一、数据预处理
????????a、步态轮廓图
????????????????先使用Opencv对采集到的视频进行处理,得到一帧帧图像,而后通过背景减除法(
????????????????cv2.bgsegm.createBackgroundSubtractorMOG()
????????????????)从一组图像中得到图中人的轮廓图:
? ?
? ?
核心代码:
def from_video_get_img(video_path):
print(video_path)
print(video_path + ' Is Loading...')
cap = cv2.VideoCapture(video_path)
if cap.isOpened():
# best>MOG
fgbg = cv2.bgsegm.createBackgroundSubtractorMOG()
origrial_path_list = []
id = 0
flag = True
while flag:
ret, frame = cap.read()
if ret:
fgmask = fgbg.apply(frame)
save_file_name = video_path.split('/')[-1].split('.')[0]
save_origrial_path = 'Package/Origrial/' + save_file_name + '/'
save_normalPic_path = 'Package/Normal/' + save_file_name + '/'
if not os.path.exists(save_origrial_path):
os.makedirs(save_origrial_path)
if not os.path.exists(save_normalPic_path):
os.makedirs(save_normalPic_path)
a, b = fgmask.shape
origrial_path_list.append(fgmask)
#cv2.imwrite(save_origrial_path+format_id(id)+'.png', fgmask)
#cv2.imwrite(save_normalPic_path+format_id(id)+'.png', frame)
id += 1
cv2.imshow('frame', fgmask)
cv2.waitKey(1)
else:
break
cap.release()
cv2.destroyAllWindows()
????????b、头部轮廓图
? ? ? ????????? 在得到人体轮廓后,我们通过分析数据集可以发现每一个人的头部轮廓差距非常明显!下面几张图片可以说明:
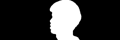 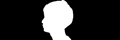 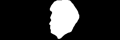
????????????????实现方式:
? ? ? ? ? ? ? ? ????????1、从人体轮廓图中把人给裁剪出来,效果图如下:?
?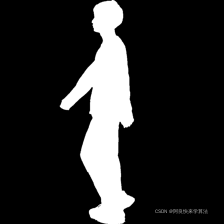 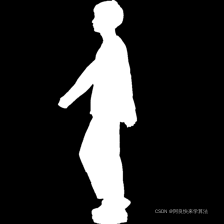 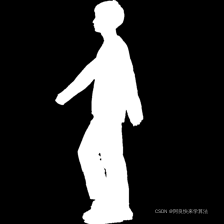
? ? ? ? ? ? ? ????????? 2、从裁剪的图片中二次裁剪出人的头部轮廓
? ?核心代码:
def get_cImg(path, cut_path, size):
'''
剪切图片
:param path: 输入图片路径
:param cut_path: 剪切图片后的输出路径
:param size: 要剪切的图片大小
:return:
'''
dirs_list = os.listdir(path)
for dir in dirs_list:
files_list = os.listdir(os.path.join(path, dir))
firstId, lastId = 10000000000000000, 0
for pic in files_list:
firstId = min(int(pic.split('.')[0]), firstId)
lastId = max(int(pic.split('.')[0]), lastId)
midId = (firstId+lastId)>>1
for id in range(midId-30, midId+30):
img_name = str(id) + '.png'
img = Image.open(os.path.join(path, dir, img_name))
image, flag = cut(img)
goal_dir = os.path.join(cut_path, dir)
create_file(goal_dir)
if not flag:
Image.fromarray(image).convert('L').resize((size, size)).save(os.path.join(goal_dir, img_name))
print(dir + ' is Cuted!')
def cut(image):
'''
通过找到人的最小最大高度与宽度把人的轮廓分割出来,、
因为原始轮廓图为二值图,因此头顶为将二值图像列相加后,形成一列后第一个像素值不为0的索引。
同理脚底为形成一列后最后一个像素值不为0的索引。
人的宽度也同理。
:param image: 需要裁剪的图片 N*M的矩阵
:return: temp: 裁剪后的图片 size*size的矩阵。flag:是否是符合要求的图片
'''
image = np.array(image)
# 找到人的最小最大高度与宽度
height_min = (image.sum(axis=1) != 0).argmax()
height_max = ((image.sum(axis=1) != 0).cumsum()).argmax()
width_min = (image.sum(axis=0) != 0).argmax()
width_max = ((image.sum(axis=0) != 0).cumsum()).argmax()
head_top = image[height_min, :].argmax()
# 设置切割后图片的大小,为size*size,因为人的高一般都会大于宽
size = height_max - height_min
temp = np.zeros((size, size))
# 将width_max-width_min(宽)乘height_max-height_min(高,szie)的人的轮廓图,放在size*size的图片中央
# l = (width_max-width_min)//2
# r = width_max-width_min-l
# 以头为中心,将width_max-width_min(宽)乘height_max-height_min(高,size)的人的轮廓图,放在size*size的图片中央
l1 = head_top-width_min
r1 = width_max-head_top
# 若宽大于高,或头的左侧或右侧身子比要生成图片的一般要大。则此图片为不符合要求的图片
flag = False
if size <= width_max-width_min or size//2 < r1 or size//2 < l1:
flag = True
return temp, flag
# centroid = np.array([(width_max+width_min)/2,(height_max+height_min)/2],dtype='int')
temp[:, (size//2-l1):(size//2+r1)] = image[height_min:height_max, width_min:width_max]
return temp, flag
def get_humanHead(root_path):
save_img_path = 'DetectDataSetsget\Head'
create_file(save_img_path)
for dir_name in os.listdir(root_path):
src_dir = os.path.join(root_path, dir_name)
#print(src_dir)
dst_dir = src_dir.replace(root_path, save_img_path)
#print(dst_dir)
create_file(dst_dir)
for img_name in os.listdir(src_dir):
img = cv.imread(os.path.join(src_dir, img_name))
x, y, w, h = 50, 0, 120, 40
croped_img = img[y: y+h, x: x+w]
cv.imwrite(os.path.join(dst_dir, img_name), croped_img)
cv.waitKey(0)
? ? ? ? c、骨架图
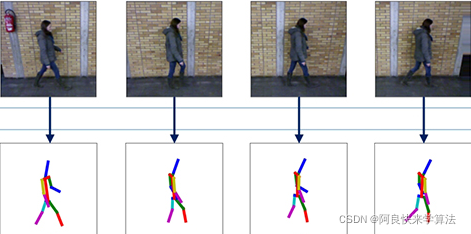
二、提取步态特征
? ? ? ? 提取的特征分为角度特征、下肢特征和头部特征;
? ? ? ? ?a、角度特征
? ? ? ? ? ? ? ? 实现概述:使用姿态估计算法得到人体各个关节点的坐标,并通过这些坐标信息从一系列图像中提取到角度的变化特征。
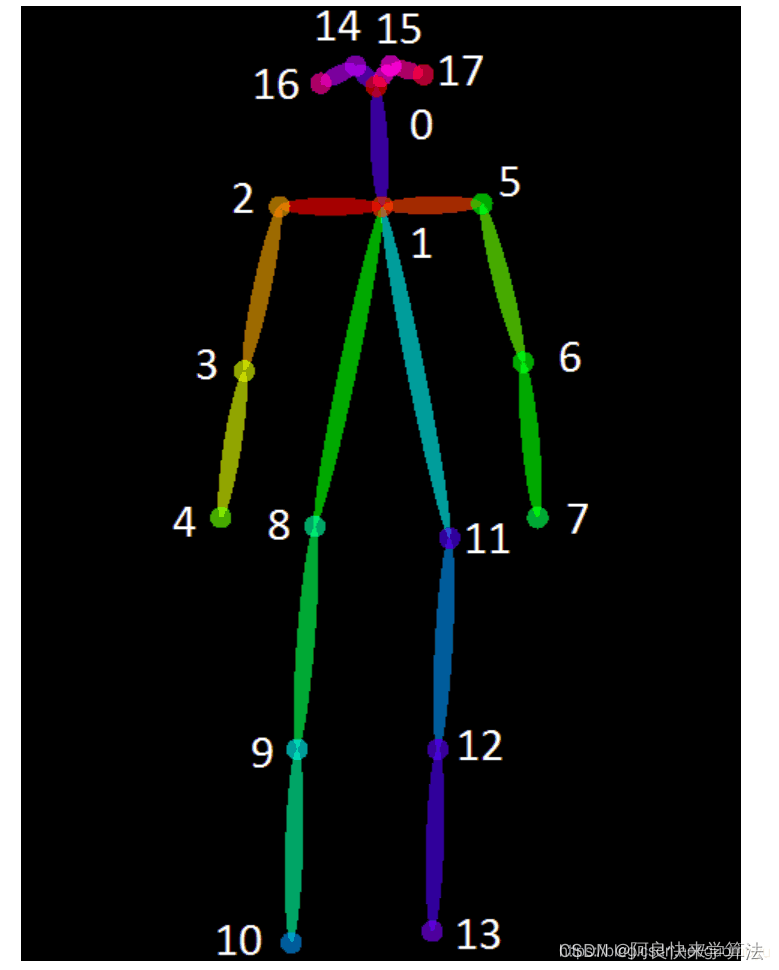
? ? ? ? ?b、下肢特征
? ? ? ? ? ? ? ? 实现概述:使用图像处理算法对一系列步态轮廓图处理得到人体双腿和双手的摆动周期,上下起伏的波动,和左右增缩的波动。
? ? ? ? ?c、头部特征
? ? ? ? ? ? ? ? 实现概述:使用mobilent轻量级卷积神经网络做图像识别,把预测目标的头部特征数据放入模型中训练,最后通过模型预测的分数作为参考做身份识别。
三、特征匹配
? ? ? ? a.特征模型(头部轮廓)
? ? ? ? ? ? ? ? 使用之前训练好的图像识别网络进行对未知人物的识别,得到他与数据库中已经录入的人物信息的相似程度,选取相似程度最大的人物,提取出他的标签。
? ? ? ? b.特征数据(下肢特征,角度特征)
? ? ? ? ? ? ? ? 利用特征模型中提取出来的标签,在特征数据库中依照标签进行哈希匹配,将时间复杂度从O(n)下降到O(1)。
四、搭建GUI可视化界面
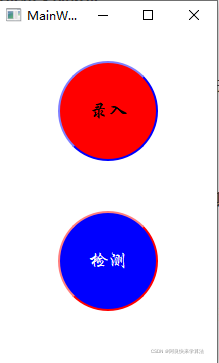
? ? ? ? ? 功能一、录入数据
????????????????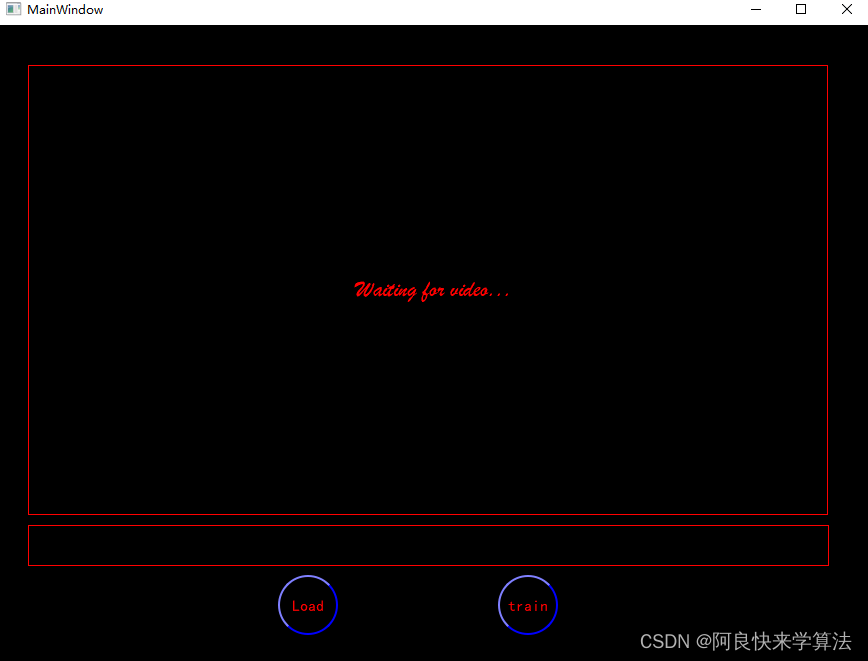
?? ? ? ? ? 功能二、匹配检测
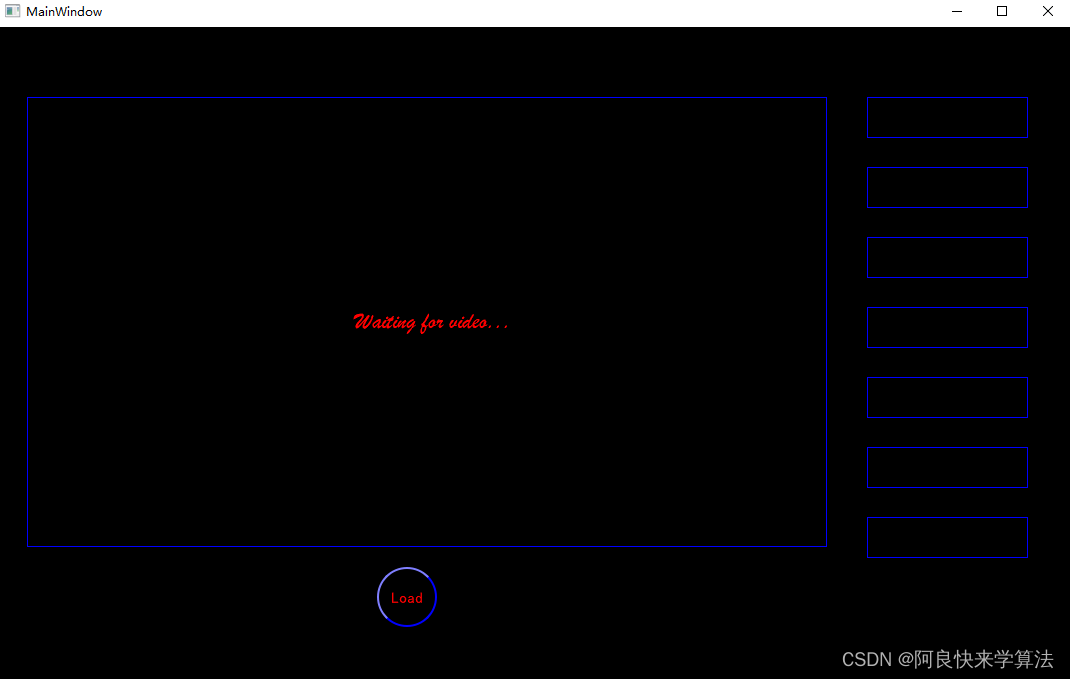 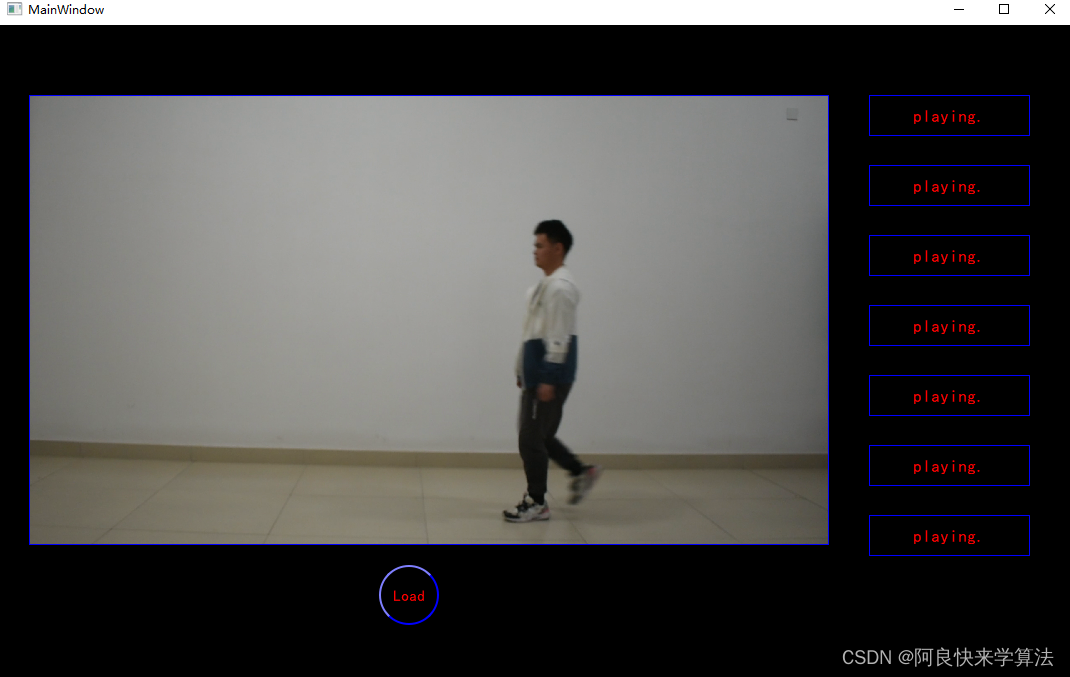
?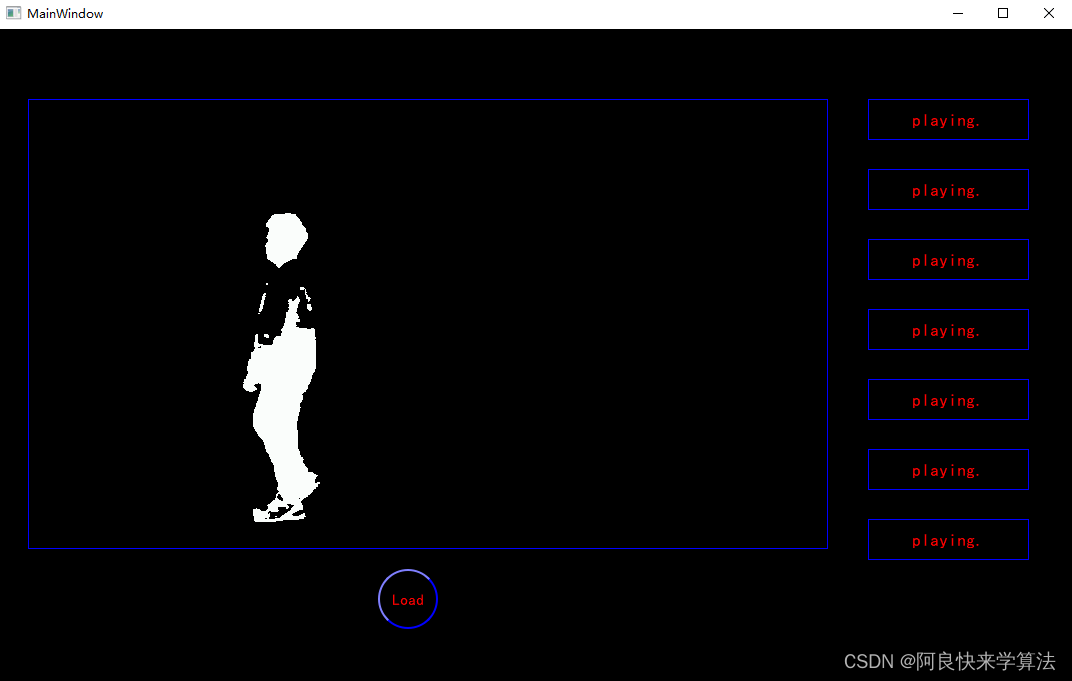
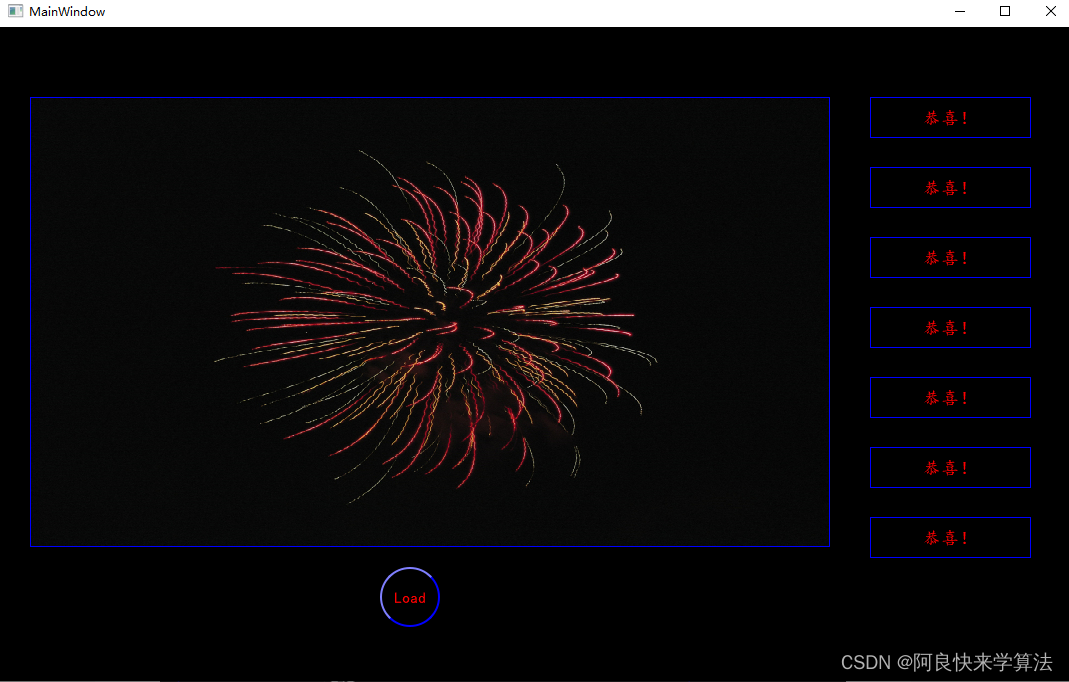
|