(一)参考博客和PPT原文件下载连接
首先感谢一下各位博主写的优秀文章供我们参考。
链接: 李宏毅老师self-attention和本文中用到的PPT下载 提取码:p63y –来自百度网盘超级会员V4的分享
(二)VIT原理详解
我们首先看一下Self-Attention的整体计算过程的结构图:(此图片来源于Multi-headed Self-attention(多头自注意力)机制介绍)。 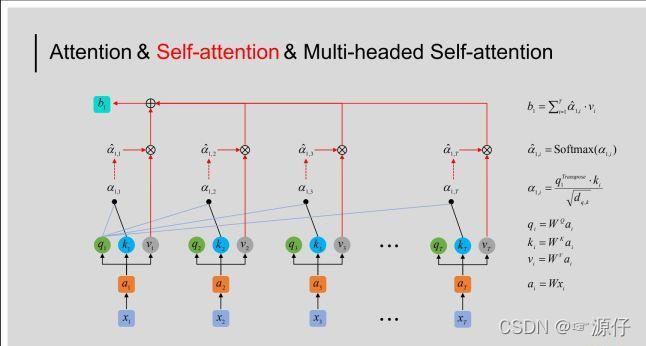
2.1、self-attention
首先我们看 图1,attention是啥意思? 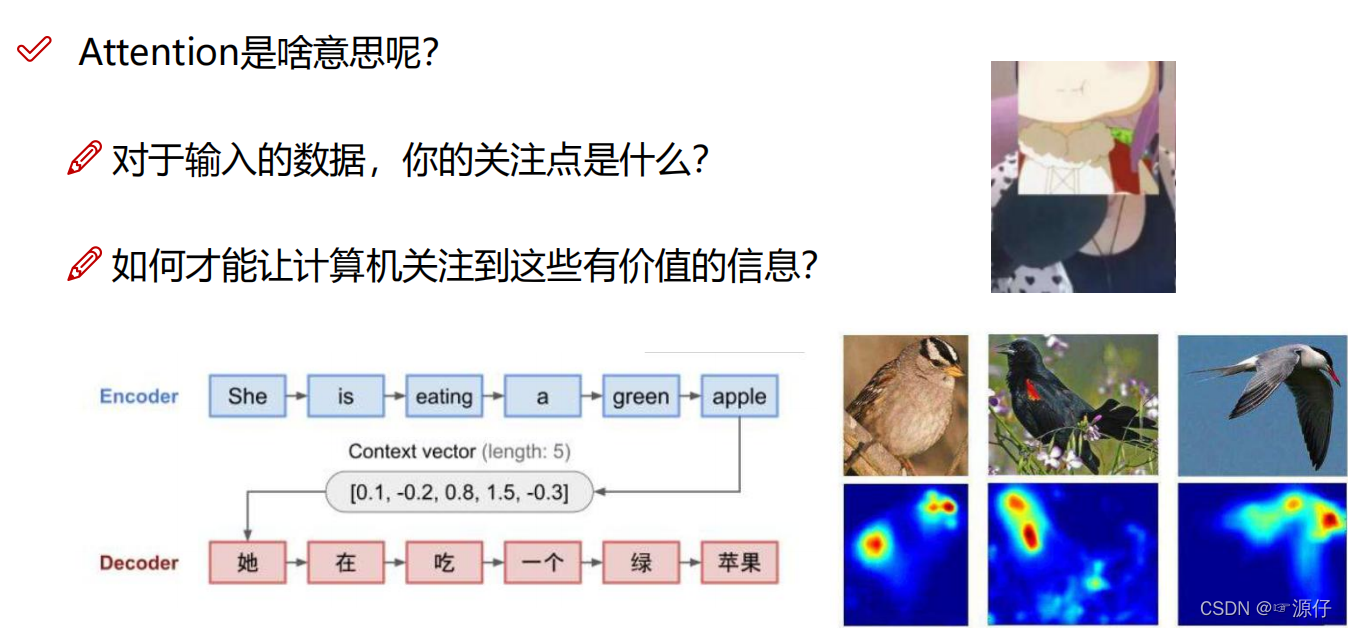 ????????????图1 、什么是attention 2、Transformer的整体框架 图2 所示: 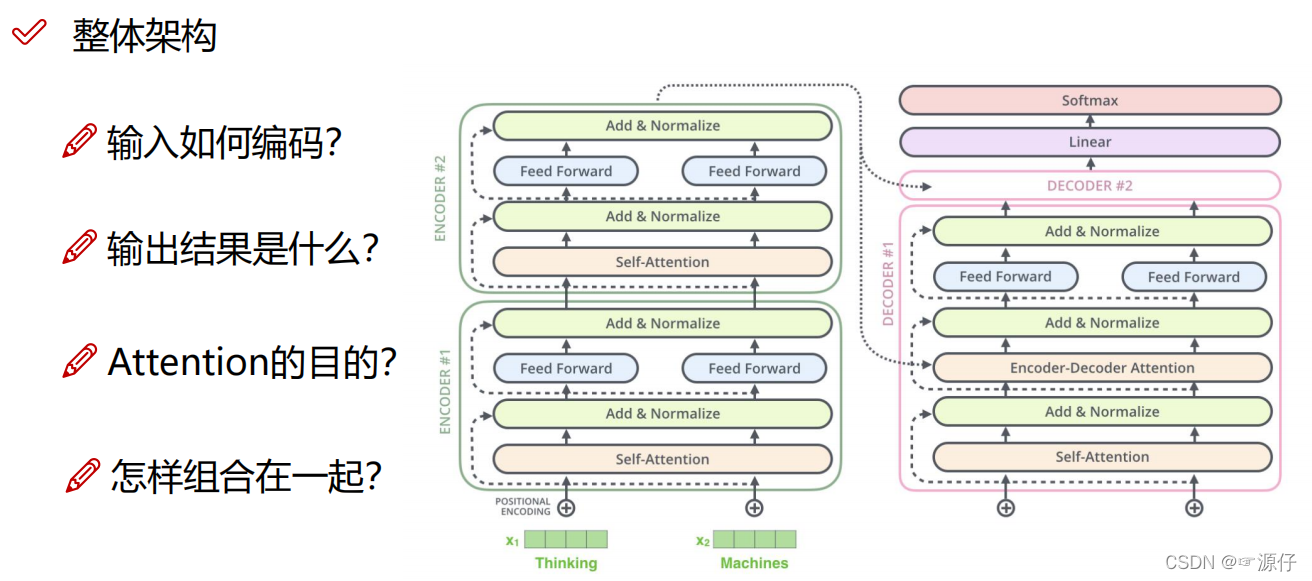 ????????????图2、Transformer的整体框架
3、self-attention是怎样计算的。如图3 所示: 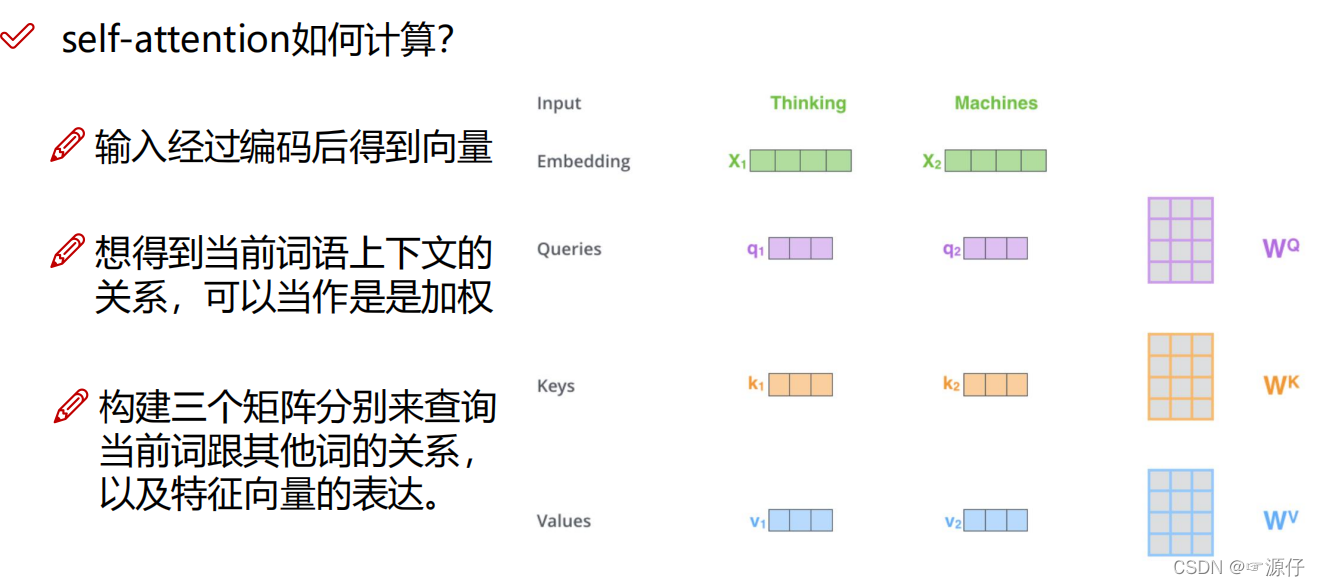 ???????????图3、self-attention是怎样计算的? 4、首先我们要知道三个参数量的名称和大概的作用:如图4:
- Q : query(查询):我们要去查询什么东西
- K : Key(关键):指被查询的东西
- V :value(值) :指的是对实际输入信息的提取的特征信息(大概和CNN中提取Feature Map的含义差不多)。
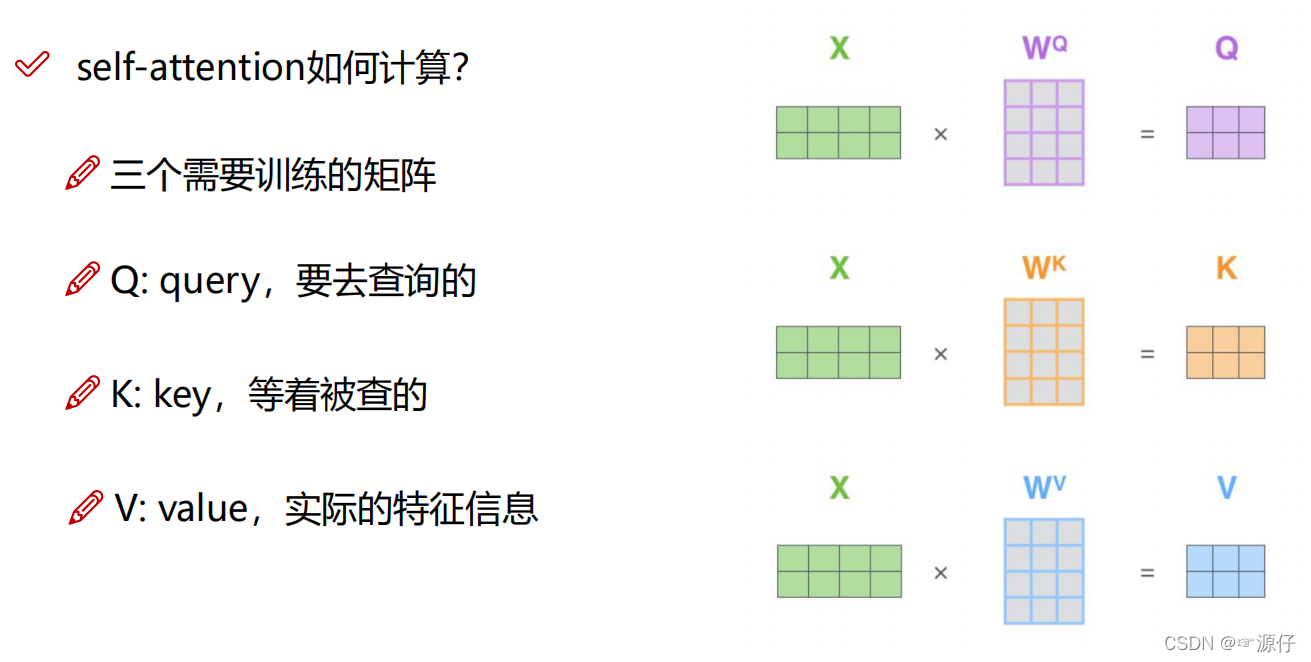
???????????图4、Q、K、V的含义
2.2、sequence序列之间相关性
α
\boldsymbol{\alpha}
α的求解
sequence序列之间相关性的求解:相关性用
α
\boldsymbol{\alpha}
α表示。因为self-attention的特点就是具有全局性,但是拥有全局性,必须使每个序列之间都要有关联。如下图5所示: 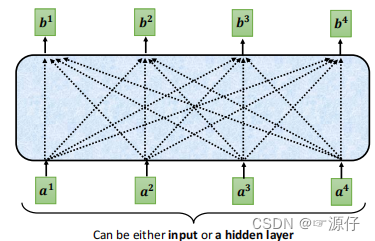 ???????????图5、全局性的表达 ??图5中是不是和全连接层很像,中间的是隐层,也就是权重
W
W
W。但是我们好像不能按照上面图5这样直接连接吧,不然
a
1
a^1
a1,
a
2
a^2
a2,…,
a
4
a^4
a4之间的相关性都一样,没有任何区别,那么输出的
b
1
b^1
b1,
b
2
b^2
b2,…,
b
4
b^4
b4那不就都一样了哈。所以我们要计算
a
1
a^1
a1,
a
2
a^2
a2,…,
a
4
a^4
a4之间的相关性
α
\boldsymbol{\alpha}
α。 ??我们先看图,好理解: 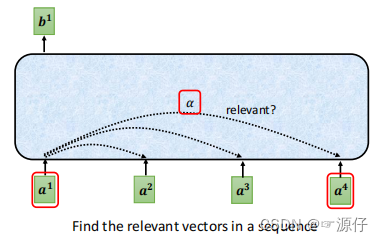 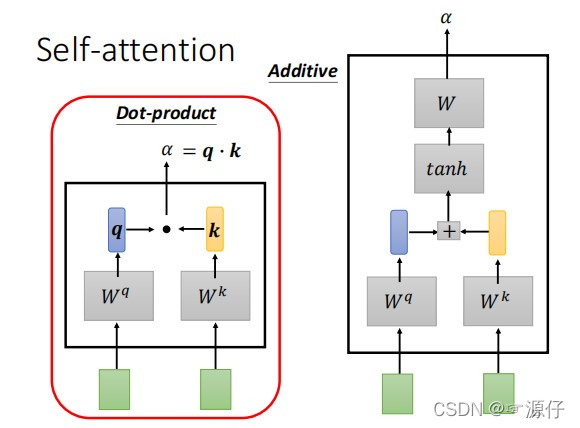 为什么上面用Dot-Product去计算相关性
α
\boldsymbol{\alpha}
α呢? ??向量的点乘可以用来计算两个向量之间的夹角,进一步判断这两个向量是否正交(垂直)等方向关系。 同时,还可以用来计算一个向量在另一个向量方向上的投影长度。 ??那么当两个向量的夹角为
9
0
°
90^\circ
90°时,Dot-Product的结果为0,这里表示相关性为0;当两个向量重合或平行时,Dot-Product的结果为无穷大,想一想当两个向量平行时,是不是代表这两个向量之间是不是成比例关系,那这两个向量是不是相似(即指这里的相关性),所以当点乘之间的结果越大,他们的相关性越强。 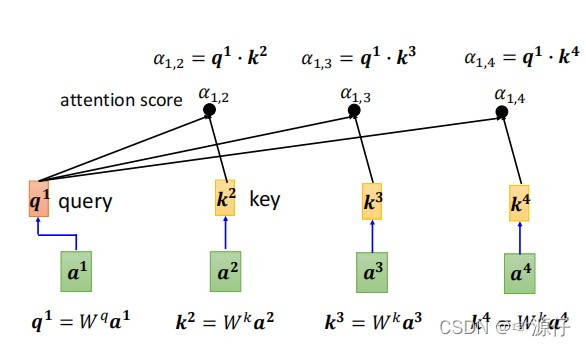 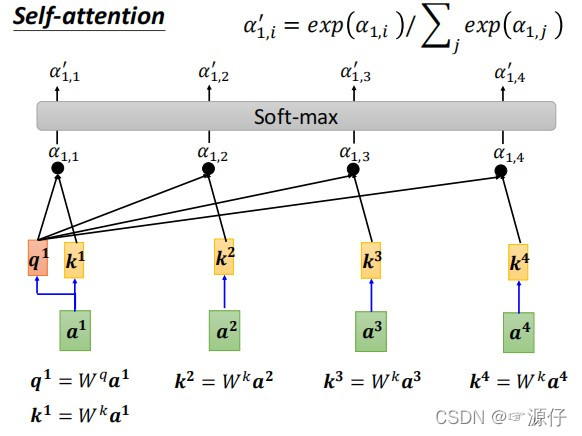 下面我们看一下用矩阵表示时候的计算过程图吧: 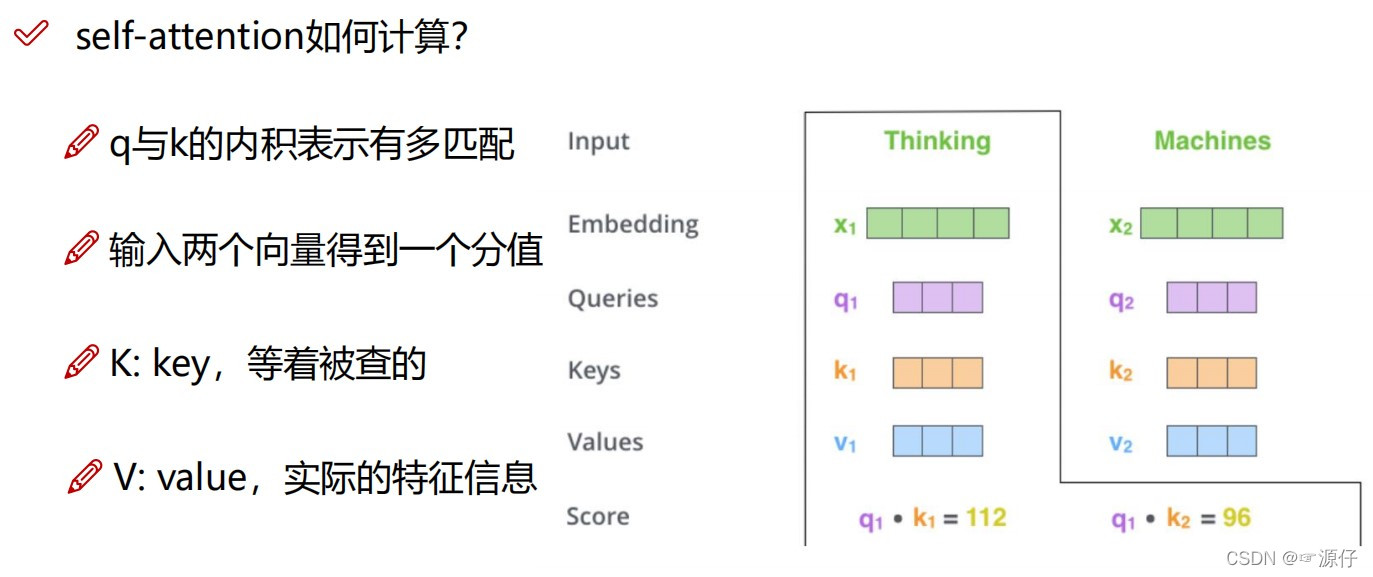 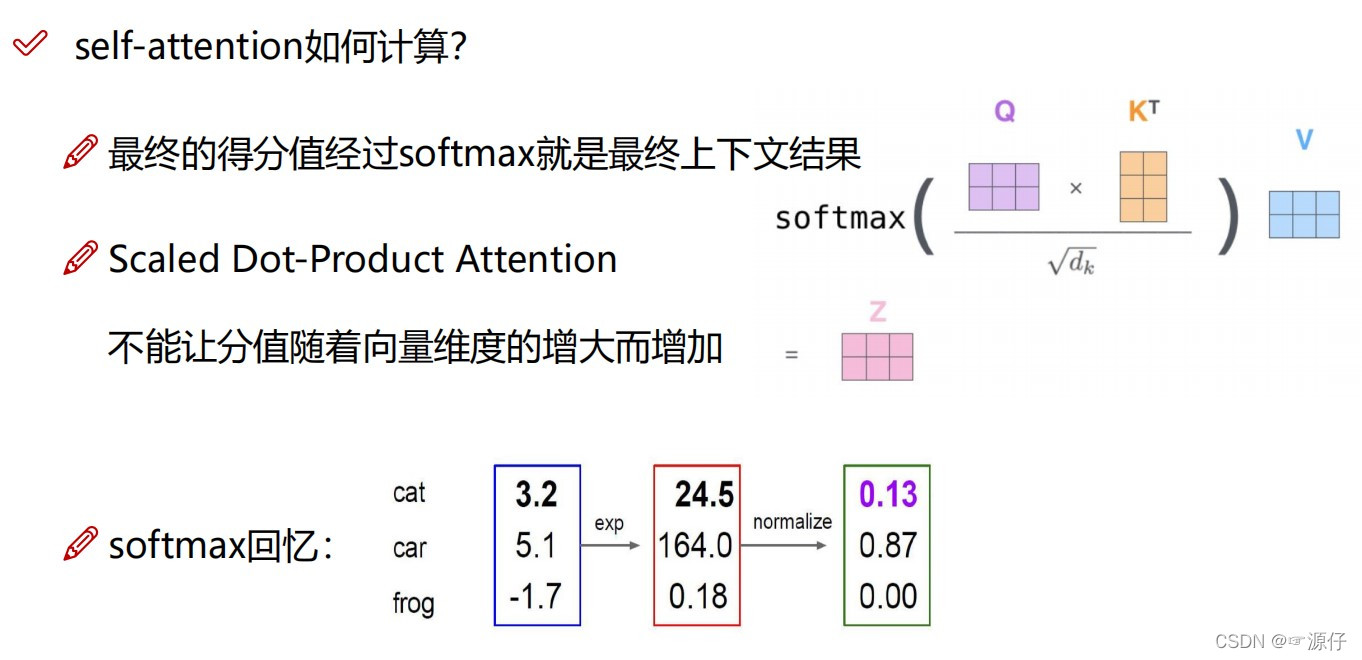 由上图我们可以注意到一个公式:
A
t
t
e
n
t
i
o
n
(
Q
,
K
,
V
)
=
s
o
f
t
m
a
x
(
Q
K
T
d
k
)
V
=
s
o
f
t
m
a
x
(
α
d
k
)
V
Attention(Q,K,V) = softmax(\frac {QK^T}{\sqrt{d_k}}) V= softmax(\frac {\boldsymbol{\alpha}}{\sqrt{d_k}}) V
Attention(Q,K,V)=softmax(dk?
?QKT?)V=softmax(dk?
?α?)V:
除以
d
k
\sqrt{d_k}
dk?
?的作用:
??如果Dot-Product点乘的结果很小,Additive Attention 和 Dot-Product-Attention的效果差不多。 ??如果Dot-Product点乘的结果很大,如果不除以
d
k
\sqrt{d_k}
dk?
?做Scaling,那么结果就不如Additive Attention。 ??此外,点乘结果过大,在进行Softmax之后的梯度会变得很小,不利于反向传播。
2.3、Value与相关性
α
\boldsymbol{\alpha}
α之间的计算
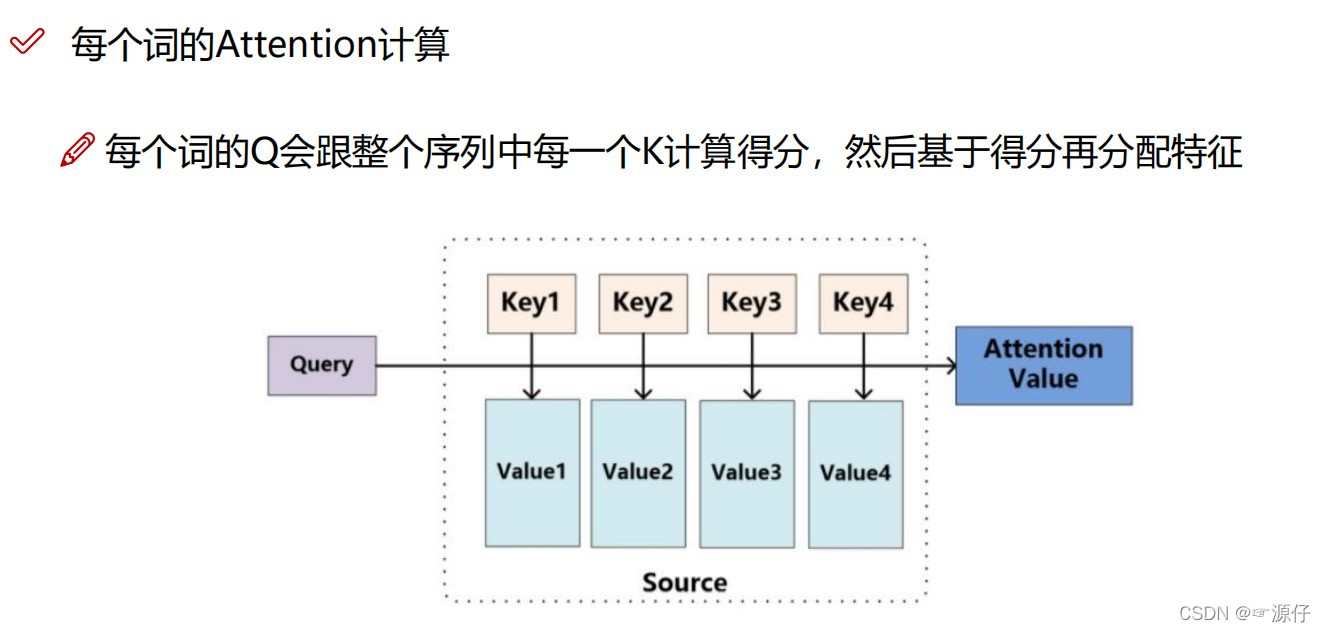 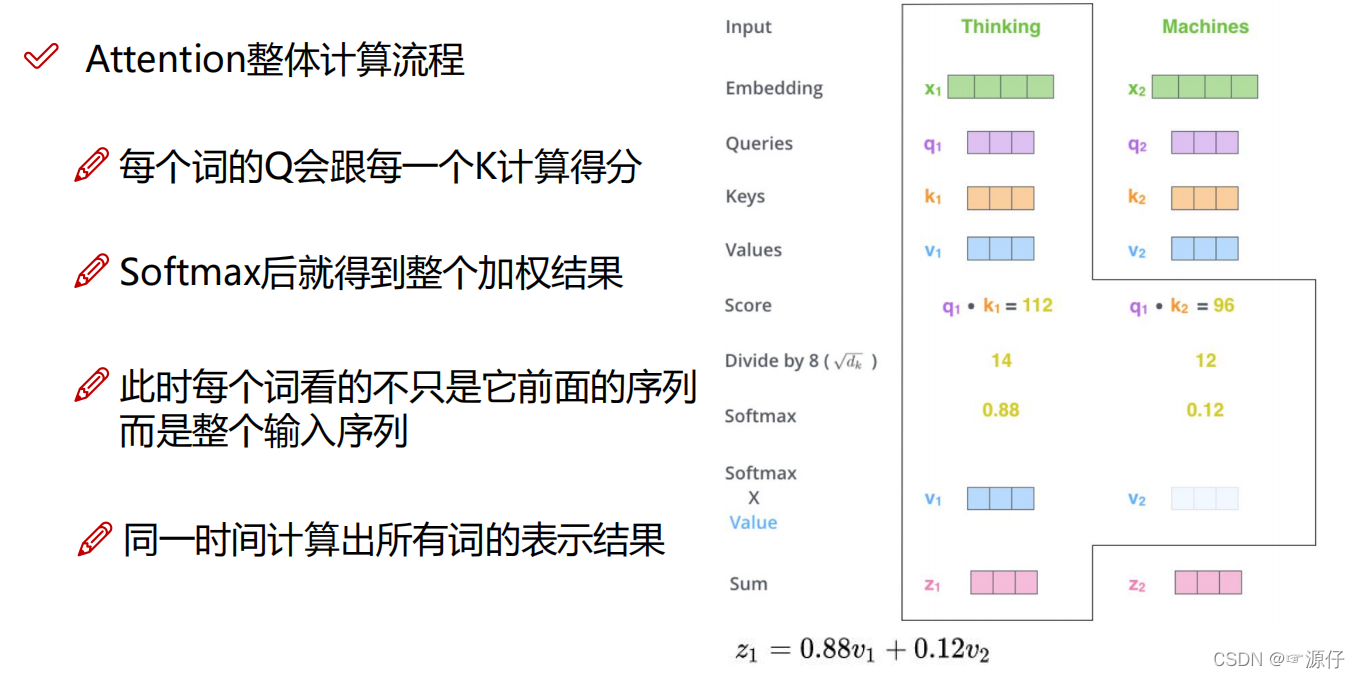
2.4、多头注意力机制
在Transformer及BERT模型中用到的Multi-headed Self-attention结构与之略有差异,具体体现在:如果将前文中得到的
q
i
,
k
i
,
v
i
q_i,k_i,v_i
qi?,ki?,vi?,整体看做一个“头”,则“多头”即指对于特定的
x
i
x_i
xi?来说,需要用多组
W
Q
,
W
K
,
W
V
W^Q,W^K,W^V
WQ,WK,WV与之相乘,进而得到多组
q
i
,
k
i
,
v
i
q_i,k_i,v_i
qi?,ki?,vi?。如下图所示: 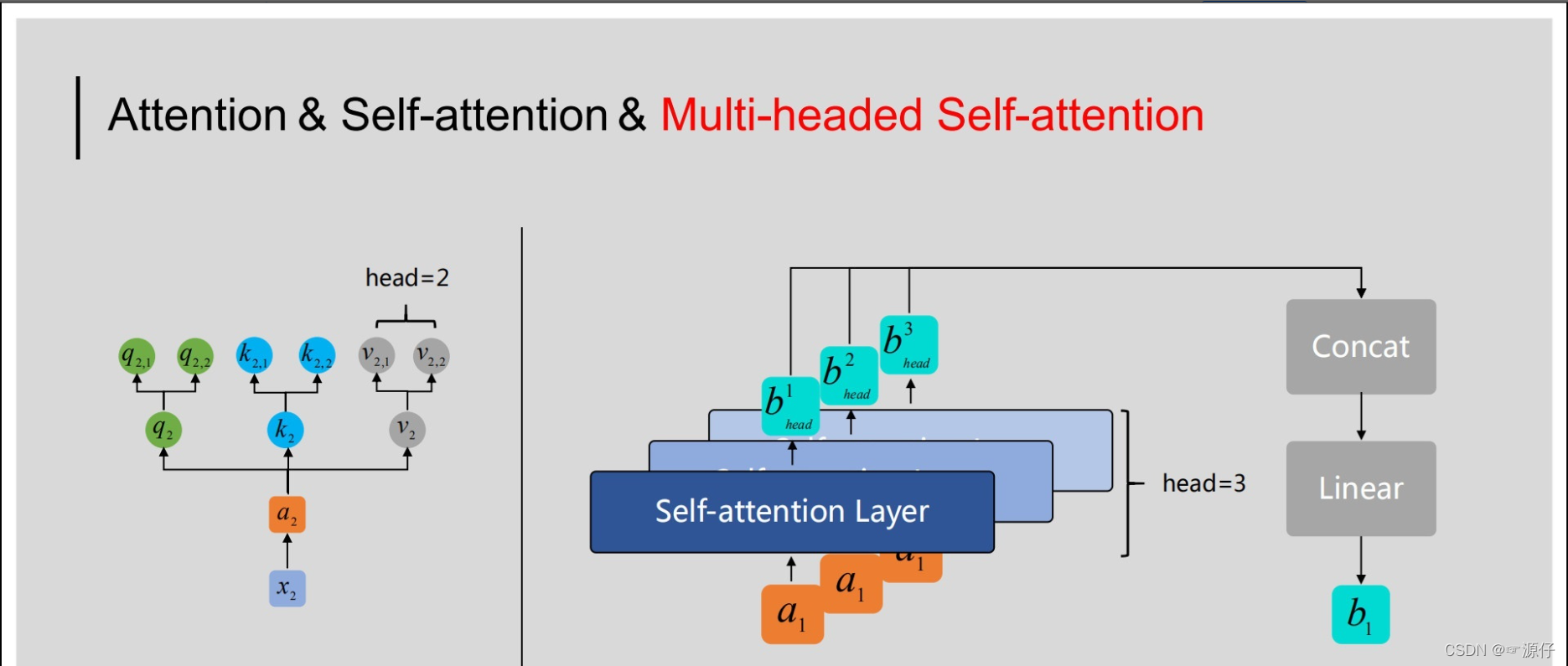 如上图所示,以右侧示意图中输入的
a
1
a_1
a1?为例,通过多头(这里取head=3)机制得到了三个输出
b
h
e
a
d
1
,
b
h
e
a
d
2
,
b
h
e
a
d
3
b_{head}^1, b_{head}^2,b_{head}^3
bhead1?,bhead2?,bhead3?,为了获得与
a
1
a_1
a1?对应的输出
b
1
b_1
b1?,在Multi-headed Self-attention中,我们会将这里得到的
b
h
e
a
d
1
,
b
h
e
a
d
2
,
b
h
e
a
d
3
b_{head}^1, b_{head}^2,b_{head}^3
bhead1?,bhead2?,bhead3?进行拼接(向量首尾相连),然后通过线性转换(即不含非线性激活层的单层全连接神经网络)得到
b
1
b_1
b1?。对于序列中的其他输入也是同样的处理过程,且它们共享这些网络的参数。
(三)Transformer代码详解
(1)、VIT 的 总的前向传播代码:
class VisionTransformer(nn.Module):
def __init__(self, config, img_size=224, num_classes=21843, zero_head=False, vis=False):
super(VisionTransformer, self).__init__()
self.num_classes = num_classes
self.zero_head = zero_head
self.classifier = config.classifier
self.transformer = Transformer(config, img_size, vis)
self.head = Linear(config.hidden_size, num_classes)
def forward(self, x, labels=None):
x, attn_weights = self.transformer(x)
print(x.shape)
logits = self.head(x[:, 0])
print(logits.shape)
if labels is not None:
loss_fct = CrossEntropyLoss()
loss = loss_fct(logits.view(-1, self.num_classes), labels.view(-1))
return loss
else:
return logits, attn_weights
如下会类Transformer代码中Embeddings和Encoder两个定义结合结果图讲解。
class Transformer(nn.Module):
def __init__(self, config, img_size, vis):
super(Transformer, self).__init__()
self.embeddings = Embeddings(config, img_size=img_size)
self.encoder = Encoder(config, vis)
def forward(self, input_ids):
embedding_output = self.embeddings(input_ids)
encoded, attn_weights = self.encoder(embedding_output)
return encoded, attn_weights
3.1、Transformer中Embeddings类的讲解
class Embeddings(nn.Module):
"""Construct the embeddings from patch, position embeddings.
"""
def __init__(self, config, img_size, in_channels=3):
super(Embeddings, self).__init__()
self.hybrid = None
img_size = _pair(img_size)
if config.patches.get("grid") is not None:
grid_size = config.patches["grid"]
patch_size = (img_size[0] // 16 // grid_size[0], img_size[1] // 16 // grid_size[1])
n_patches = (img_size[0] // 16) * (img_size[1] // 16)
self.hybrid = True
else:
patch_size = _pair(config.patches["size"])
n_patches = (img_size[0] // patch_size[0]) * (img_size[1] // patch_size[1])
self.hybrid = False
if self.hybrid:
self.hybrid_model = ResNetV2(block_units=config.resnet.num_layers,
width_factor=config.resnet.width_factor)
in_channels = self.hybrid_model.width * 16
self.patch_embeddings = Conv2d(in_channels=in_channels,
out_channels=config.hidden_size,
kernel_size=patch_size,
stride=patch_size)
self.position_embeddings = nn.Parameter(torch.zeros(1, n_patches+1, config.hidden_size))
self.cls_token = nn.Parameter(torch.zeros(1, 1, config.hidden_size))
self.dropout = Dropout(config.transformer["dropout_rate"])
def forward(self, x):
print(x.shape)
B = x.shape[0]
cls_tokens = self.cls_token.expand(B, -1, -1)
print(cls_tokens.shape)
if self.hybrid:
x = self.hybrid_model(x)
x = self.patch_embeddings(x)
print(x.shape)
x = x.flatten(2)
print(x.shape)
x = x.transpose(-1, -2)
print(x.shape)
x = torch.cat((cls_tokens, x), dim=1)
print(x.shape)
embeddings = x + self.position_embeddings
print(embeddings.shape)
embeddings = self.dropout(embeddings)
print(embeddings.shape)
return embeddings
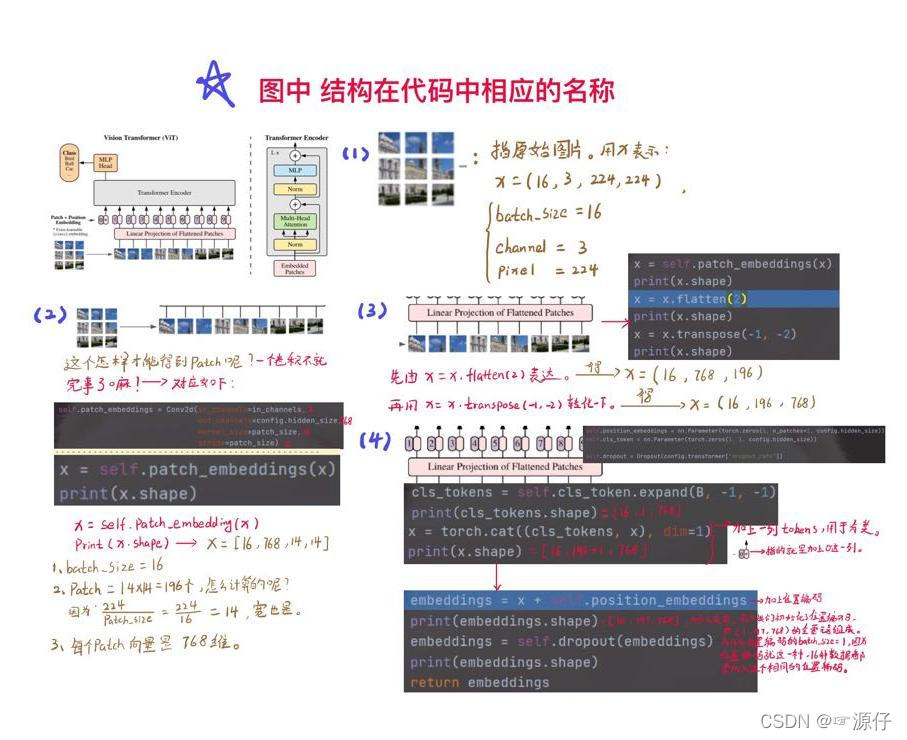
3.2、Transformer中Encoder类的讲解
class Encoder(nn.Module):
def __init__(self, config, vis):
super(Encoder, self).__init__()
self.vis = vis
self.layer = nn.ModuleList()
self.encoder_norm = LayerNorm(config.hidden_size, eps=1e-6)
for _ in range(config.transformer["num_layers"]):
layer = Block(config, vis)
self.layer.append(copy.deepcopy(layer))
def forward(self, hidden_states):
print(hidden_states.shape)
attn_weights = []
for layer_block in self.layer:
hidden_states, weights = layer_block(hidden_states)
if self.vis:
attn_weights.append(weights)
encoded = self.encoder_norm(hidden_states)
return encoded, attn_weights
- (1)以下for循环代码是指形成L个Transformer Encoder Block结构,如下图所示
# 定义了L个Block,如下图
for _ in range(config.transformer["num_layers"]):
layer = Block(config, vis)
self.layer.append(copy.deepcopy(layer))
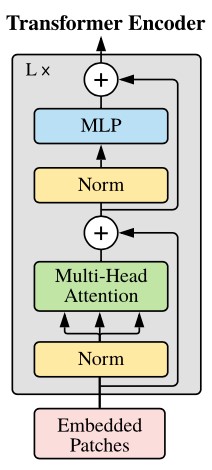
3.2.1、Encoder类中的Block类(拼图学习法)
- (2)查看Encoder类中的Block类:是如何定义的。
class Block(nn.Module):
def __init__(self, config, vis):
super(Block, self).__init__()
self.hidden_size = config.hidden_size
self.attention_norm = LayerNorm(config.hidden_size, eps=1e-6)
self.ffn_norm = LayerNorm(config.hidden_size, eps=1e-6)
self.ffn = Mlp(config)
self.attn = Attention(config, vis)
def forward(self, x):
print(x.shape)
h = x
x = self.attention_norm(x)
print(x.shape)
x, weights = self.attn(x)
x = x + h
print(x.shape)
h = x
x = self.ffn_norm(x)
print(x.shape)
x = self.ffn(x)
print(x.shape)
x = x + h
print(x.shape)
return x, weights
(1)、这一部分代码如下图所示:
h = x
x = self.attention_norm(x)
print(x.shape)
x, weights = self.attn(x)
x = x + h
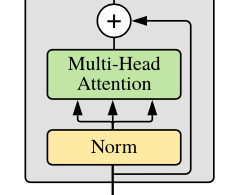 (2)、这一部分代码指的是如下图的结构:
h = x
x = self.ffn_norm(x)
print(x.shape)
x = self.ffn(x)
print(x.shape)
x = x + h
print(x.shape)
return x, weights
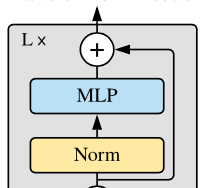
3.2.1.1、Encoder类中的Block类中的Attention类
指的是Block类中的x, weights = self.attn(x)这一行代码,这个attn就是Attention类,这个是重点奥。
class Attention(nn.Module):
def __init__(self, config, vis):
super(Attention, self).__init__()
self.vis = vis
self.num_attention_heads = config.transformer["num_heads"]
self.attention_head_size = int(config.hidden_size / self.num_attention_heads)
self.all_head_size = self.num_attention_heads * self.attention_head_size
self.query = Linear(config.hidden_size, self.all_head_size)
self.key = Linear(config.hidden_size, self.all_head_size)
self.value = Linear(config.hidden_size, self.all_head_size)
self.out = Linear(config.hidden_size, config.hidden_size)
self.attn_dropout = Dropout(config.transformer["attention_dropout_rate"])
self.proj_dropout = Dropout(config.transformer["attention_dropout_rate"])
self.softmax = Softmax(dim=-1)
def transpose_for_scores(self, x):
new_x_shape = x.size()[:-1] + (self.num_attention_heads, self.attention_head_size)
print(new_x_shape)
x = x.view(*new_x_shape)
print(x.shape)
print(x.permute(0, 2, 1, 3).shape)
return x.permute(0, 2, 1, 3)
def forward(self, hidden_states):
print(hidden_states.shape)
mixed_query_layer = self.query(hidden_states)
print(mixed_query_layer.shape)
mixed_key_layer = self.key(hidden_states)
print(mixed_key_layer.shape)
mixed_value_layer = self.value(hidden_states)
print(mixed_value_layer.shape)
query_layer = self.transpose_for_scores(mixed_query_layer)
print(query_layer.shape)
key_layer = self.transpose_for_scores(mixed_key_layer)
print(key_layer.shape)
value_layer = self.transpose_for_scores(mixed_value_layer)
print(value_layer.shape)
attention_scores = torch.matmul(query_layer, key_layer.transpose(-1, -2))
print(attention_scores.shape)
attention_scores = attention_scores / math.sqrt(self.attention_head_size)
print(attention_scores.shape)
attention_probs = self.softmax(attention_scores)
print(attention_probs.shape)
weights = attention_probs if self.vis else None
attention_probs = self.attn_dropout(attention_probs)
print(attention_probs.shape)
context_layer = torch.matmul(attention_probs, value_layer)
print(context_layer.shape)
context_layer = context_layer.permute(0, 2, 1, 3).contiguous()
print(context_layer.shape)
new_context_layer_shape = context_layer.size()[:-2] + (self.all_head_size,)
context_layer = context_layer.view(*new_context_layer_shape)
print(context_layer.shape)
attention_output = self.out(context_layer)
print(attention_output.shape)
attention_output = self.proj_dropout(attention_output)
print(attention_output.shape)
return attention_output, weights
3.2.1.1.1、self.transpose_for_scores()
把query、key、value转化为多头注意力的size。
size():函数介绍,不会的简单看一下,浅显易懂。 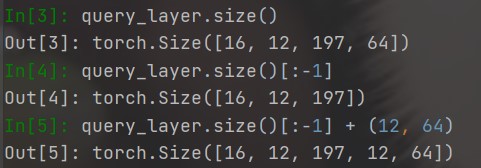
def transpose_for_scores(self, x):
new_x_shape = x.size()[:-1] + (self.num_attention_heads, self.attention_head_size)
print(new_x_shape)
x = x.view(*new_x_shape)
print(x.shape)
print(x.permute(0, 2, 1, 3).shape)
return x.permute(0, 2, 1, 3)
我们记得原始的query是 ([16,197,768]) 的,现在为啥转化为了 ([16, 197, 12, 64]) ,这个12指的是num_attention_heads = 12(多头注意力机制),attention_head_size = 64(注意num_attention_heads的设置一定要被tokens的维度整除,这里tokens的维度维768)。由于在第一节我们详细的讲述了self-attention,所以下面我们看一下多头注意力机制的图片就懂了。每个attention_heads都是单独训练的,就和12个人鸣人会产生12种战斗想法一样,他们是相互独立的。 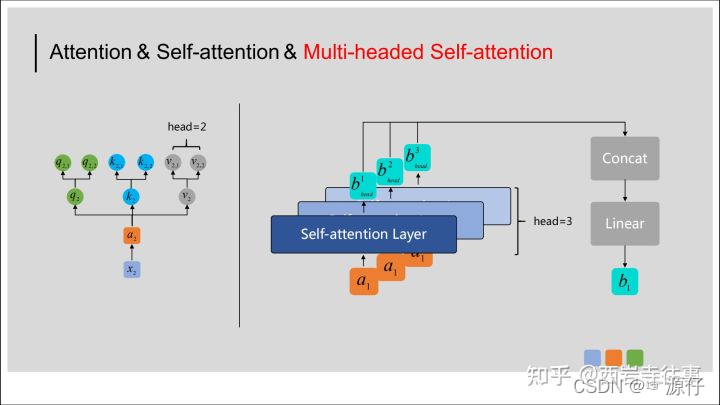
3.2.2、VIT代码总的前向传播
我们在3.2.1章节中已经详细的讲解了x, attn_weights = self.transformer(x)这一行代码debug的详细过程,那么现在我们再来看VIT总的代码的前向传播就不难理解了。下面我们主要讲解logits = self.head(x[:, 0])这一行代码的作用。 如下图的红色方框部分所示。代那么到此为止,这张图形的所有部分我们都已经用代码按循序凭借完成。所以VIT的主要model代码到此为止,相信大家也完全弄懂了VIT。 (1)、VIT 的 总的前向传播代码:
class VisionTransformer(nn.Module):
def __init__(self, config, img_size=224, num_classes=21843, zero_head=False, vis=False):
super(VisionTransformer, self).__init__()
self.num_classes = num_classes
self.zero_head = zero_head
self.classifier = config.classifier
self.transformer = Transformer(config, img_size, vis)
self.head = Linear(config.hidden_size, num_classes)
def forward(self, x, labels=None):
x, attn_weights = self.transformer(x)
print(x.shape)
logits = self.head(x[:, 0])
print(logits.shape)
if labels is not None:
loss_fct = CrossEntropyLoss()
loss = loss_fct(logits.view(-1, self.num_classes), labels.view(-1))
return loss
else:
return logits, attn_weights
|