什么是激活函数?
Sigmoid函数
sigmoid函数是最经典、最早使用的激活函数,公式如下:
ρ
=
1
1
+
e
?
z
\rho = \frac{1}{1+ e^{-z}}
ρ=1+e?z1?
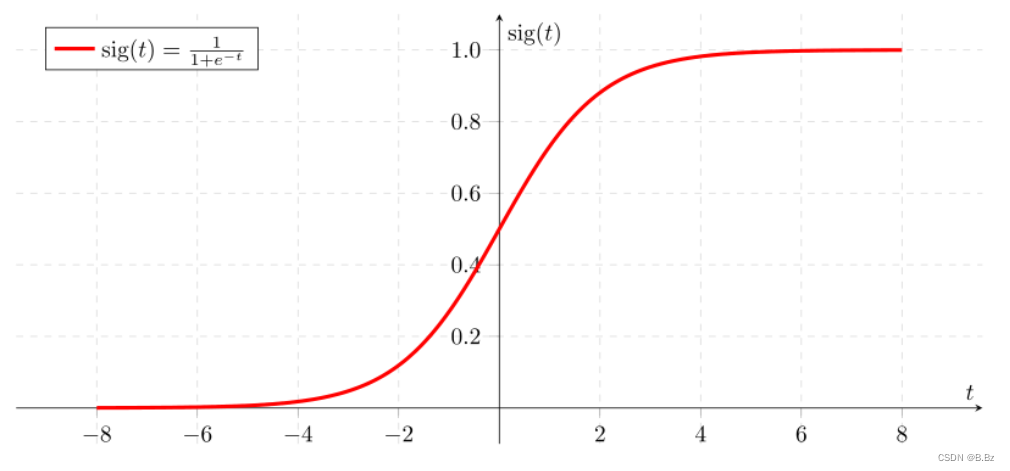
- 激活函数Sigmoid在定义域内处处可以求导,当输入一个较小或者较大的数据时,该函数的导数会变得很小,梯度趋近于0。
- 如果每次梯度值都减小,神经网络具有很多层,当梯度穿过很多层,会逐渐趋近于0,出现
梯度消失现象 ,模型无法继续收敛,sigmoid函数以前被广泛使用,现在很少被人使用。
import torch
import torch.nn as nn
x = torch.tensor([-1.0,1.0,2.0,3.0])
output = torch.sigmoid(x)
print(output)
s = nn.Sigmoid()
output = s(x)
print(output)

Tanh激活函数
-
Tanh是双曲函数中的双曲正切函数.在数学中, 双曲正切函数都是由双曲正弦函数和双曲余弦函数推导而来 -
t
a
n
h
(
x
)
=
e
x
?
e
?
x
e
x
+
e
?
x
tanh(x) = \frac{e^x - e^{-x}}{e^x + e^{-x}}
tanh(x)=ex+e?xex?e?x?
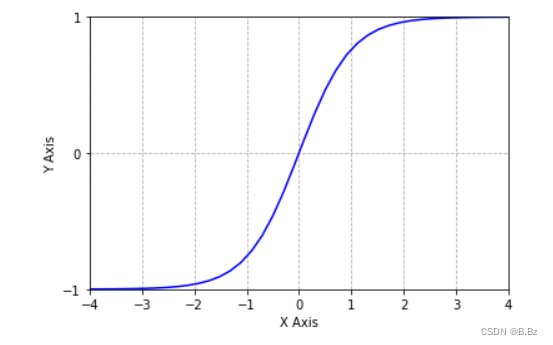
- 和Sigmoid函数很相似,但是Tanh函数的输出范围为(-1,1),Sigmoid函数的输出范围为(0,1)。
- PyTorch中同样有两种实现方式
import torch
import torch.nn as nn
output = torch.tanh(x)
print(output)
t = nn.Tanh()
output = t(x)
print(output)

ReLU激活函数
ReLU函数是现在最常用的激活函数之一:
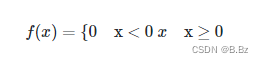
import torch
import torch.nn as nn
output = torch.relu(x)
print(output)
t = nn.ReLU()
output = t(x)
print(output)
总结
-
解决二分类问题, 我们一般会将最后一层设置为Sigmoid函数层. 因此, 从Sigmoid函数图像可以看出,该函数范围为(0,1) 这样可以很好的表示概率值。 -
如果在神经网络内部(隐藏层)需要使用激活函数,一般会使用ReLU函数或者ReLU函数的改进来进行激活。 -
如果是二分类问题,那么在神经网络最后一层加上Sigmoid函数层。 -
如果是多分类问题,那么会在神经网络最后一层加上Softmax函数层。 -
Softmax函数通常和交叉熵函数一起使用。
|