该指标在2016年的leCun的一篇文章中有详细的解释,https://arxiv.org/abs/1511.05440
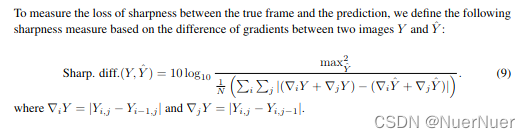
更多的指标:https://blog.csdn.net/hacker_long/article/details/104509523
具体的实现方式:
import numpy as np
import os
import cv2
import tensorflow as tf
from tensorflow.keras import layers
import tensorflow.compat.v1 as tf1
tf1.disable_v2_behavior()
def log10(t):
"""
Calculates the base-10 log of each element in t.
@param t: The tensor from which to calculate the base-10 log.
@return: A tensor with the base-10 log of each element in t.
"""
numerator = tf1.log(t)
denominator = tf1.log(tf.constant(10, dtype=numerator.dtype))
return numerator / denominator
def sharp_diff_error(gen_frames, gt_frames):
"""
Computes the Sharpness Difference error between the generated images and the ground truth
images.
@param gen_frames: A tensor of shape [batch_size, height, width, 3]. The frames generated by the
generator model.
@param gt_frames: A tensor of shape [batch_size, height, width, 3]. The ground-truth frames for
each frame in gen_frames.
@return: A scalar tensor. The Sharpness Difference error over each frame in the batch.
"""
gen_frames = tf1.to_float(gen_frames) / 255
gt_frames = tf1.to_float(gt_frames) / 255
shape = tf.shape(gen_frames)
num_pixels = tf1.to_float(shape[1] * shape[2] * shape[3])
# gradient difference
# create filters [-1, 1] and [[1],[-1]] for diffing to the left and down respectively.
# TODO: Could this be simplified with one filter [[-1, 2], [0, -1]]?
pos = tf.constant(np.identity(3), dtype=tf.float32)
neg = -1 * pos
filter_x = tf.expand_dims(tf.stack([neg, pos]), 0) # #[1, 2, 3, 3]
filter_y = tf.stack([tf.expand_dims(pos, 0), tf.expand_dims(neg, 0)]) # #[2,1,3,3]
strides = [1, 1, 1, 1] # stride of (1, 1)
padding = 'SAME'
gen_dx = tf.abs(tf.nn.conv2d(gen_frames, filter_x, strides, padding=padding))
gen_dy = tf.abs(tf.nn.conv2d(gen_frames, filter_y, strides, padding=padding))
gt_dx = tf.abs(tf.nn.conv2d(gt_frames, filter_x, strides, padding=padding))
gt_dy = tf.abs(tf.nn.conv2d(gt_frames, filter_y, strides, padding=padding))
gen_grad_sum = gen_dx + gen_dy
gt_grad_sum = gt_dx + gt_dy
grad_diff = tf.abs(gt_grad_sum - gen_grad_sum)
batch_errors = 10 * log10(1 / ((1 / num_pixels) * tf.reduce_sum(grad_diff, [1, 2, 3])))
return tf.reduce_mean(batch_errors)
if __name__ == "__main__":
ori_path = 'xxx.jpg'
recon_path = 'xxx.png'
batch_ori_img = []
batch_recon_img = []
ori_img = cv2.resize(cv2.imread(ori_path), (616, 112))
recon_img = cv2.resize(cv2.imread(recon_path), (616, 112))
print(ori_img.shape)
batch_ori_img.append(ori_img)
batch_recon_img.append(recon_img)
batch_ori_img_n = np.array(batch_ori_img)
batch_recon_img_n = np.array(batch_recon_img)
print(batch_recon_img_n.shape)
sd = sharp_diff_error(batch_recon_img_n, batch_ori_img_n)
sess = tf1.Session()
sd_ = sess.run(sd)
print(sd_)
值得注意的是:这里比较巧妙的通过设计卷积核的参数,通过使用卷积来实现图像列于列间,行与行间的作差,其中filter_x的尺寸为[1,2,3,3], filter_y的尺寸为[2,1,3,3],分别对应filter的height,width, in_channel和out_channel。详细原理大家可以参考tf.nn.conv2d的用法。
PS:PSNR和SSIM的计算pandas都提供了成熟的方法可直接调用
|