用notebook写的
必做部分
2.1 Plotting the Data
import numpy as np
import pandas as pd
import matplotlib.pyplot as plt
data=pd.read_csv('ex1data1.txt',names=['x','y'])
data_x=np.array(data['x'])
data_y=np.array(data['y'])
plt.figure()
plt.scatter(data_x,data_y,marker='x',color='r')
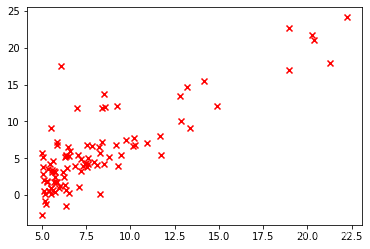
def computeCost(X,y,theta):
return np.sum((X@theta-data_y)**2)/(2*m)
m=len(data_y)
X=np.column_stack([np.ones(len(data_x)),data_x])
y=data_y
theta=np.array([0,0])
J=computeCost(X,y,theta)
print(J)
J=computeCost(X,y,np.array([-1,2]))
print(J)
def gradientDescent(X,y,theta,alpha,num_iters):
for _ in range(num_iters):
temp=np.zeros(len(theta))
for j in range(len(theta)):
temp[j]=theta[j]-alpha*(1/m)*np.sum((X@theta-y)@X[:,j])
for j in range(len(theta)):
theta[j]=temp[j]
return theta
theta=np.zeros(2)
iterations=1500
alpha=0.01
theta=gradientDescent(X,y,theta,alpha,iterations)
theta
plt.figure()
plt.scatter(data_x,data_y,marker='x',color='r')
plt.plot(X[:,1],X@theta,color='b')
plt.show()
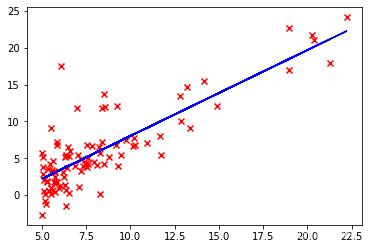
theta0_vals=np.arange(-10,10,0.1)
theta1_vals=np.arange(-1,4,0.025)
J_vals=np.zeros((len(theta0_vals),len(theta1_vals)))
for i in range(len(theta0_vals)):
for j in range(len(theta1_vals)):
t=np.array([theta0_vals[i],theta1_vals[j]])
J_vals[i][j]=computeCost(X,y,t)
J_vals=J_vals.T
plt.figure()
ax=plt.axes(projection='3d')
grid_x,grid_y=np.meshgrid(theta0_vals,theta1_vals)
grid_z=-grid_x**2-grid_y**2
ax.plot_surface(grid_x,grid_y,J_vals,cmap='summer')
ax.set_xlabel('theta_0')
ax.set_ylabel('theta_1')
plt.show()
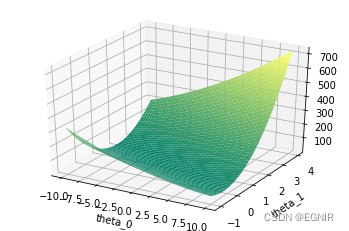
plt.figure()
plt.contour(grid_x,grid_y,J_vals,np.logspace(-2,3,20))
plt.xlabel('theta_0')
plt.ylabel('theta_1')
plt.plot(theta[0],theta[1],'rx')
plt.show()
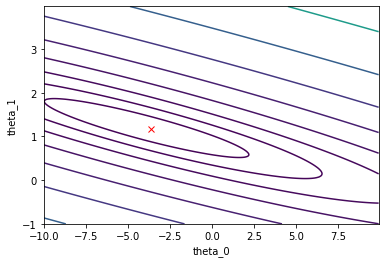
选做
下面是一个错误的归一化。numpy中的std求的是总体标准差,pandas中的std求的是样本标准差。这两个是不一样的!在这里应该用样本标准差,所以下面这种写法不对。
import numpy as np
import pandas as pd
import matplotlib.pyplot as plt
def featureNormalize(X):
X_norm=X
mu=np.mean(X_norm,axis=0)
sigma=np.std(X_norm,axis=0)
print(sigma)
X_norm=(X_norm-sigma)
return X_norm,mu,sigma
data=pd.read_csv('ex1data2.txt',names=['area','num','y'])
X=data[['area','num']].values
y=data['y'].values
X_norm,mu,sigma=featureNormalize(X)
(麻了,之后心情好再继续写吧。。。)
|