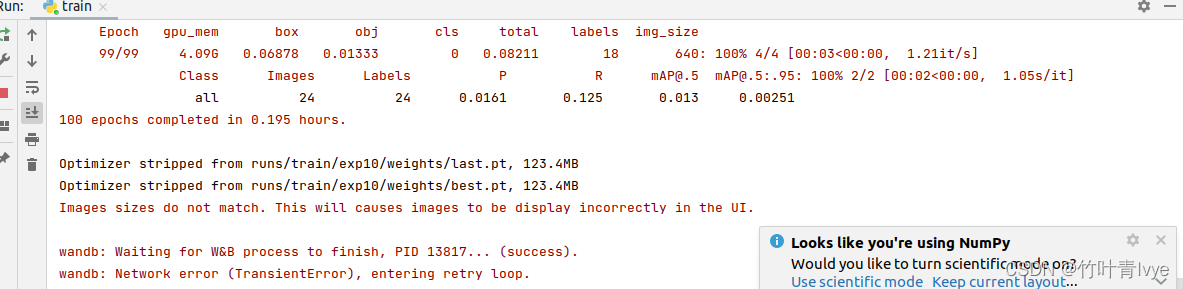
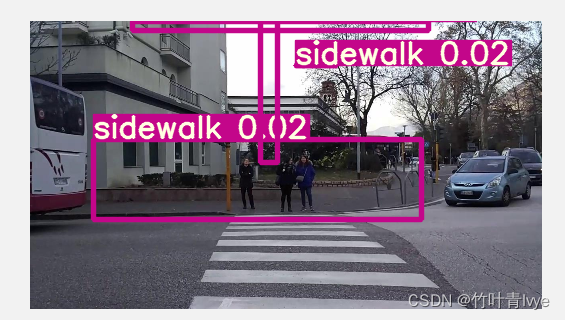
?
?对比可以知道加载预训练模型后,能够大大缩短模型到一个理想检测状态的训练时间。
第二个实验:
语义分割之SegNet训练预测自己的数据集_竹叶青lvye的博客-CSDN博客_segnet训练自己的数据集
?拿上面博客中的网络做实验,比较有预训练模型和没有之间的性能差异(在同样的参数设定,迭代步数下)
有预训练模型:
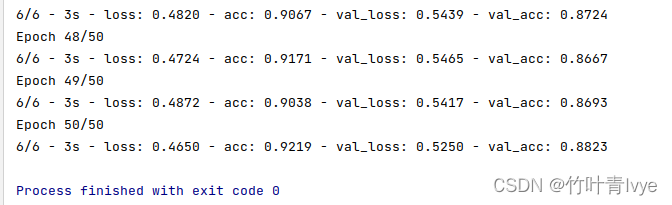
?
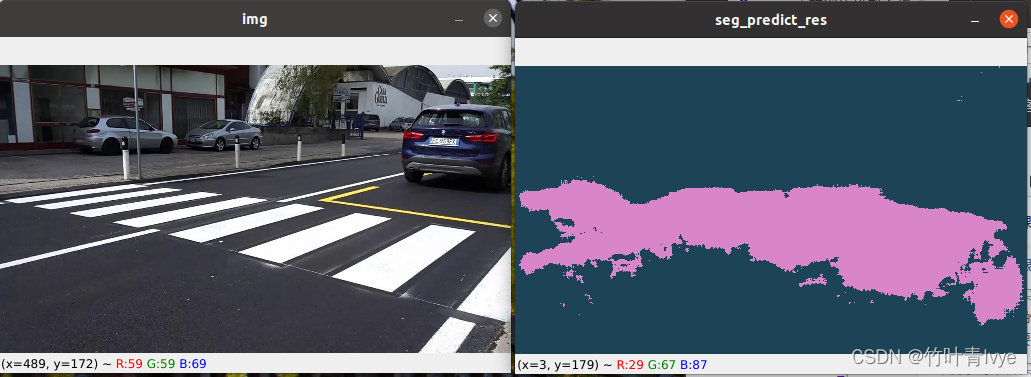
?
无预训练模型 ?
?
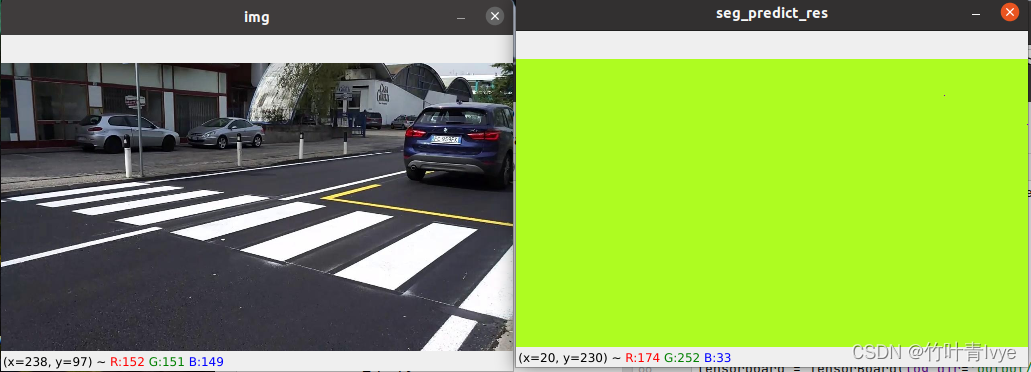
?可以看到预测结果不如有模型的,这里连检测结果都没有
第三个实验:
实验博客最后实现的MLP
无预训练模型
?
有预训练模型
修改代码如下:
model.load_weights("MLP.h5")
model_checkpoint = ModelCheckpoint("MLP.h5", monitor='val_loss', save_best_only=True, save_weights_only=True)
model.fit(X_train, Y_train, batch_size=200, epochs=2, shuffle=True, verbose=1, validation_split=0.3,callbacks=[model_checkpoint])
?
?可以看到有预训练效果模型是要更好的
第四个实验:
可以看到博客中的一些代码
ultralytics/yolov3训练预测自己数据集的配置过程_竹叶青lvye的博客-CSDN博客_ultralytics yolov3
如下是从detect.py中截取的一些代码,可以看到其中有cpu和gpu的一些控制语句(pytorch),有模型的,有数据的
parser.add_argument('--device', default='', help='cuda device, i.e. 0 or 0,1,2,3 or cpu')
device = select_device(opt.device)
half = device.type != 'cpu' # half precision only supported on CUDA
half = False
if classify:
modelc = load_classifier(name='resnet101', n=2) # initialize
modelc.load_state_dict(torch.load('weights/resnet101.pt', map_location=device)['model']).to(device).eval()
for path, img, im0s, vid_cap in dataset:
img = torch.from_numpy(img).to(device)
def select_device(device='', batch_size=None):
# device = 'cpu' or '0' or '0,1,2,3'
s = f'YOLOv3 🚀 {git_describe() or date_modified()} torch {torch.__version__} ' # string
cpu = device.lower() == 'cpu'
if cpu:
os.environ['CUDA_VISIBLE_DEVICES'] = '-1' # force torch.cuda.is_available() = False
elif device: # non-cpu device requested
os.environ['CUDA_VISIBLE_DEVICES'] = device # set environment variable
assert torch.cuda.is_available(), f'CUDA unavailable, invalid device {device} requested' # check availability
cuda = not cpu and torch.cuda.is_available()
if cuda:
n = torch.cuda.device_count()
if n > 1 and batch_size: # check that batch_size is compatible with device_count
assert batch_size % n == 0, f'batch-size {batch_size} not multiple of GPU count {n}'
space = ' ' * len(s)
for i, d in enumerate(device.split(',') if device else range(n)):
p = torch.cuda.get_device_properties(i)
s += f"{'' if i == 0 else space}CUDA:{d} ({p.name}, {p.total_memory / 1024 ** 2}MB)\n" # bytes to MB
else:
s += 'CPU\n'
logger.info(s.encode().decode('ascii', 'ignore') if platform.system() == 'Windows' else s) # emoji-safe
return torch.device('cuda:0' if cuda else 'cpu')
python常用功能代码_竹叶青lvye的博客-CSDN博客 博客里可以看到pytorch和numpy的互转
TensorRT加速方法介绍(python pytorch模型)_竹叶青lvye的博客-CSDN博客_tensorrt加速pytorch
?博客里也有pytorch和numpy的互转示例
https://blog.csdn.net/jiugeshao/article/details/123318715?spm=1001.2014.3001.5502博客中可以看到pytorch自带模型预测一张图片的过程,其中也有cpu和gpu的控制。
注意:
在pytorch中,即使是有GPU的机器,它也不会自动使用GPU,而是需要在程序中显示指定。调用model.cuda(),可以将模型加载到GPU上去。这种方法不被提倡,而建议使用model.to(device)的方式,这样可以显示指定需要使用的计算资源,特别是有多个GPU的情况下。
博主之前在玩keras的时候,会加一些全局变量,指定gpu,类似如下:
os.environ['TF_CPP_MIN_LOG_LEVEL']='0'
os.environ["CUDA_VISIBLE_DEVICES"] = executor_id
print("CUDA_VISIBLE_DEVICES: ",executor_id)
sys.stdout.flush()
from tensorflow.python.client import device_lib
print(device_lib.list_local_devices())
?pytorch也是类似,也有,类似如下博客
记录下用pytorch踩过的GPU坑 - 知乎
pytorch中 如何将gpu与gpu、gpu与cpu 在load时相互转化载入_qq_41895190的博客-CSDN博客_pytorch 将gpu转到cpu
pytorch 中tensor在CPU和GPU之间转换 - cltt - 博客园
浅谈将Pytorch模型从CPU转换成GPU - 知乎
第五个实验
使用Pytorch自带模型预测图片_竹叶青lvye的博客-CSDN博客_pytorch自带模型
?选择cpu时,ct如下:
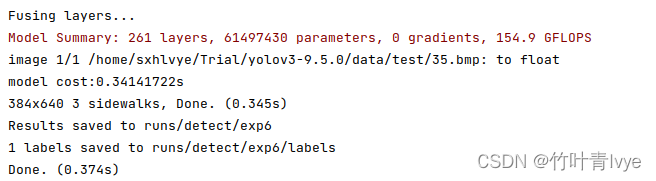
?在gpu上时,ct如下:
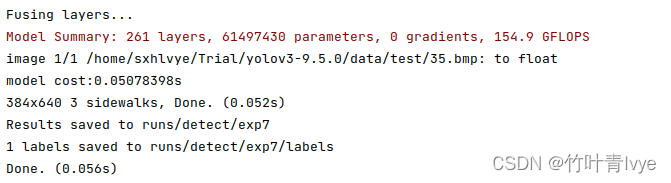
?即使指定了跑在gpu,但是加了语句
os.environ["CUDA_VISIBLE_DEVICES"] = '-1'
ct如下:
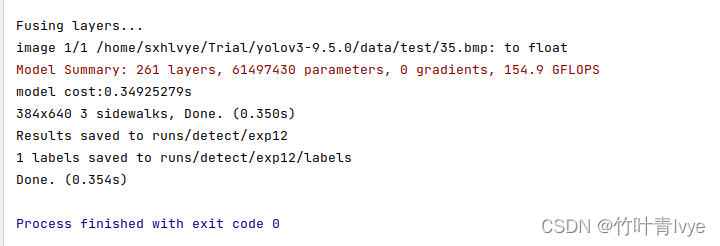
?可知这句话能够强制跑在cpu上
第六个实验
使用Pytorch自带模型预测图片_竹叶青lvye的博客-CSDN博客_pytorch自带模型
?拿上面博客中例子做实验,如下是第一种方式指定跑在gpu上
import torch
import torchvision
from PIL import Image
from torchvision import transforms
import torchvision.models as models
import matplotlib.pyplot as plt
import time
print(torch.cuda.is_available())
print(torch.cuda.device_count())
print(torch.cuda.get_device_name(0))
print(torch.cuda.current_device())
print(torch.cuda.get_device_properties(0))
vgg16 = torchvision.models.vgg16(pretrained=True).cuda()
normalize = transforms.Normalize(mean=[0.485, 0.456, 0.406], std=[0.229, 0.224, 0.225])
transform = transforms.Compose([
transforms.Resize(256),
transforms.CenterCrop(224),
transforms.ToTensor(), normalize]
)
img = Image.open("2008_002682.jpg")
print(img.size)
# 对图像进行归一化
img_p = transform(img)
print(img_p.shape)
# 增加一个维度
img_normalize = torch.unsqueeze(img_p, 0).cuda()
print(img_normalize.shape)
vgg16.eval()
t_model = time.perf_counter()
out = vgg16(img_normalize)
print(f'model cost:{time.perf_counter() - t_model:.8f}s')
# 最后一层是1000的一维向量,每一个表示对应类别的概率
print(out.shape)
with open('imagenet_classes.txt') as f:
classes = [line.strip() for line in f.readlines()]
_, indices = torch.sort(out, descending=True)
percentage = torch.nn.functional.softmax(out, dim=1)[0] * 100
prediction = [[classes[idx], percentage[idx].item()] for idx in indices[0][:5]]
print(prediction)
score = []
label = []
for i in prediction:
print('Prediciton-> {:<25} Accuracy-> ({:.2f}%)'.format(i[0][:], i[1]))
score.append(i[1])
label.append(i[0])
print(score)
?运行结果如下:
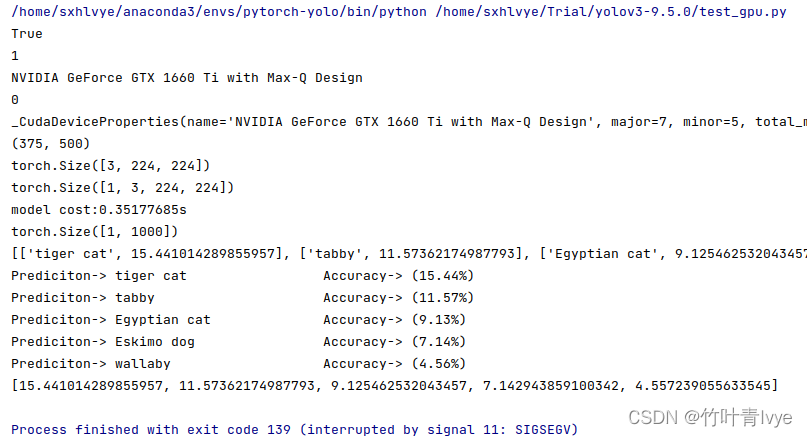
如下是第二种方式指定跑在gpu上
import torch
import torchvision
from PIL import Image
from torchvision import transforms
import torchvision.models as models
import matplotlib.pyplot as plt
import time
import os
print(torch.cuda.is_available())
print(torch.cuda.device_count())
print(torch.cuda.get_device_name(0))
print(torch.cuda.current_device())
print(torch.cuda.get_device_properties(0))
device = torch.device("cuda:0" if torch.cuda.is_available() else "cpu")
#os.environ["CUDA_VISIBLE_DEVICES"] = str(0)
vgg16 = torchvision.models.vgg16(pretrained=True).to(device)
normalize = transforms.Normalize(mean=[0.485, 0.456, 0.406], std=[0.229, 0.224, 0.225])
transform = transforms.Compose([
transforms.Resize(256),
transforms.CenterCrop(224),
transforms.ToTensor(), normalize]
)
img = Image.open("2008_002682.jpg")
print(img.size)
# 对图像进行归一化
img_p = transform(img)
print(img_p.shape)
# 增加一个维度
img_normalize = torch.unsqueeze(img_p, 0).to(device)
print(img_normalize.shape)
vgg16.eval()
t_model = time.perf_counter()
out = vgg16(img_normalize)
print(f'model cost:{time.perf_counter() - t_model:.8f}s')
# 最后一层是1000的一维向量,每一个表示对应类别的概率
print(out.shape)
with open('imagenet_classes.txt') as f:
classes = [line.strip() for line in f.readlines()]
_, indices = torch.sort(out, descending=True)
percentage = torch.nn.functional.softmax(out, dim=1)[0] * 100
prediction = [[classes[idx], percentage[idx].item()] for idx in indices[0][:5]]
print(prediction)
score = []
label = []
for i in prediction:
print('Prediciton-> {:<25} Accuracy-> ({:.2f}%)'.format(i[0][:], i[1]))
score.append(i[1])
label.append(i[0])
print(score)
运行结果如下:
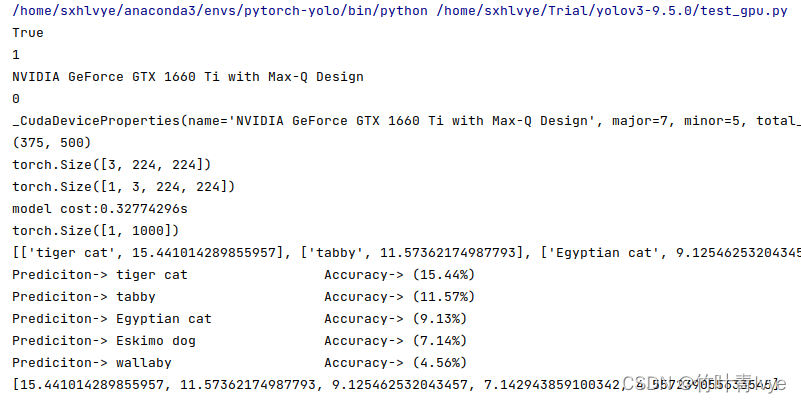
速度还不够快的话,可以继续设置tensorrt来加速
TensorRT加速方法介绍(python pytorch模型)_竹叶青lvye的博客-CSDN博客_tensorrt加速pytorch
参考此博客,加速后,时间为0.00034539s
?后续也可以用此模型跑在树莓派上、树莓派(插了movidius 2神经棒)、英伟达jetson TX2上
四.各框架自带的模型
TensorFlow模型,需要额外安装
GitHub - tensorflow/models: Models and examples built with TensorFlow
Keras模型
应用 Applications - Keras 中文文档
?
?keras 预训练模型的使用方法_苍蓝儿的博客-CSDN博客_keras预训练模型
博主之前博客也有用过keras的预训练模型过
keras离线下载模型的存储位置 - 简书
PyTorch使用预训练模型 - 知乎
如下可以看到相关源码
https://github.com/pytorch/vision/blob/main/torchvision/models/detection/ssd.py
可以看到官网对其的介绍,很详细
?主页 - PyTorch中文文档
?Models and pre-trained weights — Torchvision 0.12 documentation
博主之前有博客使用了预训练模型
对比复现34个预训练模型,PyTorch和Keras你选谁? - 知乎
五.python版本的管控
树莓派4B上多版本python切换(一)_竹叶青lvye的博客-CSDN博客_树莓派python多版本
?树莓派4B上多版本python切换(二)_竹叶青lvye的博客-CSDN博客_树莓派安装python2
?上面两篇博客值得参考。
|