读研太忙了才发现一年没更新,就以当初学习机器学习的一个简单例子作为一个新的开始吧。以下为正文。
一、安装sklearn库
执行以下命令安装,?注意:在此之前需要提前安装numpy、matplotlib、scipy库
pip install scikit-learn
如果速度较慢可更换国内镜像源,例如清华镜像源
pip config set global.index-url https://pypi.tuna.tsinghua.edu.cn/simple
二、实例代码
1、引入所需模块
from sklearn.datasets import load_iris
from sklearn.model_selection import train_test_split, GridSearchCV
from sklearn.preprocessing import StandardScaler
from sklearn.neighbors import KNeighborsClassifier
2、读入数据
x_data = load_iris().data # 特征值
y_data = load_iris().target # 目标值
x_data代表每个样本有4个特征值,对应[花萼长],[花萼宽],[花瓣长],[花瓣宽]。
?y_data代表每个样本对应的目标值,共有3个类别。
3、划分数据集
x_train, x_test, y_train, y_test = train_test_split(
x_data, y_data, test_size=0.2, random_state=10)
按照测试集占总样本数20%的比例划分训练集和测试集
4、特征标准化
scaler = StandardScaler()
x_train = scaler.fit_transform(x_train)
x_test = scaler.transform(x_test)
5、训练KNN模型
estimator = KNeighborsClassifier(n_neighbors=5) # 构建KNN模型
estimator.fit(x_train, y_train) # 训练模型
对于每个样本来说,取最邻近的5个样本作为当前样本分类的划分依据
6、模型评估
y_pre = estimator.predict(x_test) # 预测值
score = estimator.score(x_test, y_test) # 准确率
print("实际结果为:\n", y_test)
print("预测结果为:\n", y_pre)
print("对比结果为:\n", y_pre == y_test)
print("准确率为:\n", score)
运行结果为:
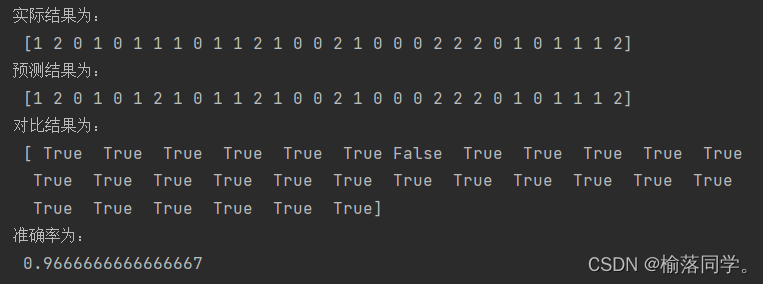
|