系列文章目录
强化学习
提示:写完文章后,目录可以自动生成,如何生成可参考右边的帮助文档
前言
强化学习(Reinforcement Learning, RL),又称再励学习、评价学习或增强学习,是机器学习的范式和方法论之一,用于描述和解决智能体(agent)在与环境的交互过程中通过学习策略以达成回报最大化或实现特定目标的问题 。
一、强化学习是什么?
强化学习是智能体(Agent)以“试错”的方式进行学习,通过与环境进行交互获得的奖赏指导行为,目标是使智能体获得最大的奖赏,强化学习不同于连接主义学习中的监督学习,主要表现在强化信号上,强化学习中由环境提供的强化信号是对产生动作的好坏作一种评价(通常为标量信号),而不是告诉强化学习系统RLS(reinforcement learning system)如何去产生正确的动作。由于外部环境提供的信息很少,RLS必须靠自身的经历进行学习。通过这种方式,RLS在行动-评价的环境中获得知识,改进行动方案以适应环境。 理解:强化学习其实就是和人一样,一开始是什么都不懂的,所谓吃一堑长一智,他像一个新生的孩子,它在不断的试错过程中慢慢知道了做什么有奖励,做什么对得到奖励会有一定的价值,做什么会被打。在这个过程中不会像监督学习一样有个师傅带你,完全需要自己去摸索,就像修仙宗门一样,有背景的宗门弟子是继承掌门之位(监督),创立宗门的人是开山立派(强化),必须一步一个脚印去不断成长。
其实强化学习吸引我的就是因为它主要使用在游戏上,例如: 在 Flappy bird 这个游戏中,我们需要简单的点击操作来控制小鸟,躲过各种水管,飞的越远越好,因为飞的越远就能获得更高的积分奖励。
机器有一个玩家小鸟——Agent 需要控制小鸟飞的更远——目标 整个游戏过程中需要躲避各种水管——环境 躲避水管的方法是让小鸟用力飞一下——行动 飞的越远,就会获得越多的积分——奖励 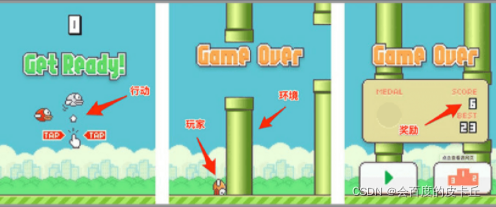
二、核心算法(深度强化学习) DQN(先学会1.0的再搞2.0的)
DQN其实可以拆分成Qlearning的主框架加上一些功能: 记忆库:重复学习,缩短时间 神经网络计算Q值:代替原有的表格形式,分析Q值 冻结q_target参数:切断相关性,让训练的效果更好,效率更高
from maze_env import Maze
from RL_brain import DeepQNetwork
def run_maze():
step = 0
for episode in range(300):
observation = env.reset()
while True:
env.render()
action = RL.choose_action(observation)
observation_, reward, done = env.step(action)
RL.store_transition(observation, action, reward, observation_)
if (step > 200) and (step % 5 == 0):
RL.learn()
observation = observation_
if done:
break
step += 1
print('game over')
env.destroy()
if __name__ == "__main__":
env = Maze()
RL = DeepQNetwork(env.n_actions, env.n_features,
learning_rate=0.01,
reward_decay=0.9,
e_greedy=0.9,
replace_target_iter=200,
memory_size=2000,
)
env.after(100, run_maze)
env.mainloop()
RL.plot_cost()
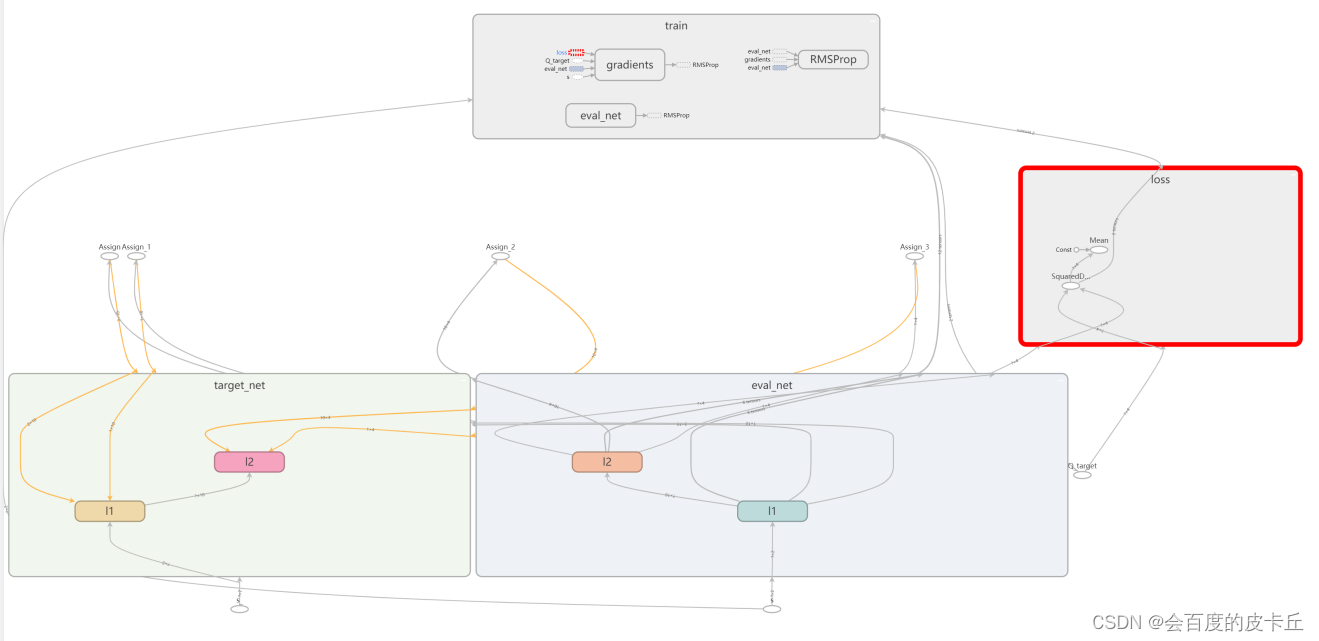 DQN的神经网络是有两个的,一个是记录最新的,一个是记录最近的动作,奖励等。 tensorflow1.x模型如下:
import numpy as np
import pandas as pd
import tensorflow as tf
np.random.seed(1)
tf.set_random_seed(1)
class DeepQNetwork:
def __init__(
self,
n_actions,
n_features,
learning_rate=0.01,
reward_decay=0.9,
e_greedy=0.9,
replace_target_iter=300,
memory_size=500,
batch_size=32,
e_greedy_increment=None,
output_graph=False,
):
self.n_actions = n_actions
self.n_features = n_features
self.lr = learning_rate
self.gamma = reward_decay
self.epsilon_max = e_greedy
self.replace_target_iter = replace_target_iter
self.memory_size = memory_size
self.batch_size = batch_size
self.epsilon_increment = e_greedy_increment
self.epsilon = 0 if e_greedy_increment is not None else self.epsilon_max
self.learn_step_counter = 0
self.memory = np.zeros((self.memory_size, n_features * 2 + 2))
self._build_net()
t_params = tf.get_collection('target_net_params')
e_params = tf.get_collection('eval_net_params')
self.replace_target_op = [tf.assign(t, e) for t, e in zip(t_params, e_params)]
self.sess = tf.Session()
if output_graph:
tf.summary.FileWriter("logs/", self.sess.graph)
self.sess.run(tf.global_variables_initializer())
self.cost_his = []
def _build_net(self):
self.s = tf.placeholder(tf.float32, [None, self.n_features], name='s')
self.q_target = tf.placeholder(tf.float32, [None, self.n_actions], name='Q_target')
with tf.variable_scope('eval_net'):
c_names, n_l1, w_initializer, b_initializer = \
['eval_net_params', tf.GraphKeys.GLOBAL_VARIABLES], 10, \
tf.random_normal_initializer(0., 0.3), tf.constant_initializer(0.1)
with tf.variable_scope('l1'):
w1 = tf.get_variable('w1', [self.n_features, n_l1], initializer=w_initializer, collections=c_names)
b1 = tf.get_variable('b1', [1, n_l1], initializer=b_initializer, collections=c_names)
l1 = tf.nn.relu(tf.matmul(self.s, w1) + b1)
with tf.variable_scope('l2'):
w2 = tf.get_variable('w2', [n_l1, self.n_actions], initializer=w_initializer, collections=c_names)
b2 = tf.get_variable('b2', [1, self.n_actions], initializer=b_initializer, collections=c_names)
self.q_eval = tf.matmul(l1, w2) + b2
with tf.variable_scope('loss'):
self.loss = tf.reduce_mean(tf.squared_difference(self.q_target, self.q_eval))
with tf.variable_scope('train'):
self._train_op = tf.train.RMSPropOptimizer(self.lr).minimize(self.loss)
self.s_ = tf.placeholder(tf.float32, [None, self.n_features], name='s_')
with tf.variable_scope('target_net'):
c_names = ['target_net_params', tf.GraphKeys.GLOBAL_VARIABLES]
with tf.variable_scope('l1'):
w1 = tf.get_variable('w1', [self.n_features, n_l1], initializer=w_initializer, collections=c_names)
b1 = tf.get_variable('b1', [1, n_l1], initializer=b_initializer, collections=c_names)
l1 = tf.nn.relu(tf.matmul(self.s_, w1) + b1)
with tf.variable_scope('l2'):
w2 = tf.get_variable('w2', [n_l1, self.n_actions], initializer=w_initializer, collections=c_names)
b2 = tf.get_variable('b2', [1, self.n_actions], initializer=b_initializer, collections=c_names)
self.q_next = tf.matmul(l1, w2) + b2
def store_transition(self, s, a, r, s_):
if not hasattr(self, 'memory_counter'):
self.memory_counter = 0
transition = np.hstack((s, [a, r], s_))
index = self.memory_counter % self.memory_size
self.memory[index, :] = transition
self.memory_counter += 1
def choose_action(self, observation):
observation = observation[np.newaxis, :]
if np.random.uniform() < self.epsilon:
actions_value = self.sess.run(self.q_eval, feed_dict={self.s: observation})
action = np.argmax(actions_value)
else:
action = np.random.randint(0, self.n_actions)
return action
def learn(self):
if self.learn_step_counter % self.replace_target_iter == 0:
self.sess.run(self.replace_target_op)
print('\ntarget_params_replaced\n')
if self.memory_counter > self.memory_size:
sample_index = np.random.choice(self.memory_size, size=self.batch_size)
else:
sample_index = np.random.choice(self.memory_counter, size=self.batch_size)
batch_memory = self.memory[sample_index, :]
q_next, q_eval = self.sess.run(
[self.q_next, self.q_eval],
feed_dict={
self.s_: batch_memory[:, -self.n_features:],
self.s: batch_memory[:, :self.n_features],
})
q_target = q_eval.copy()
batch_index = np.arange(self.batch_size, dtype=np.int32)
eval_act_index = batch_memory[:, self.n_features].astype(int)
reward = batch_memory[:, self.n_features + 1]
q_target[batch_index, eval_act_index] = reward + self.gamma * np.max(q_next, axis=1)
"""
For example in this batch I have 2 samples and 3 actions:
q_eval =
[[1, 2, 3],
[4, 5, 6]]
q_target = q_eval =
[[1, 2, 3],
[4, 5, 6]]
Then change q_target with the real q_target value w.r.t the q_eval's action.
For example in:
sample 0, I took action 0, and the max q_target value is -1;
sample 1, I took action 2, and the max q_target value is -2:
q_target =
[[-1, 2, 3],
[4, 5, -2]]
So the (q_target - q_eval) becomes:
[[(-1)-(1), 0, 0],
[0, 0, (-2)-(6)]]
We then backpropagate this error w.r.t the corresponding action to network,
leave other action as error=0 cause we didn't choose it.
"""
_, self.cost = self.sess.run([self._train_op, self.loss],
feed_dict={self.s: batch_memory[:, :self.n_features],
self.q_target: q_target})
self.cost_his.append(self.cost)
self.epsilon = self.epsilon + self.epsilon_increment if self.epsilon < self.epsilon_max else self.epsilon_max
self.learn_step_counter += 1
def plot_cost(self):
import matplotlib.pyplot as plt
plt.plot(np.arange(len(self.cost_his)), self.cost_his)
plt.ylabel('Cost')
plt.xlabel('training steps')
plt.show()
总结
|