?tf.train.Feature.?https://www.tensorflow.org/api_docs/python/tf/train/Feature?
?tf.train.Example?https://www.tensorflow.org/api_docs/python/tf/train/Example
tf.io.parse_example??tf.io.parse_example ?|? TensorFlow Core v2.8.0?
?tf.RaggedTensor?
int_feature = tf.train.Feature(
int64_list=tf.train.Int64List(value=[1, 2, 3, 4]))
float_feature = tf.train.Feature(
float_list=tf.train.FloatList(value=[1., 2., 3., 4.]))
bytes_feature = tf.train.Feature(
bytes_list=tf.train.BytesList(value=[b"abc", b"1234"]))
example = tf.train.Example(
features=tf.train.Features(feature={
'my_ints': int_feature,
'my_floats': float_feature,
'my_bytes': bytes_feature,
}))
tf.io.parse_example(
example.SerializeToString(),
features = {
'my_ints': tf.io.RaggedFeature(dtype=tf.int64),
'my_floats': tf.io.RaggedFeature(dtype=tf.float32),
'my_bytes': tf.io.RaggedFeature(dtype=tf.string)})
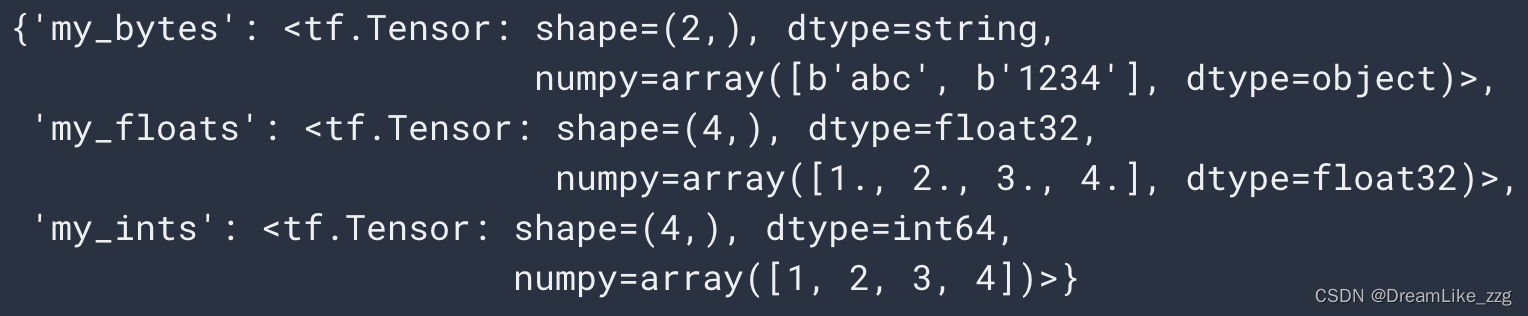
???tf.Example.?https://www.tensorflow.org/tutorials/load_data/tfrecord?hl=zh-cn
# The number of observations in the dataset.
n_observations = int(1e4)
# Boolean feature, encoded as False or True.
feature0 = np.random.choice([False, True], n_observations)
# Integer feature, random from 0 to 4.
feature1 = np.random.randint(0, 5, n_observations)
# String feature
strings = np.array([b'cat', b'dog', b'chicken', b'horse', b'goat'])
feature2 = strings[feature1]
# Float feature, from a standard normal distribution
feature3 = np.random.randn(n_observations)
def serialize_example(feature0, feature1, feature2, feature3):
"""
Creates a tf.Example message ready to be written to a file.
"""
# Create a dictionary mapping the feature name to the tf.Example-compatible
# data type.
feature = {
'feature0': _int64_feature(feature0),
'feature1': _int64_feature(feature1),
'feature2': _bytes_feature(feature2),
'feature3': _float_feature(feature3),
}
# Create a Features message using tf.train.Example.
example_proto = tf.train.Example(features=tf.train.Features(feature=feature))
return example_proto.SerializeToString()
make_parse_example_spec?
# Define features and transformations
feature_a = tf.feature_column.categorical_column_with_vocabulary_file(...)
feature_b = tf.feature_column.numeric_column(...)
feature_c_bucketized = tf.feature_column.bucketized_column(
tf.feature_column.numeric_column("feature_c"), ...)
feature_a_x_feature_c = tf.feature_column.crossed_column(
columns=["feature_a", feature_c_bucketized], ...)
feature_columns = set(
[feature_b, feature_c_bucketized, feature_a_x_feature_c])
features = tf.io.parse_example(
serialized=serialized_examples,
features=tf.feature_column.make_parse_example_spec(feature_columns))
# For the above example, make_parse_example_spec would return the dict:
{
"feature_a": parsing_ops.VarLenFeature(tf.string),
"feature_b": parsing_ops.FixedLenFeature([1], dtype=tf.float32),
"feature_c": parsing_ops.FixedLenFeature([1], dtype=tf.float32)
}
|