官网API
一、线性回归
针对的是损失函数loss faction
Ⅰ、Lasso Regression
采用L1正则,会使得w值整体偏小;w会变小从而达到降维的目的 
import numpy as np
from sklearn.linear_model import Lasso
from sklearn.linear_model import SGDRegressor
X = 2 * np.random.rand(100, 1)
y = 4 + 3 * X + np.random.randn(100, 1)
lasso_reg = Lasso(alpha=0.15, max_iter=10000)
lasso_reg.fit(X, y)
print("w0=",lasso_reg.intercept_)
print("w1=",lasso_reg.coef_)
sgd_reg = SGDRegressor(penalty='l1', max_iter=10000)
sgd_reg.fit(X, y.ravel())
print("w0=",sgd_reg.intercept_)
print("w1=",lasso_reg.coef_)
Ⅱ、Ridge Regression(岭回归)
采用L2正则,会使得有的w趋近1,有的w趋近0;当w趋近于0的时候,相对于可以忽略,w会变少也可以达到降维的目的(深度学习模型建立首选 ) 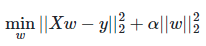
import numpy as np
from sklearn.linear_model import Ridge
from sklearn.linear_model import SGDRegressor
X = 2 * np.random.rand(100,1)
y = 4 + 3 * X + np.random.randn(100,1)
ridge_reg = Ridge(alpha=1,solver='auto')
ridge_reg.fit(X,y)
print("w0=",ridge_reg.intercept_)
print("w1=",ridge_reg.coef_)
sgd_reg = SGDRegressor(penalty='l2')
sgd_reg.fit(X,y.ravel())
print("w0=",sgd_reg.intercept_)
print("w1=",sgd_reg.coef_)
Ⅲ、Elastic Net回归
当你不清楚使用L1正则化还是L2正则化的时候,可以采用Elastic Net 
import numpy as np
from sklearn.linear_model import ElasticNet
from sklearn.linear_model import SGDRegressor
X = 2 * np.random.rand(100, 1)
y = 4 + 3 * X + np.random.randn(100, 1)
elastic_net = ElasticNet(alpha=0.0001, l1_ratio=0.15)
elastic_net.fit(X, y)
print("w0=",elastic_net.intercept_)
print("w1=",elastic_net.coef_)
sgd_reg = SGDRegressor(penalty='elasticnet', max_iter=1000)
sgd_reg.fit(X, y.ravel())
print("w0=",sgd_reg.intercept_)
print("w1=",sgd_reg.coef_)
Ⅳ、总结
①算法选择顺序,Ridge Regression (L2正则化) --> ElasticNet (即包含L1又包含L2) --> Lasso Regression (L1正则化)
②正则化L1和L2有什么区别?
答:L1是w绝对值加和,L2是w平方加和。L1的有趣的现象是会使得w有的接近于0,有的接近于1, L1更多的会用在降维上面,因为有的是0有的是1,我们也称之为稀疏编码。 L2是更常用的正则化手段,它会使得w整体变小
超参数alpha 在Rideg类里面就直接是L2正则的权重 超参数alpha 在Lasso类里面就直接是L1正则的权重 超参数alpha 在ElasticNet和SGDRegressor里面是损失函数里面的alpha 超参数l1_ration 在ElasticNet和SGDRegressor里面是损失函数的p
二、多项式回归
针对的是数据预处理进行特征处理,跟归一化一样,都是针对的是数据 多项式回归其实是线性回归的一种拓展。 多项式回归:叫回归但并不是去做拟合的算法 PolynomialFeatures是来做预处理的,来转换我们的数据,把数据进行升维!
import numpy as np
import matplotlib.pyplot as plt
from sklearn.preprocessing import PolynomialFeatures
from sklearn.linear_model import LinearRegression
m = 100
X = 6 * np.random.rand(m, 1) - 3
y = 0.5 * X ** 2 + X + 2 + np.random.randn(m, 1)
plt.plot(X, y, 'b.')
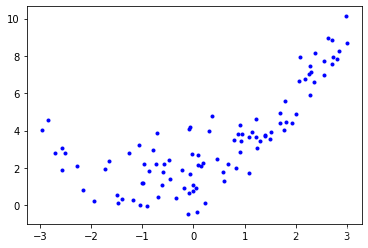
d = {1: 'g-', 2: 'r+', 10: 'y*'}
for i in d:
poly_features = PolynomialFeatures(degree=i, include_bias=False)
X_poly = poly_features.fit_transform(X)
print(X[0])
print(X_poly[0])
print(X_poly[:, 0])
lin_reg = LinearRegression(fit_intercept=True)
lin_reg.fit(X_poly, y)
print(lin_reg.intercept_, lin_reg.coef_)
y_predict = lin_reg.predict(X_poly)
plt.plot(X_poly[:, 0], y_predict, d[i])
plt.show()
"""
[-1.17608282]
[-1.17608282]
[-1.17608282 0.84008756 -0.47456115 1.09332609 -0.08048591 2.56227793
-2.16089094 2.9934595 -0.97719578 -0.35093548 -0.01833382 -2.57809549
0.90594767 1.23236141 0.5809494 -1.03761487 1.07926559 -1.01536504
2.08713351 1.68679419 0.36108667 0.0739686 -0.60676473 -0.0977825
0.93322126 -0.98029008 1.80329174 -2.7079627 2.27067075 -0.23098381
-2.84414673 2.80368239 1.13965085 0.60386564 1.5068452 0.08483579
2.54605719 2.25506764 -0.57412233 1.40321778 0.08664762 1.79293147
-0.72311264 -1.39573162 0.15066435 -2.56825076 1.6992054 0.30655144
2.27792527 0.05690445 1.91725839 2.70744724 -0.46459041 1.24513038
-0.90932212 2.71793477 -1.64319111 1.49955188 2.17534115 -2.50510391
2.72835224 -0.17797949 -0.07305404 -0.60531858 0.90754969 0.186454
2.63700818 2.00439925 -1.26906332 -0.03326623 0.95249887 2.98801031
1.39131364 -1.46984234 0.67347918 1.30899516 -0.68746311 -0.07895217
2.847029 -1.94670177 -0.73970148 -1.05884194 -2.95987324 -2.27319748
-0.01555128 -0.86999284 0.45600536 1.21528784 -1.72581767 0.22440468
-1.50353748 2.36782931 2.30633509 1.76346603 -0.79567338 -0.06153651
0.87272525 0.78535366 2.36179793 2.05667417]
[2.99104579] [[1.19669575]]
[-1.17608282]
[-1.17608282 1.38317079]
[-1.17608282 0.84008756 -0.47456115 1.09332609 -0.08048591 2.56227793
-2.16089094 2.9934595 -0.97719578 -0.35093548 -0.01833382 -2.57809549
0.90594767 1.23236141 0.5809494 -1.03761487 1.07926559 -1.01536504
2.08713351 1.68679419 0.36108667 0.0739686 -0.60676473 -0.0977825
0.93322126 -0.98029008 1.80329174 -2.7079627 2.27067075 -0.23098381
-2.84414673 2.80368239 1.13965085 0.60386564 1.5068452 0.08483579
2.54605719 2.25506764 -0.57412233 1.40321778 0.08664762 1.79293147
-0.72311264 -1.39573162 0.15066435 -2.56825076 1.6992054 0.30655144
2.27792527 0.05690445 1.91725839 2.70744724 -0.46459041 1.24513038
-0.90932212 2.71793477 -1.64319111 1.49955188 2.17534115 -2.50510391
2.72835224 -0.17797949 -0.07305404 -0.60531858 0.90754969 0.186454
2.63700818 2.00439925 -1.26906332 -0.03326623 0.95249887 2.98801031
1.39131364 -1.46984234 0.67347918 1.30899516 -0.68746311 -0.07895217
2.847029 -1.94670177 -0.73970148 -1.05884194 -2.95987324 -2.27319748
-0.01555128 -0.86999284 0.45600536 1.21528784 -1.72581767 0.22440468
-1.50353748 2.36782931 2.30633509 1.76346603 -0.79567338 -0.06153651
0.87272525 0.78535366 2.36179793 2.05667417]
[1.77398327] [[0.96156952 0.516698 ]]
[-1.17608282]
[-1.17608282 1.38317079 -1.6267234 1.91316143 -2.25003628 2.64622901
-3.11218446 3.66018667 -4.30468264 5.06266328]
[-1.17608282 0.84008756 -0.47456115 1.09332609 -0.08048591 2.56227793
-2.16089094 2.9934595 -0.97719578 -0.35093548 -0.01833382 -2.57809549
0.90594767 1.23236141 0.5809494 -1.03761487 1.07926559 -1.01536504
2.08713351 1.68679419 0.36108667 0.0739686 -0.60676473 -0.0977825
0.93322126 -0.98029008 1.80329174 -2.7079627 2.27067075 -0.23098381
-2.84414673 2.80368239 1.13965085 0.60386564 1.5068452 0.08483579
2.54605719 2.25506764 -0.57412233 1.40321778 0.08664762 1.79293147
-0.72311264 -1.39573162 0.15066435 -2.56825076 1.6992054 0.30655144
2.27792527 0.05690445 1.91725839 2.70744724 -0.46459041 1.24513038
-0.90932212 2.71793477 -1.64319111 1.49955188 2.17534115 -2.50510391
2.72835224 -0.17797949 -0.07305404 -0.60531858 0.90754969 0.186454
2.63700818 2.00439925 -1.26906332 -0.03326623 0.95249887 2.98801031
1.39131364 -1.46984234 0.67347918 1.30899516 -0.68746311 -0.07895217
2.847029 -1.94670177 -0.73970148 -1.05884194 -2.95987324 -2.27319748
-0.01555128 -0.86999284 0.45600536 1.21528784 -1.72581767 0.22440468
-1.50353748 2.36782931 2.30633509 1.76346603 -0.79567338 -0.06153651
0.87272525 0.78535366 2.36179793 2.05667417]
[1.75155522] [[ 0.96659081 1.47322289 -0.32379381 -1.09309828 0.22053653 0.3517641
-0.03986542 -0.04423885 0.00212836 0.00193739]]
"""
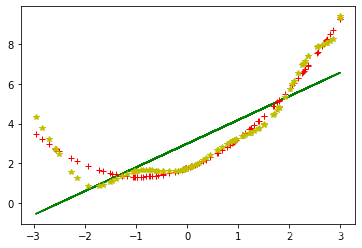
完整代码如下:
import numpy as np
import matplotlib.pyplot as plt
from sklearn.preprocessing import PolynomialFeatures
from sklearn.linear_model import LinearRegression
m = 100
X = 6 * np.random.rand(m, 1) - 3
X = 6 * np.random.rand(m, 1) - 3
y = 0.5 * X ** 2 + X + 2 + np.random.randn(m, 1)
plt.plot(X, y, 'b.')
y = 0.5 * X ** 2 + X + 2 + np.random.randn(m, 1)
plt.plot(X, y, 'b.')
d = {1: 'g-', 2: 'r+', 10: 'y*'}
for i in d:
poly_features = PolynomialFeatures(degree=i, include_bias=False)
X_poly = poly_features.fit_transform(X)
print(X[0])
print(X_poly[0])
print(X_poly[:, 0])
lin_reg = LinearRegression(fit_intercept=True)
lin_reg.fit(X_poly, y)
print(lin_reg.intercept_, lin_reg.coef_)
y_predict = lin_reg.predict(X_poly)
plt.plot(X_poly[:, 0], y_predict, d[i])
plt.show()
三、案例实战
保险公司的一份数据集,里面含有多人的age、sex、bmi、children、smoker、region、charges。 年龄、性别、BMI肥胖指数、有几个孩子、是否吸烟、居住区域、医疗开销。 很显然,这是个有监督的学习,保险公司肯定是想通过其他因素来确定处某个人的医疗开销,来一个人,我可以通过他的年龄、性别、BMI肥胖指数、有几个孩子、是否吸烟、居住区域来推测出这个人的医疗开销。 故,这里的y为charges,各因素为age、sex、bmi、children、smoker、region。 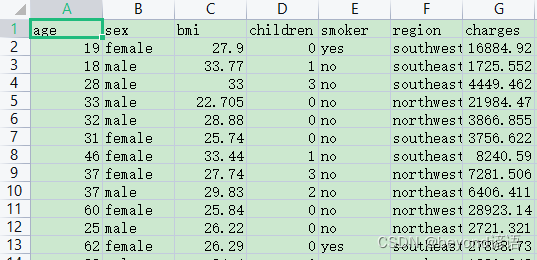
免费保险公司用户信息数据集下载,这里采用的是CSV文件,也就是数据之间通过逗号隔开的数据集。 逗号分隔值(Comma-Separated Values,CSV,有时也称为字符分隔值,因为分隔字符也可以不是逗号)
import pandas as pd
import matplotlib.pyplot as plt
from sklearn.preprocessing import PolynomialFeatures
from sklearn.linear_model import LinearRegression
data = pd.read_csv('./insurance.csv')
print(type(data))
print(data.head())
print(data.tail())
print(data.describe())
"""
<class 'pandas.core.frame.DataFrame'>
age sex bmi children smoker region charges
0 19 female 27.900 0 yes southwest 16884.92400
1 18 male 33.770 1 no southeast 1725.55230
2 28 male 33.000 3 no southeast 4449.46200
3 33 male 22.705 0 no northwest 21984.47061
4 32 male 28.880 0 no northwest 3866.85520
age sex bmi children smoker region charges
1333 50 male 30.97 3 no northwest 10600.5483
1334 18 female 31.92 0 no northeast 2205.9808
1335 18 female 36.85 0 no southeast 1629.8335
1336 21 female 25.80 0 no southwest 2007.9450
1337 61 female 29.07 0 yes northwest 29141.3603
age bmi children charges
count 1338.000000 1338.000000 1338.000000 1338.000000
mean 39.207025 30.663397 1.094918 13270.422265
std 14.049960 6.098187 1.205493 12110.011237
min 18.000000 15.960000 0.000000 1121.873900
25% 27.000000 26.296250 0.000000 4740.287150
50% 39.000000 30.400000 1.000000 9382.033000
75% 51.000000 34.693750 2.000000 16639.912515
max 64.000000 53.130000 5.000000 63770.428010
"""
data_count = data['age'].value_counts()
print(data_count)
data_count[:10].plot(kind='bar')
plt.show()
"""
18 69
19 68
51 29
45 29
46 29
47 29
48 29
50 29
52 29
20 29
26 28
54 28
53 28
25 28
24 28
49 28
23 28
22 28
21 28
27 28
28 28
31 27
29 27
30 27
41 27
43 27
44 27
40 27
42 27
57 26
34 26
33 26
32 26
56 26
55 26
59 25
58 25
39 25
38 25
35 25
36 25
37 25
63 23
60 23
61 23
62 23
64 22
Name: age, dtype: int64
"""
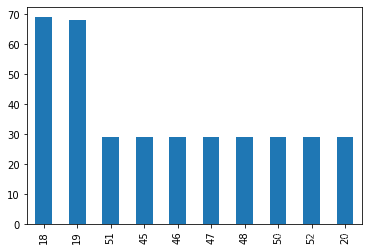
print(data.corr())
"""
age bmi children charges
age 1.000000 0.109272 0.042469 0.299008
bmi 0.109272 1.000000 0.012759 0.198341
children 0.042469 0.012759 1.000000 0.067998
charges 0.299008 0.198341 0.067998 1.000000
"""
reg = LinearRegression()
x = data[['age', 'sex', 'bmi', 'children', 'smoker', 'region']]
y = data['charges']
x = x.apply(pd.to_numeric, errors='coerce')
y = y.apply(pd.to_numeric, errors='coerce')
x.fillna(0, inplace=True)
y.fillna(0, inplace=True)
poly_features = PolynomialFeatures(degree=3, include_bias=False)
X_poly = poly_features.fit_transform(x)
reg.fit(X_poly, y)
print("w0=",reg.intercept_)
print("w1=",reg.coef_)
"""
w0= 14959.173791946276
w1= [ 5.86755877e+02 -8.66943361e-09 -2.43090741e+03 2.46877353e+03
-2.34731345e-09 6.66457112e-10 -1.20823237e+01 2.22654961e-09
6.99860524e+00 -1.94583589e+02 -2.67144085e-10 4.64133620e-10
7.31601446e-11 3.21392690e-10 -1.89132265e-10 7.45785655e-11
3.91901267e-09 9.19625484e+01 -1.49720497e+02 0.00000000e+00
0.00000000e+00 2.76208814e+03 0.00000000e+00 0.00000000e+00
0.00000000e+00 0.00000000e+00 0.00000000e+00 9.96980980e-02
0.00000000e+00 4.50579510e-02 3.12743917e+00 0.00000000e+00
0.00000000e+00 0.00000000e+00 0.00000000e+00 0.00000000e+00
0.00000000e+00 0.00000000e+00 -2.30842696e-01 -1.20554724e+00
0.00000000e+00 0.00000000e+00 -4.28257797e+00 0.00000000e+00
0.00000000e+00 0.00000000e+00 0.00000000e+00 0.00000000e+00
0.00000000e+00 0.00000000e+00 0.00000000e+00 0.00000000e+00
0.00000000e+00 0.00000000e+00 0.00000000e+00 0.00000000e+00
0.00000000e+00 0.00000000e+00 0.00000000e+00 0.00000000e+00
0.00000000e+00 0.00000000e+00 0.00000000e+00 -9.34651320e-01
5.89748395e+00 0.00000000e+00 0.00000000e+00 -5.02728643e+01
0.00000000e+00 0.00000000e+00 0.00000000e+00 0.00000000e+00
0.00000000e+00 -2.25687557e+02 0.00000000e+00 0.00000000e+00
0.00000000e+00 0.00000000e+00 0.00000000e+00 0.00000000e+00
0.00000000e+00 0.00000000e+00 0.00000000e+00]
"""
y_predict = reg.predict(X_poly)
plt.plot(x['age'], y, 'b.')
plt.plot(X_poly[:, 0], y_predict, 'r.')
plt.show()
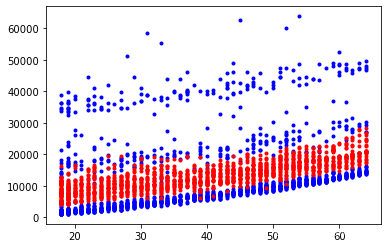
完整代码如下:
import pandas as pd
import matplotlib.pyplot as plt
from sklearn.preprocessing import PolynomialFeatures
from sklearn.linear_model import LinearRegression
data = pd.read_csv('./insurance.csv')
print(type(data))
print(data.head())
print(data.tail())
print(data.describe())
data_count = data['age'].value_counts()
print(data_count)
data_count[:10].plot(kind='bar')
plt.show()
print(data.corr())
reg = LinearRegression()
x = data[['age', 'sex', 'bmi', 'children', 'smoker', 'region']]
y = data['charges']
x = x.apply(pd.to_numeric, errors='coerce')
y = y.apply(pd.to_numeric, errors='coerce')
x.fillna(0, inplace=True)
y.fillna(0, inplace=True)
poly_features = PolynomialFeatures(degree=3, include_bias=False)
X_poly = poly_features.fit_transform(x)
reg.fit(X_poly, y)
print(reg.coef_)
print(reg.intercept_)
y_predict = reg.predict(X_poly)
plt.plot(x['age'], y, 'b.')
plt.plot(X_poly[:, 0], y_predict, 'r.')
plt.show()
四、总结
Ⅰ多项式回归:叫回归但并不是去做拟合的算法,PolynomialFeatures是来做预处理的,来转换我们的数据,把数据进行升维!
Ⅱ升维有什么用?
答:升维就是增加更多的影响Y结果的因素,这样考虑的更全面,最终的目的是要增加准确率! 还有时候,就像PolynomialFeatures去做升维,是为了让线性模型去拟合非线性的数据!
ⅢPolynomialFeatures是怎么升维的?
答:可以传入degree超参数,如果等于2,那么就会在原有维度基础之上增加二阶的数据变化!更高阶的以此类推
Ⅳ如果数据是非线性的变化,但是就想用线性的模型去拟合这个非线性的数据,怎么办?
答:1,非线性的数据去找非线性的算法生成的模型去拟合 2,可以把非线性的数据进行变化,变成类似线性的变化,然后使用线性的模型去拟合 PolynomialFeatures类其实就是这里说的第二种方式
Ⅴ保险的案例:
目的:未来来个新的人,可以通过模型来预测他的医疗花销,所以,就把charges列作为y,其他列作为X维度
Ⅵ为什么每行没有人名?
答:人名不会对最终的Y结果产生影响,所以可以不用
Ⅶ为什么要观测注意数据多样性,采样要均匀?
答:就是因为你要的模型的功能是对任何年龄段的人都有一个好的预测,那么你的模型在训练的时候 读取的数据集,就得包含各个年龄段的数据,而且各个年龄段也得数据均匀,防止过拟合!
Ⅷ什么是Pearson相关系数?
答:Pearson相关系数是来测量两组变量之间的线性相关性的!Pearson相关系数的区间范围是-1到1之间 如果越接近于-1,说明两组变量越负相关,一个变大,另一个变小,反之如果越接近于1,说明两组变量越正相关,一个变大,另一个也跟着变大,如果越接近于0,说明越不相关,即一个变大或变小,另一个没什么影响! 通过Pearson相关系数,如果发现两个维度之间,相关系数接近于1,可以把其中一个去掉,做到降维! 通过Pearson相关系数,如果发现某个维度和结果Y之间的相关系数接近于0,可以把这个维度去掉,降维!
|