TransC:Differentiating Concepts and Instances for Knowledge Graph Embedding
1 介绍
1.1 问题
作者发现在实现时,对于concept(抽象的概念,如老师 (zhangsan, instance, teacher))和instance(具体的概念,如人物(zhangsan,friendof, lisi), zhangsan 和lisi都是具体的实例)目前现有的模型都没有对其进行区分,为了简化操作通常的处理方式是将二者作为实体不加以区分,进行embeding处理,事实上二者在现实师世界总是不同的,下图就详细展示了concept和instance. 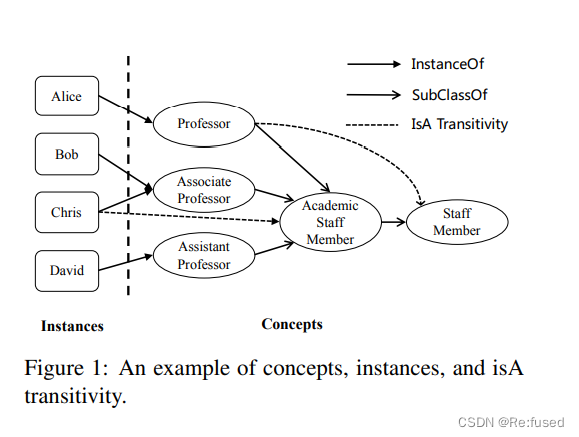 并且作者提出一个逻辑推理问题,类似于isA这种关系,中文理解就是“是”意思,如
(
z
h
a
n
g
s
a
n
,
i
n
s
t
a
n
c
e
o
f
,
t
e
a
c
h
e
r
)
∩
(
t
e
a
c
h
e
r
,
s
u
b
C
l
a
s
s
o
f
,
p
e
o
p
l
e
)
?
(
z
h
a
n
g
s
a
n
,
i
s
,
p
e
o
p
l
e
)
(zhangsan, instanceof, teacher) \cap (teacher, subClassof, people) \Rightarrow (zhangsan, is, people)
(zhangsan,instanceof,teacher)∩(teacher,subClassof,people)?(zhangsan,is,people),这种就是isA的逻辑推理,现在并没有实现相关的推理。
1.2 论文主要解决的两个问题
- Insufficient concept representation
不能区分concept和instance - Lack transitivity of both isA relations
isA这种关系缺少传递性
1.3 思想
将三元组分成三种类型:
- (Instance, Relation, Instance),即(Zhangsan, Friendof, Lisi)
这种就采用常规的TransE模型即可 - (Instance, Relation, Concept),即(Zhangsan, Is, Teacher)
对于实例应该与它们所属的概念相近,将每个concept编码为一个领域,将每个instance编码为同一语义空间中的向量,并利用相对位置对concept和instance之间的关系进行建模。 - (Concept, Relation, Concept),即(Teacher, Is, People)
将这种关系分为四种不同的关系,即(A, Relation, B),A和B之间的关系为B包含于A, 二者无关, 二者相交, A包含于B。
然后将三着进行负采样使用margin-based ranking loss,进行优化。
2 模型
2.1 知识图谱结构
知识图谱分为三类:
- InstanceOf 即(Instance, Relation, Concept)
- SubClassOf 即(Concept, Relation, Concept)
- Relational triple 即(Instance, Relation, Instance)
K
G
=
{
C
,
I
,
R
,
S
}
,
其
中
C
,
I
\mathcal{KG} = \{ \mathcal{C,I,R, S} \}, 其中\mathcal{C, I}
KG={C,I,R,S},其中C,I分别表示为concept和instance,
R
\mathcal{R}
R表示所有的关系的集合,S是三种不同分类的总集合,其中小写字母e表示instance类型,c表示concept类型。
2.2 关于推理
推理分为两种不同的类型,即从concept到concept的推理subClassOf-subClassOf,从instance到concept的推理instanceOf-subClassOf。
- instanceOf-subClassOf
(
张
三
,
是
,
教
授
)
∩
(
教
授
,
是
,
学
者
)
?
(
张
三
,
是
,
学
者
)
(张三,是,教授)\cap(教授,是,学者)\Rightarrow (张三,是,学者)
(张三,是,教授)∩(教授,是,学者)?(张三,是,学者) - subClassOf-subClassOf
(
教
授
,
是
,
学
者
)
∩
(
学
者
,
是
,
人
)
?
(
教
授
,
是
,
人
)
(教授,是,学者)\cap(学者,是,人)\Rightarrow (教授,是, 人)
(教授,是,学者)∩(学者,是,人)?(教授,是,人) 
2.3 TransC
提出一个理论,
s
(
p
,
m
)
s(\mathcal {p}, m)
s(p,m), p是其embedding 向量, m是其作用的领域半径,该理论只对于concept提出的。
InstanceOf Triple Representation(Instance, Relation, Concept)
评分函数为:  其中
i
i
i为instance的embedding,
p
p
p为concept的embedding, m为其作用的领域范围半径。
SubClassOf Triple Representation(Concept, Relation, Concept)
对于三元组
(
c
i
,
r
,
c
j
)
(c_i, r, c_j)
(ci?,r,cj?), 其中
s
(
p
i
,
m
i
)
,
s
(
p
j
,
m
j
)
分
别
为
c
i
,
c
j
s(p_i, m_i), s(p_j, m_j)分别为c_i, c_j
s(pi?,mi?),s(pj?,mj?)分别为ci?,cj?的领域内容。 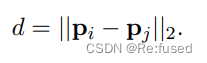 d为领域核心之间的距离。 若三元组
(
c
i
,
r
,
c
j
)
(c_i, r, c_j)
(ci?,r,cj?)是正确的,则效果如下图所示: 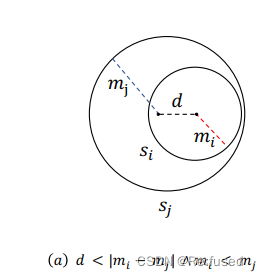
- 两个concept相互分开
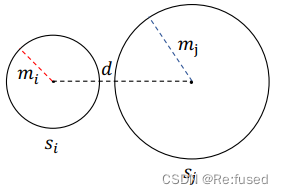 公式:  - 二者相交
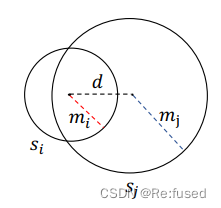 公式: 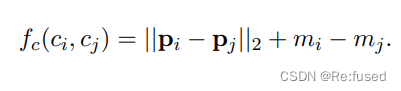 以上两种目的都是让其不断接近于图(a)。 -
c
j
包
含
c
i
c_j包含c_i
cj?包含ci?
这个关系正好与图(a)相反 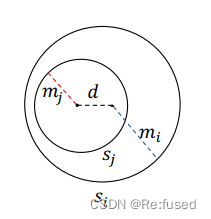 公式: 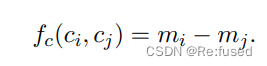 缩小大的范围,放大小的范围,让二者趋于一致。
Relational Triple Representation(Instance, Relation, Instance)
采取常规的方式TransE的方法。 
3 损失函数
对于不同种类的类型的数据,对其进行负采样,负采样内容在其相应的数据类型范围内进行采集。
InstanceOf Triple Representation(Instance, Relation, Concept)

SubClassOf Triple Representation(Concept, Relation, Concept)

Relational Triple Representation(Instance, Relation, Instance)
 所有的损失函数共同训练,损失函数汇总: 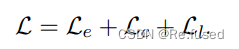
|