内部业务,记录一下过程
1、首先将解决方案文档用数字-项目名缩写重命名排序,方便标签命名,并新建一个文件夹保存报错截图 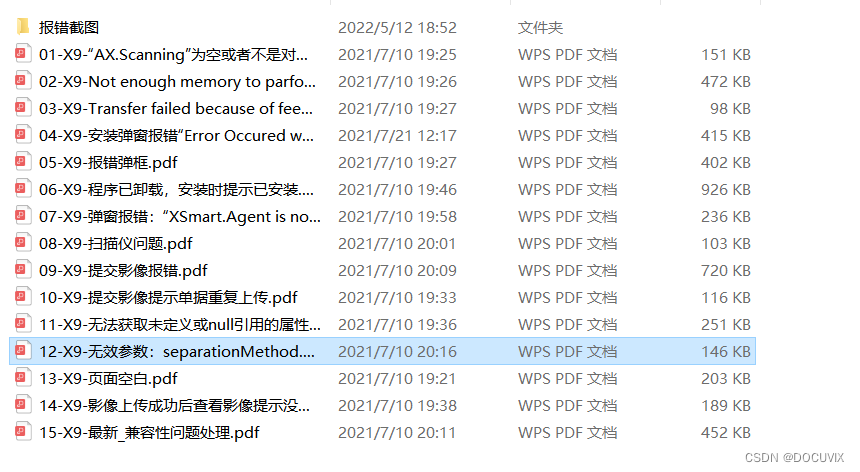
2、打开其中一个解决方案文档 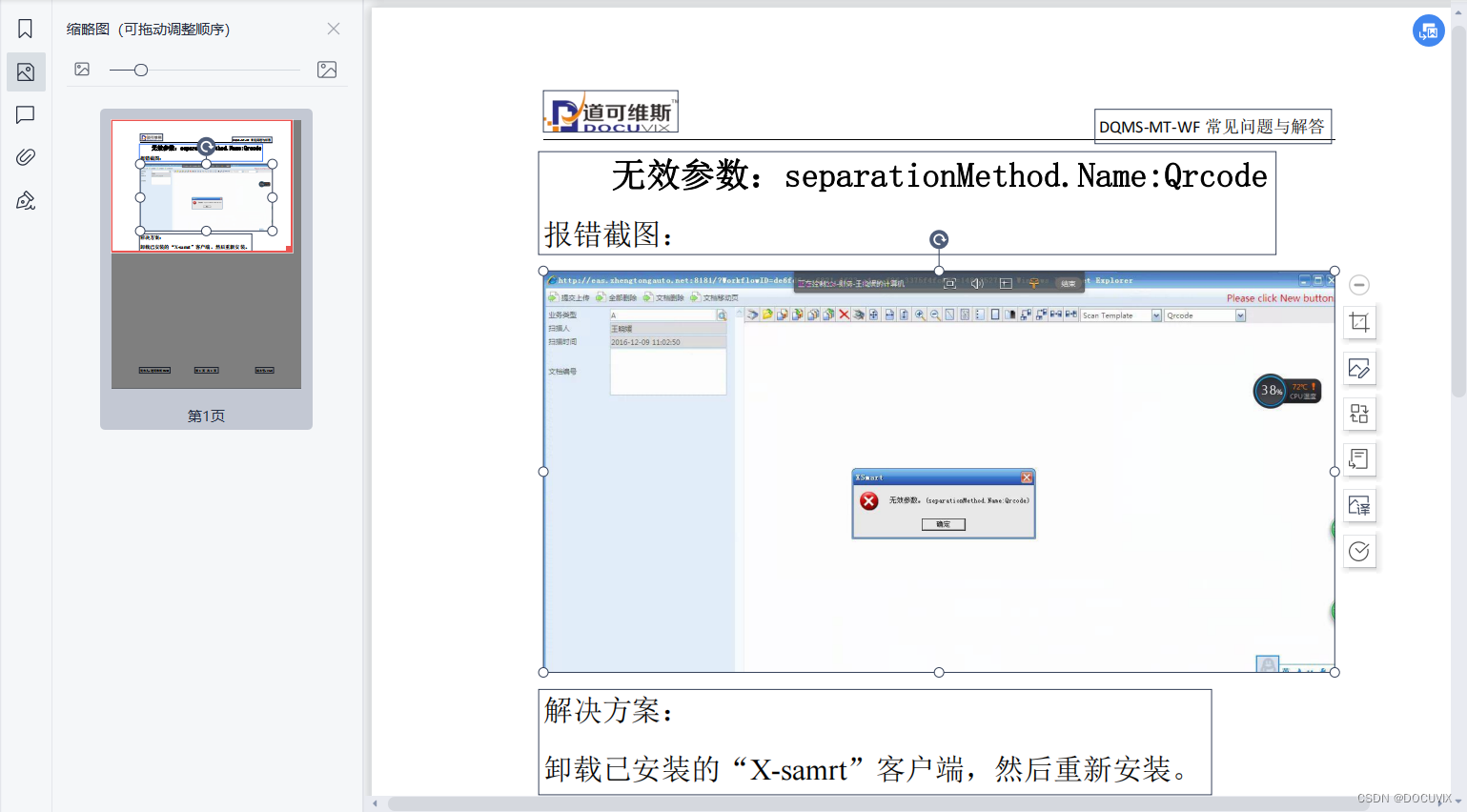
3、将里面的图另存出来并单独截取成如下报错截图 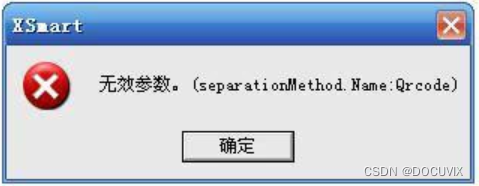
4、另存到报错截图文件夹中,并重命名为解决方案文件同名 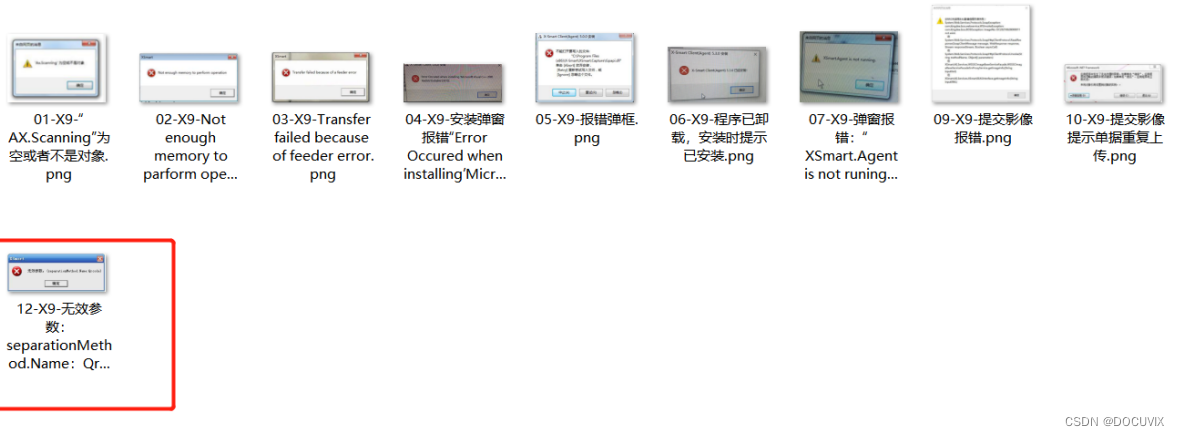
5、点开图片,在不同的背景下用连拍拍不同角度
6、新建一个文件夹,重命名为数字+项目缩写前缀(如“12-X9-无效参数:separationMethod.Name:Qrcode”重命名为“12-X9”)将图片全部存入进去 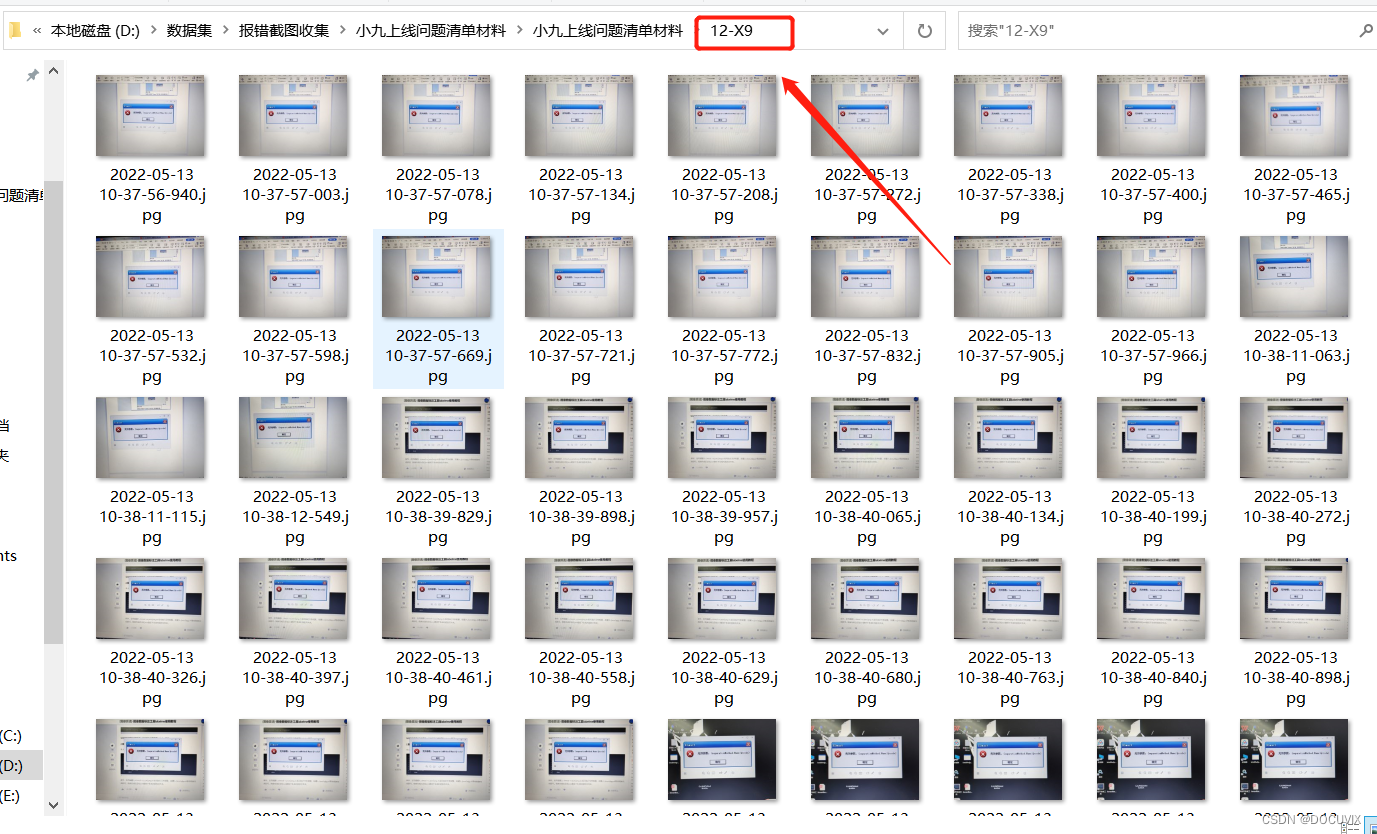
7、打开lableme软件,选择open dir,打开上一步新建的文件夹 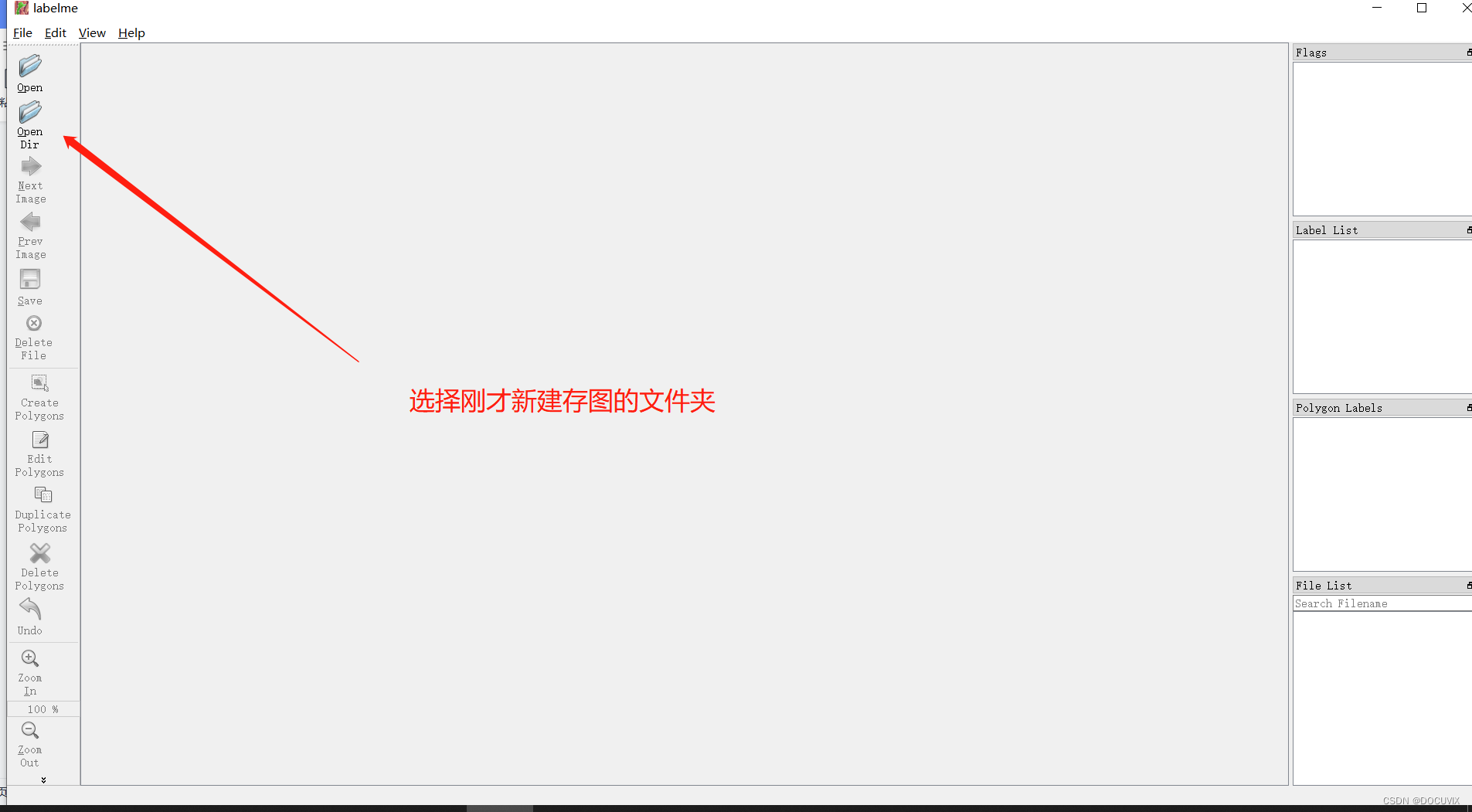
选择后如下 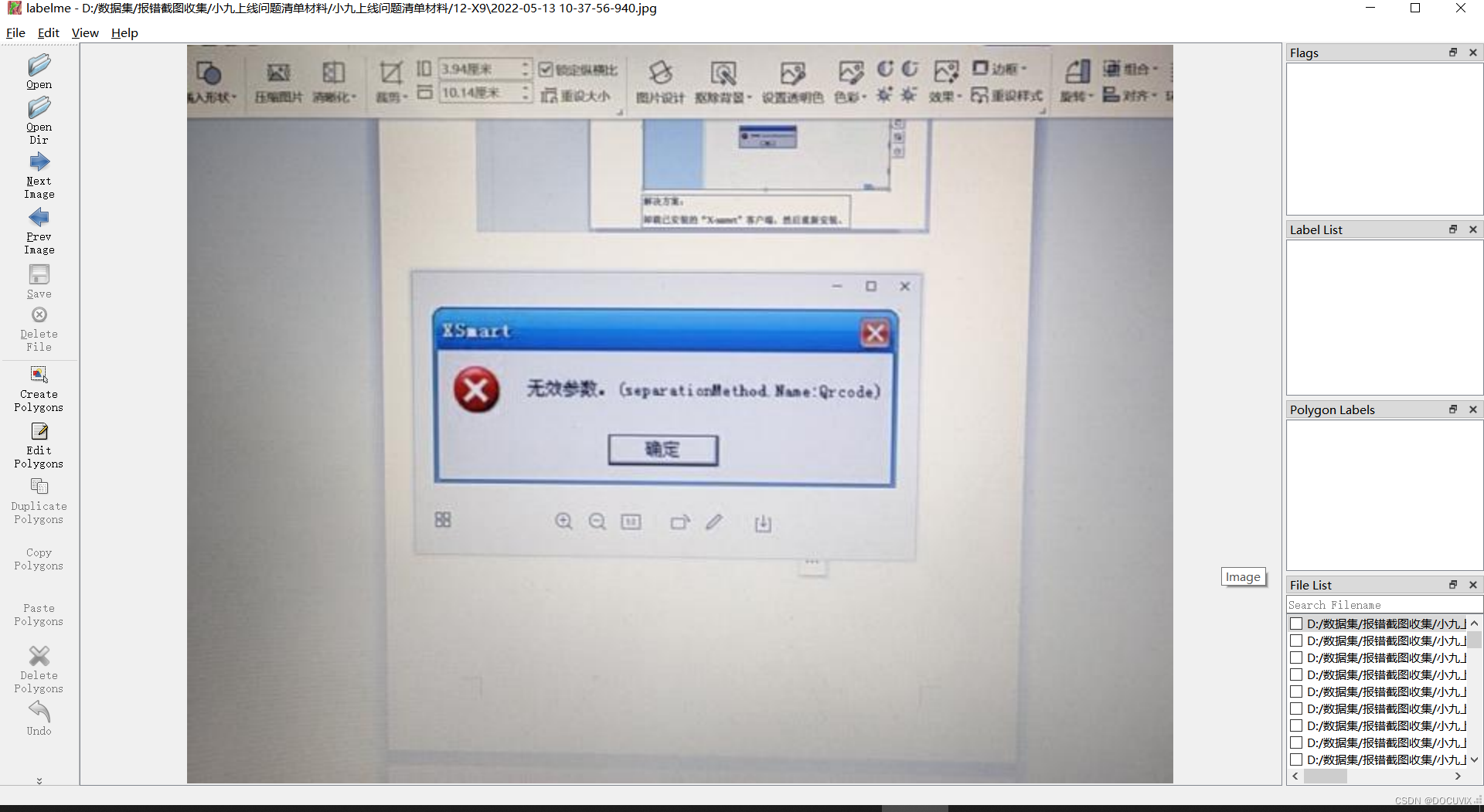
点开file-save automatically,这样你标注好了就会自动保存 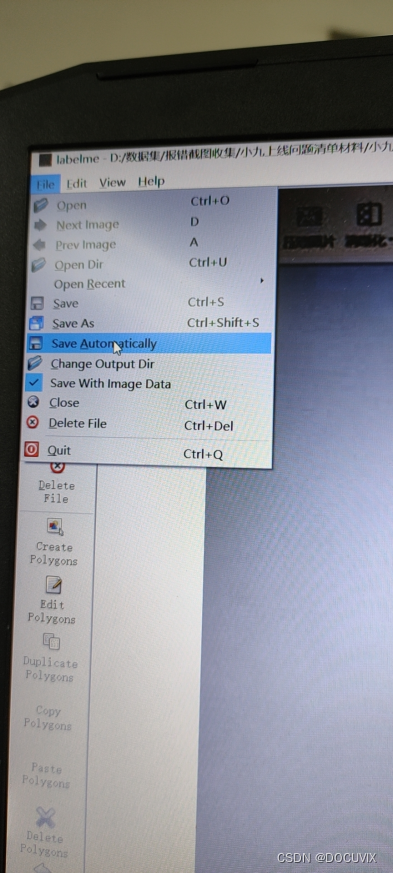
8、点开create polygons
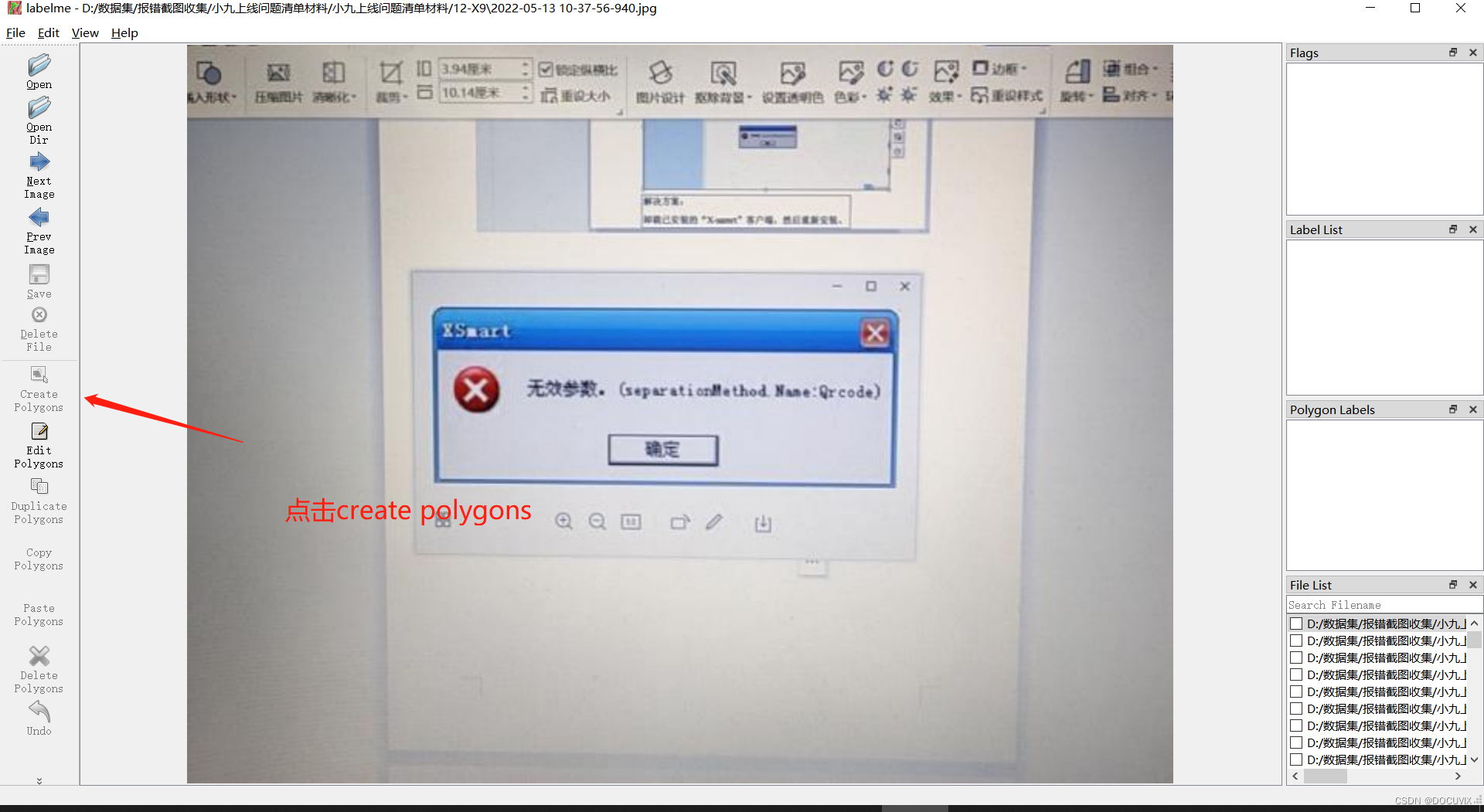
标注的时候就选择截图所在的位置,并将标签名命名为选择的文件夹名(如12-X9)
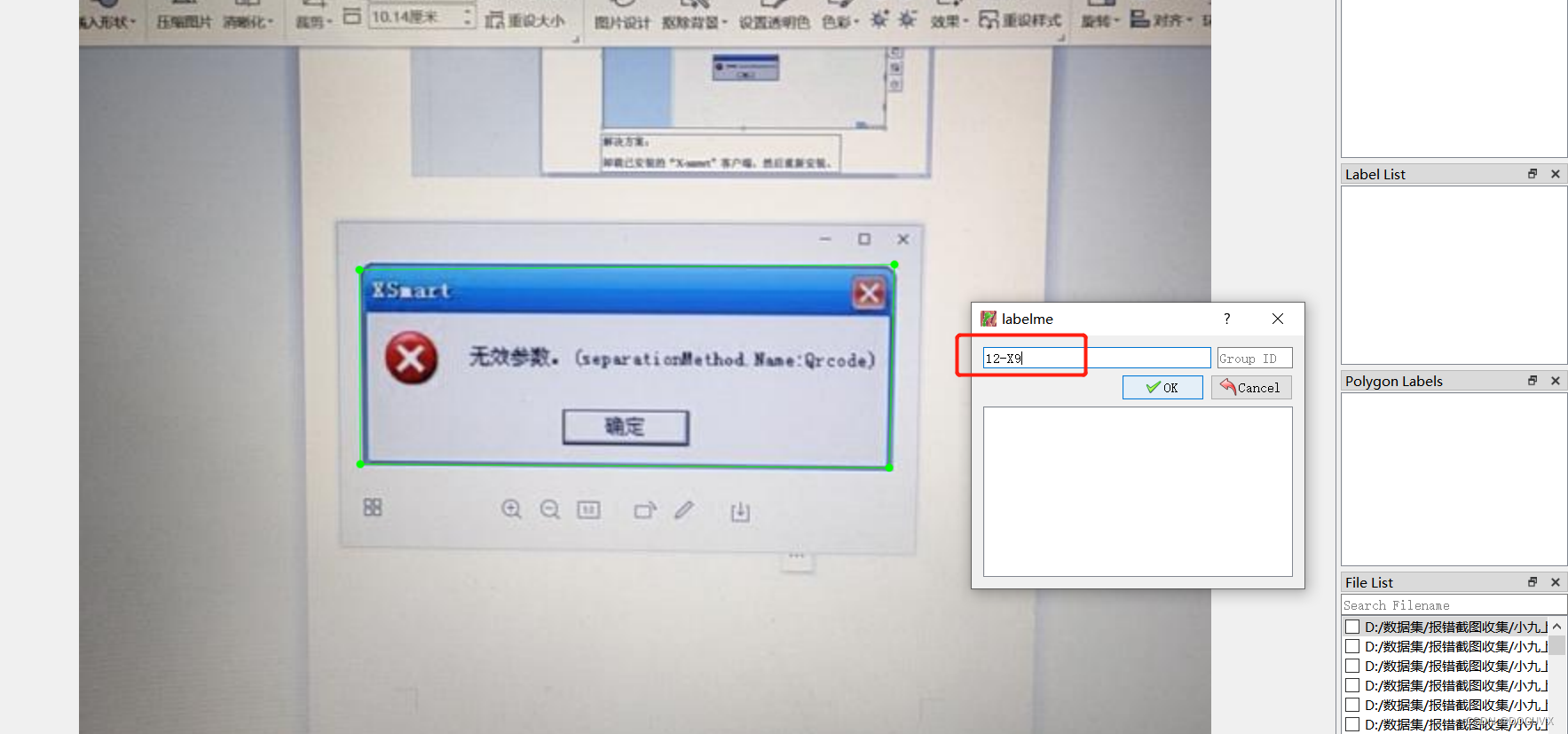
如果标歪了可以选择edit polygons调整 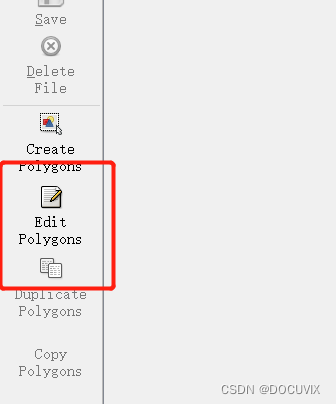
按一下D,跳转到下一个图片,继续标注,同一种报错用同一个标签 在这里插入图片描述 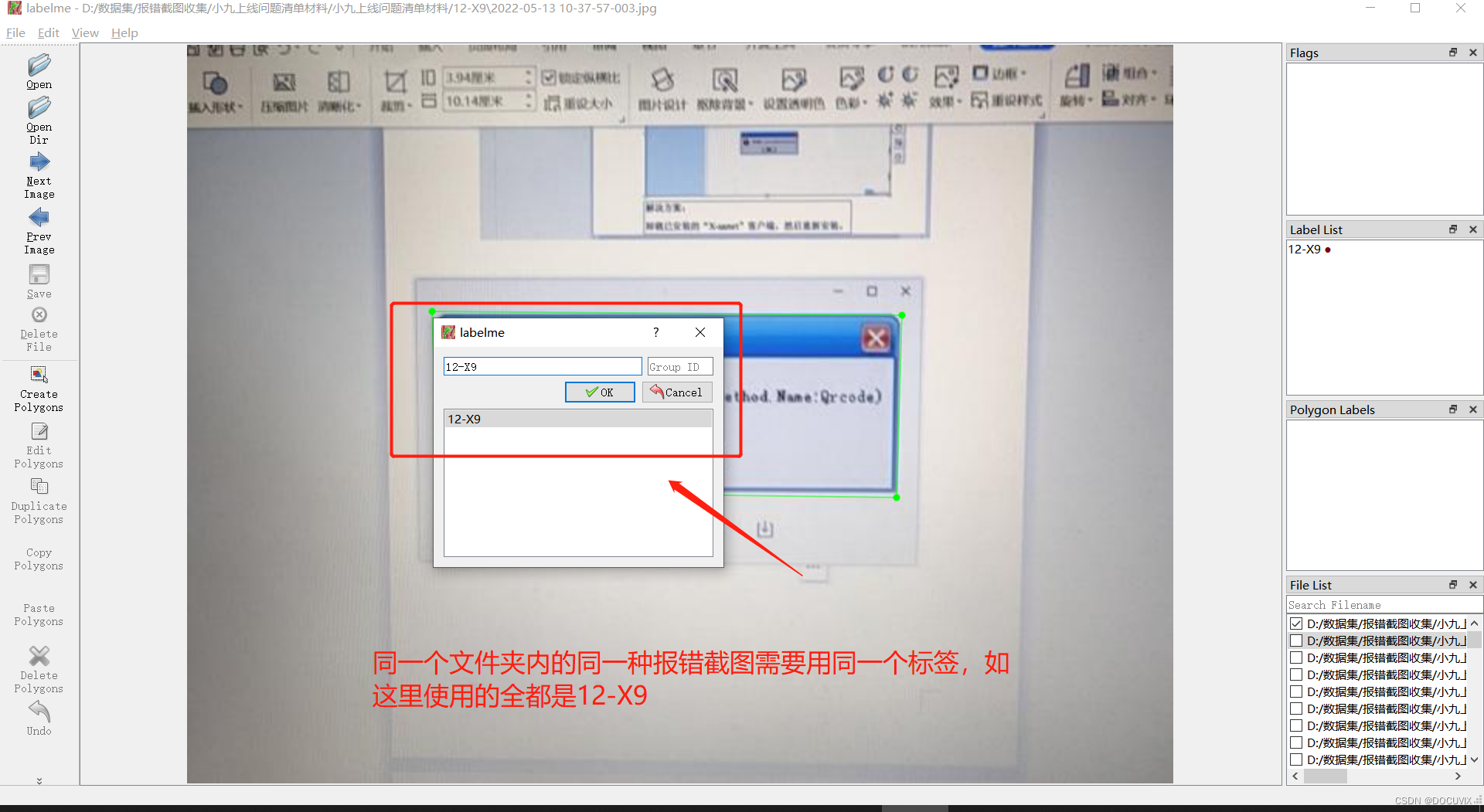
比如另一种报错就用另一种标签 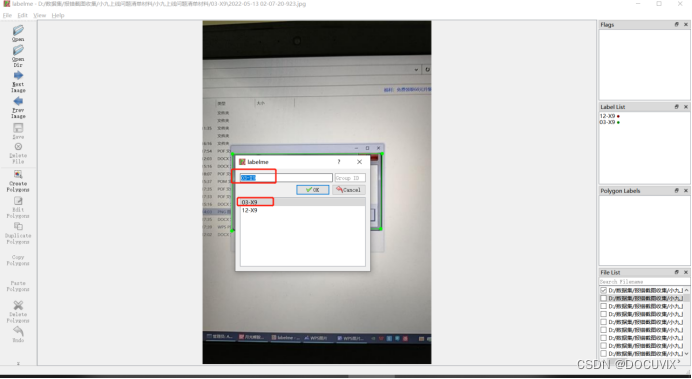
接下来就是循环标注了 最终出来的是这种效果,图片和json文件一一对应 如果标签选错了删除对应的json文件重新标注即可 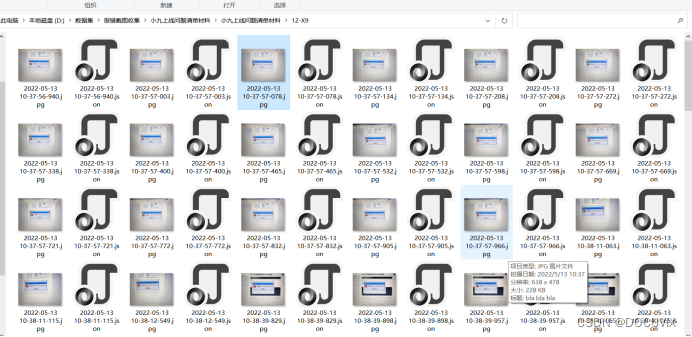
收集起来集中在一个文件夹中用python代码进行一下重命名
import os,json
def findAllFile(base):
j=0
for root, ds, fs in os.walk(base):
for i ,f in enumerate(fs):
if f.endswith('.jpg'):
j+=1
print(j)
name = f.split(".")[0]
jpgpath = os.path.join(root,f)
jsonpath = os.path.join(root,f"{name}.json")
newjpgpath = jpgpath.replace(name,str(j))
newjsonpath = jsonpath.replace(name,str(j))
print(newjpgpath,newjsonpath)
with open(jsonpath,'r') as fp:
data = json.load(fp)
print(data['imagePath'])
data['imagePath'] = f"{str(j)}.jpg"
with open(newjsonpath, 'w') as fp1:
fp1.write(json.dumps(data, indent=4, ensure_ascii=False))
os.rename(jpgpath, newjpgpath)
findAllFile(r'F:\pathroad')
然后再用代码转换成voc格式
```python
"""
Created on Thu Sep 19 14:51:00 2019
@author: Andrea
"""
import os
import numpy as np
import codecs
import json
from glob import glob
import cv2
import shutil
from sklearn.model_selection import train_test_split
labelme_path = r"F:\beto\trains"
img_format = "jpg"
saved_path = "./VOC2007/"
if not os.path.exists(saved_path + "Annotations"):
os.makedirs(saved_path + "Annotations")
if not os.path.exists(saved_path + "JPEGImages/"):
os.makedirs(saved_path + "JPEGImages/")
if not os.path.exists(saved_path + "ImageSets/Main/"):
os.makedirs(saved_path + "ImageSets/Main/")
for json_file_ in os.listdir(labelme_path):
if ('.json' not in json_file_):
continue
else:
json_file_ = json_file_.split('.json')[0]
print(json_file_)
json_filename = os.path.join(labelme_path, json_file_ + ".json")
print(json_filename)
json_file = json.load(open(json_filename, "r", encoding="utf-8"))
print(os.path.join(labelme_path, json_file_ + '.' + img_format))
print()
height, width, channels = cv2.imread(os.path.join(labelme_path, json_file_ + "." + img_format)).shape
with codecs.open(saved_path + "Annotations/" + json_file_ + ".xml", "w", "utf-8") as xml:
xml.write('<annotation>\n')
xml.write('\t<folder>' + 'UAV_data' + '</folder>\n')
xml.write('\t<filename>' + json_file_ + '.' + img_format + '</filename>\n')
xml.write('\t<source>\n')
xml.write('\t\t<database>The UAV autolanding</database>\n')
xml.write('\t\t<annotation>UAV AutoLanding</annotation>\n')
xml.write('\t\t<image>flickr</image>\n')
xml.write('\t\t<flickrid>NULL</flickrid>\n')
xml.write('\t</source>\n')
xml.write('\t<owner>\n')
xml.write('\t\t<flickrid>NULL</flickrid>\n')
xml.write('\t\t<name>Yuanyiqin</name>\n')
xml.write('\t</owner>\n')
xml.write('\t<size>\n')
xml.write('\t\t<width>' + str(width) + '</width>\n')
xml.write('\t\t<height>' + str(height) + '</height>\n')
xml.write('\t\t<depth>' + str(channels) + '</depth>\n')
xml.write('\t</size>\n')
xml.write('\t\t<segmented>0</segmented>\n')
for multi in json_file["shapes"]:
points = np.array(multi["points"])
xmin = min(points[:, 0])
xmax = max(points[:, 0])
ymin = min(points[:, 1])
ymax = max(points[:, 1])
label = multi["label"]
if xmax <= xmin:
pass
elif ymax <= ymin:
pass
else:
xml.write('\t<object>\n')
xml.write('\t\t<name>' + str(label) + '</name>\n')
xml.write('\t\t<pose>Unspecified</pose>\n')
xml.write('\t\t<truncated>1</truncated>\n')
xml.write('\t\t<difficult>0</difficult>\n')
xml.write('\t\t<bndbox>\n')
xml.write('\t\t\t<xmin>' + str(xmin) + '</xmin>\n')
xml.write('\t\t\t<ymin>' + str(ymin) + '</ymin>\n')
xml.write('\t\t\t<xmax>' + str(xmax) + '</xmax>\n')
xml.write('\t\t\t<ymax>' + str(ymax) + '</ymax>\n')
xml.write('\t\t</bndbox>\n')
xml.write('\t</object>\n')
print(json_filename, xmin, ymin, xmax, ymax, label)
xml.write('</annotation>')
image_files = glob(os.path.join(labelme_path, "*." + img_format))
print("发现图片:{}张".format(image_files.__len__()))
print("copy image files to VOC007/JPEGImages/")
for image in image_files:
shutil.copy(image, saved_path + "JPEGImages/")
txtsavepath = saved_path + "ImageSets/Main/"
ftrainval = open(txtsavepath + '/trainval.txt', 'w')
ftest = open(txtsavepath + '/test.txt', 'w')
ftrain = open(txtsavepath + '/train.txt', 'w')
fval = open(txtsavepath + '/val.txt', 'w')
total_files = glob("./VOC2007/Annotations/*.xml")
total_files = [i.split("/")[-1].split(".xml")[0] for i in total_files]
for file in total_files:
ftrainval.write(file + "\n")
train_files, val_files = train_test_split(total_files, test_size=0.2, random_state=42)
for file in train_files:
ftrain.write(file + "\n")
for file in val_files:
fval.write(file + "\n")
ftrainval.close()
ftrain.close()
fval.close()
这样数据集就做好了
|