应用Transformer框架对分子属性进行预测,代码:MAT,原文:Molecule Attention Transformer。变量名,函数名很多来自The Annotated Transformer,在《深入浅出Embedding》一书中也做了讲解。本文主要从实例运行开始一步步看代码具体内容,整体模型如下: 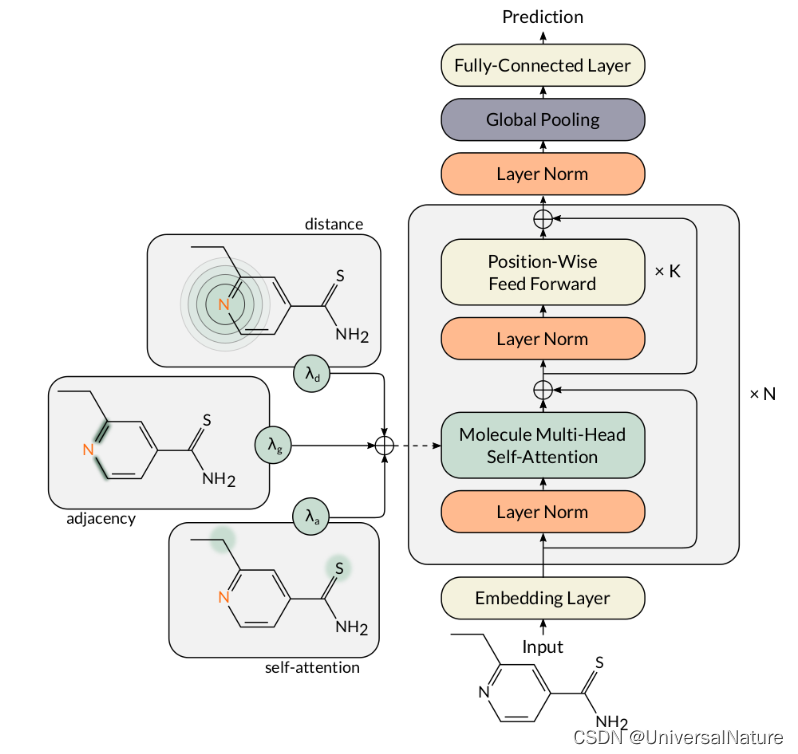
2.模型构建
from transformer import make_model
d_atom = X[0][0].shape[1]
model_params = {
'd_atom': d_atom,
'd_model': 1024,
'N': 8,
'h': 16,
'N_dense': 1,
'lambda_attention': 0.33,
'lambda_distance': 0.33,
'leaky_relu_slope': 0.1,
'dense_output_nonlinearity': 'relu',
'distance_matrix_kernel': 'exp',
'dropout': 0.0,
'aggregation_type': 'mean'
}
model = make_model(**model_params)
- 利用 make_model 返回构建模型,d_model 是每个原子的特征数,此处是28,d_model 是经过 Embedding 后的维度,N 是 Transformer 块的重复次数,h 是头数,N_dense 是最终模型输出维度,输出标量应该设为1。整个模型构建与 Transformer 类似。
2.1.make_model & run
def make_model(d_atom, N=2, d_model=128, h=8, dropout=0.1,
lambda_attention=0.3, lambda_distance=0.3, trainable_lambda=False,
N_dense=2, leaky_relu_slope=0.0, aggregation_type='mean',
dense_output_nonlinearity='relu', distance_matrix_kernel='softmax',
use_edge_features=False, n_output=1,
control_edges=False, integrated_distances=False,
scale_norm=False, init_type='uniform', use_adapter=False, n_generator_layers=1):
"Helper: Construct a model from hyperparameters."
c = copy.deepcopy
attn = MultiHeadedAttention(h, d_model, dropout, lambda_attention, lambda_distance, trainable_lambda, distance_matrix_kernel, use_edge_features, control_edges, integrated_distances)
ff = PositionwiseFeedForward(d_model, N_dense, dropout, leaky_relu_slope, dense_output_nonlinearity)
model = GraphTransformer(
Encoder(EncoderLayer(d_model, c(attn), c(ff), dropout, scale_norm, use_adapter), N, scale_norm),
Embeddings(d_model, d_atom, dropout),
Generator(d_model, aggregation_type, n_output, n_generator_layers, leaky_relu_slope, dropout, scale_norm))
for p in model.parameters():
if p.dim() > 1:
if init_type == 'uniform':
nn.init.xavier_uniform_(p)
elif init_type == 'normal':
nn.init.xavier_normal_(p)
elif init_type == 'small_normal_init':
xavier_normal_small_init_(p)
elif init_type == 'small_uniform_init':
xavier_uniform_small_init_(p)
return model
for batch in data_loader:
adjacency_matrix, node_features, distance_matrix, y = batch
batch_mask = torch.sum(torch.abs(node_features), dim=-1) != 0
output = model(node_features, batch_mask, adjacency_matrix, distance_matrix, None)
- GraphTransformer 由 Embeddings,Encoder,Generator 构成,根据参数初始化,forward 中 src = node_features,下面以(batch_size,max_size,28)(即分子 padding 后"有" max_size 个原子,每个原子以28维 one-hot 编码)为例说明维度变化,batch_mask 是原子成功编码的标志,只要分子中此原子被编码就会为 True,padding 的不存在原子为 False,用来标明有效长度。adj_matrix 和 distances_matrix 是邻接矩阵和距离矩阵,用于做 Molecule self attention。None 表示不使用 edges_att,原文提到使用 edges_att 并没有提升模型性能。
batch_size=2
for batch in data_loader:
adjacency_matrix, node_features, distance_matrix, y = batch
batch_mask = torch.sum(torch.abs(node_features), dim=-1) != 0
print(node_features)
print(batch_mask)
break
"""
tensor([[[1., 0., 0., 0., 0., 0., 0., 0., 0., 0., 0., 0., 0., 0., 0., 0., 0.,
0., 0., 0., 0., 0., 0., 0., 0., 0., 0., 0.],
[0., 0., 1., 0., 0., 0., 0., 0., 0., 0., 0., 0., 0., 1., 0., 0., 0.,
0., 0., 0., 0., 1., 0., 0., 1., 0., 0., 0.],
[0., 0., 1., 0., 0., 0., 0., 0., 0., 0., 0., 0., 0., 0., 0., 1., 0.,
0., 0., 1., 0., 0., 0., 0., 1., 0., 0., 0.],
[0., 0., 0., 0., 1., 0., 0., 0., 0., 0., 0., 0., 0., 0., 1., 0., 0.,
0., 1., 0., 0., 0., 0., 0., 1., 0., 0., 0.],
[0., 0., 1., 0., 0., 0., 0., 0., 0., 0., 0., 0., 0., 0., 0., 1., 0.,
0., 1., 0., 0., 0., 0., 0., 1., 0., 0., 0.],
[0., 0., 0., 0., 1., 0., 0., 0., 0., 0., 0., 0., 0., 1., 0., 0., 0.,
0., 1., 0., 0., 0., 0., 0., 1., 0., 0., 0.],
[0., 0., 1., 0., 0., 0., 0., 0., 0., 0., 0., 0., 0., 1., 0., 0., 0.,
0., 0., 0., 0., 1., 0., 0., 1., 0., 0., 0.],
[0., 0., 0., 0., 1., 0., 0., 0., 0., 0., 0., 0., 0., 0., 1., 0., 0.,
0., 1., 0., 0., 0., 0., 0., 1., 0., 0., 0.],
[0., 0., 1., 0., 0., 0., 0., 0., 0., 0., 0., 0., 0., 0., 0., 1., 0.,
0., 1., 0., 0., 0., 0., 0., 1., 0., 0., 0.],
[0., 0., 0., 0., 1., 0., 0., 0., 0., 0., 0., 0., 0., 1., 0., 0., 0.,
0., 1., 0., 0., 0., 0., 0., 1., 0., 0., 0.],
[0., 0., 1., 0., 0., 0., 0., 0., 0., 0., 0., 0., 0., 1., 0., 0., 0.,
0., 0., 0., 0., 1., 0., 0., 1., 0., 0., 0.]],
[[1., 0., 0., 0., 0., 0., 0., 0., 0., 0., 0., 0., 0., 0., 0., 0., 0.,
0., 0., 0., 0., 0., 0., 0., 0., 0., 0., 0.],
[0., 0., 1., 0., 0., 0., 0., 0., 0., 0., 0., 0., 0., 1., 0., 0., 0.,
0., 0., 0., 0., 1., 0., 0., 1., 0., 0., 0.],
[0., 0., 1., 0., 0., 0., 0., 0., 0., 0., 0., 0., 0., 0., 0., 1., 0.,
0., 1., 0., 0., 0., 0., 0., 1., 0., 1., 1.],
[0., 0., 1., 0., 0., 0., 0., 0., 0., 0., 0., 0., 0., 0., 1., 0., 0.,
0., 0., 1., 0., 0., 0., 0., 1., 0., 1., 1.],
[0., 0., 1., 0., 0., 0., 0., 0., 0., 0., 0., 0., 0., 0., 1., 0., 0.,
0., 0., 1., 0., 0., 0., 0., 1., 0., 1., 1.],
[0., 0., 1., 0., 0., 0., 0., 0., 0., 0., 0., 0., 0., 0., 1., 0., 0.,
0., 0., 1., 0., 0., 0., 0., 1., 0., 1., 1.],
[0., 0., 1., 0., 0., 0., 0., 0., 0., 0., 0., 0., 0., 0., 0., 1., 0.,
0., 1., 0., 0., 0., 0., 0., 1., 0., 1., 1.],
[0., 0., 1., 0., 0., 0., 0., 0., 0., 0., 0., 0., 0., 0., 1., 0., 0.,
0., 0., 1., 0., 0., 0., 0., 1., 0., 1., 1.],
[0., 0., 0., 0., 1., 0., 0., 0., 0., 0., 0., 0., 0., 1., 0., 0., 0.,
0., 0., 1., 0., 0., 0., 0., 1., 0., 0., 0.],
[0., 0., 0., 0., 0., 0., 0., 0., 0., 0., 0., 0., 0., 0., 0., 0., 0.,
0., 0., 0., 0., 0., 0., 0., 0., 0., 0., 0.],
[0., 0., 0., 0., 0., 0., 0., 0., 0., 0., 0., 0., 0., 0., 0., 0., 0.,
0., 0., 0., 0., 0., 0., 0., 0., 0., 0., 0.]]])
tensor([[ True, True, True, True, True, True, True, True, True, True,
True],
[ True, True, True, True, True, True, True, True, True, False,
False]])
"""
2.2.GraphTransformer
class GraphTransformer(nn.Module):
def __init__(self, encoder, src_embed, generator):
super(GraphTransformer, self).__init__()
self.encoder = encoder
self.src_embed = src_embed
self.generator = generator
def forward(self, src, src_mask, adj_matrix, distances_matrix, edges_att):
"Take in and process masked src and target sequences."
return self.predict(self.encode(src, src_mask, adj_matrix, distances_matrix, edges_att), src_mask)
def encode(self, src, src_mask, adj_matrix, distances_matrix, edges_att):
return self.encoder(self.src_embed(src), src_mask, adj_matrix, distances_matrix, edges_att)
def predict(self, out, out_mask):
return self.generator(out, out_mask)
- 先经过 Encoder 编码,再用 Generator 输出,Encoder 中先对 src 进行Embedding
2.3.Embedding
class Embeddings(nn.Module):
def __init__(self, d_model, d_atom, dropout):
super(Embeddings, self).__init__()
self.lut = nn.Linear(d_atom, d_model)
self.dropout = nn.Dropout(dropout)
def forward(self, x):
return self.dropout(self.lut(x))
- 经过线性变换和 dropout,max_size 个原子的分子被编码为 (batch_size,max_size,1024) 维矩阵,这里没有用 torch.nn.Embedding,Transformer 的实现中使用的是 torch.nn.Embedding
2.4.Encoder
def clones(module, N):
"Produce N identical layers."
return nn.ModuleList([copy.deepcopy(module) for _ in range(N)])
class Encoder(nn.Module):
"Core encoder is a stack of N layers"
def __init__(self, layer, N, scale_norm):
super(Encoder, self).__init__()
self.layers = clones(layer, N)
self.norm = ScaleNorm(layer.size) if scale_norm else LayerNorm(layer.size)
def forward(self, x, mask, adj_matrix, distances_matrix, edges_att):
"Pass the input (and mask) through each layer in turn."
for layer in self.layers:
x = layer(x, mask, adj_matrix, distances_matrix, edges_att)
return self.norm(x)
- Encoder 是 N 个 EncoderLayer 的堆叠,最后添加 Norm 层。Norm 分为 ScaleNorm 和 LayerNorm
2.5.Norm
class LayerNorm(nn.Module):
"Construct a layernorm module (See citation for details)."
def __init__(self, features, eps=1e-6):
super(LayerNorm, self).__init__()
self.a_2 = nn.Parameter(torch.ones(features))
self.b_2 = nn.Parameter(torch.zeros(features))
self.eps = eps
def forward(self, x):
mean = x.mean(-1, keepdim=True)
std = x.std(-1, keepdim=True)
return self.a_2 * (x - mean) / (std + self.eps) + self.b_2
class ScaleNorm(nn.Module):
"""ScaleNorm"""
"All g’s in SCALE NORM are initialized to sqrt(d)"
def __init__(self, scale, eps=1e-5):
super(ScaleNorm, self).__init__()
self.scale = nn.Parameter(torch.tensor(math.sqrt(scale)))
self.eps = eps
def forward(self, x):
norm = self.scale / torch.norm(x, dim=-1, keepdim=True).clamp(min=self.eps)
return x * norm
2.6.EncoderLayer
class EncoderLayer(nn.Module):
"Encoder is made up of self-attn and feed forward (defined below)"
def __init__(self, size, self_attn, feed_forward, dropout, scale_norm, use_adapter):
super(EncoderLayer, self).__init__()
self.self_attn = self_attn
self.feed_forward = feed_forward
self.sublayer = clones(SublayerConnection(size, dropout, scale_norm, use_adapter), 2)
self.size = size
def forward(self, x, mask, adj_matrix, distances_matrix, edges_att):
"Follow Figure 1 (left) for connections."
x = self.sublayer[0](x, lambda x: self.self_attn(x, x, x, adj_matrix, distances_matrix, edges_att, mask))
return self.sublayer[1](x, self.feed_forward)
- EncoderLayer 包含 2 个 SublayerConnection 层,每个 SublayerConnection 层包含一个自注意力层和一个全连接层,SublayerConnection 作为一个类抽象出残差连接
2.7.SublayerConnection
class SublayerConnection(nn.Module):
"""
A residual connection followed by a layer norm.
Note for code simplicity the norm is first as opposed to last.
"""
def __init__(self, size, dropout, scale_norm, use_adapter):
super(SublayerConnection, self).__init__()
self.norm = ScaleNorm(size) if scale_norm else LayerNorm(size)
self.dropout = nn.Dropout(dropout)
self.use_adapter = use_adapter
self.adapter = Adapter(size, 8) if use_adapter else None
def forward(self, x, sublayer):
"Apply residual connection to any sublayer with the same size."
if self.use_adapter:
return x + self.dropout(self.adapter(sublayer(self.norm(x))))
return x + self.dropout(sublayer(self.norm(x)))
- Adapter 暂时不清楚哪里来的…但 run 的时候设置为是 False,所以不影响。forward 通过传入输入和层函数来发挥残差连接的作用
2.8.MultiHeadedAttention
class MultiHeadedAttention(nn.Module):
def __init__(self, h, d_model, dropout=0.1, lambda_attention=0.3, lambda_distance=0.3, trainable_lambda=False,
distance_matrix_kernel='softmax', use_edge_features=False, control_edges=False, integrated_distances=False):
"Take in model size and number of heads."
super(MultiHeadedAttention, self).__init__()
assert d_model % h == 0
self.d_k = d_model // h
self.h = h
self.trainable_lambda = trainable_lambda
if trainable_lambda:
lambda_adjacency = 1. - lambda_attention - lambda_distance
lambdas_tensor = torch.tensor([lambda_attention, lambda_distance, lambda_adjacency], requires_grad=True)
self.lambdas = torch.nn.Parameter(lambdas_tensor)
else:
lambda_adjacency = 1. - lambda_attention - lambda_distance
self.lambdas = (lambda_attention, lambda_distance, lambda_adjacency)
self.linears = clones(nn.Linear(d_model, d_model), 4)
self.attn = None
self.dropout = nn.Dropout(p=dropout)
if distance_matrix_kernel == 'softmax':
self.distance_matrix_kernel = lambda x: F.softmax(-x, dim = -1)
elif distance_matrix_kernel == 'exp':
self.distance_matrix_kernel = lambda x: torch.exp(-x)
self.integrated_distances = integrated_distances
self.use_edge_features = use_edge_features
self.control_edges = control_edges
if use_edge_features:
d_edge = 11 if not integrated_distances else 12
self.edges_feature_layer = EdgeFeaturesLayer(d_model, d_edge, h, dropout)
def forward(self, query, key, value, adj_matrix, distances_matrix, edges_att, mask=None):
"Implements Figure 2"
if mask is not None:
mask = mask.unsqueeze(1)
nbatches = query.size(0)
query, key, value = \
[l(x).view(nbatches, -1, self.h, self.d_k).transpose(1, 2)
for l, x in zip(self.linears, (query, key, value))]
distances_matrix = distances_matrix.masked_fill(mask.repeat(1, mask.shape[-1], 1) == 0, np.inf)
distances_matrix = self.distance_matrix_kernel(distances_matrix)
p_dist = distances_matrix.unsqueeze(1).repeat(1, query.shape[1], 1, 1)
if self.use_edge_features:
if self.integrated_distances:
edges_att = torch.cat((edges_att, distances_matrix.unsqueeze(1)), dim=1)
edges_att = self.edges_feature_layer(edges_att)
x, self.attn, self.self_attn = attention(query, key, value, adj_matrix,
p_dist, edges_att,
mask=mask, dropout=self.dropout,
lambdas=self.lambdas,
trainable_lambda=self.trainable_lambda,
distance_matrix_kernel=self.distance_matrix_kernel,
use_edge_features=self.use_edge_features,
control_edges=self.control_edges)
x = x.transpose(1, 2).contiguous() \
.view(nbatches, -1, self.h * self.d_k)
return self.linears[-1](x)
-
这里的参数基本与 Transformer 中的一致,self.lambdas 是 MAT 中不同于 Transformer 的点,当不训练时设置为定值 -
self.linears 基本对应 Transformer中的
W
Q
,
W
K
,
W
V
,
W
O
W^Q,W^K,W^V,W^O
WQ,WK,WV,WO,但维度不一致,此代码中没有进行 concat,而是统一处理 -
forward 中的 query, key, value 都是 x,即 node_featues 经过 Embedding 后的矩阵,维度是 (max_size,1024),mask 是 batch_mask,标明有效长度的矩阵,维度是 (batch_size,max_size),unsqueeze 在维度为 1 处增加维度,最终维度变为 (batch_size,1,max_size),示例如下:
import torch
batch_size=2
max_size=14
mask=torch.ones((batch_size,max_size))
print(mask)
print(mask.unsqueeze(1))
"""
tensor([[1., 1., 1., 1., 1., 1., 1., 1., 1., 1., 1., 1., 1., 1.],
[1., 1., 1., 1., 1., 1., 1., 1., 1., 1., 1., 1., 1., 1.]])
tensor([[[1., 1., 1., 1., 1., 1., 1., 1., 1., 1., 1., 1., 1., 1.]],
[[1., 1., 1., 1., 1., 1., 1., 1., 1., 1., 1., 1., 1., 1.]]])
"""
- 接下来用线性层将 query,key,value 进行转换,但并没有维度变化,它们的维度仍然是 (batch_size,max_size,d_model),继续使用 view(nbatches, -1, self.h, self.d_k).transpose(1, 2) 进行维度转换,最后它们的维度变为(batch_size,h,max_size,d_k),示例如下:
query=torch.Tensor(64,14,1024)
l=torch.nn.Linear(1024,1024)
nbatches,h,d_k=64,16,64
l(query).view(nbatches, -1, h, d_k).transpose(1, 2).shape
- mask.repeat(1, mask.shape[-1], 1) == 0 对之前添加的维度扩充,变为 (batch_size,max_size,max_size),这是为了与 distance_matrix 的维度匹配,示例如下:
mask=torch.tensor([[ True, True, True, True, True, True, True, True, True, True,
True],
[ True, True, True, True, True, True, True, True, True, False,
False]])
mask=mask.unsqueeze(1).repeat(1, mask.shape[-1], 1)
print(mask.shape)
print(mask)
"""
tensor([[[ True, True, True, True, True, True, True, True, True, True,
True],
[ True, True, True, True, True, True, True, True, True, True,
True],
[ True, True, True, True, True, True, True, True, True, True,
True],
[ True, True, True, True, True, True, True, True, True, True,
True],
[ True, True, True, True, True, True, True, True, True, True,
True],
[ True, True, True, True, True, True, True, True, True, True,
True],
[ True, True, True, True, True, True, True, True, True, True,
True],
[ True, True, True, True, True, True, True, True, True, True,
True],
[ True, True, True, True, True, True, True, True, True, True,
True],
[ True, True, True, True, True, True, True, True, True, True,
True],
[ True, True, True, True, True, True, True, True, True, True,
True]],
[[ True, True, True, True, True, True, True, True, True, False,
False],
[ True, True, True, True, True, True, True, True, True, False,
False],
[ True, True, True, True, True, True, True, True, True, False,
False],
[ True, True, True, True, True, True, True, True, True, False,
False],
[ True, True, True, True, True, True, True, True, True, False,
False],
[ True, True, True, True, True, True, True, True, True, False,
False],
[ True, True, True, True, True, True, True, True, True, False,
False],
[ True, True, True, True, True, True, True, True, True, False,
False],
[ True, True, True, True, True, True, True, True, True, False,
False],
[ True, True, True, True, True, True, True, True, True, False,
False],
[ True, True, True, True, True, True, True, True, True, False,
False]]])
"""
- 对 mask 为 False 的地方在 distance_matrix 填充 np.inf,再进行 lambda x: torch.exp(-x) 的映射,距离为无穷大的地方会变成0
- distances_matrix.unsqueeze(1).repeat(1, query.shape[1], 1, 1),p_distance 的维度变为(batch_size,h,max_size,max_size)
- use_edge_features 为 False,将数据输入 attention
2.9.attention
def attention(query, key, value, adj_matrix, distances_matrix, edges_att,
mask=None, dropout=None,
lambdas=(0.3, 0.3, 0.4), trainable_lambda=False,
distance_matrix_kernel=None, use_edge_features=False, control_edges=False,
eps=1e-6, inf=1e12):
"Compute 'Scaled Dot Product Attention'"
d_k = query.size(-1)
scores = torch.matmul(query, key.transpose(-2, -1)) \
/ math.sqrt(d_k)
if mask is not None:
scores = scores.masked_fill(mask.unsqueeze(1).repeat(1, query.shape[1], query.shape[2], 1) == 0, -inf)
p_attn = F.softmax(scores, dim = -1)
if use_edge_features:
adj_matrix = edges_att.view(adj_matrix.shape)
adj_matrix = adj_matrix / (adj_matrix.sum(dim=-1).unsqueeze(2) + eps)
adj_matrix = adj_matrix.unsqueeze(1).repeat(1, query.shape[1], 1, 1)
p_adj = adj_matrix
p_dist = distances_matrix
if trainable_lambda:
softmax_attention, softmax_distance, softmax_adjacency = lambdas.cuda()
p_weighted = softmax_attention * p_attn + softmax_distance * p_dist + softmax_adjacency * p_adj
else:
lambda_attention, lambda_distance, lambda_adjacency = lambdas
p_weighted = lambda_attention * p_attn + lambda_distance * p_dist + lambda_adjacency * p_adj
if dropout is not None:
p_weighted = dropout(p_weighted)
atoms_featrues = torch.matmul(p_weighted, value)
return atoms_featrues, p_weighted, p_attn
- scores 是 query 和 key 的相似度得分,
Q
b
a
t
c
h
_
s
i
z
e
×
h
×
m
a
x
_
s
i
z
e
×
d
_
k
K
b
a
t
c
h
_
s
i
z
e
×
h
×
d
_
k
×
m
a
x
_
s
i
z
e
=
S
b
a
t
c
h
_
s
i
z
e
×
h
×
m
a
x
_
s
i
z
e
×
m
a
x
_
s
i
z
e
Q_{batch\_size\times h\times max\_size\times d\_k} K_{batch\_size\times h\times d\_k\times max\_size}=S_{batch\_size\times h\times max\_size\times max\_size}
Qbatch_size×h×max_size×d_k?Kbatch_size×h×d_k×max_size?=Sbatch_size×h×max_size×max_size?,mask 的维度是(batch_size,1,max_size),mask.unsqueeze(1).repeat(1, query.shape[1], query.shape[2], 1) 后的维度是(batch_size,h,max_size,max_size)与scores的维度匹配,将 padding 的部分scores设为负无穷,相当于注意力为0
- adj_matrix 的维度是(batch_size,max_size,max_size),adj_matrix.sum(dim=-1) 得到的是矩阵维度是 (batch_size,max_size),代表的意义是 batch 中每个分子的原子所连原子(包括本身)的数量,第一个原子是 dummy_node。示例如下
batch_size=1
eps=1e-6
adj_matrix=torch.tensor([
[[0., 0., 0., 0., 0., 0., 0., 0., 0., 0., 0., 0., 0., 0.],
[0., 1., 1., 0., 0., 0., 0., 0., 0., 0., 0., 0., 0., 0.],
[0., 1., 1., 1., 1., 0., 0., 0., 0., 0., 0., 0., 0., 0.],
[0., 0., 1., 1., 0., 0., 0., 0., 0., 0., 0., 0., 0., 0.],
[0., 0., 1., 0., 1., 1., 1., 0., 0., 0., 0., 0., 0., 0.],
[0., 0., 0., 0., 1., 1., 0., 0., 0., 0., 0., 0., 0., 0.],
[0., 0., 0., 0., 1., 0., 1., 1., 0., 0., 0., 1., 0., 0.],
[0., 0., 0., 0., 0., 0., 1., 1., 1., 0., 0., 0., 0., 0.],
[0., 0., 0., 0., 0., 0., 0., 1., 1., 1., 0., 0., 0., 0.],
[0., 0., 0., 0., 0., 0., 0., 0., 1., 1., 1., 0., 1., 0.],
[0., 0., 0., 0., 0., 0., 0., 0., 0., 1., 1., 1., 0., 0.],
[0., 0., 0., 0., 0., 0., 1., 0., 0., 0., 1., 1., 0., 0.],
[0., 0., 0., 0., 0., 0., 0., 0., 0., 1., 0., 0., 1., 1.],
[0., 0., 0., 0., 0., 0., 0., 0., 0., 0., 0., 0., 1., 1.]]])
adj_matrix / (adj_matrix.sum(dim=-1).unsqueeze(2) + eps)
"""
tensor([[[0.0000, 0.0000, 0.0000, 0.0000, 0.0000, 0.0000, 0.0000, 0.0000,
0.0000, 0.0000, 0.0000, 0.0000, 0.0000, 0.0000],
[0.0000, 0.5000, 0.5000, 0.0000, 0.0000, 0.0000, 0.0000, 0.0000,
0.0000, 0.0000, 0.0000, 0.0000, 0.0000, 0.0000],
[0.0000, 0.2500, 0.2500, 0.2500, 0.2500, 0.0000, 0.0000, 0.0000,
0.0000, 0.0000, 0.0000, 0.0000, 0.0000, 0.0000],
[0.0000, 0.0000, 0.5000, 0.5000, 0.0000, 0.0000, 0.0000, 0.0000,
0.0000, 0.0000, 0.0000, 0.0000, 0.0000, 0.0000],
[0.0000, 0.0000, 0.2500, 0.0000, 0.2500, 0.2500, 0.2500, 0.0000,
0.0000, 0.0000, 0.0000, 0.0000, 0.0000, 0.0000],
[0.0000, 0.0000, 0.0000, 0.0000, 0.5000, 0.5000, 0.0000, 0.0000,
0.0000, 0.0000, 0.0000, 0.0000, 0.0000, 0.0000],
[0.0000, 0.0000, 0.0000, 0.0000, 0.2500, 0.0000, 0.2500, 0.2500,
0.0000, 0.0000, 0.0000, 0.2500, 0.0000, 0.0000],
[0.0000, 0.0000, 0.0000, 0.0000, 0.0000, 0.0000, 0.3333, 0.3333,
0.3333, 0.0000, 0.0000, 0.0000, 0.0000, 0.0000],
[0.0000, 0.0000, 0.0000, 0.0000, 0.0000, 0.0000, 0.0000, 0.3333,
0.3333, 0.3333, 0.0000, 0.0000, 0.0000, 0.0000],
[0.0000, 0.0000, 0.0000, 0.0000, 0.0000, 0.0000, 0.0000, 0.0000,
0.2500, 0.2500, 0.2500, 0.0000, 0.2500, 0.0000],
[0.0000, 0.0000, 0.0000, 0.0000, 0.0000, 0.0000, 0.0000, 0.0000,
0.0000, 0.3333, 0.3333, 0.3333, 0.0000, 0.0000],
[0.0000, 0.0000, 0.0000, 0.0000, 0.0000, 0.0000, 0.3333, 0.0000,
0.0000, 0.0000, 0.3333, 0.3333, 0.0000, 0.0000],
[0.0000, 0.0000, 0.0000, 0.0000, 0.0000, 0.0000, 0.0000, 0.0000,
0.0000, 0.3333, 0.0000, 0.0000, 0.3333, 0.3333],
[0.0000, 0.0000, 0.0000, 0.0000, 0.0000, 0.0000, 0.0000, 0.0000,
0.0000, 0.0000, 0.0000, 0.0000, 0.5000, 0.5000]]])
"""
-
p_adj 维度变为(batch_size,h,max_size,max_size),与 p_dis 和 p_attn 维度统一,与对应稀疏相乘后得到最后的 MolculeAttention 得分,p_weighted 维度也是 (batch_size,h,max_size,max_size) -
value 的维度是 (batch_size,h,max_size,d_k),atoms_featrues 最终的维度为 (batch_size,h,max_size,d_k) -
x.transpose(1, 2).contiguous().view(nbatches, -1, self.h * self.d_k) 将 x 的维度先变为 (batch_size,max_size,h,d_k),再进一步变成 (batch_size,max_size,d_model),相当于进行了 concat,最后进行线性映射,维度不改变
2.10.PositionwiseFeedForward
class PositionwiseFeedForward(nn.Module):
"Implements FFN equation."
def __init__(self, d_model, N_dense, dropout=0.1, leaky_relu_slope=0.0, dense_output_nonlinearity='relu'):
super(PositionwiseFeedForward, self).__init__()
self.N_dense = N_dense
self.linears = clones(nn.Linear(d_model, d_model), N_dense)
self.dropout = clones(nn.Dropout(dropout), N_dense)
self.leaky_relu_slope = leaky_relu_slope
if dense_output_nonlinearity == 'relu':
self.dense_output_nonlinearity = lambda x: F.leaky_relu(x, negative_slope=self.leaky_relu_slope)
elif dense_output_nonlinearity == 'tanh':
self.tanh = torch.nn.Tanh()
self.dense_output_nonlinearity = lambda x: self.tanh(x)
elif dense_output_nonlinearity == 'none':
self.dense_output_nonlinearity = lambda x: x
def forward(self, x):
if self.N_dense == 0:
return x
for i in range(len(self.linears)-1):
x = self.dropout[i](F.leaky_relu(self.linears[i](x), negative_slope=self.leaky_relu_slope))
return self.dropout[-1](self.dense_output_nonlinearity(self.linears[-1](x)))
- N_dense 是 线性层的数量,最后输出的维度不变,进入下一个 EncoderLayer 块的维度是 (batch_size,max_size,d_model),与刚经过 Embedding 的维度一致,重复 N 次后进入 Norm 层,再进入 Generator
2.11.Generator
class Generator(nn.Module):
"Define standard linear + softmax generation step."
def __init__(self, d_model, aggregation_type='mean', n_output=1, n_layers=1,
leaky_relu_slope=0.01, dropout=0.0, scale_norm=False):
super(Generator, self).__init__()
if n_layers == 1:
self.proj = nn.Linear(d_model, n_output)
else:
self.proj = []
for i in range(n_layers-1):
self.proj.append(nn.Linear(d_model, d_model))
self.proj.append(nn.LeakyReLU(leaky_relu_slope))
self.proj.append(ScaleNorm(d_model) if scale_norm else LayerNorm(d_model))
self.proj.append(nn.Dropout(dropout))
self.proj.append(nn.Linear(d_model, n_output))
self.proj = torch.nn.Sequential(*self.proj)
self.aggregation_type = aggregation_type
def forward(self, x, mask):
mask = mask.unsqueeze(-1).float()
out_masked = x * mask
if self.aggregation_type == 'mean':
out_sum = out_masked.sum(dim=1)
mask_sum = mask.sum(dim=(1))
out_avg_pooling = out_sum / mask_sum
elif self.aggregation_type == 'sum':
out_sum = out_masked.sum(dim=1)
out_avg_pooling = out_sum
elif self.aggregation_type == 'dummy_node':
out_avg_pooling = out_masked[:,0]
projected = self.proj(out_avg_pooling)
return projected
- forward 中的 mask 是 batch_mask,维度是 (batch_size,max_size),x 的维度是 (batch_size,max_size,d_model),padding 的部分为 0,相乘有 broadcast,最终 out_masked 维度与 x 维度一致,之后进行聚合,消除 max_size 维度,再进入 Sequential,最终输出(batch_size,n_output)维度的预测值
2.12.总结
GraphTransformer(
(encoder): Encoder(
(layers): ModuleList(
(0): EncoderLayer(
(self_attn): MultiHeadedAttention(
(linears): ModuleList(
(0): Linear(in_features=1024, out_features=1024, bias=True)
(1): Linear(in_features=1024, out_features=1024, bias=True)
(2): Linear(in_features=1024, out_features=1024, bias=True)
(3): Linear(in_features=1024, out_features=1024, bias=True)
)
(dropout): Dropout(p=0.0, inplace=False)
)
(feed_forward): PositionwiseFeedForward(
(linears): ModuleList(
(0): Linear(in_features=1024, out_features=1024, bias=True)
)
(dropout): ModuleList(
(0): Dropout(p=0.0, inplace=False)
)
)
(sublayer): ModuleList(
(0): SublayerConnection(
(norm): LayerNorm()
(dropout): Dropout(p=0.0, inplace=False)
)
(1): SublayerConnection(
(norm): LayerNorm()
(dropout): Dropout(p=0.0, inplace=False)
)
)
)
(1): EncoderLayer(
(self_attn): MultiHeadedAttention(
(linears): ModuleList(
(0): Linear(in_features=1024, out_features=1024, bias=True)
(1): Linear(in_features=1024, out_features=1024, bias=True)
(2): Linear(in_features=1024, out_features=1024, bias=True)
(3): Linear(in_features=1024, out_features=1024, bias=True)
)
(dropout): Dropout(p=0.0, inplace=False)
)
(feed_forward): PositionwiseFeedForward(
(linears): ModuleList(
(0): Linear(in_features=1024, out_features=1024, bias=True)
)
(dropout): ModuleList(
(0): Dropout(p=0.0, inplace=False)
)
)
(sublayer): ModuleList(
(0): SublayerConnection(
(norm): LayerNorm()
(dropout): Dropout(p=0.0, inplace=False)
)
(1): SublayerConnection(
(norm): LayerNorm()
(dropout): Dropout(p=0.0, inplace=False)
)
)
)
(2): EncoderLayer(
(self_attn): MultiHeadedAttention(
(linears): ModuleList(
(0): Linear(in_features=1024, out_features=1024, bias=True)
(1): Linear(in_features=1024, out_features=1024, bias=True)
(2): Linear(in_features=1024, out_features=1024, bias=True)
(3): Linear(in_features=1024, out_features=1024, bias=True)
)
(dropout): Dropout(p=0.0, inplace=False)
)
(feed_forward): PositionwiseFeedForward(
(linears): ModuleList(
(0): Linear(in_features=1024, out_features=1024, bias=True)
)
(dropout): ModuleList(
(0): Dropout(p=0.0, inplace=False)
)
)
(sublayer): ModuleList(
(0): SublayerConnection(
(norm): LayerNorm()
(dropout): Dropout(p=0.0, inplace=False)
)
(1): SublayerConnection(
(norm): LayerNorm()
(dropout): Dropout(p=0.0, inplace=False)
)
)
)
(3): EncoderLayer(
(self_attn): MultiHeadedAttention(
(linears): ModuleList(
(0): Linear(in_features=1024, out_features=1024, bias=True)
(1): Linear(in_features=1024, out_features=1024, bias=True)
(2): Linear(in_features=1024, out_features=1024, bias=True)
(3): Linear(in_features=1024, out_features=1024, bias=True)
)
(dropout): Dropout(p=0.0, inplace=False)
)
(feed_forward): PositionwiseFeedForward(
(linears): ModuleList(
(0): Linear(in_features=1024, out_features=1024, bias=True)
)
(dropout): ModuleList(
(0): Dropout(p=0.0, inplace=False)
)
)
(sublayer): ModuleList(
(0): SublayerConnection(
(norm): LayerNorm()
(dropout): Dropout(p=0.0, inplace=False)
)
(1): SublayerConnection(
(norm): LayerNorm()
(dropout): Dropout(p=0.0, inplace=False)
)
)
)
(4): EncoderLayer(
(self_attn): MultiHeadedAttention(
(linears): ModuleList(
(0): Linear(in_features=1024, out_features=1024, bias=True)
(1): Linear(in_features=1024, out_features=1024, bias=True)
(2): Linear(in_features=1024, out_features=1024, bias=True)
(3): Linear(in_features=1024, out_features=1024, bias=True)
)
(dropout): Dropout(p=0.0, inplace=False)
)
(feed_forward): PositionwiseFeedForward(
(linears): ModuleList(
(0): Linear(in_features=1024, out_features=1024, bias=True)
)
(dropout): ModuleList(
(0): Dropout(p=0.0, inplace=False)
)
)
(sublayer): ModuleList(
(0): SublayerConnection(
(norm): LayerNorm()
(dropout): Dropout(p=0.0, inplace=False)
)
(1): SublayerConnection(
(norm): LayerNorm()
(dropout): Dropout(p=0.0, inplace=False)
)
)
)
(5): EncoderLayer(
(self_attn): MultiHeadedAttention(
(linears): ModuleList(
(0): Linear(in_features=1024, out_features=1024, bias=True)
(1): Linear(in_features=1024, out_features=1024, bias=True)
(2): Linear(in_features=1024, out_features=1024, bias=True)
(3): Linear(in_features=1024, out_features=1024, bias=True)
)
(dropout): Dropout(p=0.0, inplace=False)
)
(feed_forward): PositionwiseFeedForward(
(linears): ModuleList(
(0): Linear(in_features=1024, out_features=1024, bias=True)
)
(dropout): ModuleList(
(0): Dropout(p=0.0, inplace=False)
)
)
(sublayer): ModuleList(
(0): SublayerConnection(
(norm): LayerNorm()
(dropout): Dropout(p=0.0, inplace=False)
)
(1): SublayerConnection(
(norm): LayerNorm()
(dropout): Dropout(p=0.0, inplace=False)
)
)
)
(6): EncoderLayer(
(self_attn): MultiHeadedAttention(
(linears): ModuleList(
(0): Linear(in_features=1024, out_features=1024, bias=True)
(1): Linear(in_features=1024, out_features=1024, bias=True)
(2): Linear(in_features=1024, out_features=1024, bias=True)
(3): Linear(in_features=1024, out_features=1024, bias=True)
)
(dropout): Dropout(p=0.0, inplace=False)
)
(feed_forward): PositionwiseFeedForward(
(linears): ModuleList(
(0): Linear(in_features=1024, out_features=1024, bias=True)
)
(dropout): ModuleList(
(0): Dropout(p=0.0, inplace=False)
)
)
(sublayer): ModuleList(
(0): SublayerConnection(
(norm): LayerNorm()
(dropout): Dropout(p=0.0, inplace=False)
)
(1): SublayerConnection(
(norm): LayerNorm()
(dropout): Dropout(p=0.0, inplace=False)
)
)
)
(7): EncoderLayer(
(self_attn): MultiHeadedAttention(
(linears): ModuleList(
(0): Linear(in_features=1024, out_features=1024, bias=True)
(1): Linear(in_features=1024, out_features=1024, bias=True)
(2): Linear(in_features=1024, out_features=1024, bias=True)
(3): Linear(in_features=1024, out_features=1024, bias=True)
)
(dropout): Dropout(p=0.0, inplace=False)
)
(feed_forward): PositionwiseFeedForward(
(linears): ModuleList(
(0): Linear(in_features=1024, out_features=1024, bias=True)
)
(dropout): ModuleList(
(0): Dropout(p=0.0, inplace=False)
)
)
(sublayer): ModuleList(
(0): SublayerConnection(
(norm): LayerNorm()
(dropout): Dropout(p=0.0, inplace=False)
)
(1): SublayerConnection(
(norm): LayerNorm()
(dropout): Dropout(p=0.0, inplace=False)
)
)
)
)
(norm): LayerNorm()
)
(src_embed): Embeddings(
(lut): Linear(in_features=28, out_features=1024, bias=True)
(dropout): Dropout(p=0.0, inplace=False)
)
(generator): Generator(
(proj): Linear(in_features=1024, out_features=1, bias=True)
)
)
- 模型构建基本与 Transformer 一致,不同之处是没有进行位置编码,且 attention 略微不同,除了进行自注意力,还利用了邻接矩阵和距离矩阵的信息,这里没有使用 use_edge_features。另外现在不清楚 PositionGenerator 和 Adapter 的作用
|