今天第二天接触PINN,用深度学习的方法求解偏微分方程PDE,看来是非常不错的方法。做了一个简单易懂的例子,这个例子非常适合初学者。跟着教程做了一个小demo, 大家可以参考参考。本文代码亲测可用,直接复制就能使用,非常方便。
所用工具
使用了python和pytorch进行实现 python3.6 toch1.10
数学方程
使用一个最简单的偏微分方程:
{
u
t
+
u
×
u
x
?
w
×
u
x
x
=
0
(
1
)
u
(
0
,
x
)
=
?
s
i
n
(
π
x
)
(
2
)
u
(
t
,
1
)
=
0
(
3
)
u
(
t
,
?
1
)
=
0
(
4
)
w
=
0.01
π
,
x
∈
(
?
1
,
1
)
,
t
∈
(
0
,
1
)
\left\{ \begin{aligned} & u_t+u\times u_x -w\times u_{xx} = 0 \hspace{1cm} & (1)\\ & u(0,x) = -sin(\pi x) &(2)\\ & u(t,\hspace{0.28cm}1)=0 & (3)\\ & u(t,-1)=0 & (4) \\ & w= \dfrac{0.01}{\pi} , x \in (-1, 1) , t \in (0,1) & \\ \end{aligned} \right.
?????????????????????ut?+u×ux??w×uxx?=0u(0,x)=?sin(πx)u(t,1)=0u(t,?1)=0w=π0.01?,x∈(?1,1),t∈(0,1)?(1)(2)(3)(4)?
要拟合的显示方程可以表示为
u
=
u
(
t
,
x
)
u=u(t,x)
u=u(t,x)
模型搭建
核心-使用最简单的全连接层:
class Net(nn.Module):
def __init__(self, NN):
super(Net, self).__init__()
self.input_layer = nn.Linear(2, NN)
self.hidden_layer1 = nn.Linear(NN,int(NN/2))
self.hidden_layer2 = nn.Linear(int(NN/2), 5)
self.output_layer = nn.Linear(5, 1)
def forward(self, x):
out = torch.tanh(self.input_layer(x))
out = torch.tanh(self.hidden_layer1(out))
out = torch.tanh(self.hidden_layer2(out))
out_final = self.output_layer(out)
return out_final
偏微分方程定义,也就是公式(1):
def pde(x, net):
u = net(x)
u_tx = torch.autograd.grad(u, x, grad_outputs=torch.ones_like(net(x)),
create_graph=True, allow_unused=True)[0]
d_t = u_tx[:, 0].unsqueeze(-1)
d_x = u_tx[:, 1].unsqueeze(-1)
u_xx = torch.autograd.grad(d_x, x, grad_outputs=torch.ones_like(d_x),
create_graph=True, allow_unused=True)[0][:,1].unsqueeze(-1)
w = torch.tensor(0.01 / np.pi)
return d_t + u * d_x - w * u_xx
所有实现代码
一下代码复制粘贴,可直接运行:
import torch
import torch.nn as nn
import torch
import torch.nn as nn
import numpy as np
import matplotlib.pyplot as plt
import torch.optim as optim
from torch.autograd import Variable
import numpy as np
from matplotlib import cm
class Net(nn.Module):
def __init__(self, NN):
super(Net, self).__init__()
self.input_layer = nn.Linear(2, NN)
self.hidden_layer1 = nn.Linear(NN,int(NN/2))
self.hidden_layer2 = nn.Linear(int(NN/2), 5)
self.output_layer = nn.Linear(5, 1)
def forward(self, x):
out = torch.tanh(self.input_layer(x))
out = torch.tanh(self.hidden_layer1(out))
out = torch.tanh(self.hidden_layer2(out))
out_final = self.output_layer(out)
return out_final
def pde(x, net):
u = net(x)
u_tx = torch.autograd.grad(u, x, grad_outputs=torch.ones_like(net(x)),
create_graph=True, allow_unused=True)[0]
d_t = u_tx[:, 0].unsqueeze(-1)
d_x = u_tx[:, 1].unsqueeze(-1)
u_xx = torch.autograd.grad(d_x, x, grad_outputs=torch.ones_like(d_x),
create_graph=True, allow_unused=True)[0][:,1].unsqueeze(-1)
w = torch.tensor(0.01 / np.pi)
return d_t + u * d_x - w * u_xx
net = Net(30)
mse_cost_function = torch.nn.MSELoss(reduction='mean')
optimizer = torch.optim.Adam(net.parameters(), lr=1e-4)
t_bc_zeros = np.zeros((2000, 1))
x_in_pos_one = np.ones((2000, 1))
x_in_neg_one = -np.ones((2000, 1))
u_in_zeros = np.zeros((2000, 1))
iterations = 10000
for epoch in range(iterations):
optimizer.zero_grad()
t_in_var = np.random.uniform(low=0, high=1.0, size=(2000, 1))
x_bc_var = np.random.uniform(low=-1.0, high=1.0, size=(2000, 1))
u_bc_sin = -np.sin(np.pi * x_bc_var)
pt_x_bc_var = Variable(torch.from_numpy(x_bc_var).float(), requires_grad=False)
pt_t_bc_zeros = Variable(torch.from_numpy(t_bc_zeros).float(), requires_grad=False)
pt_u_bc_sin = Variable(torch.from_numpy(u_bc_sin).float(), requires_grad=False)
pt_x_in_pos_one = Variable(torch.from_numpy(x_in_pos_one).float(), requires_grad=False)
pt_x_in_neg_one = Variable(torch.from_numpy(x_in_neg_one).float(), requires_grad=False)
pt_t_in_var = Variable(torch.from_numpy(t_in_var).float(), requires_grad=False)
pt_u_in_zeros = Variable(torch.from_numpy(u_in_zeros).float(), requires_grad=False)
net_bc_out = net(torch.cat([pt_t_bc_zeros, pt_x_bc_var], 1))
mse_u_2 = mse_cost_function(net_bc_out, pt_u_bc_sin)
net_bc_inr = net(torch.cat([pt_t_in_var, pt_x_in_pos_one], 1))
net_bc_inl = net(torch.cat([pt_t_in_var, pt_x_in_neg_one], 1))
mse_u_3 = mse_cost_function(net_bc_inr, pt_u_in_zeros)
mse_u_4 = mse_cost_function(net_bc_inl, pt_u_in_zeros)
x_collocation = np.random.uniform(low=-1.0, high=1.0, size=(2000, 1))
t_collocation = np.random.uniform(low=0.0, high=1.0, size=(2000, 1))
all_zeros = np.zeros((2000, 1))
pt_x_collocation = Variable(torch.from_numpy(x_collocation).float(), requires_grad=True)
pt_t_collocation = Variable(torch.from_numpy(t_collocation).float(), requires_grad=True)
pt_all_zeros = Variable(torch.from_numpy(all_zeros).float(), requires_grad=False)
f_out = pde(torch.cat([pt_t_collocation, pt_x_collocation], 1), net)
mse_f_1 = mse_cost_function(f_out, pt_all_zeros)
loss = mse_f_1 + mse_u_2 + mse_u_3 + mse_u_4
loss.backward()
optimizer.step()
with torch.autograd.no_grad():
if epoch % 100 == 0:
print(epoch, "Traning Loss:", loss.data)
t = np.linspace(0, 1, 100)
x = np.linspace(-1, 1, 256)
ms_t, ms_x = np.meshgrid(t, x)
x = np.ravel(ms_x).reshape(-1, 1)
t = np.ravel(ms_t).reshape(-1, 1)
pt_x = Variable(torch.from_numpy(x).float(), requires_grad=True)
pt_t = Variable(torch.from_numpy(t).float(), requires_grad=True)
pt_u0 = net(torch.cat([pt_t, pt_x], 1))
u = pt_u0.data.cpu().numpy()
pt_u0 = u.reshape(256, 100)
fig = plt.figure()
ax = fig.add_subplot(projection='3d')
ax.set_zlim([-1, 1])
ax.plot_surface(ms_t, ms_x, pt_u0, cmap=cm.RdYlBu_r, edgecolor='blue', linewidth=0.0003, antialiased=True)
ax.set_xlabel('t')
ax.set_ylabel('x')
ax.set_zlabel('u')
plt.savefig('Preddata.png')
plt.close(fig)
结果展示
训练0次时的结果也就是没训练,下面为模拟值: 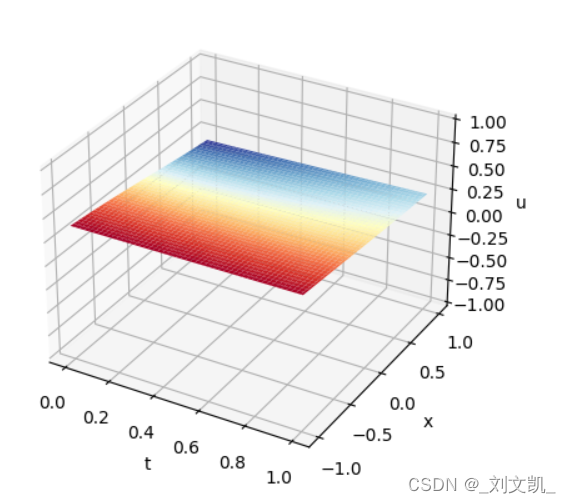
训练10000时的结果: 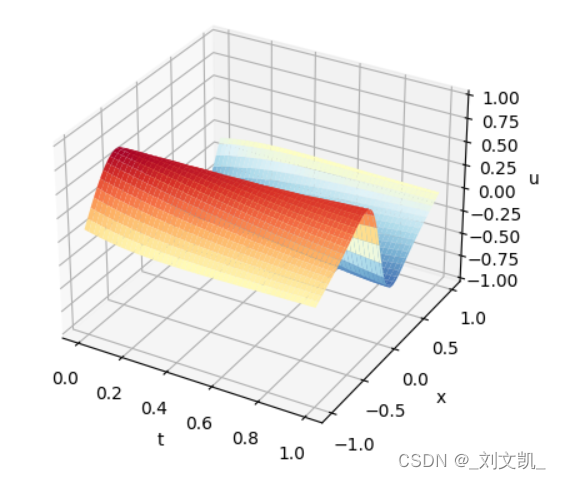
参考文献
[1]. 每天进步一点点吧. PINN学习记录. https://blog.csdn.net/weixin_45805559/article/details/121574293
|