注:仅仅是学习记录笔记,搬运了学习课程的ppt内容,本意不是抄袭!望大家不要误解!纯属学习记录笔记!!!!!!
一、机器学习中的优化思想
模型训练的目标:求解一组最适合的权重向量,令神经网络的输出结果与真实值尽量接近。
关键概念:损失函数是可以衡量真实值与预测结果的差异,评价模型学习过程中产生的损失的函数。
如果损失函数的值很小,则说明模型预测与真实值很接近,模型在数据集上表现优异,权重优秀;如果顺势函数的值很大,则说明模型预测值与真实值差异很大,模型在数据集上表现很差,权重糟糕。
二、回归:误差平方和SSE
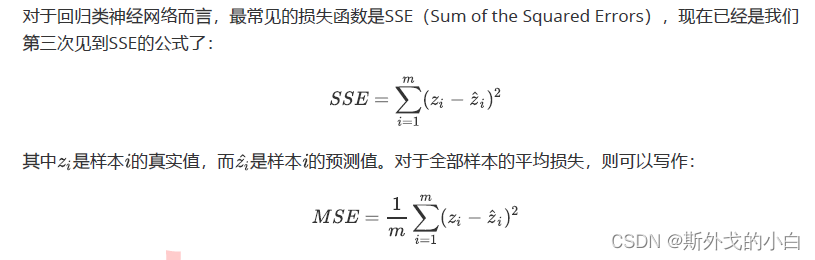
from torch.nn import MSELoss
import torch
torch.random.manual_seed(420)
yhat = torch.randn(size=(50, 1), dtype=torch.float32)
y = torch.randn(size=(50, 1), dtype=torch.float32)
criterion = MSELoss()
loss = criterion(yhat, y)
print(loss)
对于MESloss来说,有一个重要的参数是reduction,reduction默认是mean,求得是平均损失,reduction设置为sum时,求的是全部样本的损失值之和。
criterion_1 = MSELoss(reduction='sum')
loss_1 = criterion_1(yhat, y)
print(loss_1)
criterion_2 = MSELoss(reduction='mean')
loss_2 = criterion_2(yhat, y)
print(loss_2)
三、二分类交叉熵损失函数
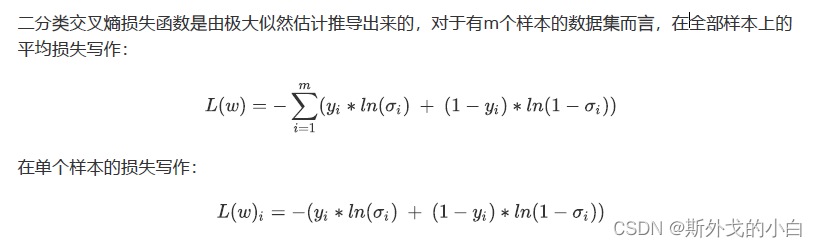
1 极大似然估计求解二分类交叉熵损失
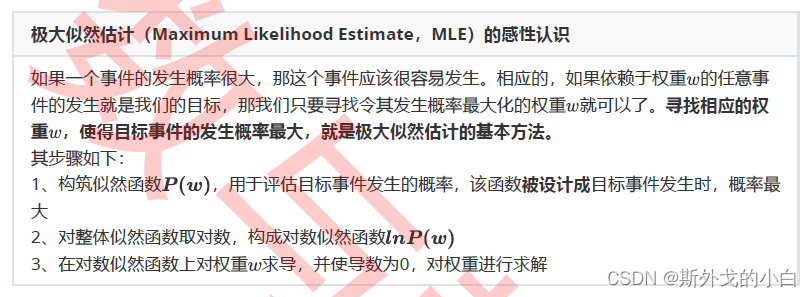 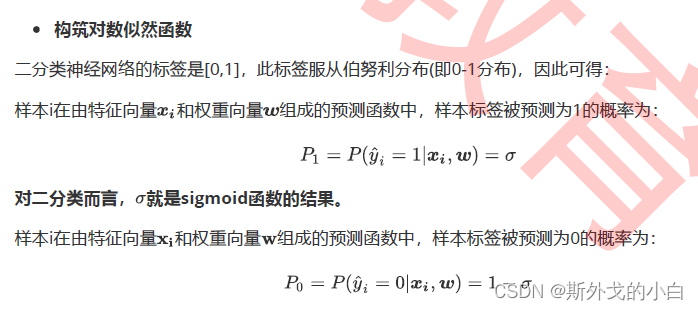
我们将极大似然概率公式表示为:  第i个样本预测为1的概率为p1,被预测为0的概率为p0,yi代表第i个样本的真实标签,这个标签为1的时候,p1就保留下来了,如果真实标签为0的话,p0就保留下来了。然后我们使得这个预测概率达到最大即可。 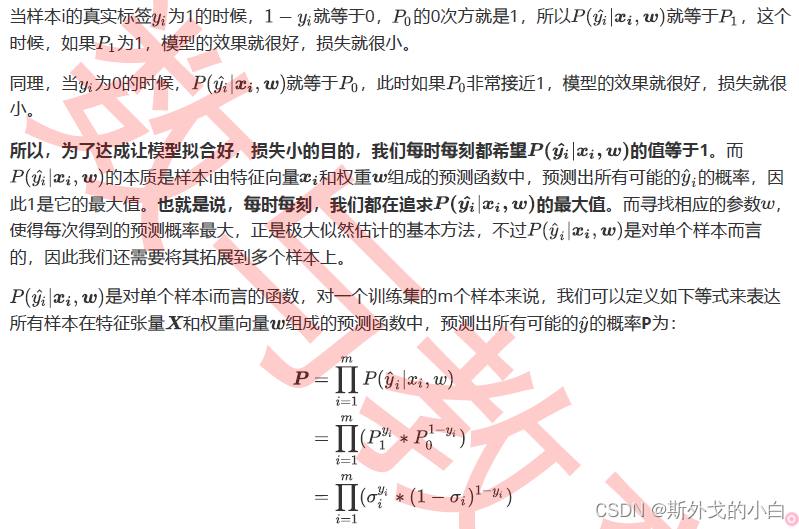 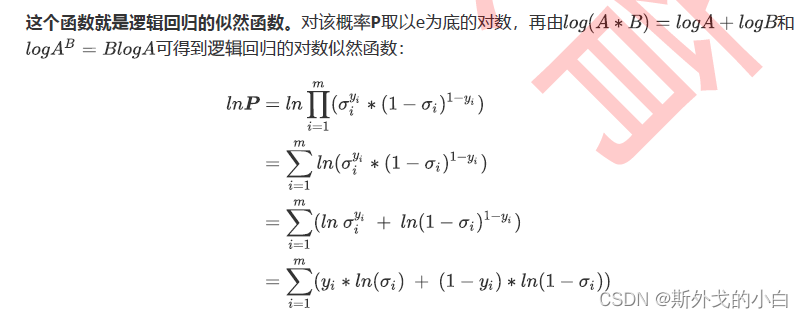 
2 用tensor实现二分类交叉熵损失
N = 3 * pow(10, 3)
torch.random.manual_seed(420)
x = torch.rand((N, 4), dtype=torch.float32)
w = torch.rand((4, 1), dtype=torch.float32)
y = torch.randint(low=0, high=2, size=(N, 1), dtype=torch.float32)
zhat = torch.mm(x, w)
sigma = torch.sigmoid(zhat)
loss = (-1/N) * torch.sum(y * torch.log(sigma) + (1 - y) * torch.log(1 - sigma))
print(loss)
注意,在深度学习pytorch框架下,尽量使用torch中的函数,这样的话,计算速度较快
案例比较
N = 3 * pow(10, 6)
torch.random.manual_seed(420)
x = torch.rand((N, 4), dtype=torch.float32)
w = torch.rand((4, 1), dtype=torch.float32)
y = torch.randint(low=0, high=2, size=(N, 1), dtype=torch.float32)
zhat = torch.mm(x, w)
sigma = torch.sigmoid(zhat)
starttime = time.time()
loss_1 = (-1/N) * torch.sum(y * torch.log(sigma) + (1 - y) * torch.log(1 - sigma))
endtime = time.time()
print(endtime - starttime)
starttime = time.time()
loss_2 = (-1/N) * sum(y * torch.log(sigma) + (1 - y) * torch.log(1 - sigma))
endtime = time.time()
print(endtime - starttime)
通过这两个例子可以看出,使用torch.sum()的计算速度远远比python自带的sum()要快得多
3 用PyTorch中的类实现二分类交叉熵损失
BCEWithLogitsLoss以及BCELoss 其中BCEWithLogitsLoss自带sigmoid函数,只需要输入预测值zhat即可 对于BCELoss来说,需要输入激活函数激活后的sigma
在N = 3 * pow(10, 6)
torch.random.manual_seed(420)
x = torch.rand((N, 4), dtype=torch.float32)
w = torch.rand((4, 1), dtype=torch.float32)
y = torch.randint(low=0, high=2, size=(N, 1), dtype=torch.float32)
zhat = torch.mm(x, w)
sigma = torch.sigmoid(zhat)
criterion = nn.BCELoss()
LOSS = criterion(sigma, y)
print(LOSS)
criterion2 = nn.BCEWithLogitsLoss()
loss2 = criterion2(zhat, y)
print(loss2)
四、多分类交叉熵损失函数
1 由二分类推广到多分类
假设样本的真实标签为1,我们就希望p1最大,同理,如果样本的真实标签为其他值,我们就希望其他的值对应的概率最大。 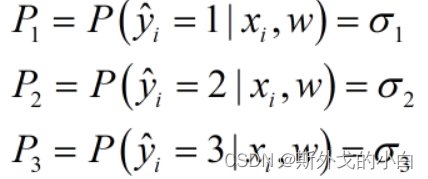 但是在二分类问题中,我们将y和1-y作为概率p的指数,这是因为真实标签只有0和1两种状况,但是在多分类中,我们的真实标签可能是任意整数,无法使用y的1-y这样的机构来构建似然函数,所以我们认为,如果多分类的标签也可以用0和1来表示就好了,这样我们就可以继续使用真实标签作为指数的方式。
因此我们对标签进行了one-hot编码,如下所示:  在矩阵中, 每一行依旧对应样本,但却由三分类衍生出了三个新的列,分别代表:真实标签是否等于1、等于2以及等于3。把标签整合为标签矩阵后,我们就可以将单个样本在总共k个分类情况整合为以下的似然函数:  j为样本i所对应的真实标签的编号 虽有所有训练集的样本来说,我们可以定义如下等式来表达所有样本在特征张量X和权重向量w组成的预测函数中,预测出所有可能的预测值yhat的概率p为: 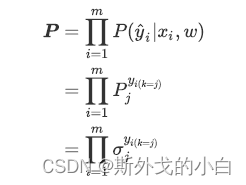 与二分类问题一致,似然函数解出来以后,我们对似然函数求对数得到: 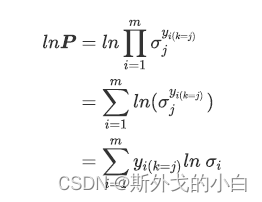 这个函数就是交叉熵损失函数,不难看出,二分类交叉熵函数是多分类的一种特殊情况 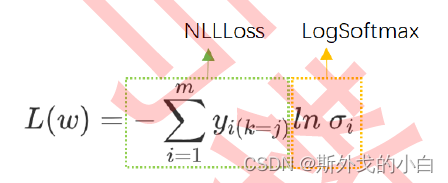 在pytorch里面直通通过nn.logsoftmax类调用这个功能,我们把对数之外的:乘以标签、加和、取负等等过程打包起来,称之为负对数似然函数(Negative Log Likelihood function),也就是说,在计算损失函数时,我们就不再需要单独的softmax函数了。
2 用PyTorch实现多分类交叉熵损失
在pytorch中实现交叉熵函数的时候,有两种方法:
调用logsoftmax和NLLLoss实现
N = 3*pow(10, 2)
torch.random.manual_seed(420)
X = torch.rand((N, 4), dtype=torch.float32)
w = torch.rand((4, 3), dtype=torch.float32, requires_grad=True)
y = torch.randint(low=0, high=3, size=(N,), dtype=torch.float32)
zhat = torch.mm(X, w)
logsm = nn.LogSoftmax(dim=1)
logsigma = logsm(zhat)
criterion = nn.NLLLoss()
criterion(logsigma, y.long())
直接调用CrossEntropyLoss()
N = 3*pow(10, 2)
torch.random.manual_seed(420)
X = torch.rand((N, 4), dtype=torch.float32)
w = torch.rand((4, 3), dtype=torch.float32, requires_grad=True)
y = torch.randint(low=0, high=3, size=(N,), dtype=torch.float32)
zhat = torch.mm(X, w)
criterion = torch.nn.CrossEntropyLoss()
loss = criterion(zhat, y.long())
print(loss)
|