- 参考:动手学深度学习
- 注:本文是 jupyter notebook 文档转换而来,部分代码可能无法直接复制运行!
- 前文介绍的 经典机器学习方法(1)—— 线性回归 适用于连续值预测问题(回归问题),本文介绍适用于离散值预测(分类问题)的 softmax 回归模型,这是一种基于神经网络的经典分类模型
- softmax 回归和线性回归内部一样是线性模型,区别在于
- softmax 回归的输出从一个变成了多个
- softmax 回归中引入了 softmax 运算,使其更适合于离散值的预测和训练
1. softmax 回归原理
1.1 分类问题
1.2 softmax 回归模型
-
softmax 回归模型内部和线性回归模型几乎一致,也是一个简单的单层全连接神经网络,只是在输出层增加了节点,以获得
∣
Y
∣
|\mathcal{Y}|
∣Y∣ 个输出,以 1.1 节的 4 维输入(特征维度为4) 3 维输出(类别总数为3)为例 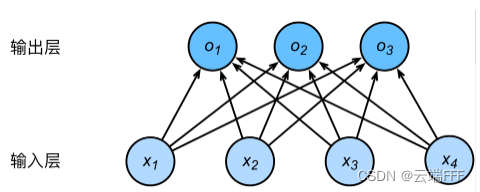 其中每个输出层节点都是输入的线性组合,即
o
1
=
x
1
w
11
+
x
2
w
21
+
x
3
w
31
+
x
4
w
41
+
b
1
o
2
=
x
1
w
12
+
x
2
w
22
+
x
3
w
32
+
x
4
w
42
+
b
2
o
3
=
x
1
w
13
+
x
2
w
23
+
x
3
w
33
+
x
4
w
43
+
b
3
\begin{aligned} &o_1 = x_1w_{11}+x_2w_{21}+x_3w_{31}+x_4w_{41} + b_1\\ &o_2 = x_1w_{12}+x_2w_{22}+x_3w_{32}+x_4w_{42} + b_2\\ &o_3 = x_1w_{13}+x_2w_{23}+x_3w_{33}+x_4w_{43} + b_3\\ \end{aligned}
?o1?=x1?w11?+x2?w21?+x3?w31?+x4?w41?+b1?o2?=x1?w12?+x2?w22?+x3?w32?+x4?w42?+b2?o3?=x1?w13?+x2?w23?+x3?w33?+x4?w43?+b3?? -
为了得到离散的预测输出,我们可以把输出值
o
i
o_i
oi? 看作置信度,输出越大的节点,对应的标记越可能是真实标签。在这里我们使用 softmax 运算将其输出值转换为正且和为 1 的概率分布,即
y
^
1
,
y
^
2
,
y
^
3
=
softmax
(
o
1
,
o
2
,
o
3
)
\hat{y}_1,\hat{y}_2,\hat{y}_3 = \text{softmax}(o_1,o_2,o_3)
y^?1?,y^?2?,y^?3?=softmax(o1?,o2?,o3?) 其中
y
^
i
\hat{y}_i
y^?i? 是如下计算的
y
^
i
=
exp
?
(
o
i
)
∑
j
exp
?
(
o
j
)
\hat{y}_i = \frac{\exp(o_i)}{\sum_j \exp(o_j)}
y^?i?=∑j?exp(oj?)exp(oi?)? 我们注意到
arg
?
max
?
i
o
i
=
arg
?
max
?
i
y
^
i
\arg\max_i o_i = \arg\max_i \hat{y}_i
argmaxi?oi?=argmaxi?y^?i?,因此 softmax 运算不改变预测类别输出
1.2.1 单样本分类的矢量计算表达式
- 为了提升运算效率,将上述运算都改成矩阵形式,
- softmax 回归的权重和偏置参数为
W
=
[
w
11
w
12
w
13
w
21
w
22
w
23
w
31
w
32
w
33
w
41
w
42
w
43
]
???
b
=
[
b
1
,
b
2
,
b
3
]
\pmb{W} = \begin{bmatrix} w_{11} & w_{12} & w_{13} \\ w_{21} & w_{22} & w_{23} \\ w_{31} &w_{32} & w_{33} \\ w_{41} &w_{42} & w_{43} \\ \end{bmatrix}\space\space\space \pmb{b} = [b_1,b_2,b_3]
WWW=?????w11?w21?w31?w41??w12?w22?w32?w42??w13?w23?w33?w43??????????bbb=[b1?,b2?,b3?] - 第
i
i
i 个样本特征为
x
1
×
4
(
i
)
=
[
x
1
(
i
)
,
x
2
(
i
)
,
x
3
(
i
)
,
x
4
(
i
)
]
\pmb{x}^{(i)}_{1\times 4} = [x_1^{(i)},x_2^{(i)},x_3^{(i)},x_4^{(i)}]
xxx1×4(i)?=[x1(i)?,x2(i)?,x3(i)?,x4(i)?] - 输出层输出为
o
1
×
3
(
i
)
=
[
o
1
(
i
)
,
o
2
(
i
)
,
o
3
(
i
)
]
\pmb{o}^{(i)}_{1\times 3} = [o_1^{(i)},o_2^{(i)},o_3^{(i)}]
ooo1×3(i)?=[o1(i)?,o2(i)?,o3(i)?] - 预测概率分布为
y
^
1
×
3
(
i
)
=
[
y
^
1
(
i
)
,
y
^
2
(
i
)
,
y
^
3
(
i
)
]
\pmb{\hat{y}}^{(i)}_{1\times 3} = [\pmb{\hat{y}}_1^{(i)},\pmb{\hat{y}}_2^{(i)},\pmb{\hat{y}}_3^{(i)}]
y^??y^???y^?1×3(i)?=[y^??y^???y^?1(i)?,y^??y^???y^?2(i)?,y^??y^???y^?3(i)?] - 通常把预测概率最大的类别作为预测类别
y
1
×
3
(
i
)
=
arg
?
max
?
j
y
^
j
(
i
)
y^{(i)}_{1\times 3} = \arg\max_{j}\pmb{\hat{y}}_j^{(i)}
y1×3(i)?=argjmax?y^??y^???y^?j(i)? 注意我们习惯使用离散的数值来表示类别,比如将其处理为
y
1
=
1
,
y
2
=
2
,
y
3
=
3
y_1=1,y_2=2,y_3=3
y1?=1,y2?=2,y3?=3,这样需要输出 1,2,3 这三个数字中的一个 - softmax 回归对样本
x
(
i
)
\pmb{x}^{(i)}
xxx(i) 进行的运算为
o
1
×
3
(
i
)
=
x
1
×
4
(
i
)
W
4
×
3
+
b
1
×
3
y
^
1
×
3
(
i
)
=
softmax
(
o
1
×
3
(
i
)
)
\begin{aligned} &\pmb{o}^{(i)}_{1\times 3} = \pmb{x}^{(i)}_{1\times 4}\pmb{W}_{4\times 3} +\pmb{b}_{1\times 3} \\ &\pmb{\hat{y}}^{(i)}_{1\times 3} = \text{softmax}(\pmb{o}^{(i)}_{1\times 3}) \end{aligned}
?ooo1×3(i)?=xxx1×4(i)?WWW4×3?+bbb1×3?y^??y^???y^?1×3(i)?=softmax(ooo1×3(i)?)?
1.2.2 mini-batch 样本分类的矢量计算表达式
- 为了进一步提升计算效率,结合常用的 mini-batch 梯度下降优化算法,我们常常对小批量数据做矢量运算。设一个小批量样本批量大小为
n
n
n,输入特征个数为
d
d
d,输出个数(类别数为)
q
q
q,则
- 批量样本特征为
X
∈
R
n
×
d
\pmb{X}\in\mathbb{R}^{n\times d}
XXX∈Rn×d
- 权重参数为
W
∈
R
d
×
q
\pmb{W} \in\mathbb{R}^{d\times q}
WWW∈Rd×q
- 偏置参数为
b
∈
R
1
×
q
\pmb{b}\in\mathbb{R}^{1\times q}
bbb∈R1×q
- 矢量计算表达式为
O
n
×
q
=
X
n
×
d
W
d
×
q
+
b
1
×
q
Y
^
n
×
q
=
softmax
(
O
n
×
q
)
\begin{aligned} &\pmb{O}_{n\times q} = \pmb{X}_{n\times d}\pmb{W}_{d\times q}+\pmb{b}_{1\times q}\\ &\pmb{\hat{Y}}_{n\times q} = \text{softmax}(\pmb{O}_{n\times q}) \end{aligned}
?OOOn×q?=XXXn×d?WWWd×q?+bbb1×q?Y^Y^Y^n×q?=softmax(OOOn×q?)? 其中加法使用了广播机制,
O
,
Y
^
∈
R
n
×
q
\pmb{O},\hat{\pmb{Y}}\in\mathbb{R}^{n\times q}
OOO,YYY^∈Rn×q 且其中第
i
i
i 行分别为样本
i
i
i 的输出
o
(
i
)
\pmb{o}^{(i)}
ooo(i) 和概率分布
y
^
(
i
)
\pmb{\hat{y}}^{(i)}
y^??y^???y^?(i)
1.3 交叉熵损失函数
-
对于某个样本
x
i
\pmb{x}_i
xxxi?,上面我们利用 softmax 运算得到看其预测标记分布
y
^
(
i
)
\pmb{\hat{y}}^{(i)}
y^??y^???y^?(i)。另一方面,此样本的真实标记也可以用一个输出空间上的分布
y
(
i
)
\pmb{y}^{(i)}
y?y??y(i) 来表示
比如样本只有一个标记时,可以构造一个 one-hot 向量
y
(
i
)
∈
R
q
\pmb{y}^{(i)}\in\mathbb{R}^q
y?y??y(i)∈Rq,使其真实标记对应的向量元素设为 1,其他设为 0,从而将真实标记转换为一个输出空间上的分布
这样我们的训练目标可以设为使预测概率分布
y
^
(
i
)
\pmb{\hat{y}}^{(i)}
y^??y^???y^?(i) 尽量接近真实概率分布
y
(
i
)
\pmb{y}^{(i)}
y?y??y(i) -
这里不太适合使用线性回归的平方损失函数
∣
∣
y
^
(
i
)
?
y
(
i
)
∣
∣
2
/
2
||\hat{\pmb{y}}^{(i)}-\pmb{y}^{(i)}||^2/2
∣∣y?y??y^?(i)?y?y??y(i)∣∣2/2,因为想得到正确的预测分类结果,只要保证真实类别的预测概率最大即可,平方损失函数要求所有可能类别的预测概率和真实概率都相等,这过于严格
假设真实标记是
y
^
3
(
i
)
\pmb{\hat{y}}^{(i)}_3
y^??y^???y^?3(i)?,当
y
^
3
(
i
)
\pmb{\hat{y}}^{(i)}_3
y^??y^???y^?3(i)? 预测值为 0.6 时即可以保证一定预测正确,如果用平方损失,这时
y
^
1
(
i
)
=
y
^
2
(
i
)
=
0.2
\pmb{\hat{y}}^{(i)}_1=\pmb{\hat{y}}^{(i)}_2=0.2
y^??y^???y^?1(i)?=y^??y^???y^?2(i)?=0.2 比
y
^
1
(
i
)
=
0
\pmb{\hat{y}}^{(i)}_1=0
y^??y^???y^?1(i)?=0,
y
^
2
(
i
)
=
0.4
\pmb{\hat{y}}^{(i)}_2=0.4
y^??y^???y^?2(i)?=0.4 的损失小很多,尽管二者有同样的分类结果
关于这个问题其实还有不少可讲的,请参考:分类问题为什么用交叉熵损失不用 MSE 损失 -
我们可以使用衡量两个分布间差异的测量函数作为损失,交叉熵(cross entropy)是一个常用的选择,它将分布
y
(
i
)
\pmb{y}^{(i)}
y?y??y(i) 和
y
^
(
i
)
\hat{\pmb{y}}^{(i)}
y?y??y^?(i) 的差距表示为
H
(
y
(
i
)
,
y
^
(
i
)
)
=
?
∑
j
=
1
q
y
j
(
i
)
log
?
y
^
j
(
i
)
H(\pmb{y}^{(i)},\hat{\pmb{y}}^{(i)}) = -\sum_{j=1}^q y_j^{(i)}\log\hat{y}_j^{(i)}
H(y?y??y(i),y?y??y^?(i))=?j=1∑q?yj(i)?logy^?j(i)? 注意其中
y
j
(
i
)
y_j^{(i)}
yj(i)? 是真实标记分布
y
(
i
)
\pmb{y}^{(i)}
y?y??y(i) 中非零即一的元素,样本真实标记为
y
(
i
)
y^{(i)}
y(i),因此
y
(
i
)
\pmb{y}^{(i)}
y?y??y(i) 中只有
y
y
(
i
)
(
i
)
=
1
y^{(i)}_{y^{(i)}}=1
yy(i)(i)?=1,其他全为 0,因此上述交叉熵可以化简为
H
(
y
(
i
)
,
y
^
(
i
)
)
=
?
log
?
y
^
y
(
i
)
(
i
)
H(\pmb{y}^{(i)},\hat{\pmb{y}}^{(i)}) = -\log\hat{y}_{y^{(i)}}^{(i)}
H(y?y??y(i),y?y??y^?(i))=?logy^?y(i)(i)? 可见最小化交叉熵损失等价于最大化对正确类别的预测概率,它只关心对正确类别的预测概率,这是合理的,因为只要其值足够大就能保证分类正确
当遇到一个多标签样本时,例如图像中含有不止一个物体时,不能做这样的简化,但是这种情况下交叉熵损失也仅仅关心图像中出现的物体类别的预测概率
-
假设训练数据样本量为
n
n
n,交叉熵损失函数定义为
l
(
Θ
)
=
1
n
∑
i
=
1
n
H
(
y
(
i
)
,
y
^
(
i
)
)
\mathscr{l}(\Theta) = \frac{1}{n}\sum_{i=1}^n H(\pmb{y}^{(i)},\hat{\pmb{y}}^{(i)})
l(Θ)=n1?i=1∑n?H(y?y??y(i),y?y??y^?(i)) 其中
Θ
\Theta
Θ 是模型参数,如果每个样本只有一个标签,则上述损失可以化简为
l
(
Θ
)
=
1
n
∑
i
=
1
n
?
log
?
y
^
y
(
i
)
(
i
)
\mathscr{l}(\Theta) = \frac{1}{n}\sum_{i=1}^n -\log\hat{y}_{y^{(i)}}^{(i)}
l(Θ)=n1?i=1∑n??logy^?y(i)(i)? 最小化
l
(
Θ
)
\mathscr{l}(\Theta)
l(Θ) 等价于最大化
exp
?
(
?
n
l
(
Θ
)
)
=
∏
i
=
1
n
y
^
y
(
i
)
(
i
)
\exp(-n\mathscr{l}(\Theta)) = \prod_{i=1}^n \hat{y}_{y^{(i)}}^{(i)}
exp(?nl(Θ))=∏i=1n?y^?y(i)(i)?,即最小化交叉熵损失函数等价于最大化训练数据集所有标签类别的联合预测概率
1.4 模型预测与评价
- 训练好 softmax 回归模型后,给定任一样本特征,就可以预测每个输出类别的概率
- 通常把预测概率最大的类别作为输出类别,如果它与真实类别(标签)一致,说明这次预测是正确
- 对于分类问题,可以使用
准确率accuracy 来评价模型的表现,它等于正确预测数量与总预测数量之比
2. 实现 softmax 回归
2.1 数据准备
-
使用 Fashion-MNIST 图像分类数据集进行试验,该数据集可以使用 torchvision.datasets 方便地获取和使用,具体请参考:在 pytorch 中加载和使用图像分类数据集 Fashion-MNIST -
先定义好读取小批量数据的方法,构造数据读取迭代器 import torch
import torchvision
import torchvision.transforms as transforms
import numpy as np
def load_data_fashion_mnist(batch_size, num_workers=0, root='./Datasets/FashionMNIST'):
mnist_train = torchvision.datasets.FashionMNIST(root=root, train=True, download=True,transform=transforms.ToTensor())
mnist_test = torchvision.datasets.FashionMNIST(root=root, train=False, download=True,transform=transforms.ToTensor())
train_iter = torch.utils.data.DataLoader(mnist_train, batch_size=batch_size, shuffle=True, num_workers=num_workers)
test_iter = torch.utils.data.DataLoader(mnist_test, batch_size=batch_size, shuffle=False, num_workers=num_workers)
return train_iter, test_iter
batch_size = 256
train_iter, test_iter = load_data_fashion_mnist(batch_size, 4)
2.2 模型设计
-
模型参数初始化:输入图像样本尺寸均为 28x28,拉平后输入向量长度为
28
×
28
=
784
28\times 28 = 784
28×28=784;由于图像有 10 个类别,输出层输出向量尺寸为 10,因此权重参数尺寸为
W
728
×
10
\pmb{W}_{728\times 10}
WWW728×10?,偏置参数尺寸为
b
1
×
10
\pmb{b}_{1\times 10}
bbb1×10?,如下初始化
-
w
i
j
~
N
(
0
,
0.0
1
2
)
,
?
i
=
1
,
2
,
.
.
.
,
728
;
j
=
1
,
2
,
.
.
.
,
10
w_{ij}\sim N(0,0.01^2),\space i=1,2,...,728;j=1,2,...,10
wij?~N(0,0.012),?i=1,2,...,728;j=1,2,...,10
-
b
i
=
0
,
?
i
=
1
,
2
,
.
.
.
,
10
b_i=0,\space i=1,2,...,10
bi?=0,?i=1,2,...,10
注意设置属性 requires_grad = True ,这样在后续训练过程中才能对这些参数求梯度并迭代更新参数值
num_inputs = 28*28
num_outputs = 10
W = torch.tensor(np.random.normal(0, 0.01, (num_inputs, num_outputs)), dtype=torch.float, requires_grad=True)
b = torch.zeros(num_outputs, dtype=torch.float, requires_grad=True)
-
实现 softmax 运算:下面实参 O 是行数为样本数,列数为输出个数的矩阵,即
O
n
×
10
=
X
n
×
728
W
728
×
10
+
b
1
×
10
\pmb{O}_{n\times 10} = \pmb{X}_{n\times 728}\pmb{W}_{728\times 10}+\pmb{b}_{1\times 10}
OOOn×10?=XXXn×728?WWW728×10?+bbb1×10? (最后加向量
b
\pmb{b}
bbb 使用了广播机制)。首先调用 .exp() 对矩阵中所有元素求 exp 函数值,然后按列求和得到
728
×
1
728\times 1
728×1 的中间向量,最后利用广播机制将每一个元素除以其所在行的中间元素。这样处理后得到的矩阵每行元素和为1且非负,成为合法的概率分布,代表各个样本在各个输出类别上的预测概率 def softmax(O):
O_exp = O.exp()
partition = O_exp.sum(dim=1, keepdim=True)
return O_exp / partition
测试一下,假设类别数为 5,样本数为 2
output = torch.rand((2, 5))
y_hat = softmax(output)
print(y_hat, y_hat.sum(dim=1))
'''
tensor([[0.3455, 0.1642, 0.1698, 0.1335, 0.1870],
[0.1873, 0.2624, 0.2497, 0.1375, 0.1631]]) tensor([1., 1.])
'''
y = torch.tensor([0,2])
print(y_hat.gather(1, y.view(-1, 1)))
'''
tensor([[0.3455],
[0.2497]])
'''
-
定义模型
Y
^
=
softmax
(
X
W
+
b
)
\pmb{\hat{Y}} = \text{softmax}(\pmb{X}\pmb{W}+\pmb{b})
Y^Y^Y^=softmax(XXXWWW+bbb) def net(X):
return softmax(torch.mm(X.view((-1, num_inputs)), W) + b)
-
定义损失函数
H
(
y
(
i
)
,
y
^
(
i
)
)
=
?
log
?
y
^
y
(
i
)
(
i
)
H(\pmb{y}^{(i)},\hat{\pmb{y}}^{(i)}) = -\log\hat{y}_{y^{(i)}}^{(i)}
H(y?y??y(i),y?y??y^?(i))=?logy^?y(i)(i)? def cross_entropy(y_hat, y):
return - torch.log(y_hat.gather(1, y.view(-1, 1)))
2.3 模型评价
2.4 模型训练
2.4.1 优化算法
2.4.2 训练流程
- 训练流程和线性回归类似
- 设定超参数
num_epochs (迭代次数)和 lr (学习率) - 在每轮迭代中逐小批次地遍历训练集,计算损失 -> 对参数求梯度 -> 做小批量随机梯度下降优化参数
- 训练程序如下
num_epochs, lr = 5, 0.1
def train(net, train_iter, test_iter, loss, num_epochs, batch_size, params=None, lr=None):
for epoch in range(num_epochs):
train_l_sum = 0.0
train_acc_sum = 0.0
n = 0
for X, y in train_iter:
y_hat = net(X)
l = loss(y_hat, y).sum()
if params is not None and params[0].grad is not None:
for param in params:
param.grad.data.zero_()
l.backward()
sgd(params, lr, batch_size)
train_l_sum += l.item()
train_acc_sum += (y_hat.argmax(dim=1) == y).sum().item()
n += y.shape[0]
test_acc = evaluate_accuracy(test_iter, net)
print('epoch %d, loss %.4f, train acc %.3f, test acc %.3f'
% (epoch + 1, train_l_sum / n, train_acc_sum / n, test_acc))
train(net, train_iter, test_iter, cross_entropy, num_epochs, batch_size, [W, b], lr)
'''
epoch 1, loss 0.4741, train acc 0.840, test acc 0.827
epoch 2, loss 0.4649, train acc 0.842, test acc 0.832
epoch 3, loss 0.4579, train acc 0.845, test acc 0.833
epoch 4, loss 0.4520, train acc 0.847, test acc 0.835
epoch 5, loss 0.4463, train acc 0.849, test acc 0.830
'''
2.5 使用模型进行预测
-
训练完成后就可以用模型对测试图像进行分类了,先定义一些显示结果使用的工具函数 from IPython import display
import matplotlib.pyplot as plt
def get_fashion_mnist_labels(labels):
text_labels = ['t-shirt', 'trouser', 'pullover', 'dress', 'coat',
'sandal', 'shirt', 'sneaker', 'bag', 'ankle boot']
return [text_labels[int(i)] for i in labels]
def show_fashion_mnist(images, labels):
display.set_matplotlib_formats('svg')
_, figs = plt.subplots(1, len(images), figsize=(12, 12))
for f, img, lbl in zip(figs, images, labels):
f.imshow(img.view((28, 28)).numpy())
f.set_title(lbl)
f.axes.get_xaxis().set_visible(False)
f.axes.get_yaxis().set_visible(False)
-
下面给定一系列图像,真实标签和模型预测结果分别显示在第一和第二行 X, y = iter(test_iter).next()
true_labels = get_fashion_mnist_labels(y.numpy())
pred_labels = get_fashion_mnist_labels(net(X).argmax(dim=1).numpy())
titles = [true + '\n' + pred for true, pred in zip(true_labels, pred_labels)]
show_fashion_mnist(X[0:9], titles[0:9])
预测结果如下 
2.6 完整代码
- 整合上述过程,给出完整代码,可以直接粘贴进 vscode 运行
import torch
import torchvision
import torchvision.transforms as transforms
import numpy as np
from IPython import display
import matplotlib.pyplot as plt
def load_data_fashion_mnist(batch_size, num_workers=0, root='./Datasets/FashionMNIST'):
mnist_train = torchvision.datasets.FashionMNIST(root=root, train=True, download=True,transform=transforms.ToTensor())
mnist_test = torchvision.datasets.FashionMNIST(root=root, train=False, download=True,transform=transforms.ToTensor())
train_iter = torch.utils.data.DataLoader(mnist_train, batch_size=batch_size, shuffle=True, num_workers=num_workers)
test_iter = torch.utils.data.DataLoader(mnist_test, batch_size=batch_size, shuffle=False, num_workers=num_workers)
return train_iter, test_iter
def get_fashion_mnist_labels(labels):
text_labels = ['t-shirt', 'trouser', 'pullover', 'dress', 'coat',
'sandal', 'shirt', 'sneaker', 'bag', 'ankle boot']
return [text_labels[int(i)] for i in labels]
def show_fashion_mnist(images, labels):
display.set_matplotlib_formats('svg')
_, figs = plt.subplots(1, len(images), figsize=(12, 12))
for f, img, lbl in zip(figs, images, labels):
f.imshow(img.view((28, 28)).numpy())
f.set_title(lbl)
f.axes.get_xaxis().set_visible(False)
f.axes.get_yaxis().set_visible(False)
def softmax(O):
O_exp = O.exp()
partition = O_exp.sum(dim=1, keepdim=True)
return O_exp / partition
def net(X):
return softmax(torch.mm(X.view((-1, num_inputs)), W) + b)
def cross_entropy(y_hat, y):
return - torch.log(y_hat.gather(1, y.view(-1, 1)))
def sgd(params, lr, batch_size):
for param in params:
param.data -= lr * param.grad / batch_size
def evaluate_accuracy(data_iter, net):
acc_sum = 0.0
n = 0
for X, y in data_iter:
acc_sum += (net(X).argmax(dim=1) == y).float().sum().item()
n += y.shape[0]
return acc_sum / n
def train(net, train_iter, test_iter, loss, num_epochs, batch_size, params=None, lr=None):
for epoch in range(num_epochs):
train_l_sum = 0.0
train_acc_sum = 0.0
n = 0
for X, y in train_iter:
y_hat = net(X)
l = loss(y_hat, y).sum()
if params is not None and params[0].grad is not None:
for param in params:
param.grad.data.zero_()
l.backward()
sgd(params, lr, batch_size)
train_l_sum += l.item()
train_acc_sum += (y_hat.argmax(dim=1) == y).sum().item()
n += y.shape[0]
test_acc = evaluate_accuracy(test_iter, net)
print('epoch %d, loss %.4f, train acc %.3f, test acc %.3f'
% (epoch + 1, train_l_sum / n, train_acc_sum / n, test_acc))
if __name__ == '__main__':
num_inputs,num_outputs = 28*28,10
W = torch.tensor(np.random.normal(0, 0.01, (num_inputs, num_outputs)), dtype=torch.float, requires_grad=True)
b = torch.zeros(num_outputs, dtype=torch.float, requires_grad=True)
num_epochs, lr = 5, 0.1
batch_size = 256
train_iter, test_iter = load_data_fashion_mnist(batch_size, 4)
train(net, train_iter, test_iter, cross_entropy, num_epochs, batch_size, [W, b], lr)
X, y = iter(test_iter).next()
true_labels = get_fashion_mnist_labels(y.numpy())
pred_labels = get_fashion_mnist_labels(net(X).argmax(dim=1).numpy())
titles = [true + '\n' + pred for true, pred in zip(true_labels, pred_labels)]
show_fashion_mnist(X[0:9], titles[0:9])
plt.show()
3. 利用 Pytorch 简洁地实现 softmax 回归
- pytorch 中提供了大量预定义的神经网络层,常用损失函数及优化器,可以大大简化 softmax 回归模型的实现
- 数据准备、模型评价、使用模型进行预测三部分和第 2 节实现相同,本节不再重复
3.1 模型设计
3.1.2 模型定义
-
如 1.2 节所示,softmax 回归的输出是一个全连接层,可以使用 torch.nn.Linear 方法定义,如下 num_inputs = 28*28
num_outputs = 10
class LinearNet(nn.Module):
def __init__(self, num_inputs, num_outputs):
super(LinearNet, self).__init__()
self.linear = nn.Linear(num_inputs, num_outputs)
def forward(self, x):
y = self.linear(x.view(x.shape[0], -1))
return y
net = LinearNet(num_inputs, num_outputs)
注意到原始图像尺寸为 torch.Size([1, 28, 28]) ,数据迭代器返回的 batch x 尺寸为 (batch_size, 1, 28, 28),在做前向传播时,必须要把样本都拉平,即把 x 的形状转换为 (batch_size, 1x28x28) 才能送入全连接层 -
按照深度学习的习惯,可以把数据拉平这件事定义成神经网络的一个层,如下 class FlattenLayer(nn.Module):
def __init__(self):
super(FlattenLayer, self).__init__()
def forward(self, x):
return x.view(x.shape[0], -1)
这样就能更符合习惯地,利用 Sequential 容器搭建网络模型 from collections import OrderedDict
net = nn.Sequential(
OrderedDict([
('flatten', FlattenLayer()),
('linear', nn.Linear(num_inputs, num_outputs))
])
)
3.1.2 模型初始化
- 利用
torch.nn.init 包提供的方法进行初始化,初始化值同 2.2 节nn.init.normal_(net.linear.weight, mean=0, std=0.01)
nn.init.constant_(net.linear.bias, val=0)
'''
Parameter containing:
tensor([0., 0., 0., 0., 0., 0., 0., 0., 0., 0.], requires_grad=True)
'''
3.1.3 softmax 和交叉熵损失函数
-
第 2 节中我们按照数学定义分别定义了 softmax 函数和 CrossEntropy 损失,这样做可能导致数据不稳定
- softmax 函数定义为
y
^
i
=
exp
?
(
o
i
)
∑
j
exp
?
(
o
j
)
\hat{y}_i = \frac{\exp(o_i)}{\sum_j \exp(o_j)}
y^?i?=∑j?exp(oj?)exp(oi?)?注意这里都是 exp 运算,一旦网络初始化不当,或输入数值有较大噪音,很可能导致数值溢出(
o
o
o 是很大的正整数会导致上溢,
o
o
o 是很大的负整数会导致下溢),这时可以用 Log-Sum-Exp Trick(logSoftmax)处理,它在数学上就是在普通 softmax 外面套了一个 log 函数,这不会影响概率排序,但是通过有技巧地实现可以有效解决溢出问题。具体参考 深入理解softmax
- CrossEntropy损失在这里为
H
(
y
(
i
)
,
y
^
(
i
)
)
=
?
∑
j
=
1
q
y
j
(
i
)
log
?
y
^
j
(
i
)
=
?
log
?
y
^
y
(
i
)
(
i
)
H(\pmb{y}^{(i)},\hat{\pmb{y}}^{(i)}) = -\sum_{j=1}^q y_j^{(i)}\log\hat{y}_j^{(i)} = -\log\hat{y}_{y^{(i)}}^{(i)}
H(y?y??y(i),y?y??y^?(i))=?j=1∑q?yj(i)?logy^?j(i)?=?logy^?y(i)(i)? 当
y
^
y
(
i
)
(
i
)
\hat{y}_{y^{(i)}}^{(i)}
y^?y(i)(i)? 太小(趋于0)时也可能溢出。观察发现其实这里就是 logSoftmax 取了个负号,所以用了 Log-Sum-Exp 技巧后这里的就不用担心溢出了。注意到整个数据集上的损失为
l
(
Θ
)
=
1
n
∑
i
=
1
n
?
log
?
y
^
y
(
i
)
(
i
)
\mathscr{l}(\Theta) = \frac{1}{n}\sum_{i=1}^n -\log\hat{y}_{y^{(i)}}^{(i)}
l(Θ)=n1?i=1∑n??logy^?y(i)(i)? 如果已经用 logSoftmax计算了各个
log
?
y
^
y
(
i
)
(
i
)
\log\hat{y}_{y^{(i)}}^{(i)}
logy^?y(i)(i)?,可以直接用负对数似然方法
torch.NLLLoss 方法得到
l
(
Θ
)
\mathscr{l}(\Theta)
l(Θ)。NLLLosss 的具体行为可以参考 loss函数之NLLLoss,CrossEntropyLoss -
总之,我们可以用 logSoftmax + NLLLoss 避免数据溢出,保证数据稳定性,并且得到等价的交叉熵损失,pytorch 中直接把这两个放在一起封装了一个 nn.CrossEntropyLoss 方法,对于一组小批量数据,假设模型输出和真实标签分别为
o
u
t
p
u
t
output
output 和
t
r
u
t
h
truth
truth, nn.CrossEntropyLoss 如下计算小批量损失
CrossEntropyLoss
(
o
u
t
p
u
t
,
t
r
u
t
h
)
=
NLLLoss
(
logSoftmax
(
o
u
t
p
u
t
)
,
t
r
u
t
h
)
\text{CrossEntropyLoss}(output,truth) = \text{NLLLoss}(\text{logSoftmax}(output),truth)
CrossEntropyLoss(output,truth)=NLLLoss(logSoftmax(output),truth) 在这里我们直接使用它来替代前面自己定义的 softmax 函数和 CrossEntropy 损失。可参考 Pytorch中Softmax、Log_Softmax、NLLLoss以及CrossEntropyLoss的关系与区别详解 loss = nn.CrossEntropyLoss()
3.2 模型训练
3.2.1 优化器
3.2.2 训练流程
- 和 2.4.2 节完全类似,训练程序只须改一下优化器部分的处理即可
num_epochs = 5
def train(net, train_iter, test_iter, loss, num_epochs, batch_size, params=None, lr=None, optimizer=None):
for epoch in range(num_epochs):
train_l_sum = 0.0
train_acc_sum = 0.0
n = 0
for X, y in train_iter:
y_hat = net(X)
l = loss(y_hat, y).sum()
optimizer.zero_grad()
l.backward()
optimizer.step()
train_l_sum += l.item()
train_acc_sum += (y_hat.argmax(dim=1) == y).sum().item()
n += y.shape[0]
test_acc = evaluate_accuracy(test_iter, net)
print('epoch %d, loss %.4f, train acc %.3f, test acc %.3f'
% (epoch + 1, train_l_sum / n, train_acc_sum / n, test_acc))
train(net, train_iter, test_iter, loss, num_epochs, batch_size, None, None, optimizer)
'''
epoch 1, loss 0.0021, train acc 0.826, test acc 0.816
epoch 2, loss 0.0020, train acc 0.833, test acc 0.822
epoch 3, loss 0.0019, train acc 0.836, test acc 0.823
epoch 4, loss 0.0019, train acc 0.840, test acc 0.827
epoch 5, loss 0.0018, train acc 0.843, test acc 0.829
'''
3.3 完整代码
-
整合上述过程,给出完整代码,可以直接粘贴进 vscode 运行 import torch
from torch import nn
import torchvision
import torchvision.transforms as transforms
import numpy as np
from IPython import display
from collections import OrderedDict
import matplotlib.pyplot as plt
def load_data_fashion_mnist(batch_size, num_workers=0, root='./Datasets/FashionMNIST'):
mnist_train = torchvision.datasets.FashionMNIST(root=root, train=True, download=True,transform=transforms.ToTensor())
mnist_test = torchvision.datasets.FashionMNIST(root=root, train=False, download=True,transform=transforms.ToTensor())
train_iter = torch.utils.data.DataLoader(mnist_train, batch_size=batch_size, shuffle=True, num_workers=num_workers)
test_iter = torch.utils.data.DataLoader(mnist_test, batch_size=batch_size, shuffle=False, num_workers=num_workers)
return train_iter, test_iter
def get_fashion_mnist_labels(labels):
text_labels = ['t-shirt', 'trouser', 'pullover', 'dress', 'coat',
'sandal', 'shirt', 'sneaker', 'bag', 'ankle boot']
return [text_labels[int(i)] for i in labels]
def show_fashion_mnist(images, labels):
display.set_matplotlib_formats('svg')
_, figs = plt.subplots(1, len(images), figsize=(12, 12))
for f, img, lbl in zip(figs, images, labels):
f.imshow(img.view((28, 28)).numpy())
f.set_title(lbl)
f.axes.get_xaxis().set_visible(False)
f.axes.get_yaxis().set_visible(False)
class FlattenLayer(nn.Module):
def __init__(self):
super(FlattenLayer, self).__init__()
def forward(self, x):
return x.view(x.shape[0], -1)
def evaluate_accuracy(data_iter, net):
acc_sum = 0.0
n = 0
for X, y in data_iter:
acc_sum += (net(X).argmax(dim=1) == y).float().sum().item()
n += y.shape[0]
return acc_sum / n
def train(net, train_iter, test_iter, loss, num_epochs, batch_size, params=None, lr=None, optimizer=None):
for epoch in range(num_epochs):
train_l_sum = 0.0
train_acc_sum = 0.0
n = 0
for X, y in train_iter:
y_hat = net(X)
l = loss(y_hat, y).sum()
optimizer.zero_grad()
l.backward()
optimizer.step()
train_l_sum += l.item()
train_acc_sum += (y_hat.argmax(dim=1) == y).sum().item()
n += y.shape[0]
test_acc = evaluate_accuracy(test_iter, net)
print('epoch %d, loss %.4f, train acc %.3f, test acc %.3f'
% (epoch + 1, train_l_sum / n, train_acc_sum / n, test_acc))
if __name__ == '__main__':
num_inputs,num_outputs = 28*28,10
num_epochs,lr = 5,0.1
batch_size = 256
train_iter, test_iter = load_data_fashion_mnist(batch_size, 4)
net = nn.Sequential(
OrderedDict([
('flatten', FlattenLayer()),
('linear', nn.Linear(num_inputs, num_outputs))
])
)
nn.init.normal_(net.linear.weight, mean=0, std=0.01)
nn.init.constant_(net.linear.bias, val=0)
loss = nn.CrossEntropyLoss()
optimizer = torch.optim.SGD(net.parameters(), lr=lr)
train(net, train_iter, test_iter, loss, num_epochs, batch_size, None, None, optimizer)
X, y = iter(test_iter).next()
true_labels = get_fashion_mnist_labels(y.numpy())
pred_labels = get_fashion_mnist_labels(net(X).argmax(dim=1).numpy())
titles = [true + '\n' + pred for true, pred in zip(true_labels, pred_labels)]
show_fashion_mnist(X[0:9], titles[0:9])
plt.show()
|