论文:https://arxiv.org/pdf/2205.12740.pdf 代码实现(非官方):https://github.com/xialuxi/yolov5-car-plate/commit/aa41d1819b1fb03b4dc73e8a3e0000c46cfc370b 图片源自视频教程(这个大佬视频教程yyds):https://www.bilibili.com/video/BV1yi4y1g7ro?p=4
原理:
从最早的IoU到GIoU,再到DIoU和CIoU,现在出现了SIoU
L2损失与 IoU损失的比较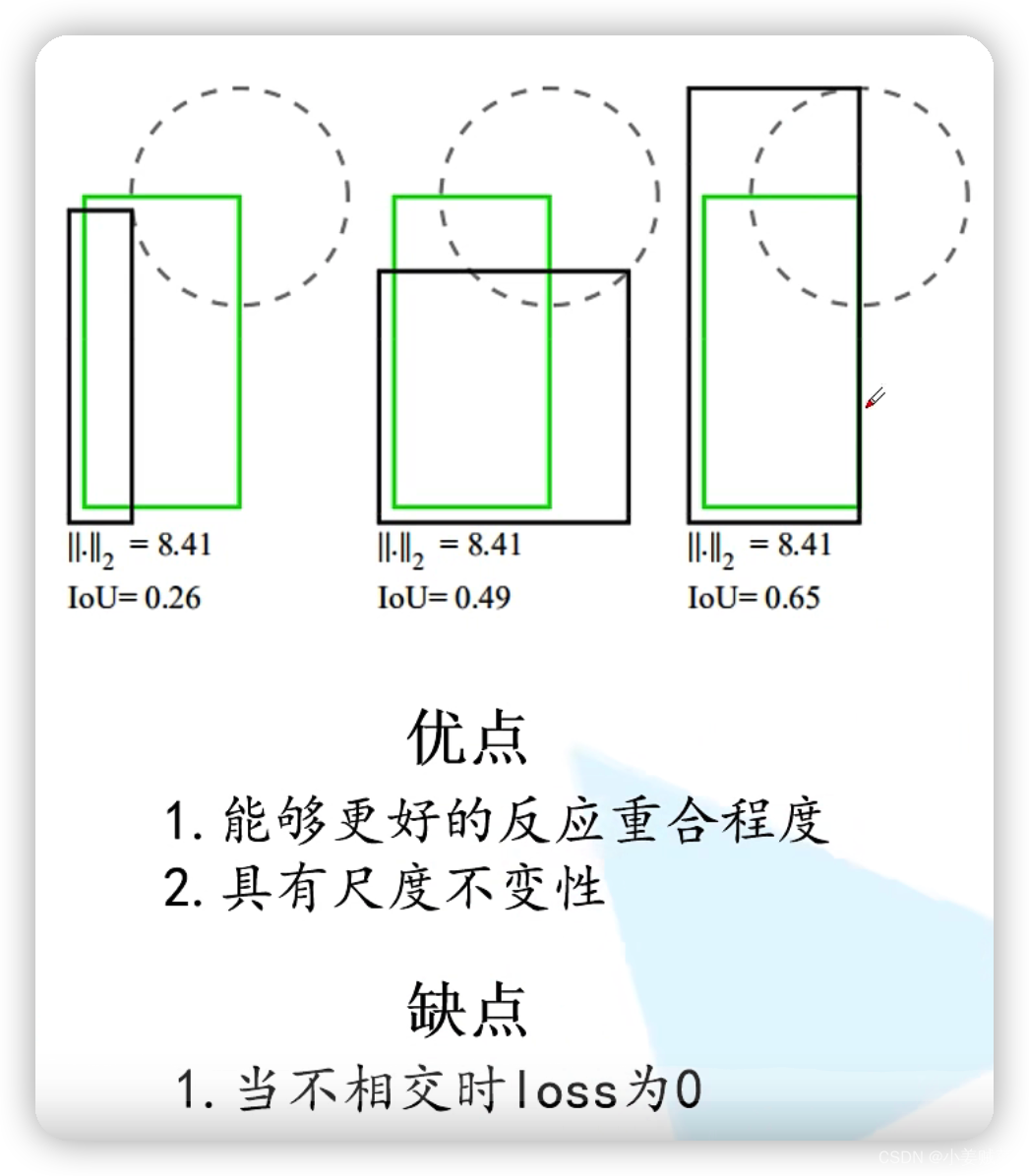
GIoU损失
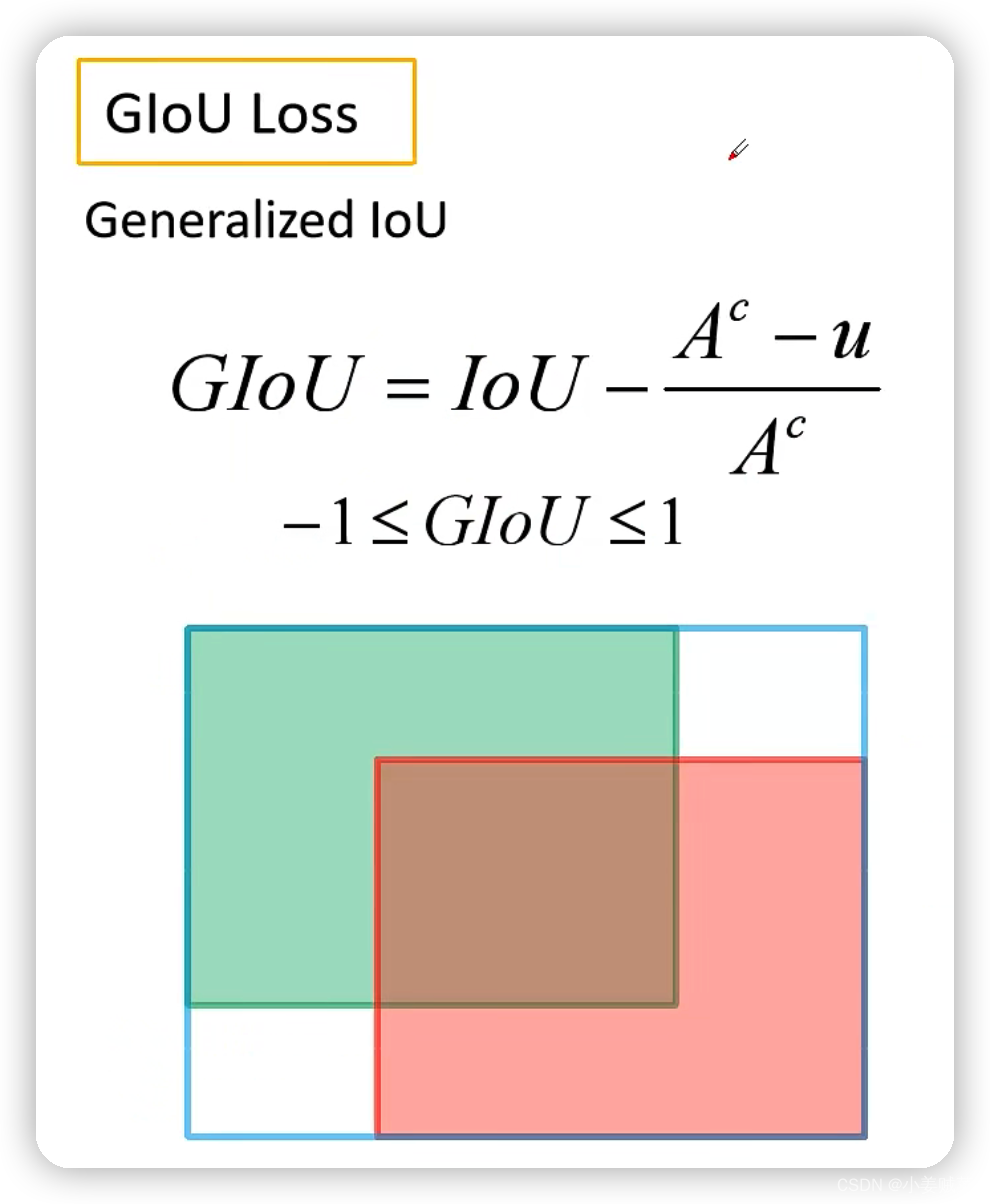 A代表蓝色的框,最大的矩形框。u代表GT和预测框的并集。
DIoU损失
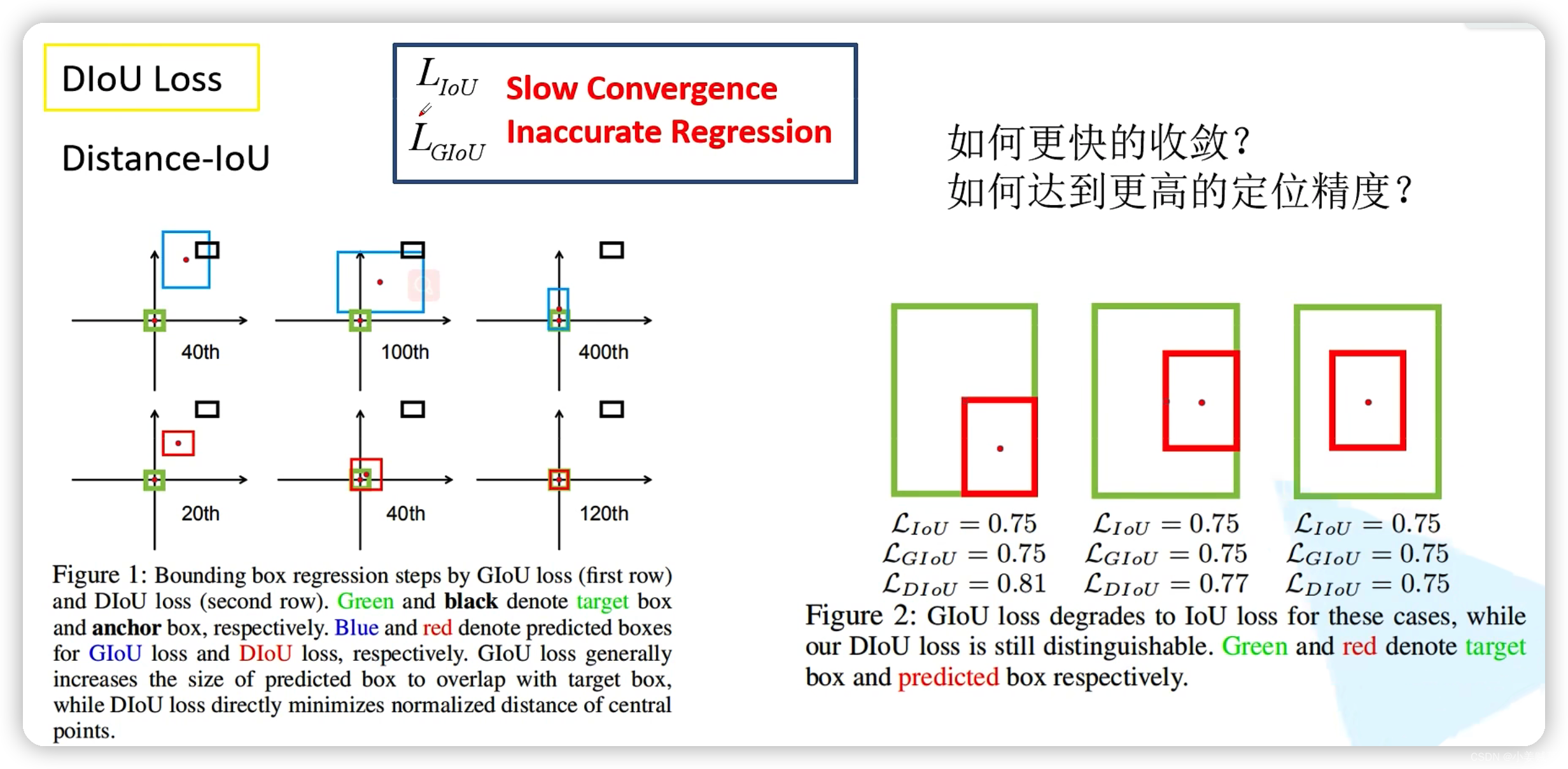 图片一左侧的上面是GIoU,下面的是DIoU:其中黑色的代表anchor,蓝色的代表预测框,绿色的为GT框
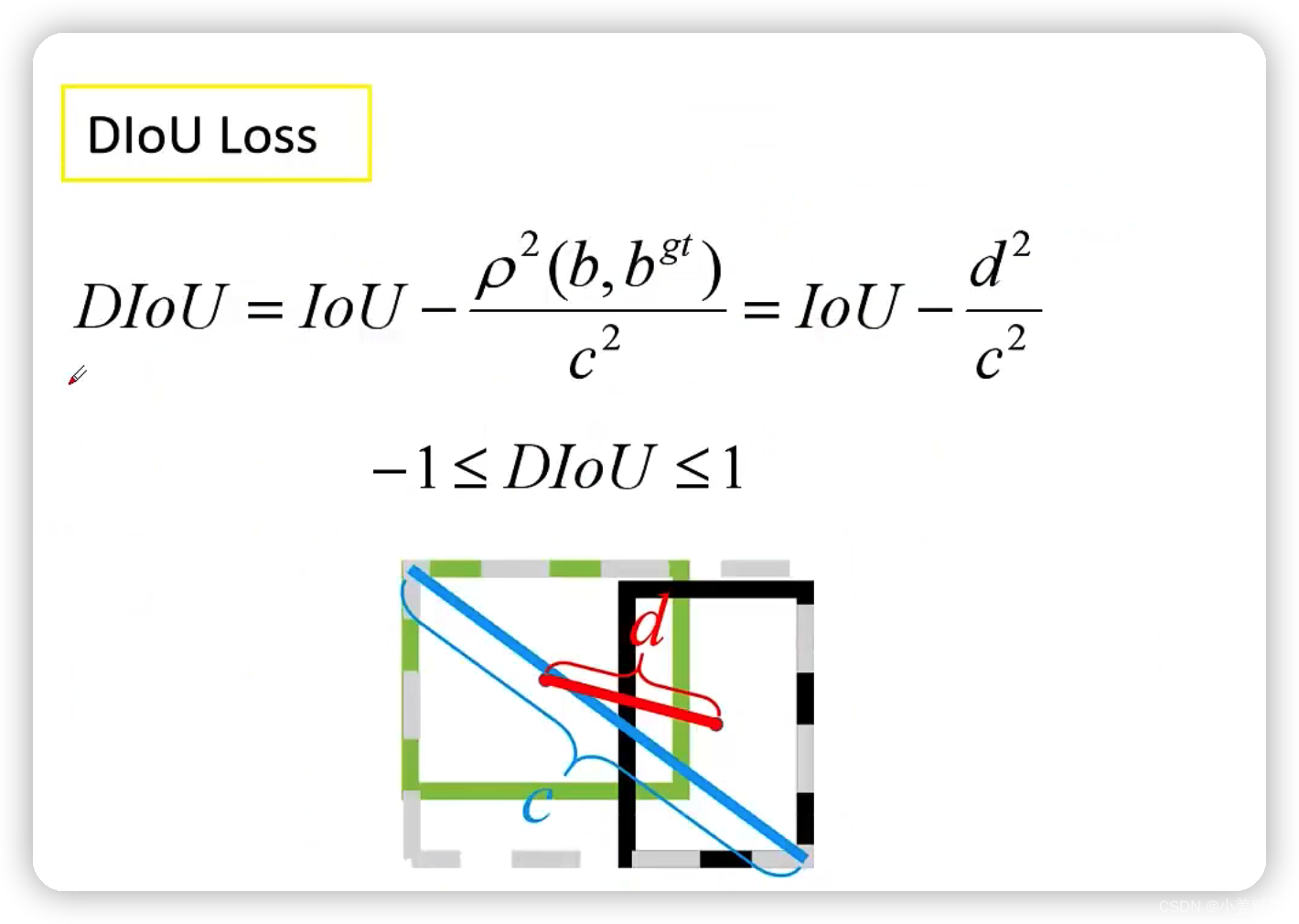
CIoU损失
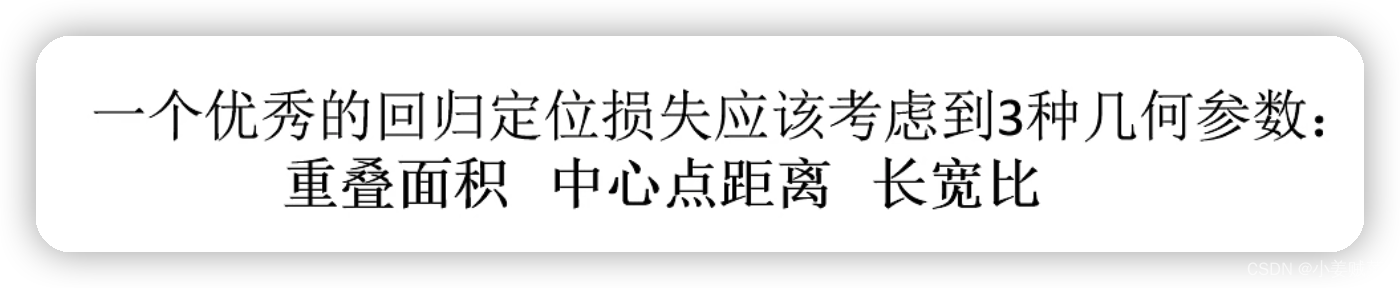 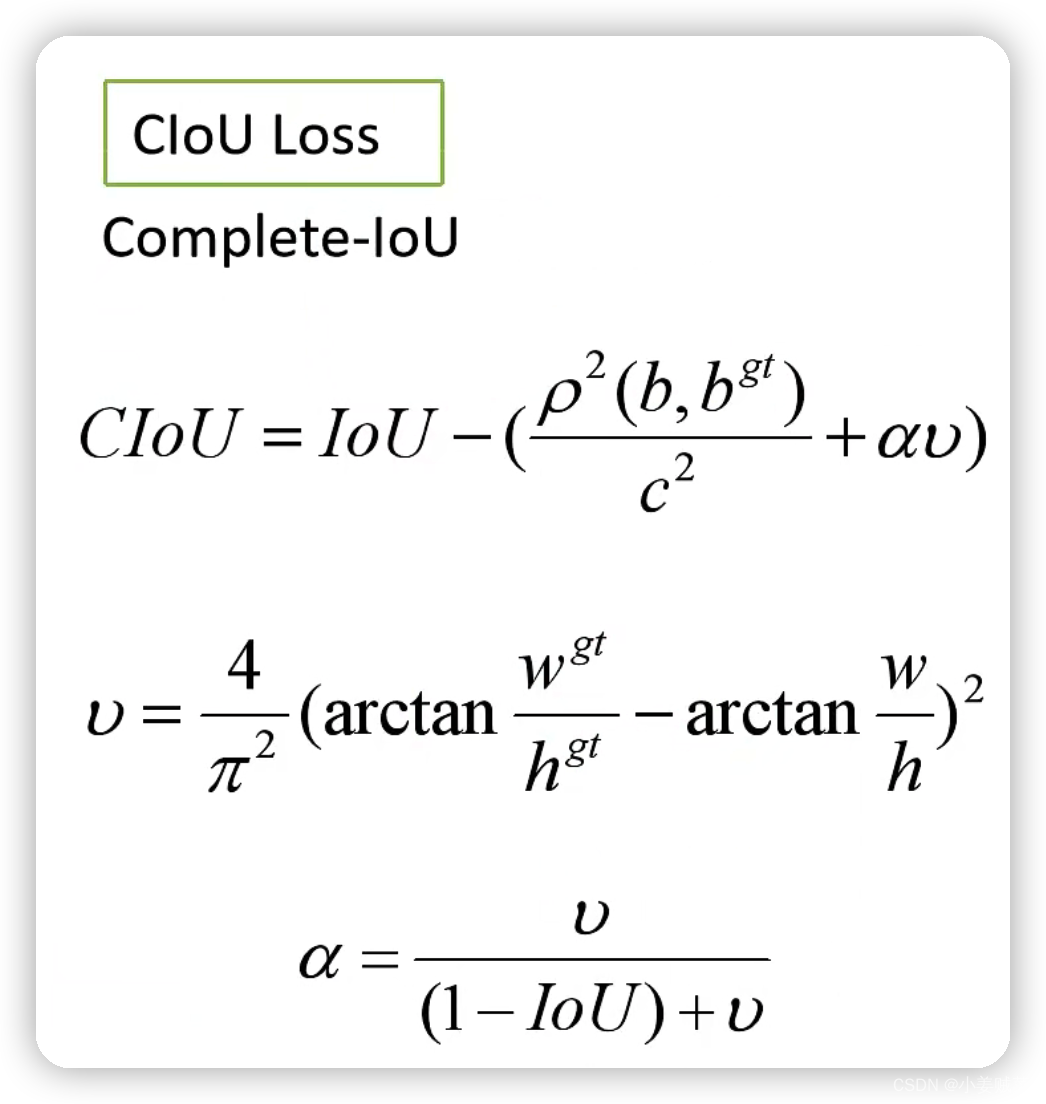
SIoU损失
再上面的基础上考虑了角度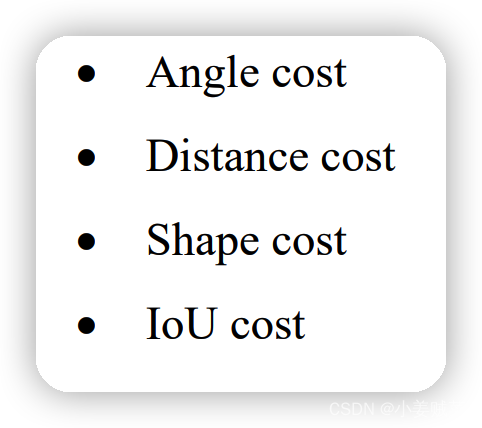 在论文中也重新定义了距离 cost和shape cost, 角度cost 定义如下: 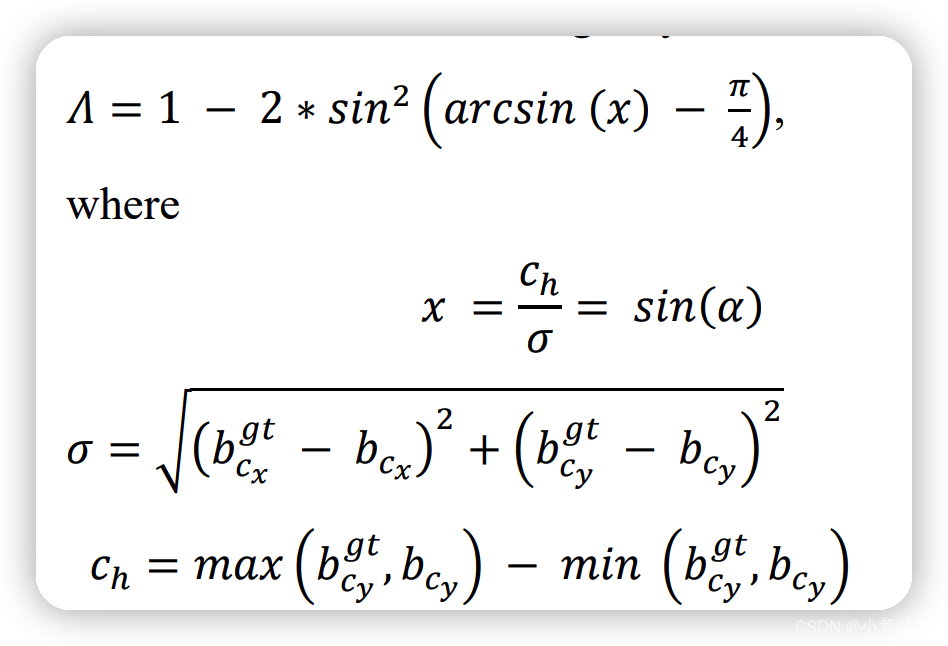 这里我看的很奇怪的一点就是,这个α为啥带入到sin,又带入到反sin,这不是多此一举吗?σ就是两个框的中心距离呗。
距离cost 定义如下: 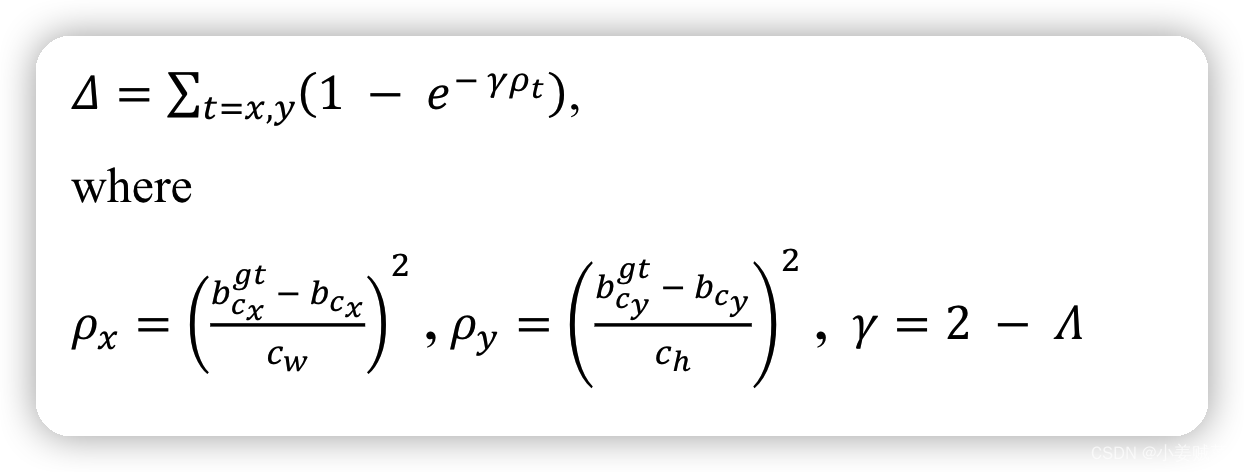 shape cost定义如下: 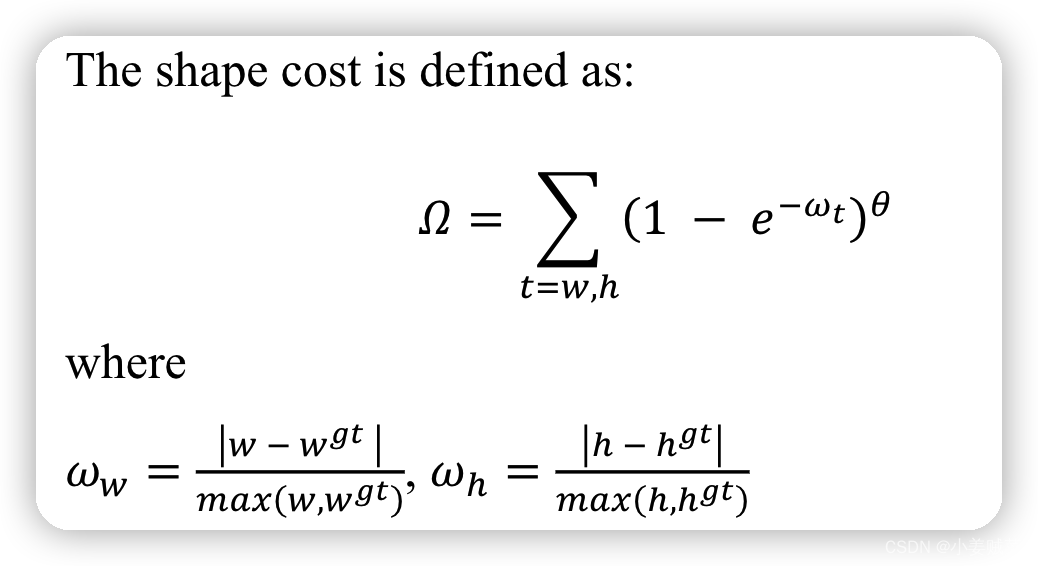 整的lost 定义: 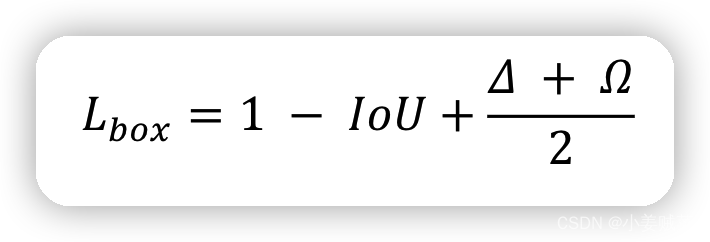 还有很多细节没有分析、挖掘、探讨,这里只是草草的分享下,记录下。
代码实现:
!!!重要的事情说三遍,不是我实现的,不是我实现的,不是我实现的。来自于开头链接的大佬:
if SIoU: # SIoU Loss https://arxiv.org/pdf/2205.12740.pdf
sigma = torch.pow(cw ** 2 + ch ** 2, 0.5)
sin_alpha_1 = ch / sigma
sin_alpha_2 = cw / sigma
threshold = pow(2, 0.5) / 2
sin_alpha = torch.where(sin_alpha_1 > threshold, sin_alpha_2, sin_alpha_1)
# angle_cost = 1 - 2 * torch.pow( torch.sin(torch.arcsin(sin_alpha) - np.pi/4), 2)
angle_cost = torch.cos(torch.arcsin(sin_alpha) * 2 - np.pi / 2)
rho_x = ((b2_x1 + b2_x2 - b1_x1 - b1_x2) / cw) ** 2
rho_y = ((b2_y1 + b2_y2 - b1_y1 - b1_y2) / ch) ** 2
gamma = 2 - angle_cost
distance_cost = 2 - torch.exp(-1 * gamma * rho_x) - torch.exp(-1 * gamma * rho_y)
omiga_w = torch.abs(w1 - w2) / torch.max(w1, w2)
omiga_h = torch.abs(h1 - h2) / torch.max(h1, h2)
shape_cost = torch.pow(1 - torch.exp(-1 * omiga_w), 4) + torch.pow(1 - torch.exp(-1 * omiga_h), 4)
return iou - 0.5 * (distance_cost + shape_cost)
|