针孔照相机模型
针孔照相机模型是计算机视觉中广泛使用的照相机模型。 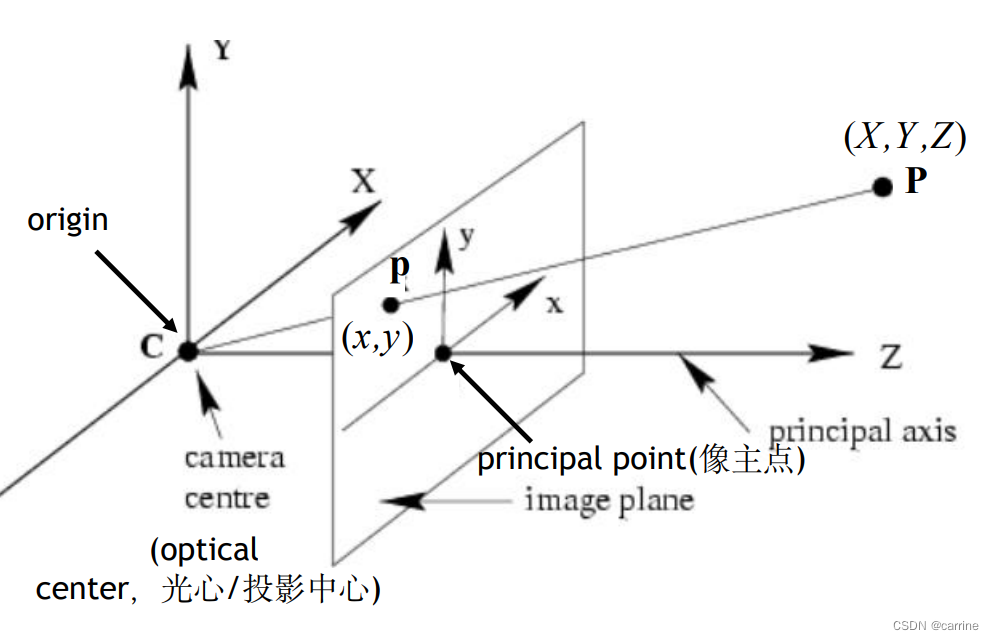 在针孔照相机模型中,光线投影到图像平面之前,从唯一的一个点经过,也就是照相机中心C,上图坐标系为相机坐标系,以照相机中心为原点,P代表了真实世界中的一个三维点,p代表了其映射到二维图像的点。
照相机矩阵
从坐标系的侧面看,可以看出P和p之间可以通过相似来求出他们的关系, 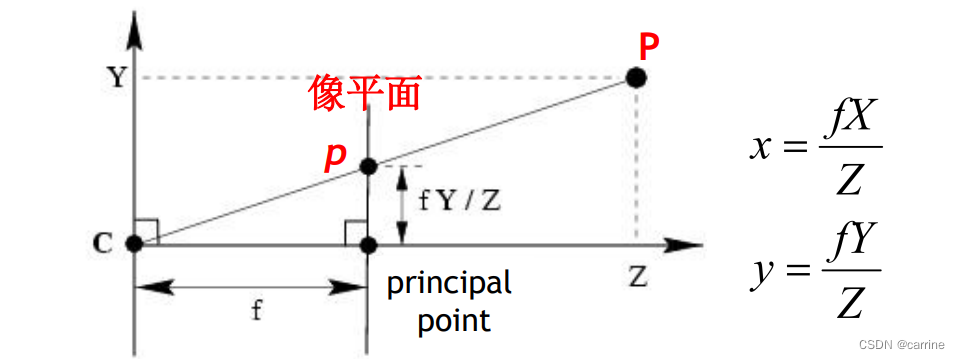
这里我们用矩阵来表示, 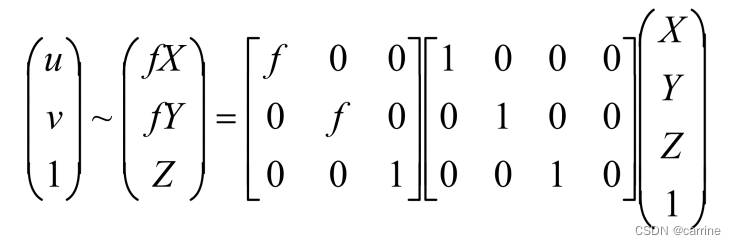 在相机的生产过程中,其镜头会发生偏移,也就是光心和坐标系的原点有偏移,称为主点偏移。要考虑到这个因素,所以上式要加上偏移量(u0,v0),u0代表了在x方向上的偏移量,v0代表了y方向上偏移量,变成了,
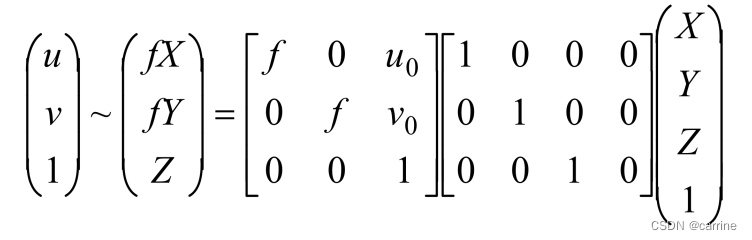 我们将其简写为,K代表了相机的内部参数矩阵
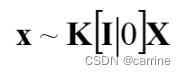 考虑到有内部参数的影响,但是还有物理层面上的影响,比如说镜头,我们日常生活中最常接触的就是广角镜头,当然了,还有许多别的镜头,只是我不知道…… 不同镜头拍摄出的照片会和普通照片拍摄出来的不一样,会在某方面产生畸变,比如径向畸变、桶状畸变、枕形畸变等,为此我们还得再K中加入畸变参数, 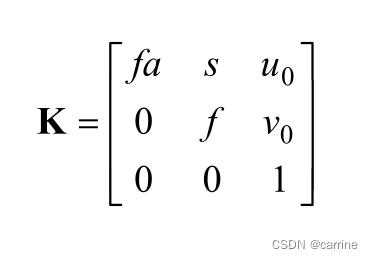 既然考虑好了内部参数的影响,外部环境的影响我们也要考虑,比如人为的因素,我们拿相机拍摄的时候,每次变换位置,相机位置也会跟着改变,而且有时我们会将相机旋转角度以达到更好的拍摄效果,所以还需要往照相机矩阵中加入外部参数的影响,上式变为, 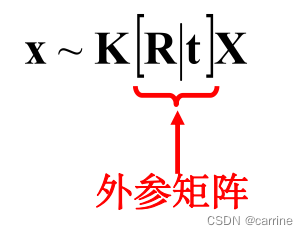
相机参数标定
-
首先,我们需要进行数据采集,也就是对棋盘格进行不同角度的拍摄,在此期间,需保证棋盘格在一个平面上,否则因为纸张弯曲导致后续角点检测时出现角点间距不一致的问题。 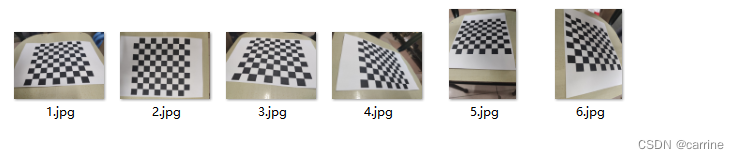 -
棋盘格角点的提取。
succ, pic_coor = cv.findChessboardCorners(pic_data, (cross_corners[0], cross_corners[1]), None)
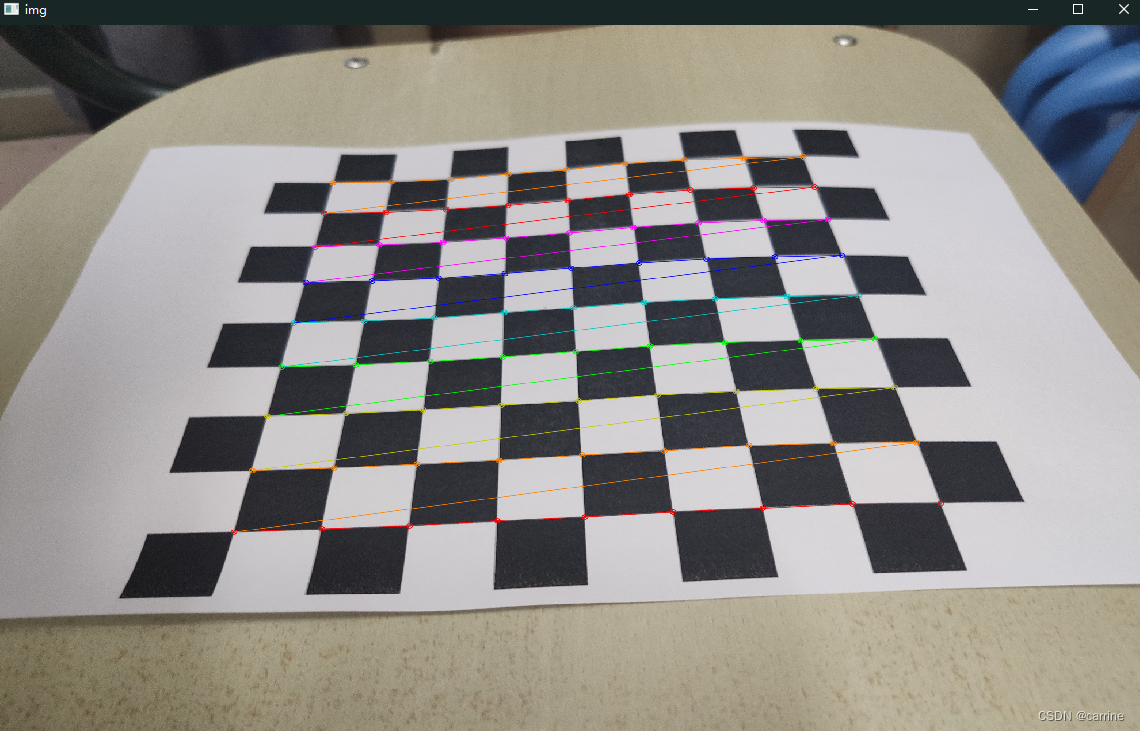
- 通过角点求解出单应性矩阵,再由单应性矩阵经SVD求解出内部参数。
H = get_homography(pic_points, real_points_x_y)
intrinsics_param = get_intrinsics_param(H)
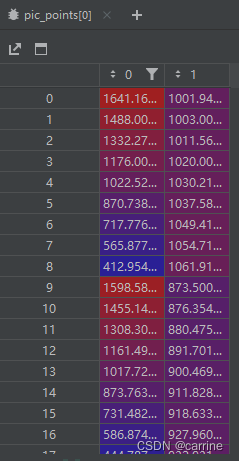 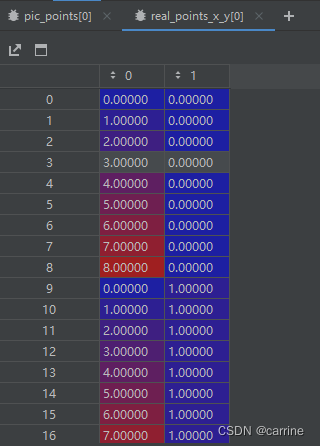 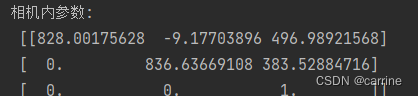
上述相机的内部参数是采用环形拍摄棋盘格获得的相机内参数,相机离棋盘比较近(
1000
?
750
1000*750
1000?750和
750
?
1000
750*1000
750?1000都有)
多种实验结果
下面采用的是平移相机对棋盘格拍照的方式获得的相机内参数,相机离棋盘比较远(
750
?
1000
750*1000
750?1000) 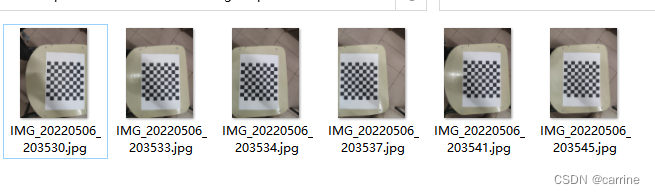 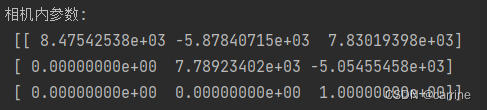 下面也是采用的是平移相机对棋盘格拍照的方式获得的相机内参数,相机离棋盘比较近(
1000
?
750
1000*750
1000?750) 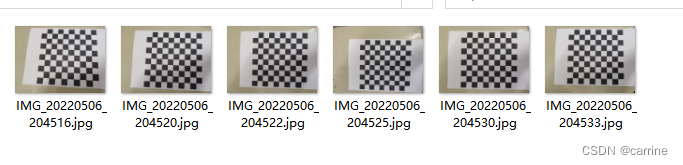
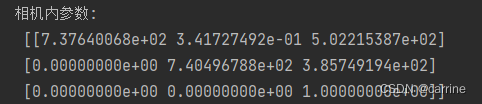 下面采用的是平移相机对棋盘格拍照的方式获得的相机内参数,相机离棋盘比较远(
1000
?
750
1000*750
1000?750) 
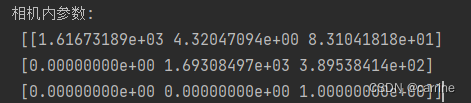
总结
通过四种不同数据得出的相机内部参数,得出几个结论:
- 采用相同拍摄手法的数据得出的相机内参数中的焦距相差不是很大,拍摄手法对光心的影响更大。
- 相机自身的旋转会造成焦距和光心与旋转前的数据相反。
- 平移相机拍摄的情况下,相机距离棋盘的远近得出的内参数的相差不是很大,而当相机相对于棋盘倾斜的情况下会导致内参数发生大的变化。
猜测是因为倾斜的情况下,相机中的棋盘格中,检测出的角点间距离不一致导致。这个理论也可以佐证为什么平移拍摄时内参数相差不大的情况。
主代码
import cv2 as cv
import numpy as np
import os
from step.homography import get_homography
from step.intrinsics import get_intrinsics_param
from step.extrinsics import get_extrinsics_param
from step.distortion import get_distortion
def calibrate():
H = get_homography(pic_points, real_points_x_y)
intrinsics_param = get_intrinsics_param(H)
extrinsics_param = get_extrinsics_param(H, intrinsics_param)
k = get_distortion(intrinsics_param, extrinsics_param, pic_points, real_points_x_y)
print("相机内参数:\t\n", intrinsics_param)
print("畸变矫正:\t", k)
print("外部参数:\t\n", extrinsics_param)
if __name__ == "__main__":
i = 1;
file_dir = r'..\pic'
pic_name = os.listdir(file_dir)
cross_corners = [9, 9]
real_coor = np.zeros((cross_corners[0] * cross_corners[1], 3), np.float32)
real_coor[:, :2] = np.mgrid[0:9, 0:9].T.reshape(-1, 2)
real_points = []
real_points_x_y = []
pic_points = []
for pic in pic_name:
pic_path = os.path.join(file_dir, pic)
pic_data = cv.imread(pic_path)
succ, pic_coor = cv.findChessboardCorners(pic_data, (cross_corners[0], cross_corners[1]), None)
if succ:
pic_coor = pic_coor.reshape(-1, 2)
pic_points.append(pic_coor)
real_points.append(real_coor)
real_points_x_y.append(real_coor[:, :2])
cv.drawChessboardCorners(pic_data, (cross_corners[0], cross_corners[1]), pic_coor, succ)
cv.namedWindow('img', cv.WINDOW_NORMAL)
cv.resizeWindow('img', 300, 300)
cv.imshow('img', pic_data)
cv.waitKey()
calibrate()
|