(相对)位置编码
Stand-Alone Self-Attention in Vision Models-NIPS 2019
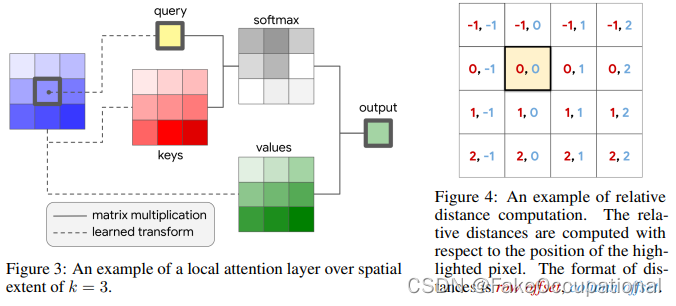
[网络主要结构](https://blog.csdn.net/baigalxy/article/details/93662282)
[Stand-Alone Self-Attention in Vision Models](https://zhuanlan.zhihu.com/p/361259616)
[paer](https://papers.nips.cc/paper/2019/file/3416a75f4cea9109507cacd8e2f2aefc-Paper.pdf)
[现在transformer有什么工作有用二维的位置编码吗?](https://www.zhihu.com/question/476052139)
[实验分析非常精彩 | Transformer中的位置嵌入到底改如何看待?](http://www.360doc.com/content/22/0209/13/99071_1016566082.shtml)
Self-Attention with Relative Position Representations-NAACL 2018
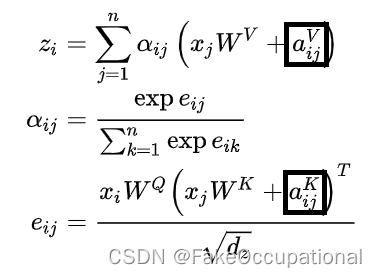
[Self-Attention with Relative Position Representations](https://www.jianshu.com/p/cb5b2d967e90)
Rethinking and Improving Relative Position Encoding for Vision Transformer-2019
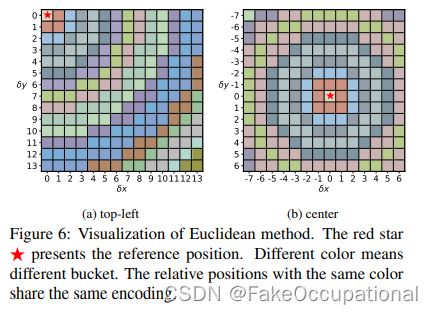 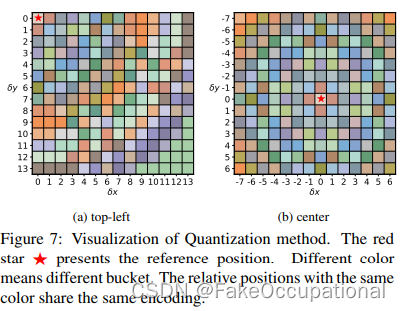 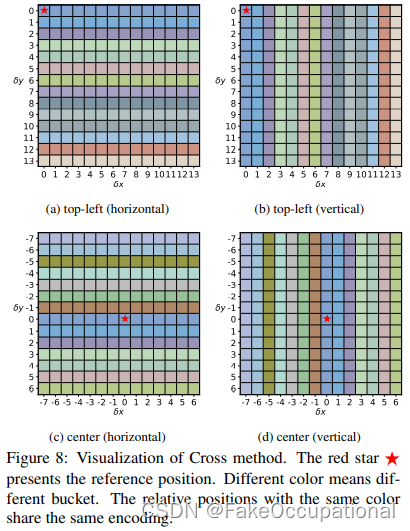 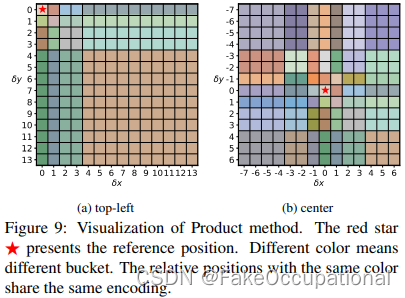
[paper](https://houwenpeng.com/publications/iRPE.pdf)
[论文阅读:本篇论文提出了四种二维相对位置编码的映射方式,同时分析了Vision Transformer中影响二维相对位置性能的关键因素](https://baijiahao.baidu.com/s?id=1706147979229455598&wfr=spider&for=pc)
二维位置的旋转式位置编码
[科学空间](https://spaces.ac.cn/archives/8265)
[Transformer升级之路:二维位置的旋转式位置编码](https://www.sohu.com/a/482611838_121119001)
[git](https://github.com/ZhuiyiTechnology/roformer)
[A PyTorch implementation of the 1d and 2d Sinusoidal positional encoding/embedding.](https://github.com/wzlxjtu/PositionalEncoding2D)
https://pypi.org/project/positional-encodings/中:
1D, 2D, and 3D Sinusoidal Postional Encoding (Pytorch and Tensorflow)
An implementation of 1D, 2D, and 3D positional encoding in Pytorch and TensorFlow
pip install positional-encodings
import torch
from positional_encodings import PositionalEncoding1D, PositionalEncoding2D, PositionalEncoding3D
p_enc_1d_model = PositionalEncoding1D(10)
p_enc_1d_model_sum = Summer(PositionalEncoding1D(10))
x = torch.rand(1,6,10)
penc_no_sum = p_enc_1d_model(x)
penc_sum = p_enc_1d_model_sum(x)
print(penc_no_sum + x == penc_sum)
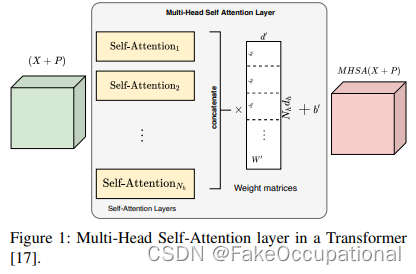
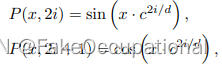
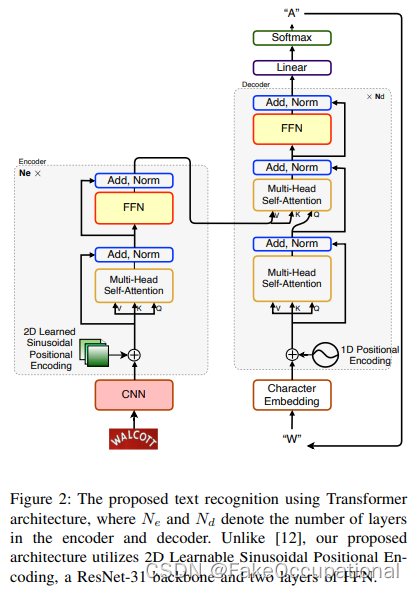
|