机器学习对分类与回归评分指标不同 在sklearn中评分函数score相当于calcError(评价模型的类函数),fit(拟合函数)相当于train(训练函数)
常见的评分指标都在sklearn.metrics里
分类指标
准确率
from sklearn import metrics
metrics.accuracy_score(y_true, y_pred)
精确率
from sklearn import metrics
metrics.precision_score(y_true, y_pred)
召回率
from sklearn import metrics
metrics.recall_score(y_true, y_pred)
回归指标
均方误差(mse)
from sklearn import metrics
metrics.mean_squared_error(y_true, y_pred)
可释误差
from sklearn import metrics
metrics.explained_variance_score(y_true, y_pred)
R
2
R^{2}
R2
from sklearn import metrics
metrics.r2_score(y_true, y_pred)
R
2
R^{2}
R2越接近1,预测值越接近实际值
分类模型
利用opencv分类模型预测类别,以KNN算法为例
from turtle import color
import cv2
from matplotlib import markers
import numpy as np
import matplotlib.pyplot as plt
from matplotlib.patches import Circle
plt.rcParams['font.family'] = ['sans-serif']
plt.rcParams['font.sans-serif'] = ['SimHei']
plt.style.use("ggplot")
np.random.seed(42)
"""
num_samples:代表数据样本个数,表示行
num_features=2:代表数据特征个数,表示列
返回一个行列表示的数组,每个数据样本的标签
"""
def generate_data(num_samples, num_features=2):
data_size = (num_samples, num_features)
data = np.random.randint(0, 100, size=data_size)
label_size = (num_samples, 1)
labels = np.random.randint(0, 2, size=label_size)
return data.astype(np.float32), labels
train_data, labels = generate_data(11)
newcomer, _ = generate_data(1)
def plot_data(all_biue, all_red):
fig =plt.figure("数据图")
ax = fig.add_subplot(111)
plt.scatter(all_biue[:, 0], all_biue[:, 1], c="b", marker="s", s=180)
plt.scatter(all_red[:, 0], all_red[:, 1], c="r", marker="^", s=180)
plt.plot(newcomer[0, 0], newcomer[0, 1], "go", markersize=14)
plt.arrow(newcomer[0, 0], newcomer[0, 1], 60-newcomer[0, 0], 20-newcomer[0, 1],
head_width=0.2, color="red", lw=2,length_includes_head = True)
circle = Circle(xy=(newcomer[0, 0], newcomer[0, 1]), radius=20, alpha=0.1, color='b')
ax.add_patch(circle)
circle = plt.Circle((newcomer[0, 0], newcomer[0, 1]), 20, color='b', fill=False)
plt.gcf().gca().add_artist(circle)
plt.text(60, 30, s="455", fontsize = 18, color = 'red')
plt.xlabel("x 坐标 (特征1)")
plt.ylabel("y 坐标 (特征2)")
plt.show()
blue = train_data[labels.ravel()==0]
red = train_data[labels.ravel()==1]
plot_data(blue, red)
knn = cv2.ml.KNearest_create()
knn.train(train_data,cv2.ml.ROW_SAMPLE,labels)
ret, results, neighbor, dist = knn.findNearest(newcomer, 1)
print("Predicted label:\t", results)
print("Neighbor's label:\t", neighbor)
print("Distance to neighbor:\t", dist)
输出结果
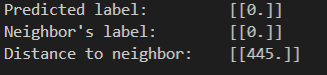

回归模型
利用opencv回归模型预测值,以线性回归算法为例
import numpy as np
from sklearn import datasets
from sklearn import metrics
from sklearn import model_selection as modsel
from sklearn import linear_model
import matplotlib.pyplot as plt
plt.rcParams['font.family'] = ['sans-serif']
plt.rcParams['font.sans-serif'] = ['SimHei']
plt.rcParams['axes.unicode_minus']=False
plt.style.use("ggplot")
"""
booton.data:(506,13)
booton.target:(506,)
"""
booton = datasets.load_boston()
x_train, x_test, y_train, y_test = modsel.train_test_split(
booton.data, booton.target, test_size=0.1, random_state=42)
linreg = linear_model.LinearRegression()
linreg.fit(x_train, y_train)
train_mse = metrics.mean_squared_error(y_train, linreg.predict(x_train))
train_r2 = linreg.score(x_train, y_train)
y_pred = linreg.predict(x_test)
test_mse = metrics.mean_squared_error(y_test, y_pred)
test_r2 = linreg.score(x_test, y_test)
plt.figure("误差可视化", figsize=(13,6))
ax = plt.subplot(121)
ax.set_title("测试值与预测值可视化")
plt.plot(y_test, linewidth=3, label="真实值")
plt.plot(y_pred, linewidth=3, label="预测值")
plt.legend(loc="best")
plt.xlabel("测试数据点")
plt.ylabel("目标值")
ax = plt.subplot(122)
ax.set_title("R方值可视化")
plt.plot(y_test, y_pred, "o")
plt.plot([-10,60], [-10,60], "k--")
plt.axis([-10,60,-10,60])
plt.xlabel("真实值")
plt.ylabel("预测值")
scorestr = r"R$^2$ = %.3f" % test_r2
errstr = "MSE = %.3f" % test_mse
plt.text(-5, 50, scorestr, fontsize=12)
plt.text(-5, 45, errstr, fontsize=12)
plt.show()
输出结果
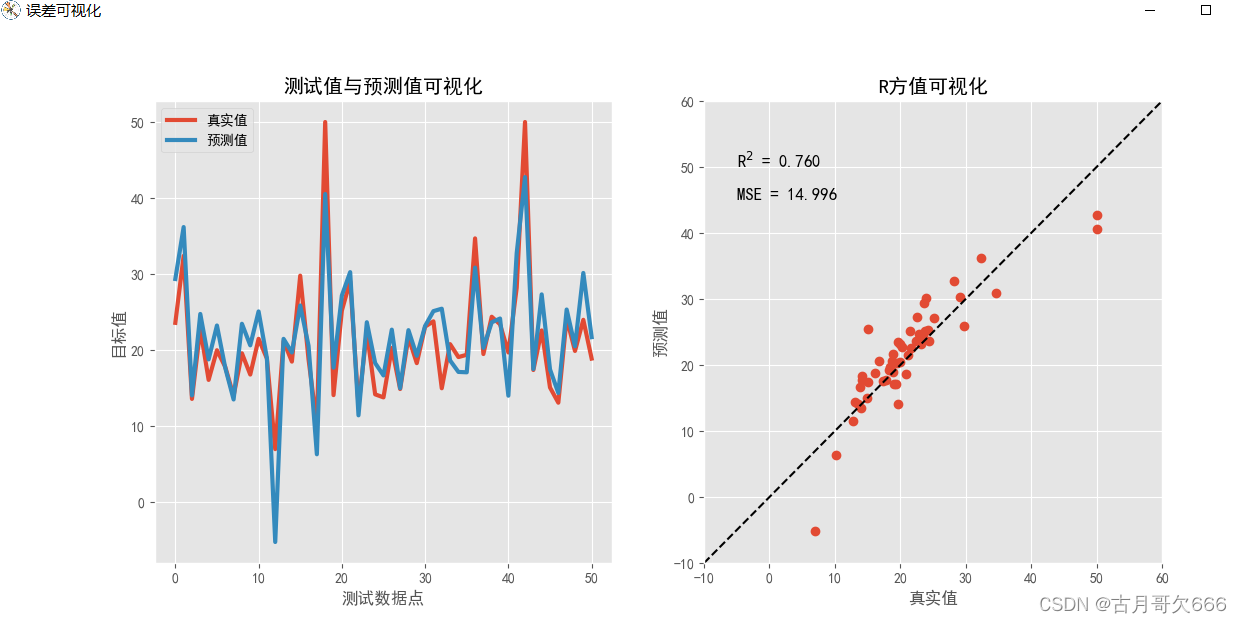 从上图可以看出二图通过描述离散数据情况,与对角线存在偏差表明模型的预测出现了一些误差或者是数据中有一些方差是模型无法解释的,
R
2
R^{2}
R2表明我们可以解释数据76%的离散程度,其中均方误差为14.996.
|