非原创,代码来源葁sir
import numpy as np
import pandas as pd
from pandas import Series,DataFrame
from sklearn.ensemble import AdaBoostRegressor,GradientBoostingRegressor
from sklearn.datasets import load_boston
from sklearn.neighbors import KNeighborsRegressor
boston = load_boston()
data = boston.data
target = boston.target
feature_names = boston.feature_names
knn = KNeighborsRegressor()
from sklearn.model_selection import train_test_split
X_train,X_test,y_train,y_test = train_test_split(data, target, test_size=0.2, random_state=1)
X_train = DataFrame(data=X_train,columns=feature_names)
X_train
| CRIM | ZN | INDUS | CHAS | NOX | RM | AGE | DIS | RAD | TAX | PTRATIO | B | LSTAT |
---|
0 | 0.14150 | 0.0 | 6.91 | 0.0 | 0.448 | 6.169 | 6.6 | 5.7209 | 3.0 | 233.0 | 17.9 | 383.37 | 5.81 |
---|
1 | 0.15445 | 25.0 | 5.13 | 0.0 | 0.453 | 6.145 | 29.2 | 7.8148 | 8.0 | 284.0 | 19.7 | 390.68 | 6.86 |
---|
2 | 16.81180 | 0.0 | 18.10 | 0.0 | 0.700 | 5.277 | 98.1 | 1.4261 | 24.0 | 666.0 | 20.2 | 396.90 | 30.81 |
---|
3 | 0.05646 | 0.0 | 12.83 | 0.0 | 0.437 | 6.232 | 53.7 | 5.0141 | 5.0 | 398.0 | 18.7 | 386.40 | 12.34 |
---|
4 | 8.79212 | 0.0 | 18.10 | 0.0 | 0.584 | 5.565 | 70.6 | 2.0635 | 24.0 | 666.0 | 20.2 | 3.65 | 17.16 |
---|
... | ... | ... | ... | ... | ... | ... | ... | ... | ... | ... | ... | ... | ... |
---|
399 | 0.03548 | 80.0 | 3.64 | 0.0 | 0.392 | 5.876 | 19.1 | 9.2203 | 1.0 | 315.0 | 16.4 | 395.18 | 9.25 |
---|
400 | 0.09164 | 0.0 | 10.81 | 0.0 | 0.413 | 6.065 | 7.8 | 5.2873 | 4.0 | 305.0 | 19.2 | 390.91 | 5.52 |
---|
401 | 5.87205 | 0.0 | 18.10 | 0.0 | 0.693 | 6.405 | 96.0 | 1.6768 | 24.0 | 666.0 | 20.2 | 396.90 | 19.37 |
---|
402 | 0.33045 | 0.0 | 6.20 | 0.0 | 0.507 | 6.086 | 61.5 | 3.6519 | 8.0 | 307.0 | 17.4 | 376.75 | 10.88 |
---|
403 | 0.08014 | 0.0 | 5.96 | 0.0 | 0.499 | 5.850 | 41.5 | 3.9342 | 5.0 | 279.0 | 19.2 | 396.90 | 8.77 |
---|
404 rows × 13 columns
X_train.describe().T
| count | mean | std | min | 25% | 50% | 75% | max |
---|
CRIM | 404.0 | 3.697455 | 9.146743 | 0.00632 | 0.082598 | 0.234405 | 3.594927 | 88.9762 |
---|
ZN | 404.0 | 11.527228 | 23.288284 | 0.00000 | 0.000000 | 0.000000 | 20.000000 | 100.0000 |
---|
INDUS | 404.0 | 11.077500 | 6.848412 | 0.46000 | 5.190000 | 9.125000 | 18.100000 | 27.7400 |
---|
CHAS | 404.0 | 0.079208 | 0.270398 | 0.00000 | 0.000000 | 0.000000 | 0.000000 | 1.0000 |
---|
NOX | 404.0 | 0.553026 | 0.116895 | 0.38500 | 0.448000 | 0.535000 | 0.624000 | 0.8710 |
---|
RM | 404.0 | 6.268792 | 0.689229 | 3.56100 | 5.876750 | 6.179000 | 6.626500 | 8.7800 |
---|
AGE | 404.0 | 67.935644 | 28.563186 | 2.90000 | 43.250000 | 76.800000 | 93.825000 | 100.0000 |
---|
DIS | 404.0 | 3.826111 | 2.120999 | 1.12960 | 2.105350 | 3.298600 | 5.141475 | 12.1265 |
---|
RAD | 404.0 | 9.470297 | 8.680237 | 1.00000 | 4.000000 | 5.000000 | 24.000000 | 24.0000 |
---|
TAX | 404.0 | 403.257426 | 169.030480 | 187.00000 | 277.000000 | 329.000000 | 666.000000 | 711.0000 |
---|
PTRATIO | 404.0 | 18.438614 | 2.169469 | 12.60000 | 17.225000 | 19.000000 | 20.200000 | 22.0000 |
---|
B | 404.0 | 357.153688 | 91.541647 | 0.32000 | 376.092500 | 391.575000 | 396.157500 | 396.9000 |
---|
LSTAT | 404.0 | 12.778540 | 7.216403 | 1.73000 | 7.092500 | 11.465000 | 17.102500 | 37.9700 |
---|
X_train.min()
CRIM 0.00632
ZN 0.00000
INDUS 0.46000
CHAS 0.00000
NOX 0.38500
RM 3.56100
AGE 2.90000
DIS 1.12960
RAD 1.00000
TAX 187.00000
PTRATIO 12.60000
B 0.32000
LSTAT 1.73000
dtype: float64
from sklearn.preprocessing import MinMaxScaler
mms = MinMaxScaler()
data = mms.fit_transform(X_train)
feature_names
array(['CRIM', 'ZN', 'INDUS', 'CHAS', 'NOX', 'RM', 'AGE', 'DIS', 'RAD',
'TAX', 'PTRATIO', 'B', 'LSTAT'], dtype='<U7')
X_train = pd.DataFrame(data=data,columns=feature_names)
X_train
| CRIM | ZN | INDUS | CHAS | NOX | RM | AGE | DIS | RAD | TAX | PTRATIO | B | LSTAT |
---|
0 | 0.001519 | 0.00 | 0.236437 | 0.0 | 0.129630 | 0.499713 | 0.038105 | 0.417509 | 0.086957 | 0.087786 | 0.563830 | 0.965883 | 0.112583 |
---|
1 | 0.001665 | 0.25 | 0.171188 | 0.0 | 0.139918 | 0.495114 | 0.270855 | 0.607917 | 0.304348 | 0.185115 | 0.755319 | 0.984316 | 0.141556 |
---|
2 | 0.188890 | 0.00 | 0.646628 | 0.0 | 0.648148 | 0.328799 | 0.980433 | 0.026962 | 1.000000 | 0.914122 | 0.808511 | 1.000000 | 0.802428 |
---|
3 | 0.000564 | 0.00 | 0.453446 | 0.0 | 0.106996 | 0.511784 | 0.523172 | 0.353236 | 0.173913 | 0.402672 | 0.648936 | 0.973524 | 0.292770 |
---|
4 | 0.098750 | 0.00 | 0.646628 | 0.0 | 0.409465 | 0.383982 | 0.697219 | 0.084924 | 1.000000 | 0.914122 | 0.808511 | 0.008397 | 0.425773 |
---|
... | ... | ... | ... | ... | ... | ... | ... | ... | ... | ... | ... | ... | ... |
---|
399 | 0.000328 | 0.80 | 0.116569 | 0.0 | 0.014403 | 0.443572 | 0.166838 | 0.735726 | 0.000000 | 0.244275 | 0.404255 | 0.995663 | 0.207506 |
---|
400 | 0.000959 | 0.00 | 0.379399 | 0.0 | 0.057613 | 0.479785 | 0.050463 | 0.378079 | 0.130435 | 0.225191 | 0.702128 | 0.984896 | 0.104581 |
---|
401 | 0.065929 | 0.00 | 0.646628 | 0.0 | 0.633745 | 0.544932 | 0.958805 | 0.049759 | 1.000000 | 0.914122 | 0.808511 | 1.000000 | 0.486755 |
---|
402 | 0.003643 | 0.00 | 0.210411 | 0.0 | 0.251029 | 0.483809 | 0.603502 | 0.229365 | 0.304348 | 0.229008 | 0.510638 | 0.949191 | 0.252483 |
---|
403 | 0.000830 | 0.00 | 0.201613 | 0.0 | 0.234568 | 0.438590 | 0.397528 | 0.255036 | 0.173913 | 0.175573 | 0.702128 | 1.000000 | 0.194260 |
---|
404 rows × 13 columns
knn.fit(X_train,y_train)
KNeighborsRegressor()
from sklearn.metrics import mean_squared_error
mean_squared_error(y_train,knn.predict(X_train))
15.028524752475246
mean_squared_error(y_test,knn.predict(X_test))
204.1549686274509
实验:adaboosting
aba = AdaBoostRegressor(base_estimator=KNeighborsRegressor(),n_estimators=100)
aba.fit(X_train,y_train)
AdaBoostRegressor(base_estimator=KNeighborsRegressor(), n_estimators=100)
mean_squared_error(y_train,aba.predict(X_train))
4.755455445544554
mean_squared_error(y_test,aba.predict(X_test))
127.99668627450978
err_list = []
for i,y_ in enumerate(aba.staged_predict(X_test)):
err = mean_squared_error(y_test,y_)
err_list.append(err)
print('C{}:ERROR:{}'.format(i,err))
C0:ERROR:199.32693725490194
C1:ERROR:199.32693725490194
C2:ERROR:220.0897333333333
C3:ERROR:199.7595607843137
C4:ERROR:207.813894117647
C5:ERROR:207.82781176470584
C6:ERROR:192.8428588235294
C7:ERROR:190.94257647058825
C8:ERROR:192.6760862745098
C9:ERROR:189.0082431372549
C10:ERROR:188.7143254901961
C11:ERROR:184.35898823529416
C12:ERROR:179.3270117647059
C13:ERROR:178.614568627451
C14:ERROR:166.1954274509804
C15:ERROR:169.03231372549018
C16:ERROR:158.3203725490196
C17:ERROR:158.4866470588235
C18:ERROR:142.9087137254902
C19:ERROR:158.1416
C20:ERROR:142.4846666666667
C21:ERROR:142.4846666666667
C22:ERROR:157.30826666666667
C23:ERROR:157.38923529411767
C24:ERROR:157.35952941176473
C25:ERROR:142.61410196078435
C26:ERROR:135.35090980392155
C27:ERROR:142.68588627450984
C28:ERROR:135.77389411764707
C29:ERROR:142.68588627450984
C30:ERROR:142.04365098039216
C31:ERROR:141.93987843137253
C32:ERROR:135.87796470588236
C33:ERROR:141.93987843137253
C34:ERROR:141.82033725490197
C35:ERROR:141.95123529411765
C36:ERROR:135.73551764705883
C37:ERROR:141.9422549019608
C38:ERROR:135.90520784313725
C39:ERROR:141.4849843137255
C40:ERROR:135.90342352941178
C41:ERROR:142.09143921568628
C42:ERROR:135.91702352941178
C43:ERROR:136.00622745098042
C44:ERROR:135.89461960784314
C45:ERROR:142.01684705882352
C46:ERROR:135.91702352941178
C47:ERROR:136.18957647058824
C48:ERROR:136.2971137254902
C49:ERROR:136.19823137254903
C50:ERROR:134.7982470588235
C51:ERROR:136.41440000000003
C52:ERROR:136.29789803921568
C53:ERROR:136.4323411764706
C54:ERROR:134.9813450980392
C55:ERROR:136.3194980392157
C56:ERROR:136.40856078431375
C57:ERROR:136.41440000000003
C58:ERROR:142.32514901960786
C59:ERROR:136.48449803921568
C60:ERROR:142.32336470588237
C61:ERROR:142.43412941176473
C62:ERROR:142.40421176470588
C63:ERROR:136.38720784313728
C64:ERROR:142.4454392156863
C65:ERROR:136.4293843137255
C66:ERROR:142.46783137254903
C67:ERROR:136.48109019607844
C68:ERROR:142.5336549019608
C69:ERROR:144.18005490196077
C70:ERROR:142.50667450980393
C71:ERROR:136.4272549019608
C72:ERROR:142.50667450980393
C73:ERROR:142.5022117647059
C74:ERROR:142.5354392156863
C75:ERROR:136.4629137254902
C76:ERROR:136.31138823529412
C77:ERROR:135.0016
C78:ERROR:136.49930196078432
C79:ERROR:135.03171764705883
C80:ERROR:135.01974901960781
C81:ERROR:128.98411372549018
C82:ERROR:135.078631372549
C83:ERROR:129.0248196078431
C84:ERROR:135.078631372549
C85:ERROR:128.98411372549018
C86:ERROR:135.078631372549
C87:ERROR:128.9571333333333
C88:ERROR:128.96369803921567
C89:ERROR:128.6254862745098
C90:ERROR:129.0248196078431
C91:ERROR:128.6512274509804
C92:ERROR:128.39080392156865
C93:ERROR:128.39080392156865
C94:ERROR:128.40443529411766
C95:ERROR:128.68032549019608
C96:ERROR:128.39080392156865
C97:ERROR:128.2014862745098
C98:ERROR:128.39080392156865
C99:ERROR:127.99668627450978
import seaborn as sns
import matplotlib.pyplot as plt
%matplotlib inline
sns.set()
plt.plot(err_list)
[<matplotlib.lines.Line2D at 0x1e4f02ccf40>]
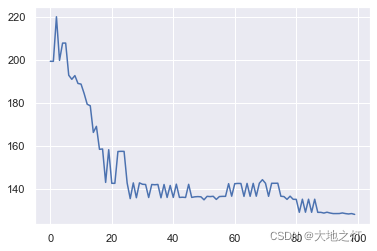
plt.plot(aba.estimator_errors_)
[<matplotlib.lines.Line2D at 0x1e4ee793070>]
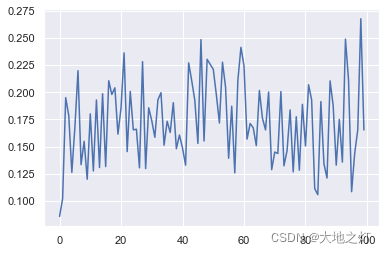
plt.plot(aba.estimator_weights_)
[<matplotlib.lines.Line2D at 0x1e4ee42f940>]
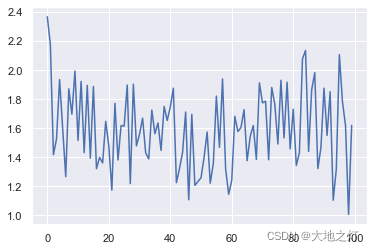
GBDT观察表现
from sklearn.ensemble import RandomForestRegressor
RandomForestRegressor()
gbdt = GradientBoostingRegressor(n_estimators=100)
gbdt.fit(X_train,y_train)
GradientBoostingRegressor()
mean_squared_error(y_train,gbdt.predict(X_train))
1.7840841714565248
mean_squared_error(y_test,gbdt.predict(X_test))
159.79621357980093
特征评估结果
---------------------------------------------------------------------------
AttributeError Traceback (most recent call last)
D:\software\anaconda\lib\site-packages\sklearn\ensemble\_weight_boosting.py in feature_importances_(self)
253 norm = self.estimator_weights_.sum()
--> 254 return (sum(weight * clf.feature_importances_ for weight, clf
255 in zip(self.estimator_weights_, self.estimators_))
D:\software\anaconda\lib\site-packages\sklearn\ensemble\_weight_boosting.py in <genexpr>(.0)
253 norm = self.estimator_weights_.sum()
--> 254 return (sum(weight * clf.feature_importances_ for weight, clf
255 in zip(self.estimator_weights_, self.estimators_))
AttributeError: 'KNeighborsRegressor' object has no attribute 'feature_importances_'
?
The above exception was the direct cause of the following exception:
AttributeError Traceback (most recent call last)
~\AppData\Local\Temp/ipykernel_12168/2668898732.py in <module>
----> 1 aba.feature_importances_
D:\software\anaconda\lib\site-packages\sklearn\ensemble\_weight_boosting.py in feature_importances_(self)
257
258 except AttributeError as e:
--> 259 raise AttributeError(
260 "Unable to compute feature importances "
261 "since base_estimator does not have a "
AttributeError: Unable to compute feature importances since base_estimator does not have a feature_importances_ attribute
pd.Series(data=gbdt.feature_importances_,index=feature_names).plot(kind='bar')
<AxesSubplot:>
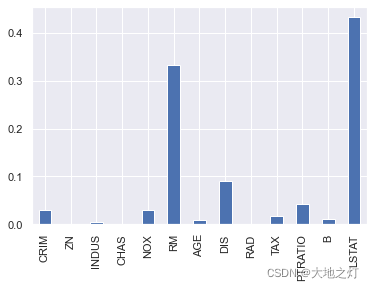
|