1.论文地址
https://arxiv.org/abs/1704.04861
2.介绍
MobileNet是谷歌轻量化卷积神经网络,轻量化网络广泛用于无人驾驶,移动端,物联网的边缘计算和人工智能算法部署,所以MobileNet也成为了很多轻量化网络的骨干网络。 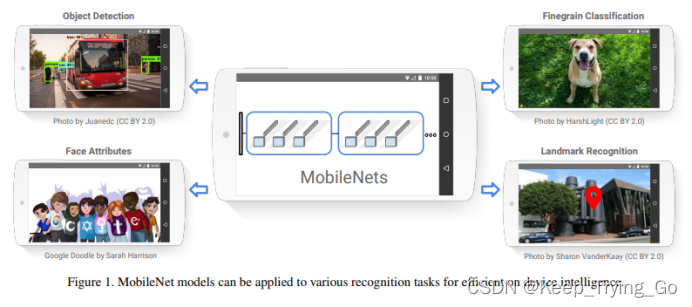
3.GPU和CPU上前向传播的计算量
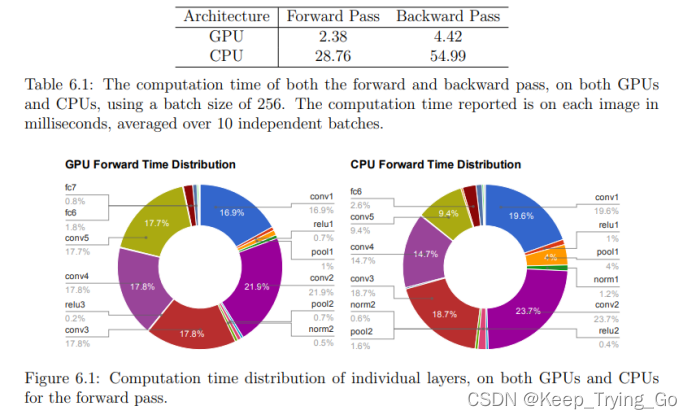 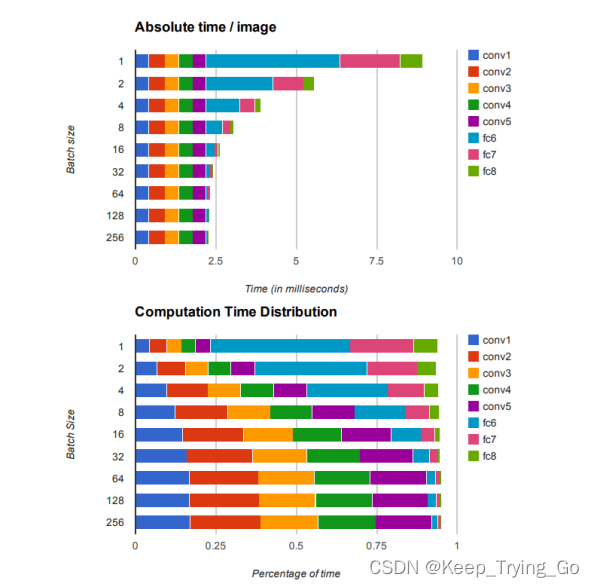 https://www2.eecs.berkeley.edu/Pubs/TechRpts/2014/EECS-2014-93.pdf 从上面的图表可以看出,随着batch size的大小不断增加,中间的卷积推理运算反而在不断的增加,而全连接层不断的减少,所以对于卷积过程的推理运算优化是很重要的。 MobileNetV1减少基于深度卷积神经网络(depthwise separable convolutions)来进行优化的.
4.标准卷积和深度可分离卷积
参考博文: https://mydreamambitious.blog.csdn.net/article/details/124503596 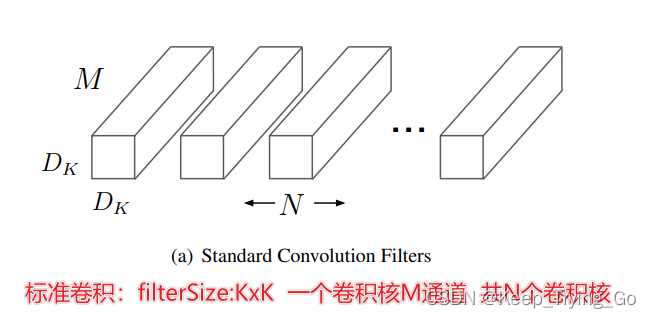 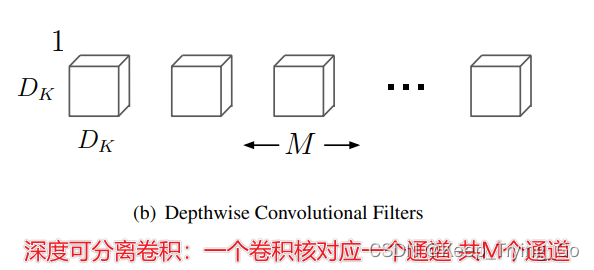 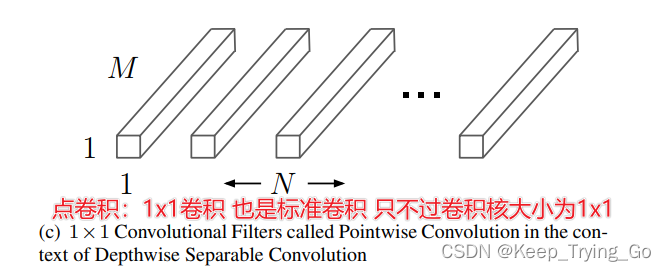 关于1x1卷积核的作用:https://mydreamambitious.blog.csdn.net/article/details/123027344 分组卷积:一个卷积只负责处理一部分feature map。 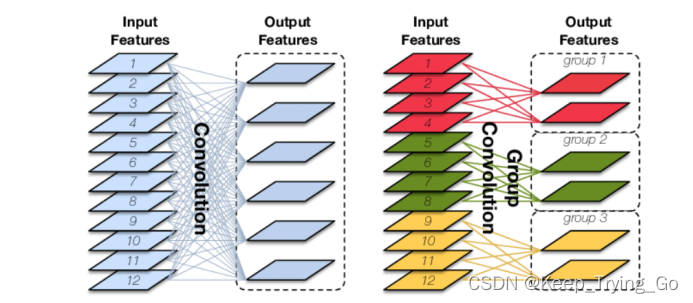 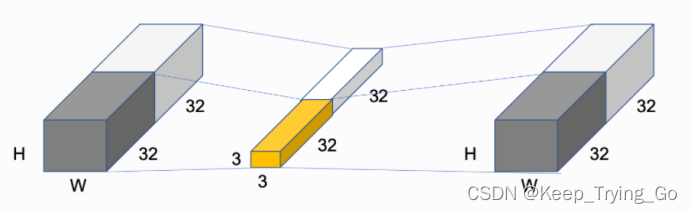 https://www.cnblogs.com/shine-lee/p/10243114.html
5.深度可分离卷积计算机量对比
  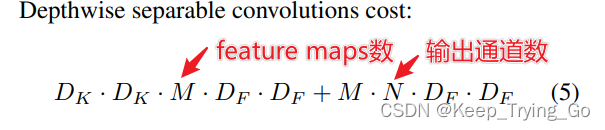 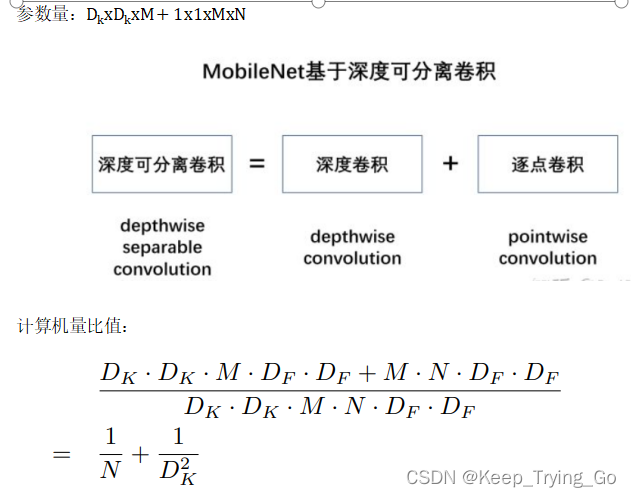 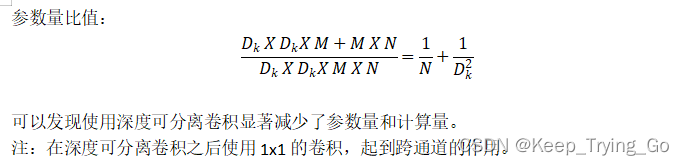  关于标准卷积,深度可分离卷积,分组卷积的参数量和计算机量的公式: https://blog.csdn.net/qq_36758914/article/details/106885400
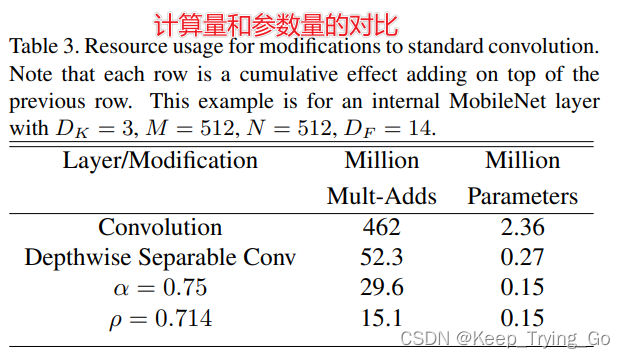 对于上面的计算量和参数计算:这里假设输入特征图大小:14x14x512;卷积核大小:3x3x512 (1)对于标准卷积: 参数量:3x3x512x512=235,293约等于2.36M 计算量:14x14x512x3x3x512=462,422,016约等于462M
(2)深度可分离卷积卷积: 参数量:3x3x512x14x14+512x512x14x14=52,283,392约等于52.3M 计算量:3x3x512+1x1x512x512=266,572约等于0.27M
(3)对于深度可分离卷积(α=0.75)卷积: 参数量:3x3x0.75x512+1x1x0.75x512x0.75x512=150,912约等于0.15M 计算量:3x3x0.75x512x14x14+0.75x512x0.75x512x14x14=29,578,752约等于29.6M
(4)对于深度可分离卷积(ρ=0.75)卷积: 参数量:3x3x0.75x512x0.714x14+0.75x512x0.75x512x0.714x14x0.714x14=15,079,129约等于15.1M 计算量:3x3x0.75x512+0.75x512x0.75x512=150,912约等于0.15M
6.MobileNetV1网络结构
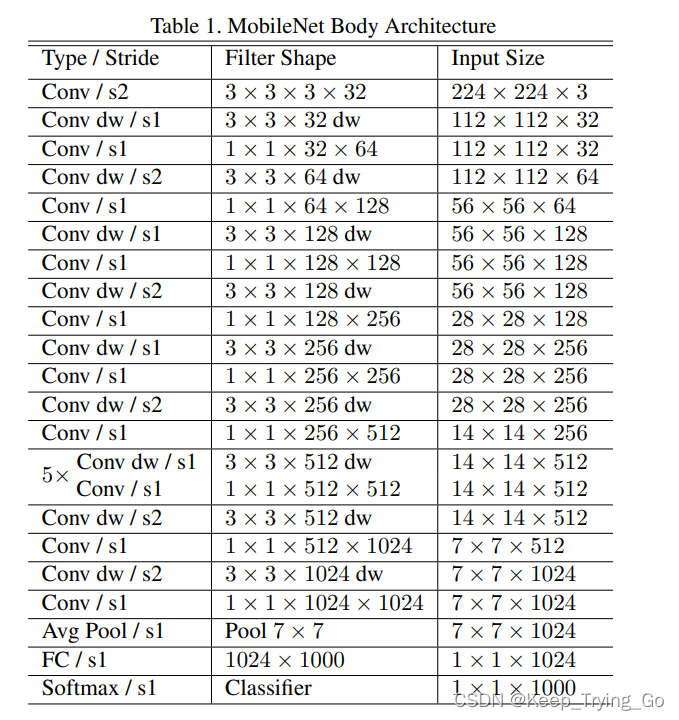 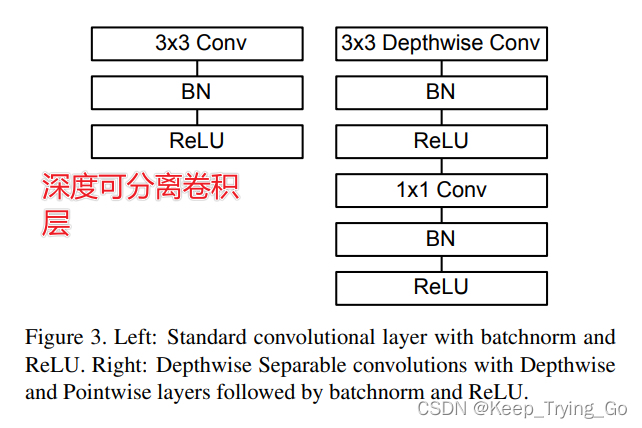 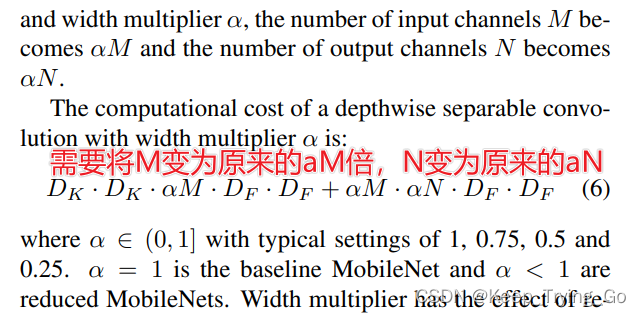 这个α等于1的时候不变,小于1的时候进行压缩;并且这个α可以被用于任何的模型结构。 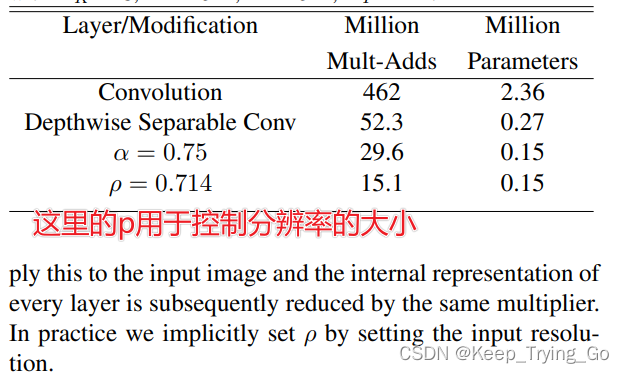 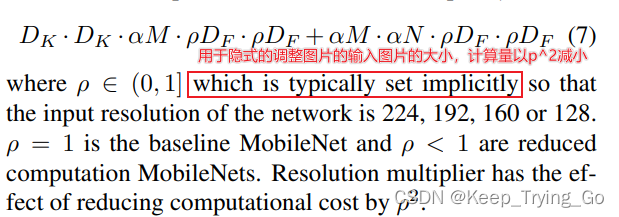
7.结果的对比
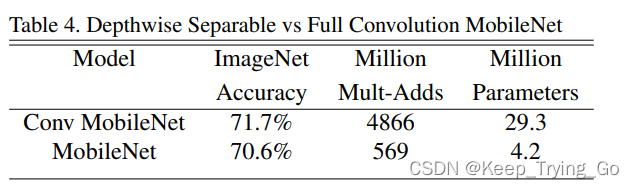 上面这张表表示了使用全卷积的MobileNet和使用深度可分离卷积的MobileNet在ImageNet上训练的结果,可以发现深度可分离卷积的MobileNet和全卷积的MobileNet在准确率上相差1.1个百分点,但是深度可分离卷积的MobileNet的计算量和参数量远远低于全卷积的MobileNet。所以使用深度可分离卷积的MobileNet是非常轻量化的。
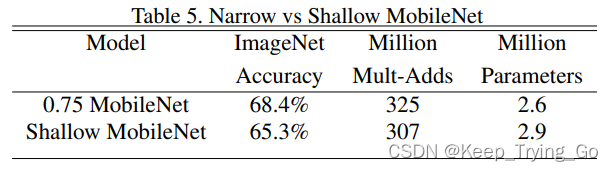 上面这张表表示了α=0.75的MobileNet和浅层的MobileNet准备率对比,发现浅层的MobileNet准确率下降较多,并且这里的计算量和参数量并没有太大的差别,所以深度的网络对于准确率的提升很重要。 注:这里的浅层的MobileNet是指将网络中的去掉之后的结果。  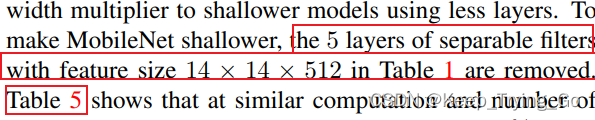 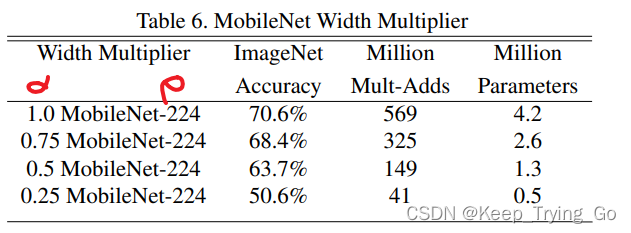 上面表示在相同的ρ(分辨率)的情况下,随着α的不断减小,准确率下降较多,虽然参数量和计算量也在不断的减少,可是这样做是不值得的。因为随着α不断的减小,卷积核的个数减少,权重减少,模型的表达能力也在不断降低,导致欠拟合。 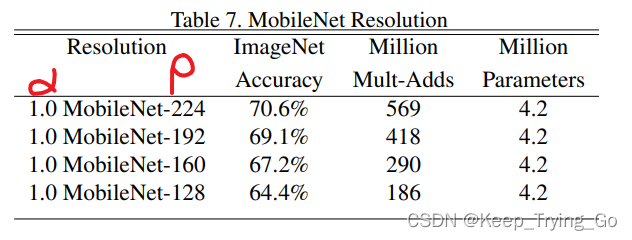 上面表示随着的ρ(分辨率)不断减小的情况下,α不变的情况下,准确率也下降了较多,虽然计算量也在不断的减少。 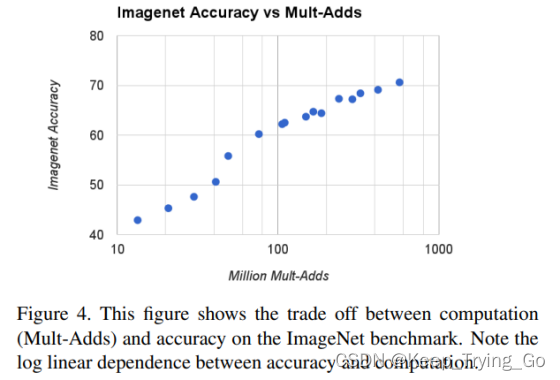 上面这张图表示了准确率随着计算量的增加而不断提高,并且是呈对数模型的增加,所以需要我们在准确率和计算量方面进行权衡。
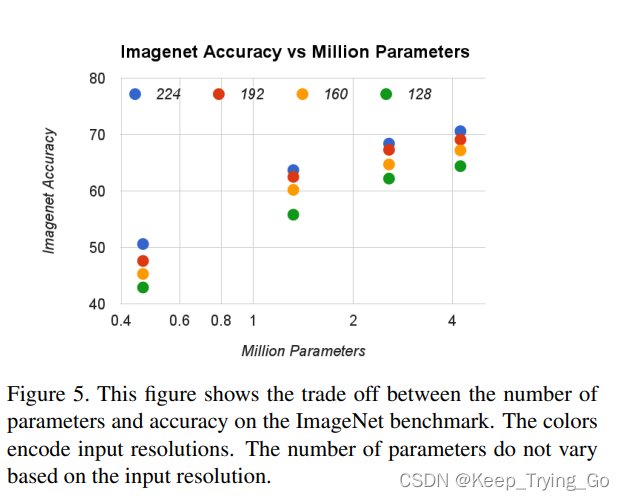 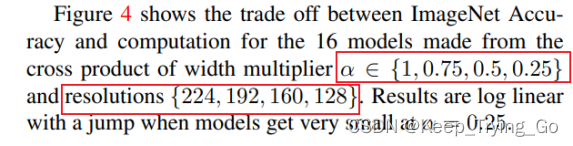 上面这个图表示了不同ρ(分辨率)和α(卷积核数)的16中组合准确率和计算量的权衡。 可以看到随着α的不断降低,准确率下降的更加快。 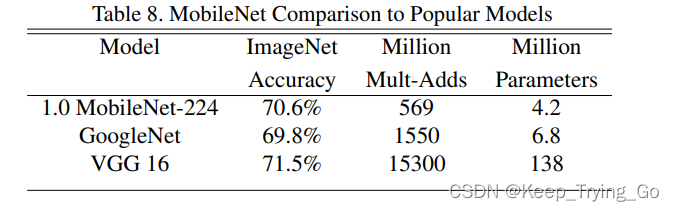 从上面的表中可以看到MobileNet准确率虽然比VGG16准确率较低,但是计算量和参数量是大大的降低。MobileNet的准确率又比GoogLeNet准确率要高。 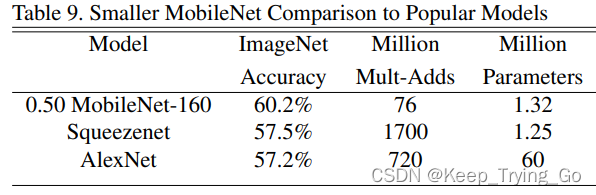 该表表示了MobileNet的准确率都比SqueezeNet和AlexNet的准确率都要高,而且参数量也是相对于他们要低很多。
8.MobileNetV1网络结构实现
import os
import keras
import numpy as np
import tensorflow as tf
from tensorflow.keras import layers
class DepthWiseConv(tf.keras.Model):
def __init__(self,filter,stride=(1,1)):
super(DepthWiseConv, self).__init__()
self.depthwiseconv=layers.DepthwiseConv2D(kernel_size=[3,3],strides=stride,padding='same')
self.depthbatch=layers.BatchNormalization()
self.depthrelu=layers.Activation('relu')
self.conv11=layers.Conv2D(filter,kernel_size=[1,1],strides=[1,1],padding='same')
self.conv11batch=layers.BatchNormalization()
self.conv11relu=layers.Activation('relu')
def call(self,inputs,training=None):
x=self.depthwiseconv(inputs)
x=self.depthbatch(x)
x=self.depthrelu(x)
x=self.conv11(x)
x=self.conv11batch(x)
x=self.conv11relu(x)
return x
class MobileNetV1(tf.keras.Model):
def __init__(self,num_classes):
super(MobileNetV1, self).__init__()
self.conv1=layers.Conv2D(32,kernel_size=[3,3],strides=[2,2],padding='same')
self.conv1batch=layers.BatchNormalization()
self.conv1relu=layers.Activation('relu')
self.depthconv=self.depthwiseconv()
self.avgpool=layers.GlobalAveragePooling2D()
self.dense=layers.Dense(num_classes)
self.dropout=layers.Dropout(rate=0.5)
self.softmax=layers.Activation('softmax')
def depthwiseconv(self):
depth=keras.Sequential([])
depth.add(
DepthWiseConv(filter=64,stride=(1,1))
)
depth.add(
DepthWiseConv(filter=128, stride=(2, 2))
)
depth.add(
DepthWiseConv(filter=128, stride=(1, 1))
)
depth.add(
DepthWiseConv(filter=256, stride=(2, 2))
)
depth.add(
DepthWiseConv(filter=256, stride=(1, 1))
)
depth.add(
DepthWiseConv(filter=256, stride=(2, 2))
)
for i in range(5):
depth.add(
DepthWiseConv(filter=512, stride=(1, 1))
)
depth.add(
DepthWiseConv(filter=512, stride=(2,2))
)
depth.add(
DepthWiseConv(filter=1024, stride=(1, 1))
)
return depth
def call(self,inputs,training=None):
x=self.conv1(inputs)
x=self.conv1batch(x)
x=self.conv1relu(x)
x=self.depthconv(x)
x=self.avgpool(x)
x=self.dense(x)
x=self.dropout(x)
x=self.softmax(x)
return x
model_mobilenetv1=MobileNetV1(num_classes=1000)
model_mobilenetv1.build(input_shape=(None,224,224,3))
model_mobilenetv1.summary()
if __name__ == '__main__':
print('pycharm')
|