1、VAE
参考:https://blog.csdn.net/hester_hester/article/details/105790530 
import os
import torch
import torch.nn as nn
import torch.nn.functional as F
import torchvision
from torchvision import transforms
from torchvision.utils import save_image
# 配置GPU或CPU设置
# device = torch.device('cuda' if torch.cuda.is_available() else 'cpu')
device='cpu'
# 创建目录保存生成的图片
sample_dir = 'samples'
if not os.path.exists(sample_dir):
os.makedirs(sample_dir)
# 超参数设置
image_size = 784 #图片大小
h_dim = 400
z_dim = 20
num_epochs = 15 #15个循环
batch_size = 128 #一批的数量
learning_rate = 1e-3 #学习率
# 获取数据集
dataset = torchvision.datasets.MNIST(root='./data',
train=True,
transform=transforms.ToTensor(),
download=True)
# 数据加载,按照batch_size大小加载,并随机打乱
data_loader = torch.utils.data.DataLoader(dataset=dataset,
batch_size=batch_size,
shuffle=True)
# VAE模型
class VAE(nn.Module):
def __init__(self, image_size=784, h_dim=400, z_dim=20):
super(VAE, self).__init__()
self.fc1 = nn.Linear(image_size, h_dim)
self.fc2 = nn.Linear(h_dim, z_dim)
self.fc3 = nn.Linear(h_dim, z_dim)
self.fc4 = nn.Linear(z_dim, h_dim)
self.fc5 = nn.Linear(h_dim, image_size)
# 编码,学习高斯分布均值与方差
def encode(self, x):
h = F.relu(self.fc1(x))
return self.fc2(h), self.fc3(h)
# 将高斯分布均值与方差参数重表示,生成隐变量z 若x~N(mu, var*var)分布,则(x-mu)/var=z~N(0, 1)分布
def reparameterize(self, mu, log_var):
std = torch.exp(log_var / 2)
eps = torch.randn_like(std)
return mu + eps * std
# 解码隐变量z
def decode(self, z):
h = F.relu(self.fc4(z))
return F.sigmoid(self.fc5(h))
# 计算重构值和隐变量z的分布参数
def forward(self, x):
mu, log_var = self.encode(x) # 从原始样本x中学习隐变量z的分布,即学习服从高斯分布均值与方差
z = self.reparameterize(mu, log_var) # 将高斯分布均值与方差参数重表示,生成隐变量z
x_reconst = self.decode(z) # 解码隐变量z,生成重构x’
return x_reconst, mu, log_var # 返回重构值和隐变量的分布参数
# 构造VAE实例对象
model = VAE().to(device)
print(model)
"""VAE(
(fc1): Linear(in_features=784, out_features=400, bias=True)
(fc2): Linear(in_features=400, out_features=20, bias=True)
(fc3): Linear(in_features=400, out_features=20, bias=True)
(fc4): Linear(in_features=20, out_features=400, bias=True)
(fc5): Linear(in_features=400, out_features=784, bias=True)
)"""
# 选择优化器,并传入VAE模型参数和学习率
optimizer = torch.optim.Adam(model.parameters(), lr=learning_rate)
# 开始训练一共15个循环
for epoch in range(num_epochs):
for i, (x, _) in enumerate(data_loader):
# 前向传播
x = x.to(device).view(-1,image_size) # 将batch_size*1*28*28 ---->batch_size*image_size 其中,image_size=1*28*28=784
x_reconst, mu, log_var = model(x) # 将batch_size*748的x输入模型进行前向传播计算,重构值和服从高斯分布的隐变量z的分布参数(均值和方差)
# 计算重构损失和KL散度
# 重构损失
reconst_loss = F.binary_cross_entropy(x_reconst, x, size_average=False)
# KL散度
kl_div = - 0.5 * torch.sum(1 + log_var - mu.pow(2) - log_var.exp())
# 反向传播与优化
# 计算误差(重构误差和KL散度值)
loss = reconst_loss + kl_div
# 清空上一步的残余更新参数值
optimizer.zero_grad()
# 误差反向传播, 计算参数更新值
loss.backward()
# 将参数更新值施加到VAE model的parameters上
optimizer.step()
# 每迭代一定步骤,打印结果值
if (i + 1) % 10 == 0:
print("Epoch[{}/{}], Step [{}/{}], Reconst Loss: {:.4f}, KL Div: {:.4f}"
.format(epoch + 1, num_epochs, i + 1, len(data_loader), reconst_loss.item(), kl_div.item()))
with torch.no_grad():
# 保存采样值
# 生成随机数 z
z = torch.randn(batch_size, z_dim).to(device) # z的大小为batch_size * z_dim = 128*20
# 对随机数 z 进行解码decode输出
out = model.decode(z).view(-1, 1, 28, 28)
# 保存结果值
save_image(out, os.path.join(sample_dir, 'sampled-{}.png'.format(epoch + 1)))
# 保存重构值
# 将batch_size*748的x输入模型进行前向传播计算,获取重构值out
out, _, _ = model(x)
# 将输入与输出拼接在一起输出保存 batch_size*1*28*(28+28)=batch_size*1*28*56
x_concat = torch.cat([x.view(-1, 1, 28, 28), out.view(-1, 1, 28, 28)], dim=3)
save_image(x_concat, os.path.join(sample_dir, 'reconst-{}.png'.format(epoch + 1)))
***第一批和15批的效果,图片中输入和重建数字在一块 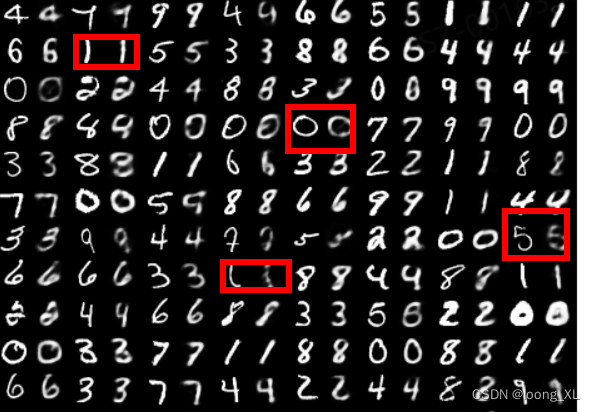
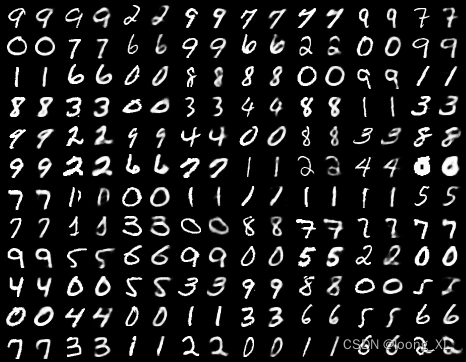
2、VQVAE
参考:https://zhuanlan.zhihu.com/p/467507030 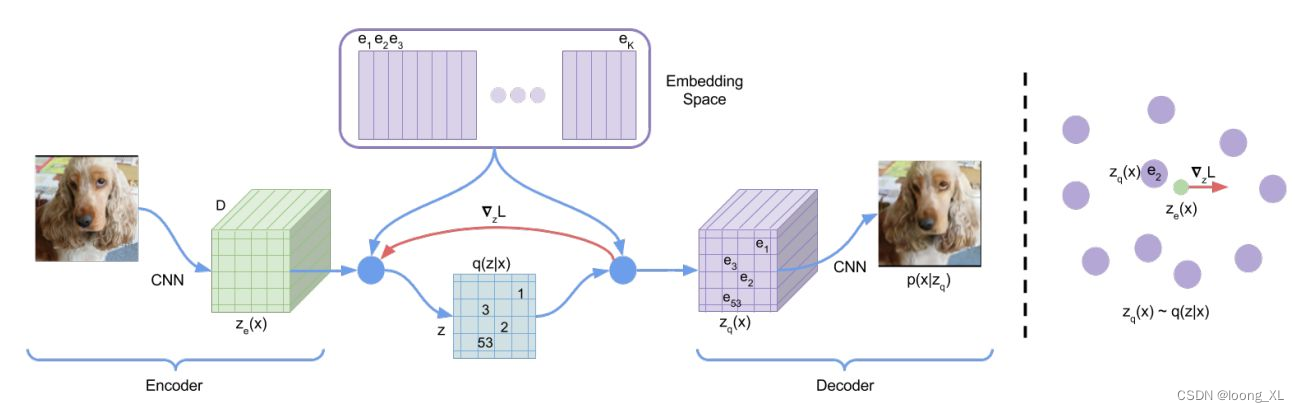
代码
import torch
import torch.nn as nn
from torch import Tensor
from typing import List, Callable, Union, Any, TypeVar
import torch.nn.functional as F
from abc import abstractmethod
class BaseVAE(nn.Module):
def __init__(self) -> None:
super(BaseVAE, self).__init__()
def encode(self, input: Tensor) -> List[Tensor]:
raise NotImplementedError
def decode(self, input: Tensor) -> Any:
raise NotImplementedError
def sample(self, batch_size:int) -> Tensor:
raise NotImplementedError
def generate(self, x: Tensor, **kwargs) -> Tensor:
raise NotImplementedError
@abstractmethod
def forward(self, *inputs: Tensor) -> Tensor:
pass
@abstractmethod
def loss_function(self, *inputs: Any, **kwargs) -> Tensor:
pass
class VectorQuantizer(nn.Module):
"""
Reference:
[1] https://github.com/deepmind/sonnet/blob/v2/sonnet/src/nets/vqvae.py
"""
def __init__(self,
num_embeddings: int,
embedding_dim: int,
beta: float = 0.25):
super(VectorQuantizer, self).__init__()
self.K = num_embeddings
self.D = embedding_dim
self.beta = beta
self.embedding = nn.Embedding(self.K, self.D)
self.embedding.weight.data.uniform_(-1 / self.K, 1 / self.K)
def forward(self, latents: Tensor) -> Tensor:
latents = latents.permute(0, 2, 3, 1).contiguous() # [B x D x H x W] -> [B x H x W x D]
latents_shape = latents.shape
flat_latents = latents.view(-1, self.D) # [BHW x D]
# Compute L2 distance between latents and embedding weights
dist = torch.sum(flat_latents ** 2, dim=1, keepdim=True) + \
torch.sum(self.embedding.weight ** 2, dim=1) - \
2 * torch.matmul(flat_latents, self.embedding.weight.t()) # [BHW x K]
# Get the encoding that has the min distance
encoding_inds = torch.argmin(dist, dim=1).unsqueeze(1) # [BHW, 1]
# Convert to one-hot encodings
device = latents.device
encoding_one_hot = torch.zeros(encoding_inds.size(0), self.K, device=device)
encoding_one_hot.scatter_(1, encoding_inds, 1) # [BHW x K]
# Quantize the latents
quantized_latents = torch.matmul(encoding_one_hot, self.embedding.weight) # [BHW, D]
quantized_latents = quantized_latents.view(latents_shape) # [B x H x W x D]
# Compute the VQ Losses
commitment_loss = F.mse_loss(quantized_latents.detach(), latents)
embedding_loss = F.mse_loss(quantized_latents, latents.detach())
vq_loss = commitment_loss * self.beta + embedding_loss
# Add the residue back to the latents
quantized_latents = latents + (quantized_latents - latents).detach()
return quantized_latents.permute(0, 3, 1, 2).contiguous(), vq_loss # [B x D x H x W]
class ResidualLayer(nn.Module):
def __init__(self,
in_channels: int,
out_channels: int):
super(ResidualLayer, self).__init__()
self.resblock = nn.Sequential(nn.Conv2d(in_channels, out_channels,
kernel_size=3, padding=1, bias=False),
nn.ReLU(True),
nn.Conv2d(out_channels, out_channels,
kernel_size=1, bias=False))
def forward(self, input: Tensor) -> Tensor:
return input + self.resblock(input)
class VQVAE(BaseVAE):
def __init__(self,
in_channels: int,
embedding_dim: int,
num_embeddings: int,
hidden_dims: List = None,
beta: float = 0.25,
img_size: int = 64,
**kwargs) -> None:
super(VQVAE, self).__init__()
self.embedding_dim = embedding_dim
self.num_embeddings = num_embeddings
self.img_size = img_size
self.beta = beta
modules = []
if hidden_dims is None:
hidden_dims = [128, 256]
# Build Encoder
for h_dim in hidden_dims:
modules.append(
nn.Sequential(
nn.Conv2d(in_channels, out_channels=h_dim,
kernel_size=4, stride=2, padding=1),
nn.LeakyReLU())
)
in_channels = h_dim
modules.append(
nn.Sequential(
nn.Conv2d(in_channels, in_channels,
kernel_size=3, stride=1, padding=1),
nn.LeakyReLU())
)
for _ in range(6):
modules.append(ResidualLayer(in_channels, in_channels))
modules.append(nn.LeakyReLU())
modules.append(
nn.Sequential(
nn.Conv2d(in_channels, embedding_dim,
kernel_size=1, stride=1),
nn.LeakyReLU())
)
self.encoder = nn.Sequential(*modules)
self.vq_layer = VectorQuantizer(num_embeddings,
embedding_dim,
self.beta)
# Build Decoder
modules = []
modules.append(
nn.Sequential(
nn.Conv2d(embedding_dim,
hidden_dims[-1],
kernel_size=3,
stride=1,
padding=1),
nn.LeakyReLU())
)
for _ in range(6):
modules.append(ResidualLayer(hidden_dims[-1], hidden_dims[-1]))
modules.append(nn.LeakyReLU())
hidden_dims.reverse()
for i in range(len(hidden_dims) - 1):
modules.append(
nn.Sequential(
nn.ConvTranspose2d(hidden_dims[i],
hidden_dims[i + 1],
kernel_size=4,
stride=2,
padding=1),
nn.LeakyReLU())
)
modules.append(
nn.Sequential(
nn.ConvTranspose2d(hidden_dims[-1],
out_channels=1,
kernel_size=4,
stride=2, padding=1),
nn.Tanh(),
nn.Upsample((28,28)))) # only for MNIST datasets to upsample at size (28, 28)
self.decoder = nn.Sequential(*modules)
def encode(self, input: Tensor) -> List[Tensor]:
"""
Encodes the input by passing through the encoder network
and returns the latent codes.
:param input: (Tensor) Input tensor to encoder [N x C x H x W]
:return: (Tensor) List of latent codes
"""
result = self.encoder(input)
return [result]
def decode(self, z: Tensor) -> Tensor:
"""
Maps the given latent codes
onto the image space.
:param z: (Tensor) [B x D x H x W]
:return: (Tensor) [B x C x H x W]
"""
result = self.decoder(z)
return result
def forward(self, input: Tensor, **kwargs) -> List[Tensor]:
encoding = self.encode(input)[0]
quantized_inputs, vq_loss = self.vq_layer(encoding)
return [self.decode(quantized_inputs), input, vq_loss]
def loss_function(self,
*args,
**kwargs) -> dict:
"""
:param args:
:param kwargs:
:return:
"""
recons = args[0]
input = args[1]
vq_loss = args[2]
recons_loss = F.mse_loss(recons, input)
loss = recons_loss + vq_loss
return {'loss': loss,
'Reconstruction_Loss': recons_loss,
'VQ_Loss':vq_loss}
def sample(self, num_samples: int, device) -> Tensor:
raise Warning
def generate(self, x: Tensor, **kwargs) -> Tensor:
"""
Given an input image x, returns the reconstructed image
:param x: (Tensor) [B x C x H x W]
:return: (Tensor) [B x C x H x W]
"""
return self.forward(x)[0]
训练
from torchvision.datasets import MNIST
from torch.utils.data import DataLoader
from torchvision.transforms import Normalize, Compose, ToTensor
vae = VQVAE(1, 28, 100, [16, 64, 128])
transform = Compose([
ToTensor(),
Normalize((0.1307,), (0.3081,))
])
dataloader = DataLoader(MNIST(root='./', download=True, transform=transform), batch_size=512)
optimizer = torch.optim.AdamW(vae.parameters(), lr=1e-3)
from tqdm import tqdm
# from torch.cuda.amp import autocast
tqdm_bar=tqdm(range(5))
for ep in tqdm_bar:
for i, (x, _) in enumerate(dataloader):
x = x.float()
# with autocast():
recon, input, vq_loss=vae(x)
loss=vae.loss_function(recon, input, vq_loss)
loss['loss'].backward()
optimizer.step()
optimizer.zero_grad()
if i%10==0:
tqdm_bar.set_description('loss: {}'.format(loss['loss']))
可视化
from torchvision.utils import make_grid
import matplotlib.pyplot as plt
dataloader_test = DataLoader(MNIST(root='./', download=True, transform=transform, train=False), batch_size=16, shuffle=True)
# vae.load_state_dict(torch.load('./vae.pt'))
vae.eval()
for x,_ in dataloader_test:
x=x.float()
print(x,_)
reconstruct_x=vae.generate(x)
print(reconstruct_x.detach())
# 前两行为输入数字
# 后两行为重建数字
new_x=torch.cat([x, reconstruct_x.detach()], dim=0)
grid_pics=make_grid(new_x.to('cpu'), 8)
plt.imshow(grid_pics.permute(1,2,0))
break
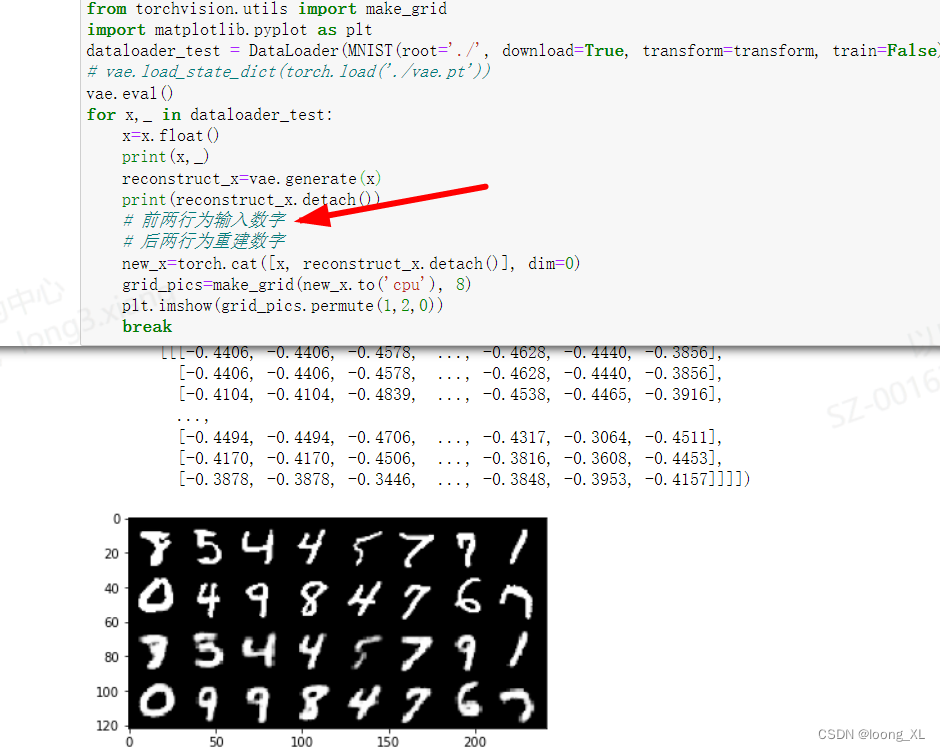
保存
torch.save(vae, "./vqvae.pth")
|