OpenCV 例程200篇 总目录-202205更新
【youcans 的 OpenCV 例程200篇】180.基于距离变换的分水岭算法 【youcans 的 OpenCV 例程200篇】181.基于 Sobel 梯度的分水岭算法 【youcans 的 OpenCV 例程200篇】182.基于形态学梯度的分水岭算法 【youcans 的 OpenCV 例程200篇】183.基于轮廓标记的分水岭算法
【youcans 的 OpenCV 例程200篇】183.基于轮廓标记的分水岭算法
7. 图像分割之分水岭算法
分水岭算法是一种图像区域分割法,以临近像素间的相似性作为重要特征,从而将空间位置相近且灰度值相近的像素点互相连接起来,构成一个封闭的轮廓。
分水岭算法是基于形态学的图像分割方法,体现了边缘检测、阈值处理和区域提取的概念和思想,往往会产生更稳定的分割结果。算法的实现过程可以理解为洪水淹没的过程:最低点首先被淹没,然后水逐渐淹没整个山谷;水位升高到一定高度就会溢出,于是在溢出位置修建堤坝;不断提高水位,重复上述过程,直到所有的点全部被淹没;所建立的一系列堤坝就成为分隔各个盆地的分水岭。
分水岭的计算过程是一个迭代标注过程,通过寻找集水盆和分水岭对图像进行分割。经典的分水岭算法分为排序过程和淹没过程两个步骤,首先对每个像素的灰度级从低到高排序,然后在从低到高的淹没过程中,对每一个局部极小值在 h 阶高度的影响域进行判断及标注。
OpenCV 提供了函数 cv.watershed 实现基于标记的分水岭算法。
使用函数 cv.watershed 需要输入一个CV_32S 类型的标记图像,图像中每个非零像素代表一个标签。对图像中部分像素做标记,表明它的所属区域是已知的。
cv.watershed(image, markers[, ] ) → markers
参数说明:
- image:输入图像,8-bit/3-channel 彩色图像
- markers:标记图像,32-bit 单通道图像,大小与 image 相同
注意事项:
- 分水岭算法要求必须在标记图像 markers 中用索引勾勒出需要分割的区域,每个区域被赋值为 1、2、3… 等索引编号,对应于不同的目标物体。
- 图像标记 markers 中未知区域的像素值设置为 0,通过分水岭算法确定这些像素属于背景还是前景区域。
- 输出的图像标记 markers 中,每个像素都被赋值为 1、2、3… 等索引编号,或以 -1 表示区域之间的边界(分水岭)。
OpenCV 提供了函数 cv.distanceTransform 实现距离变换,计算图像中每个像素到最近的零像素点的距离。
函数说明:
cv.distanceTransform(src, distanceType, maskSize[, dst=None, dstType=CV_32F]) → dst
cv.distanceTransformWithLabels(src, distanceType, maskSize[, dst=None, labels=None, labelType=DIST_LABEL_CCOMP]) → dst, labels
参数说明:
- src:输入图像,8-bit 单通道灰度图像
- distanceType:距离的类型
- cv.DIST_USER:用户定义的距离
- cv.DIST_L1:
d
i
s
t
=
∣
x
1
?
x
2
∣
+
∣
y
1
?
y
2
∣
dist = |x1-x2|+|y1-y2|
dist=∣x1?x2∣+∣y1?y2∣
- cv.DIST_L2:欧几里德距离
- cv.DIST_C:
d
i
s
t
=
m
a
x
(
∣
x
1
?
x
2
∣
,
∣
y
1
?
y
2
∣
)
dist = max(|x1-x2|, |y1-y2|)
dist=max(∣x1?x2∣,∣y1?y2∣)
- maskSize:距离变换遮罩的大小,通常取 3, 5
- labelType:生成的标签数组的类型
- cv.DIST_LABEL_CCOMP:每个连接的零组件(及最接近连接组件的所有非零像素)被指定相同的标签
- cv.DIST_LABEL_PIXEL:每个零像素(及离它最近的所有非零像素)都有自己的标签
- dst:计算距离的输出图像,8-bit 或 32-bit 单通道图像,大小与 src 相同
- labels:标签的输出图像,CV_32SC1类型, 大小与 src 相同
例程 11.40 基于轮廓标记的分水岭算法
基于标记的分水岭算法的思想是利用先验知识来帮助分割。本例程先用梯度算子进行边缘检测,然后通过查找图像轮廓,生成标记图像来引导分割。
基于轮廓标记的分水岭算法的步骤为:
(1)对图像进行梯度处理获得梯度图像; (2)对梯度图像查找和绘制轮廓; (3)基于轮廓图像生成标记图像; (4)基于标记图像使用分水岭算法进行分割,得到各个分割目标的轮廓; (5)把目标的轮廓添加到原始图像上; (6)用随机颜色填充分割图像。
img = cv2.imread("../images/imgLena.tif", flags=1)
gray = cv2.cvtColor(img, cv2.COLOR_BGR2GRAY)
Gauss = cv2.GaussianBlur(gray, (5,5), sigmaX=4.0)
grad = cv2.Canny(Gauss, 50, 150)
grad, contours, hierarchy = cv2.findContours(grad, cv2.RETR_EXTERNAL, cv2.CHAIN_APPROX_SIMPLE)
markers = np.zeros(img.shape[:2], np.int32)
for index in range(len(contours)):
markers = cv2.drawContours(markers, contours, index, (index, index, index), 1, 8, hierarchy)
ContoursMarkers = np.zeros(img.shape[:2], np.uint8)
ContoursMarkers[markers>0] = 255
markers = cv2.watershed(img, markers)
WatershedMarkers = cv2.convertScaleAbs(markers)
bgrMarkers = np.zeros_like(img)
for i in range(len(contours)):
colorKind = [np.random.randint(0, 255), np.random.randint(0, 255), np.random.randint(0, 255)]
bgrMarkers[markers==i] = colorKind
bgrFilled = cv2.addWeighted(img, 0.67, bgrMarkers, 0.33, 0)
print(len(contours))
plt.figure(figsize=(10, 6))
plt.subplot(231), plt.axis('off'), plt.title("Origin image")
plt.imshow(cv2.cvtColor(img, cv2.COLOR_BGR2RGB))
plt.subplot(232), plt.axis('off'), plt.title("Gradient")
plt.imshow(grad, 'gray')
plt.subplot(233), plt.axis('off'), plt.title("Contours markers")
plt.imshow(ContoursMarkers, 'gray')
plt.subplot(234), plt.axis('off'), plt.title("Watershed markers")
plt.imshow(WatershedMarkers, 'gray')
plt.subplot(235), plt.axis('off'), plt.title("Color Markers")
plt.imshow(cv2.cvtColor(bgrMarkers, cv2.COLOR_BGR2RGB))
plt.subplot(236), plt.axis('off'), plt.title("Cutted image")
plt.imshow(cv2.cvtColor(bgrFilled, cv2.COLOR_BGR2RGB))
plt.tight_layout()
plt.show()
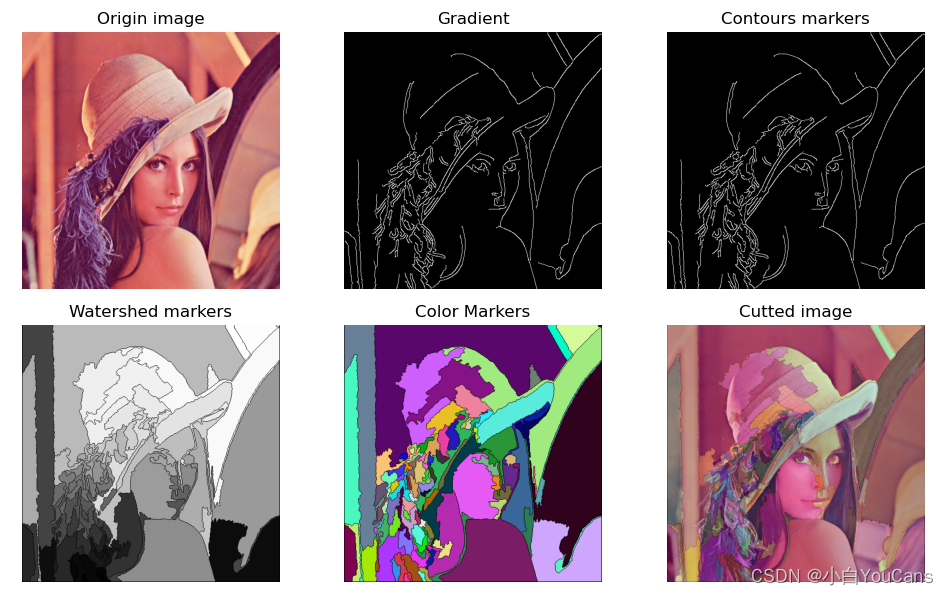
(本节完)
版权声明:
OpenCV 例程200篇 总目录-202205更新 youcans@xupt 原创作品,转载必须标注原文链接:(https://blog.csdn.net/youcans/article/details/124813571)
Copyright 2022 youcans, XUPT Crated:2022-5-15
欢迎关注 『youcans 的 OpenCV 例程 200 篇』 系列,持续更新中 欢迎关注 『youcans 的 OpenCV学习课』 系列,持续更新中
【youcans 的 OpenCV 例程200篇】147. 图像分割之孤立点检测 【youcans 的 OpenCV 例程200篇】148. 图像分割之线检测 【youcans 的 OpenCV 例程200篇】149. 图像分割之边缘模型 【youcans 的 OpenCV 例程200篇】150. 边缘检测梯度算子 【youcans 的 OpenCV 例程200篇】151. 边缘检测中的平滑处理 【youcans 的 OpenCV 例程200篇】152. 边缘检测之 LoG 算子 【youcans 的 OpenCV 例程200篇】153. 边缘检测之 DoG 算子 【youcans 的 OpenCV 例程200篇】154. 边缘检测之 Canny 算子 【youcans 的 OpenCV 例程200篇】155. 边缘连接的局部处理方法 【youcans 的 OpenCV 例程200篇】156. 边缘连接局部处理的简化算法 【youcans 的 OpenCV 例程200篇】157. 霍夫变换直线检测 【youcans 的 OpenCV 例程200篇】158. 阈值处理之固定阈值法 【youcans 的 OpenCV 例程200篇】159. 图像分割之全局阈值处理 【youcans 的 OpenCV 例程200篇】160. 图像处理之OTSU 方法 【youcans 的 OpenCV 例程200篇】161. OTSU 阈值处理算法的实现 【youcans 的 OpenCV 例程200篇】162. 全局阈值处理改进方法 【youcans 的 OpenCV 例程200篇】163. 基于边缘信息改进全局阈值处理 【youcans 的 OpenCV 例程200篇】164.使用 Laplace 边缘信息改进全局阈值处理 【youcans 的 OpenCV 例程200篇】165.多阈值 OTSU 处理方法 【youcans 的 OpenCV 例程200篇】166.自适应阈值处理 【youcans 的 OpenCV 例程200篇】167.基于移动平均的可变阈值处理 【youcans 的 OpenCV 例程200篇】168.图像分割之区域生长 【youcans 的 OpenCV 例程200篇】169.图像分割之区域分离 【youcans 的 OpenCV 例程200篇】170.图像分割之K均值聚类 【youcans 的 OpenCV 例程200篇】171.SLIC 超像素区域分割 【youcans 的 OpenCV 例程200篇】172.SLIC 超像素区域分割算法比较 【youcans 的 OpenCV 例程200篇】173.SEEDS 超像素区域分割 【youcans 的 OpenCV 例程200篇】174.LSC 超像素区域分割 【youcans 的 OpenCV 例程200篇】175.超像素区域分割方法比较 【youcans 的 OpenCV 例程200篇】176.图像分割之均值漂移算法 【youcans 的 OpenCV 例程200篇】177.图像分割之 GraphCuts 图割法 【youcans 的 OpenCV 例程200篇】178.图像分割之 GrabCut 图割法(框选前景) 【youcans 的 OpenCV 例程200篇】179.图像分割之 GrabCut 图割法(掩模图像) 【youcans 的 OpenCV 例程200篇】180.基于距离变换的分水岭算法 【youcans 的 OpenCV 例程200篇】181.基于 Sobel 梯度的分水岭算法 【youcans 的 OpenCV 例程200篇】182.基于形态学梯度的分水岭算法 【youcans 的 OpenCV 例程200篇】183.基于轮廓标记的分水岭算法
|