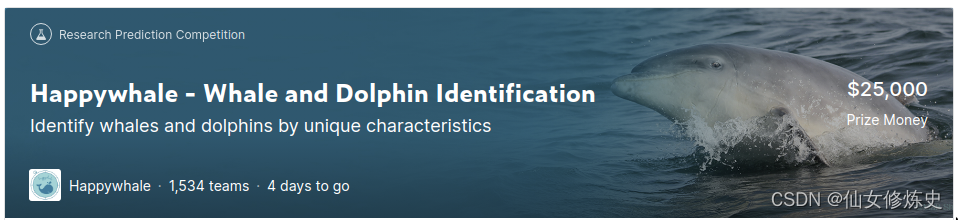
一、ID识别问题
ID1: 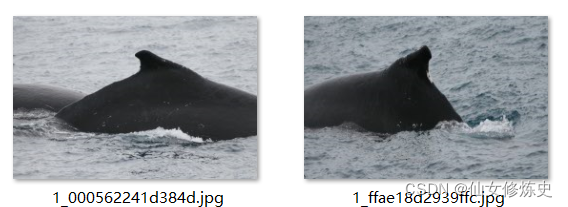 ID2: 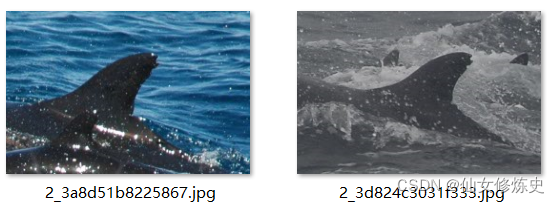 下图是哪个ID: 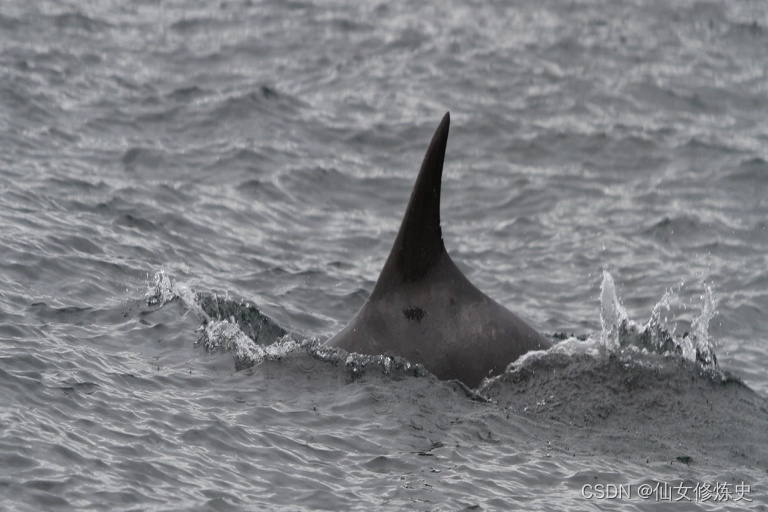
二、训练数据分布
- 51033张训练图片, 27956张测试图片,public LB用了24%的测试图片,最后结果以private LB的结果为准
- Whale和Dolphin两个类别的数据分布
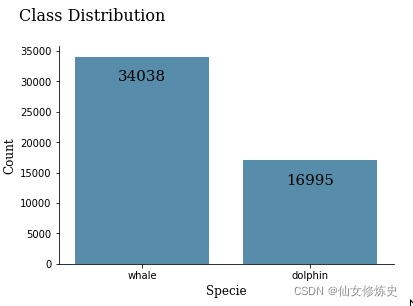 - Whale和Dolphin下面总共26中类别
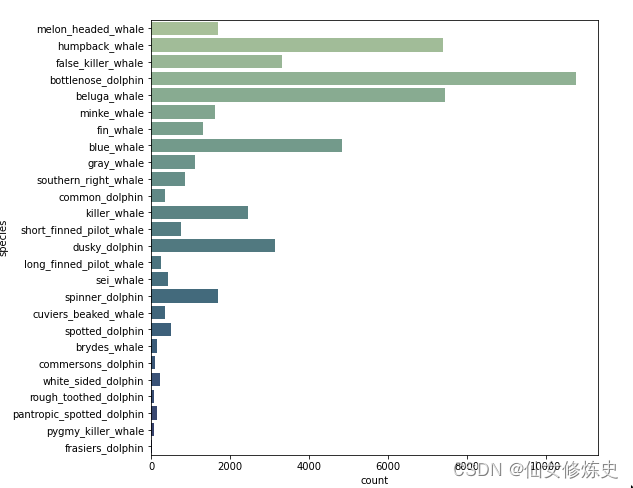 - 5w多张图片总共含有15587个ID,前10个ID的分布如下:
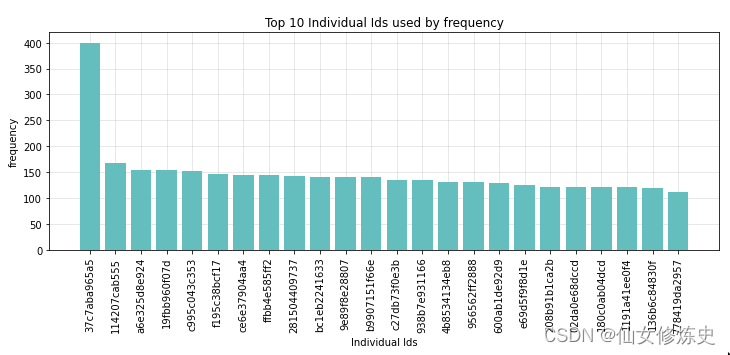 - 训练图片中有很多ID只有一张图片
- 测试图片中,有些ID 没有在训练图片中出现
三、我的解决方案
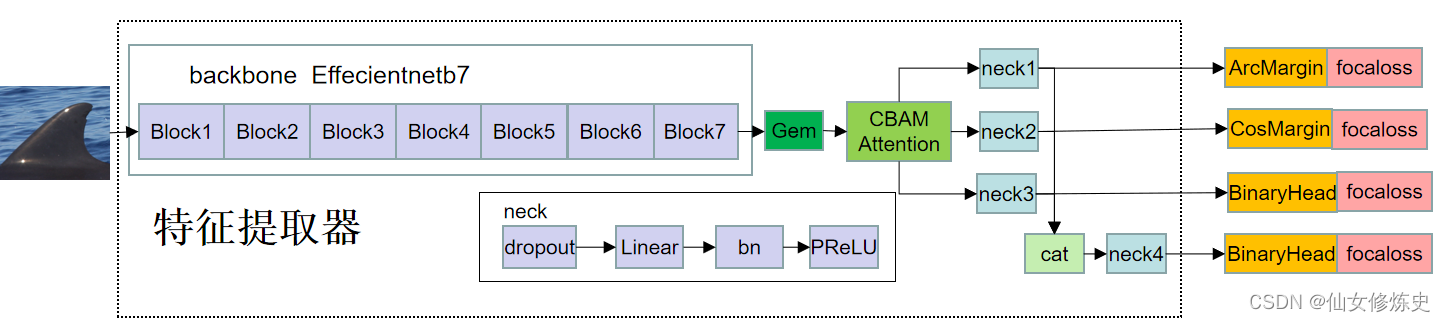 训练的时候,采用effiecientnetb7作为特征提取器,使用gem pooling,加入CBAM attention,四个neck,四个loss;测试的时候,将四个neck的特征cat到一起,就是提取的embeddings。
四、我的用到的tricks
- 学习率:warmup+cos下降
- 冻结bn层:小的batch_size下不能正确估计统计变量E(x)和V(x),还不如不更新。详细看这里
- 混合精度训练:GPU下的某些操作,将float32转换为float16,损失少量的精度,可以节省大量的内存。详细看这里
- 梯度累加:梯度累加几个batch_size之后再更新,loss求平均时防止梯度爆炸。详细看这里
- CBAM attention:通道和空间域的attention。
- test_tta:Test Time Augmentation
- 不同input size之间的ensemble:确定input size 时,CNN 学会了找到特定大小的特定特征;那么,修改input size后再训练,学习后的CNN找到的其他大小下的其他特征,因此可以用不同的input size,看哪种更接近最优值。
五、tricks的效果
下面的表格是我在effiecientnetb5上的训练结果,因为effiecientnetb5更新快,score是在public LB上的分数: 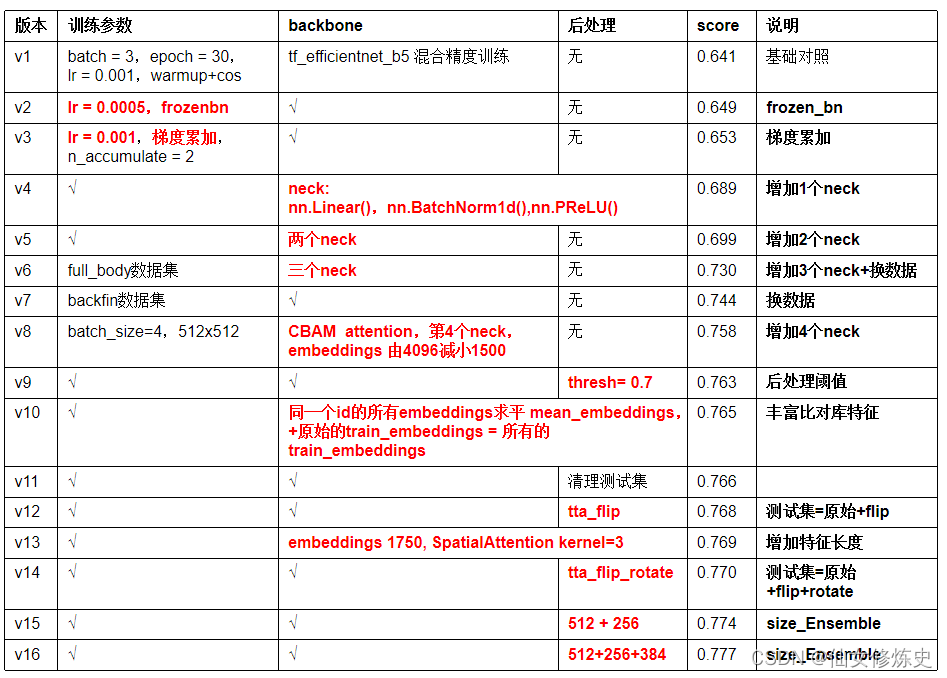
六、有用未成功的tricks
-
Progressive Resizing step1:Train model on size: small step2:Save weights and re-train model on larger image size step3:Save weights again and re-train on final image sizes -
Differential Learning Rates 上层特征,用小的学习率,低层特征,用较大学习率 -
PsuedoLabelling 训练—测试—加入训练集—再训练—再测试
下面内容是我重点参考的,觉得非常有用的
不用翻墙的kaggle 讨论: 1、9 Computer Vision Tricks to Improve Performance 2、7 More Computer Vision Tricks to Improve Score 3、Previous Happywhale Competition Solutions 4、CNN Input Size Explained
下面链接需要翻墙: 这个blog有四个大trick: trick1: freeze Novel techniques to win an Image Classification hackathon (Part-1) trick2: progressive trainning Novel techniques to win an Image Classification hackathon (Part-2) trick3: attentation Novel techniques to win an Image Classification hackathon (Part-3) trick4:Ensemble and TTA Novel techniques to win an Image Classification hackathon (Part-4)
这个第7名的方案是我主要参考的方案: Thanks Radek 7th place solution to HWI 2019 competition
|