一、Conv1d 参数设定
torch.nn.Conv1d(in_channels, "输入图像中的通道数"
out_channels, "卷积产生的通道数"
kernel_size, "卷积核的大小"
stride, "卷积的步幅。默认值:1"
padding, "添加到输入两侧的填充。默认值:0"
dilation, "内核元素之间的间距。默认值:1"
groups, "从输入通道到输出通道的阻塞连接数。默认值:1"
bias, "If True,向输出添加可学习的偏差。默认:True"
padding_mode "'zeros', 'reflect', 'replicate' 或 'circular'. 默认:'zeros'"
)
in_channels ( int ) – 输入图像中的通道数 out_channels ( int ) – 卷积产生的通道数 kernel_size ( int or tuple ) – 卷积核的大小 stride ( int or tuple , optional ) – 卷积的步幅。默认值:1 padding ( int , tuple或str , optional ) – 添加到输入两侧的填充。默认值:0 dilation ( int or tuple , optional ) – 内核元素之间的间距。默认值:1 groups ( int , optional ) – 从输入通道到输出通道的阻塞连接数。默认值:1 bias ( bool , optional ) – If True,向输出添加可学习的偏差。默认:True padding_mode (字符串,可选) – ‘zeros’, ‘reflect’, ‘replicate’或’circular’. 默认:‘zeros’
二、Conv1d 输入输出以及卷积核维度
input –
(
minibatch
,
in_channels
,
i
W
)
(\text{minibatch} , \text{in\_channels} , iW)
(minibatch,in_channels,iW) (批大小, 数据的通道数, 数据长度) output –
(
minibatch
,
out_channels?
,
i
W
)
(\text{minibatch} , \text{out\_channels } , iW)
(minibatch,out_channels?,iW) (批大小, 产生的通道数, 卷积后长度)
卷积后的维度:(n - k + 2 * p ) / s + 1 k: 卷积核大小,p: 使用边界填充,s: 步长。
卷积核维度:
(
in_channels
,
k
e
r
n
e
l
_
s
i
z
e
,
out_channels?
)
(\text{in\_channels} , kernel\_size, \text{out\_channels })
(in_channels,kernel_size,out_channels?)
其中:out_channels维度,代表卷积核的个数,用来提取多维特征。
三、Conv1d 计算过程
1. 测试一:in_channels=1, out_channels=1
定义卷积如下: 输入通道:1, 输出通道:1,卷积核:1 * 3 * 1,步长:1,填充:0 输入: 批大小:1, 数据的通道数:1, 数据长度: 5
import torch
import torch.nn as nn
input = torch.randn(1, 1, 5)
conv = nn.Conv1d(in_channels=1, out_channels=1, kernel_size=3, stride=1, padding=0)
out = conv(input)
第一次卷积计算如下: 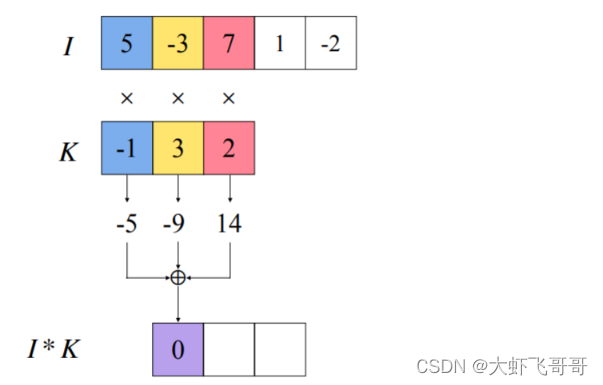 按照步长依次向后移动计算: 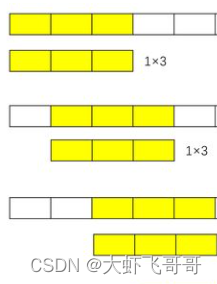
输出: 批大小:1, 数据的通道数:1, 数据长度: 3
运行结果: 
2. 测试二:in_channels=1, out_channels=2
定义卷积如下: 输入通道:1, 输出通道:2, 卷积核:1 3 2 (两个 13,提取两维特征 )*, 步长:1,填充:0 输入: 批大小:1, 数据的通道数:1, 数据长度: 5
import torch
import torch.nn as nn
input = torch.randn(1, 1, 5)
conv = nn.Conv1d(in_channels=1, out_channels=2, kernel_size=3, stride=1, padding=0)
out = conv(input)
输出: 批大小:1, 数据的通道数:2, 数据长度: 3
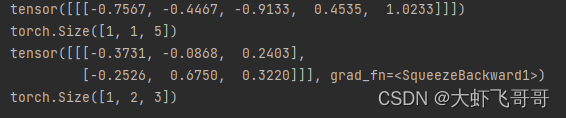
3. 测试三:in_channels=8, out_channels=1
定义卷积如下: 输入通道:8, 输出通道:1, 卷积核:8 * 3 * 1 , 步长:1,填充:0 输入: 批大小:1, 数据的通道数:1, 数据长度: 7
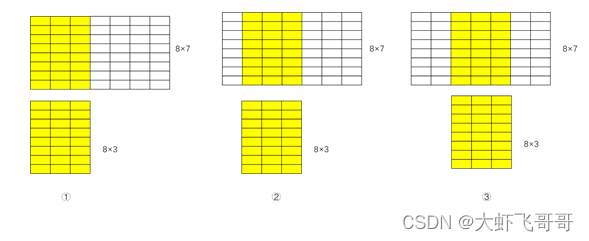
import torch
import torch.nn as nn
input = torch.randn(1, 8, 7)
conv = nn.Conv1d(in_channels=8, out_channels=1, kernel_size=3, stride=1, padding=0)
out = conv(input)
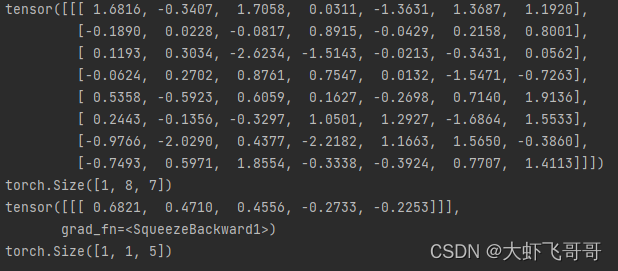
输出: 批大小:1, 数据的通道数:1, 数据长度: 5
3. 测试三:in_channels=8, out_channels=2
定义卷积如下: 输入通道:8, 输出通道:1, 卷积核:8 * 3 * 2 , 步长:1,填充:0 输入: 批大小:1, 数据的通道数:1, 数据长度: 7
import torch
import torch.nn as nn
input = torch.randn(1, 8, 7)
conv = nn.Conv1d(in_channels=8, out_channels=2, kernel_size=3, stride=1, padding=0)
out = conv(input)
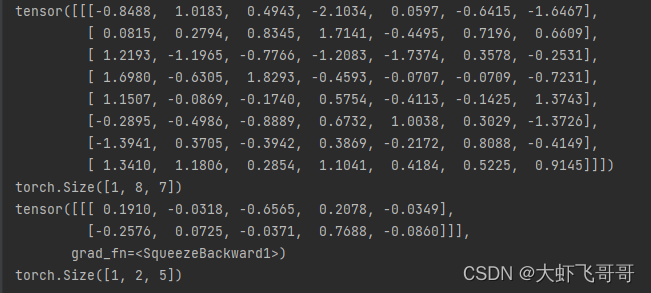 输出: 批大小:1, 数据的通道数:2, 数据长度: 5
四、Conv1d 在文本中的应用 – TextCNN
论文:Convolutional Neural Networks for Sentence Classification
模型框架如下图所示。 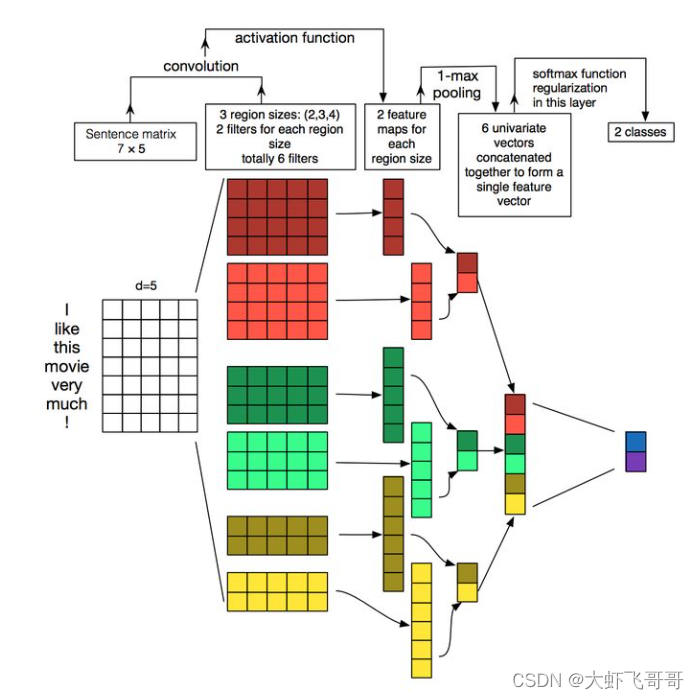
假设我们需要对句子进行分类。句子中每个词是由 n 维词向量组成的,也就是说输入矩阵大小为 m*n,其中m为句子长度。 在 pytorch 中,从左往右卷积,因此要将输入维度调换,即(n, m)— (词向量维度, 句子长度) 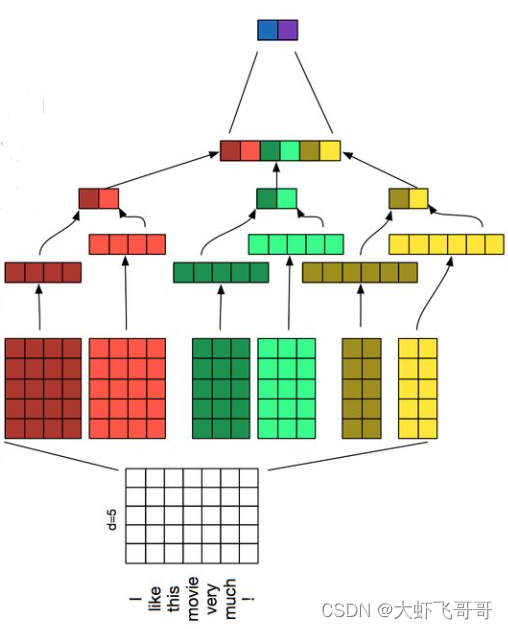
如上图所示:输入维度是:(5, 7):
import torch
import torch.nn as nn
input = torch.randn(1, 5, 7)
CNN需要对输入样本进行卷积操作,对于文本数据,有点类似于N-gram在提取词与词间的局部相关性。图中共有三种 kernel_size 的卷积,分别是2,3,4,每个 kernel_size 都有两个filter(实际训练时filter数量会很多)。在不同词窗上应用不同filter,最终得到6个卷积后的向量。
以 kernel_size = 4 为例:卷积核维度 (5, 4)
import torch
import torch.nn as nn
input = torch.randn(1, 5, 7)
conv = nn.Conv1d(in_channels=5, out_channels=1, kernel_size=4, stride=1, padding=0)
out = conv(input)
输出: 
然后对每一个向量进行最大化池化操作并拼接各个池化值,最终得到这个句子的特征表示,将这个句子向量丢给分类器进行分类,至此完成整个流程。
|