cgan结构图
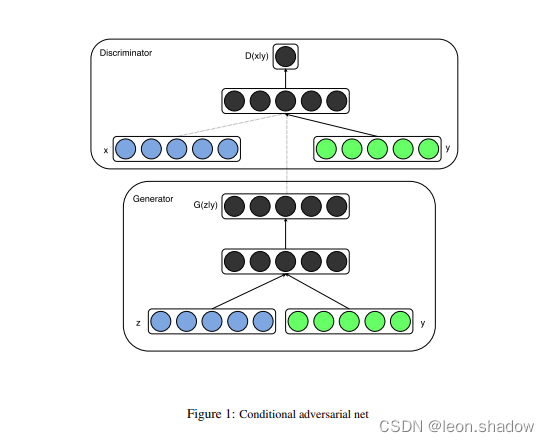
要点
- 原始gan网络无法控制输出什么图片,因此作者想通过添加一些额外的信息来控制模型输出指定的结果
- 添加的这个额外信息既要输入生成器也要输入判别器

代码
import numpy as np
import torchvision.transforms as transforms
from torchvision.utils import save_image
from torch.utils.data import DataLoader
from torchvision import datasets
from torch.autograd import Variable
import torch.nn as nn
import torch
n_epoches=300
batch_size=32
lr=0.0002
b1=0.5
b2=0.999
latent_dim=100
n_classes=4
img_size=112
channels=3
sample_interval=400
img_shape = (channels,img_size,img_size)
cuda = True if torch.cuda.is_available() else False
class Generator(nn.Module):
def __init__(self):
super(Generator, self).__init__()
self.label_emb = nn.Embedding(n_classes,n_classes)
def block(in_feat, out_feat, normalize=True):
layers = [nn.Linear(in_feat, out_feat)]
if normalize:
layers.append(nn.BatchNorm1d(out_feat, 0.8))
layers.append(nn.LeakyReLU(0.2, inplace=True))
return layers
self.model = nn.Sequential(
*block(latent_dim + n_classes, 128, normalize=False),
*block(128, 256),
*block(256, 512),
*block(512, 1024),
nn.Linear(1024, int(np.prod(img_shape))),
nn.Tanh()
)
def forward(self, noise, labels):
gen_input = torch.cat((self.label_emb(labels), noise), -1)
img = self.model(gen_input)
img = img.view(img.size(0), *img_shape)
return img
class Discriminator(nn.Module):
def __init__(self):
super(Discriminator, self).__init__()
self.label_embedding = nn.Embedding(n_classes,n_classes)
self.model = nn.Sequential(
nn.Linear(n_classes + int(np.prod(img_shape)), 512),
nn.LeakyReLU(0.2, inplace=True),
nn.Linear(512, 512),
nn.Dropout(0.4),
nn.LeakyReLU(0.2, inplace=True),
nn.Linear(512, 512),
nn.Dropout(0.4),
nn.LeakyReLU(0.2, inplace=True),
nn.Linear(512, 1),
)
def forward(self, img, labels):
d_in = torch.cat((img.view(img.size(0), -1), self.label_embedding(labels)), -1)
validity = self.model(d_in)
return validity
adversarial_loss = torch.nn.MSELoss()
generator = Generator()
discriminator = Discriminator()
if cuda:
generator.cuda()
discriminator.cuda()
adversarial_loss.cuda()
data_tramsform={
'cgan':transforms.Compose(
[transforms.RandomResizedCrop(112),
transforms.RandomHorizontalFlip(),
transforms.ToTensor(),
transforms.Normalize((0.5,0.5,0.5),(0.5,0.5,0.5))
]
)
}
image_path=r"数据集地址"
train_dataset=datasets.ImageFolder(root=os.image_path,transform=data_tramsform['cgan'])
optimizer_G = torch.optim.Adam(generator.parameters(), lr=lr, betas=(b1,b2))
optimizer_D = torch.optim.Adam(discriminator.parameters(), lr=lr, betas=(b1,b2))
train_loader=torch.utils.data.DataLoader(train_dataset,batch_size=batch_size,shuffle=True)
FloatTensor = torch.cuda.FloatTensor if cuda else torch.FloatTensor
LongTensor = torch.cuda.LongTensor if cuda else torch.LongTensor
def sample_image(n_row, batches_done):
"""产生图片"""
z = Variable(FloatTensor(np.random.normal(0, 1, (n_row ** 2,latent_dim))))
labels = np.array([num for _ in range(n_row) for num in range(n_row)])
labels = Variable(LongTensor(labels))
gen_imgs = generator(z, labels)
save_image(gen_imgs.data, r"保存地址/%d.png" % batches_done, nrow=n_row, normalize=True)
for epoch in range(n_epoches):
for i,data in enumerate(train_loader):
imgs,labels=data
batch_size = imgs.shape[0]
valid = Variable(FloatTensor(batch_size, 1).fill_(1.0), requires_grad=False)
fake = Variable(FloatTensor(batch_size, 1).fill_(0.0), requires_grad=False)
real_imgs = Variable(imgs.type(FloatTensor))
labels = Variable(labels.type(LongTensor))
optimizer_G.zero_grad()
z = Variable(FloatTensor(np.random.normal(0, 1, (batch_size,latent_dim))))
gen_labels = Variable(LongTensor(np.random.randint(0,n_classes, batch_size)))
gen_imgs = generator(z, gen_labels)
validity = discriminator(gen_imgs, gen_labels)
g_loss = adversarial_loss(validity, valid)
g_loss.backward()
optimizer_G.step()
optimizer_D.zero_grad()
validity_real = discriminator(real_imgs, labels)
d_real_loss = adversarial_loss(validity_real, valid)
validity_fake = discriminator(gen_imgs.detach(), gen_labels)
d_fake_loss = adversarial_loss(validity_fake, fake)
d_loss = (d_real_loss + d_fake_loss) / 2
d_loss.backward()
optimizer_D.step()
print(
"[Epoch %d/%d] [Batch %d/%d] [D loss: %f] [G loss: %f]"
% (epoch,n_epoches, i, len(train_loader), d_loss.item(), g_loss.item())
)
batches_done = epoch * len(train_loader) + i
if batches_done % sample_interval == 0:
sample_image(n_row=4, batches_done=batches_done)
生成器网络详解
Generator( (label_emb): Embedding(4, 4) (model): Sequential( (0): Linear(in_features=104, out_features=128, bias=True) (1): LeakyReLU(negative_slope=0.2, inplace=True) (2): Linear(in_features=128, out_features=256, bias=True) (3): BatchNorm1d(256, eps=0.8, momentum=0.1, affine=True, track_running_stats=True) (4): LeakyReLU(negative_slope=0.2, inplace=True) (5): Linear(in_features=256, out_features=512, bias=True) (6): BatchNorm1d(512, eps=0.8, momentum=0.1, affine=True, track_running_stats=True) (7): LeakyReLU(negative_slope=0.2, inplace=True) (8): Linear(in_features=512, out_features=1024, bias=True) (9): BatchNorm1d(1024, eps=0.8, momentum=0.1, affine=True, track_running_stats=True) (10): LeakyReLU(negative_slope=0.2, inplace=True) (11): Linear(in_features=1024, out_features=37632, bias=True) (12): Tanh() ) )
判别器网络详解
Discriminator( (label_embedding): Embedding(4, 4) (model): Sequential( (0): Linear(in_features=37636, out_features=512, bias=True) (1): LeakyReLU(negative_slope=0.2, inplace=True) (2): Linear(in_features=512, out_features=512, bias=True) (3): Dropout(p=0.4, inplace=False) (4): LeakyReLU(negative_slope=0.2, inplace=True) (5): Linear(in_features=512, out_features=512, bias=True) (6): Dropout(p=0.4, inplace=False) (7): LeakyReLU(negative_slope=0.2, inplace=True) (8): Linear(in_features=512, out_features=1, bias=True) ) )
|