背景概述
原始数据的整理和清洁过程中,有时需要对符合某些条件要求的数据进行更新,如:对条件筛选出来的记录赋予新数值或字符串;按类别建立新的特征属性等,本文小结了修改或匹配数据记录方法。
方法与函数简介
- replace()函数:找到对应的数值或字符串直接赋予新值:df.replace(old, new)
- where()函数:替换where条件为False的值:df.where(cond, x, y)
- 使用索引值进行修改:df[“column_name”].iloc[index]
- 使用字典进行匹配修改:df[“column_name”].map(dict)
示例代码(Python代码)
1. replace()函数:直接查找并替换数值或字符串 – 如df.replace(old, new) DataFrame.replace(to_replace = None,value = None,inplace = False,limit = None,regex = False,method =‘pad’ ) 替换给定的值to_replace:str,regex,list,dict,Series,int,float或None 如果regex=True则to_replace里的所有字符串都将被解释为正则表达
import numpy as np
import pandas as pd
# 举例1: 对整个数据表中的某个数值或字符串直接修改
li = [[1, 100],
[100, 30],
[3, 100]]
df = pd.DataFrame(li, columns=["A", "B"])
display(df)
df = df.replace(100, "aa")
df
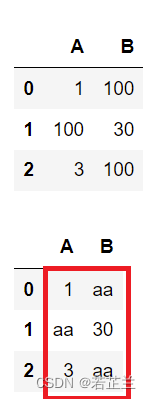 小结:表格中的所有为100的数字都被替换为"aa"字符串。
# 举例2:对表格中某个特征列的数据或字符串进行替换修改
df["B"] = df["B"].replace(“aa", 50)
df
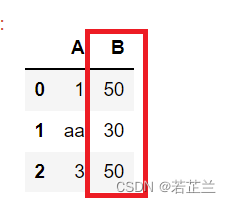 小结:表格中特征列B中的"aa"字符串都被修改为数值100,但是特征列A中的"aa"字符串则没有被修改
2. where()函数:替换where条件为False的值 – 如df.where(cond, other) 或 df.where(cond, x, y)
where(cond, other=nan, inplace=False, axis=None, level=None, errors=‘raise’, try_cast=False) 替换where条件为False的值,cond:bool Series/DataFrame, array-like,或 callable 当cond为True时,保持原始值。 当为False时,用other的相应值替换。 如果cond是可调用的,它将根据Series/DataFrame计算,并且应该返回boolean Series/DataFrame或array。 可调用对象不能更改输入Series/DataFrame(尽管panda不检查它)。 other:scalar, Series/DataFrame, 或 callable;cond为假的条目被替换为other的相应值。
# 举例3:where()函数在Series中的应用
# 将小于2的数据替换为100(即设定other=100,通常other默认为NaN)
s = pd.Series(range(1, 6))
s.where(s > 2, 100)
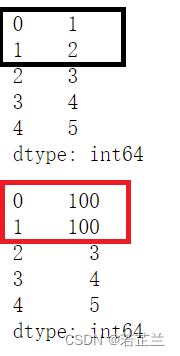 小结:s中的1,2由于不符合条件(>2),被替换修改为100(other=100)
# 举例4:where()函数在DataFrame中的使用 -- np.where(cond, x, y)
# 如果特征列A的数据小于或等于5,特征列C则等于特征列B的数值
# 如果特征列A的数据大于5,特征列C则等于特征列B的数值加上特征列A的数值
df = pd.DataFrame(np.arange(1, 11).reshape(-1, 2), columns=["A", "B"])
display(df)
df["C"] = np.where(df["A"]<=5, df["B"], (df["B"] + df["A"]))
df
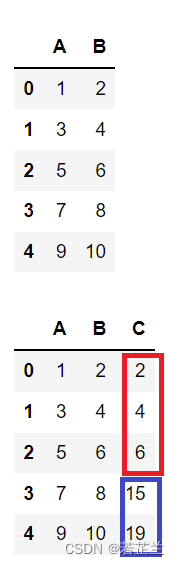 小结:表格中的特征列A的数据大于或等于5的时候,对应特征列C的数据等同于特征列B的数据;当特征列A的数据大于5时,对应特征列C的数据则为:特征列B的数据与特征列A的数据相加的值。
3. 根据条件筛选出来的数据记录的索引值进行替换修改:df[“column_name”].iloc[index]
# 举例5:通过数据的索引值进行替换修改
# 当特征列A的数据为5或9时,则特征列C等于特征列B的数值加上特征列A的数值
# 当特征列A的数据不是5或9时,特征列C将等于特征列B的数值
df = pd.DataFrame(np.arange(1, 11).reshape(-1, 2), columns=["A", "B"])
print(df)
df["C"] = df["B"]
# 根据条件对特征列A进行筛选,并获取对应的数据记录的索引值
index = df[df["A"].isin([5, 9])].index
# 根据索引值进行数据的替换或修改
df["C"].iloc[index] = df["A"].iloc[index] + df["B"].iloc[index]
df
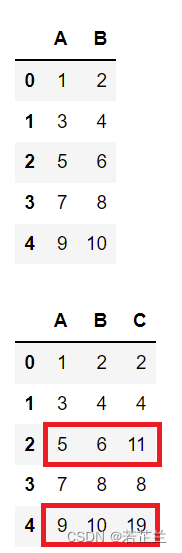
4. 使用字典进行匹配修改:df[“column_name”].map(dict)
# 举例6:使用表格df2的数据对表格df的数据进行匹配而建立新的特征属性
# 建立数据表格df 和df2
li1 = ["aa", "bb", "cc", "aa", "cc"]
li2 = np.arange(1, 6)
df = pd.DataFrame([li1, li2], index=["A", "B"]).T
display(df)
df2 = pd.DataFrame([["aa", "1st"], ["bb", "2nd"], ["cc", "3rd"]], columns=["S", "C"])
df2
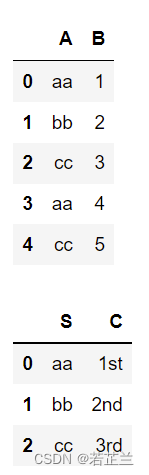
# 将表格df2的数据拆分并建立为字典
dict_ = dict(zip(df2.S, df2.C))
display(dict_)
# 使用map()函数对表格df进行匹配并对df建立新的特征列
df["C"] = df["A"].map(dict_)
df
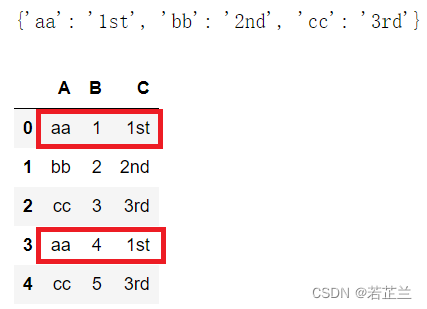
小结: 对于这种2个表格的匹配修改,也可以使用merge(), concat()函数进行表格的合并处理,同样能达到这种效果。
|