矩阵 / 图像 坐标旋转
- 定义旋转矩阵,对2D的Tensor操作时,shape应当为
[B,2,3]
import math
from torch.nn import functional as F
B = 1
angle = 45/180*math.pi
transform_matrix = torch.tensor([
[math.cos(angle),math.sin(-angle),0],
[math.sin(angle),math.cos(angle),0]])
transform_matrix = transform_matrix.unsqueeze(0).repeat(B,1,1)
- 输入图像src,shape为
[H,W] ,需要将其转换成Tensor后的shape为[B,C,H,W] :(这里做旋转时有一个非常重要的大坑细节:旋转时务必先将tensor转换为正方形,即H=W,否则非正方形旋转会导致较长边出现拉伸情况。)
- 如果输入src的
H,W 不相等,首先需要做padding 将其补全为正方形,同时要保证旋转中心点不变,再进行操作,最后将output的tensor中padding 部分去除即可,padding 操作如下所示。
src = torch.tensor(src, dtype=torch.float32).unsqueeze(0).unsqueeze(0)
如果需要padding :(假设src的shape为[1,1,400,200] )
B,C,H,W = src.shape
pad_list = torch.split(tensor=(torch.zeros_like(src, device=src.device, dtype=src.dtype)),
split_size_or_sections=[int(W/2),int(W/2)],
dim=-1)
src= torch.cat([pad_list[0], src, pad_list[1]], dim=-1)
src.shape
- 基于torch函数
affine_grid 和grid_sample 实现仿射变换:
grid = F.affine_grid(transform_matrix,
src.shape)
output = F.grid_sample(src,
grid,
mode='nearest')
如果上一步你进行了padding操作,那么需要取出原src部分:
output = torch.split(output,
split_size_or_sections=[int(W/2), int(W),int(W/2)],
dim=-1)[1]
output.shape
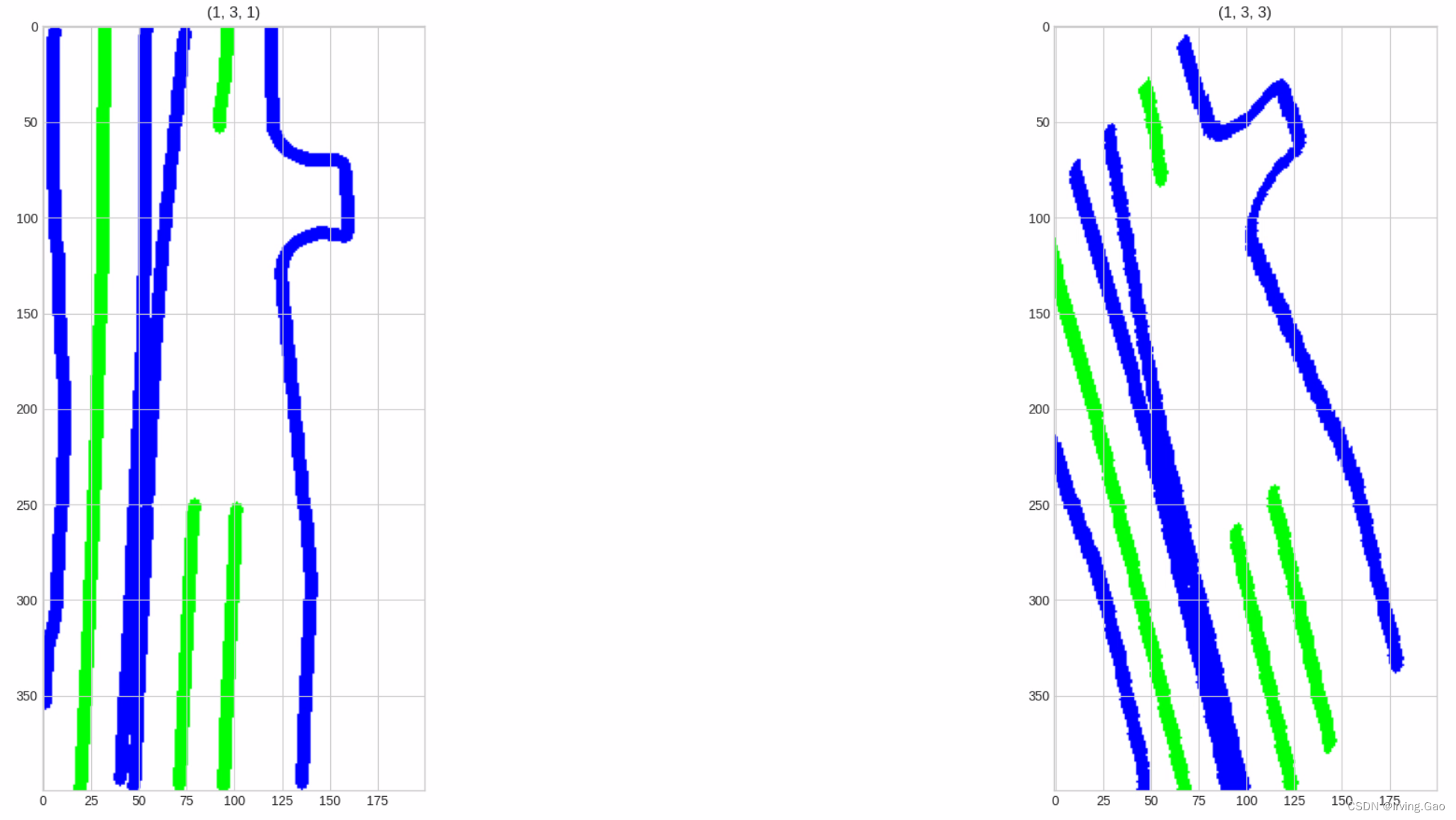
矩阵 / 图像 坐标平移
- 这里做平移时有一个非常重要的大坑细节:平移的x和y都是经过归一化的,即在[0-1]之间,务必不要以为是平移的像素个坐标。
shift_x = 0.5
shift_y = 0.5
transform_matrix = torch.tensor([
[1, 0, shift_x],
[0, 1 ,shift_y]]).unsqueeze(0)
其他步骤与旋转相同:
grid = F.affine_grid(transform_matrix,
src.shape)
output = F.grid_sample(src,
grid,
mode='nearest')
- 平移效果(左侧为原图,右侧为平移后图像):
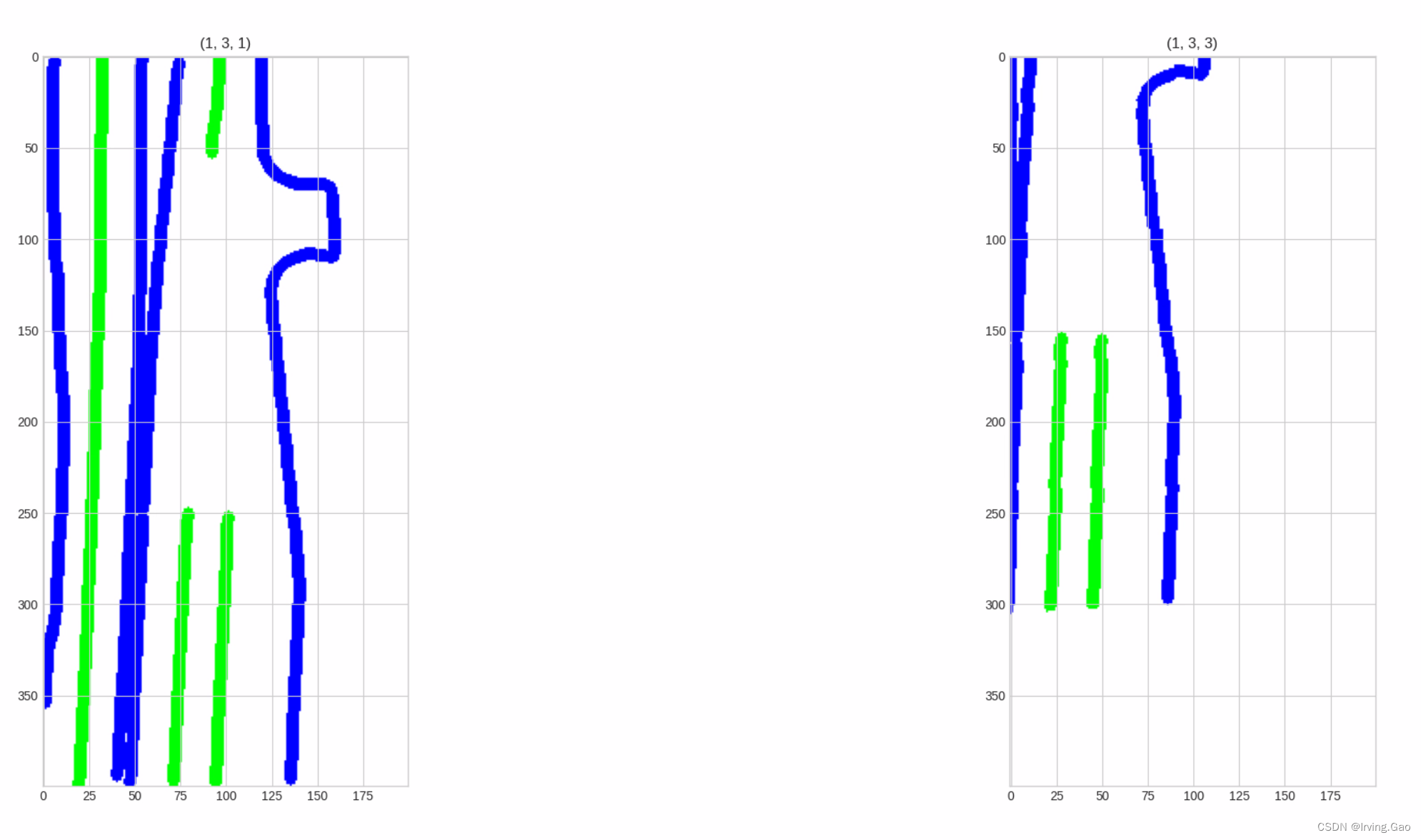
矩阵 / 图像 坐标平移+旋转
import math
from torch.nn import functional as F
B = 1
angle = 45/180*math.pi
shift_x = 0.5
shift_y = 0.5
transform_matrix = torch.tensor([
[math.cos(angle),math.sin(-angle),shift_x],
[math.sin(angle),math.cos(angle),shift_y]])
transform_matrix = transform_matrix.unsqueeze(0).repeat(B,1,1)
grid = F.affine_grid(transform_matrix,
src.shape)
output = F.grid_sample(src,
grid,
mode='nearest')
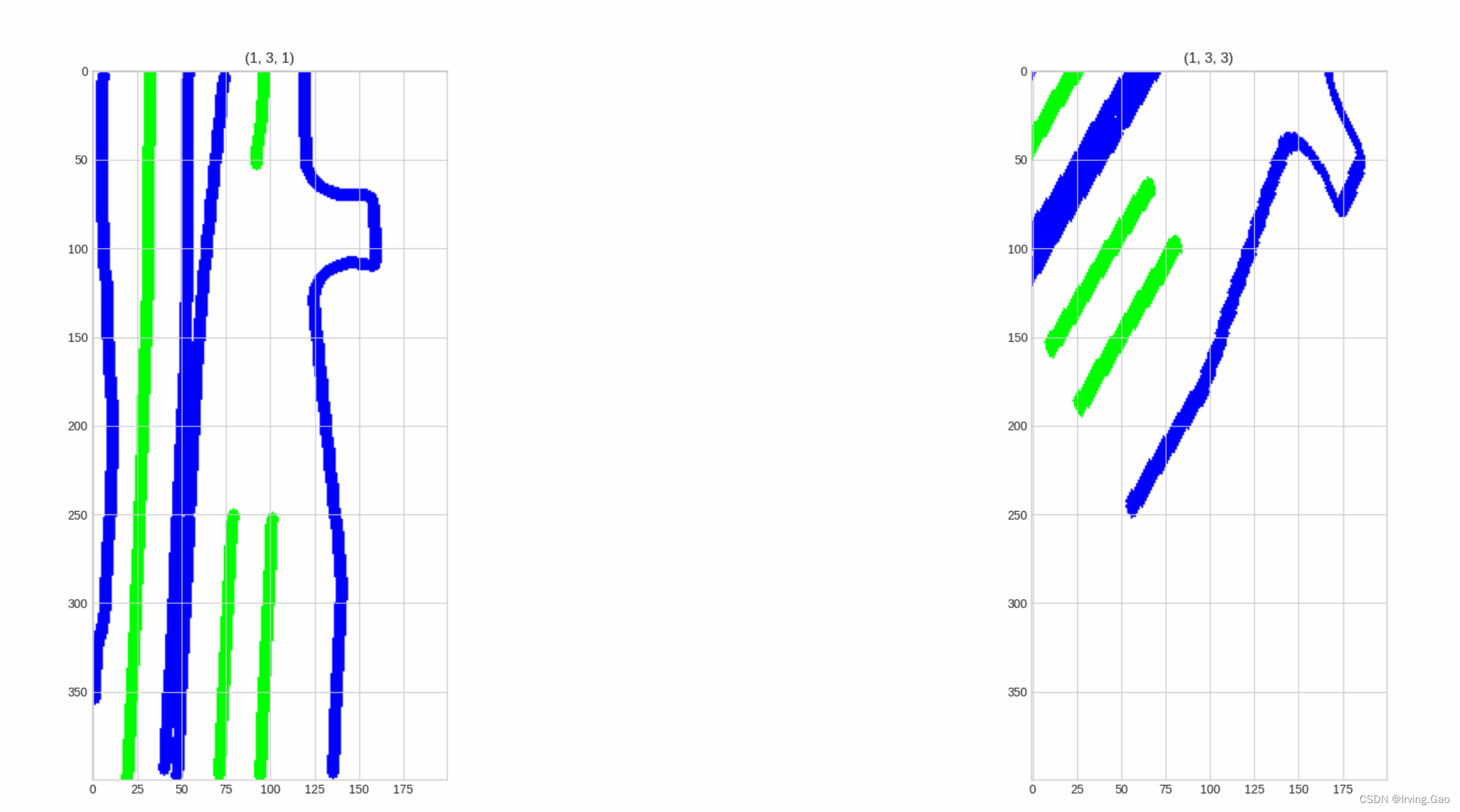
参考文章:
|