导论
OpenMV内置了好几个有关神经网络的模型,我们可以利用它们来进行基本物体的识别以及笑脸检测,数字识别等,这一节主要讲解下利用cifar_10进行识别
cifar_10是一个用于普适物体识别的数据集,"10"的意思是它可以分辨十种不同的物体,比如飞机、船、汽车、鸟、猫、狗、青蛙、路、卡车等等…
cifar_10由6万张32*32的RGB彩图构成,共有10个分类,一共有5万张的训练以及1万张的测试用于交叉验证
这个数据集最大的特点在于将数据集迁移到了普适的物体上,而且应用于多分类,他的一个子类数据集cifar_100可以达到100类,同已经成熟的人脸识别相比,普适物体的识别挑战很大,数据中含有大量的噪声、特征以及识别的物体大小不一、角度不一、比例不一等,
因此cifar_10相对于传统的图像识别数据集来说,它的挑战是巨大的,但其应用的效果是非常不错的
在OpenMV的IDE中内置了已经训练好的适用于OpenMV上面的模型网络,我们可以直接在OpenMV IDE工具——机器视觉——CNN网络库 里面打开
在我们的OpenMV上推荐用cifar10_fast.network 这一个神经网络,这个神经网络的模型稍微小一点,相对于cifar10.network来说,cifar10_fast.network 运算速度更快,并且耗费的内存更小,如果我们直接使用cifar10.network ,在我们的OpenMV上很可能会超出内存
利用神经网络进行特征识别(已停用)
在这个例程中我们首先获取OpenMV摄像头中的图像,然后对我们的图像进行特征识别,再将其与我们的神经网络模型进行对比,来得到一个相似度,通过这个相似度来判断是否其属于数据集中的某个物体,进而达到物体识别的应用
运行此例程前,请先在OpenMV IDE->工具->机器视觉->CNN网络库 中,将相应的神经网络文件保存到OpenMV的SD内存卡中哦。
注意!在对OpenMV进行文件操作后(如保存神经网络文件到OpenMV的U盘中),需要重置OpenMV!OpenMV IDE->工具->重置OpenMV Cam
当前nn模块被删除了!所以没用…当了解吧🥀
import sensor, image, time, os, nn
sensor.reset()
sensor.set_pixformat(sensor.RGB565)
sensor.set_framesize(sensor.QVGA)
sensor.set_windowing((128, 128))
sensor.skip_frames(time=750)
sensor.set_auto_gain(False)
sensor.set_auto_exposure(False)
net = nn.load('/cifar10_fast.network')
labels = ['airplane', 'automobile', 'bird', 'cat', 'deer', 'dog', 'frog', 'horse', 'ship', 'truck']
clock = time.clock()
while(True):
clock.tick()
img = sensor.snapshot()
for obj in net.search(img, threshold=0.6, min_scale=0.4, scale_mul=0.8, \
x_overlap=-1, y_overlap=-1, contrast_threshold=0.5):
print("Detected %s - Confidence %f%%" % (labels[obj.index()], obj.value()))
img.draw_rectangle(obj.rect(), color=(255, 0, 0))
print(clock.fps())
对于net.search() 方法 以“滑动窗口”方式在图像roi上运行神经网络进行匹配 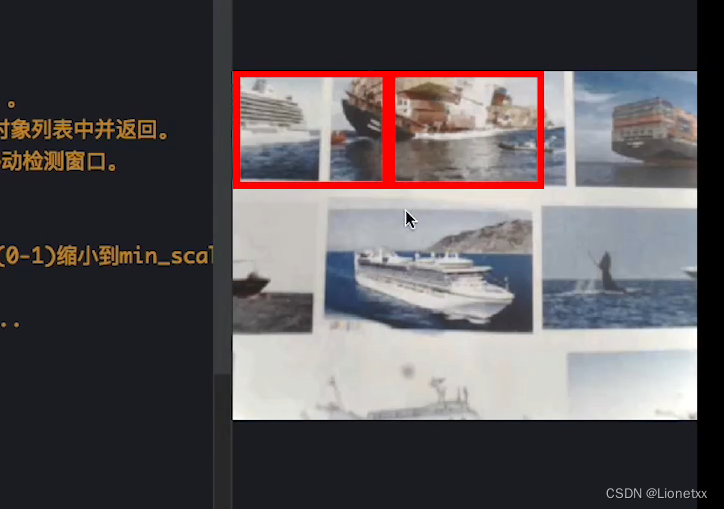
“x_overlap与y_overlap”–>如果将overlap设置为0.5,则每个检测窗口将与前一个检测窗口重叠50%。
x_overlap = 0.5,y_overlap = 0.5
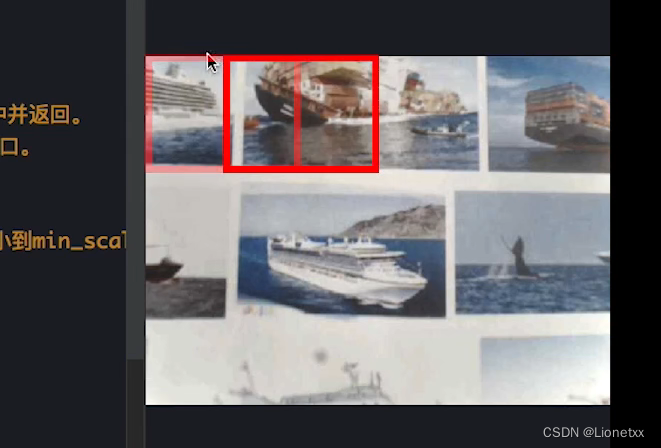
设置x_overlap = -1会强制窗口始终保持在x方向的ROI中心。 如果y_overlap不为-1,则该方法将搜索所有垂直位置。 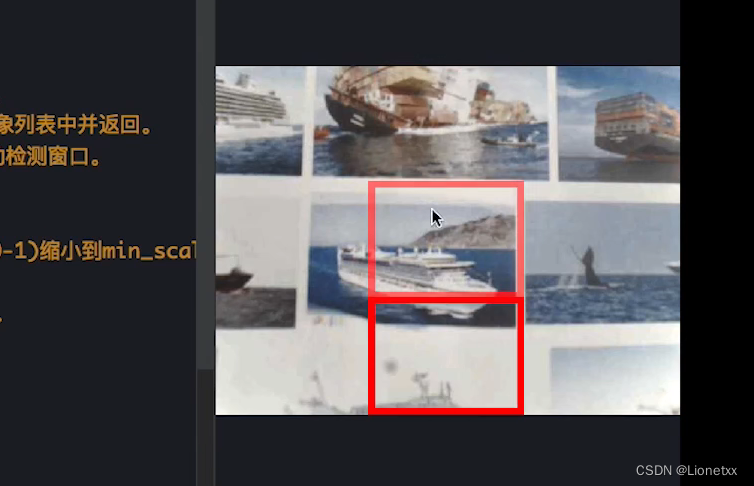
神经网络检测函数
模块nn 已经被OpenMV停用了,换成了tf 所用到的函数
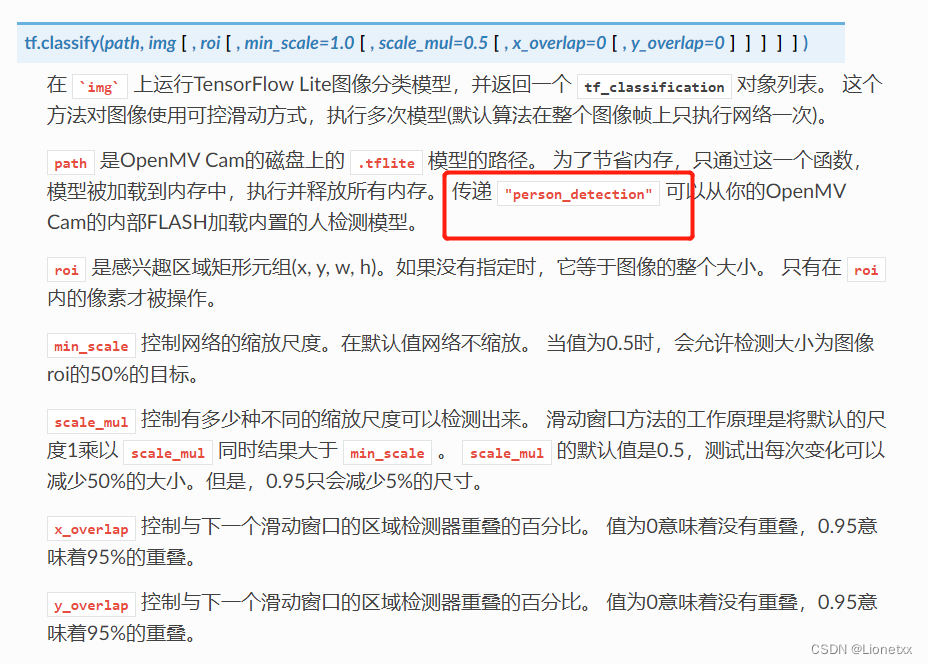
tf.segment(path, img[, roi])
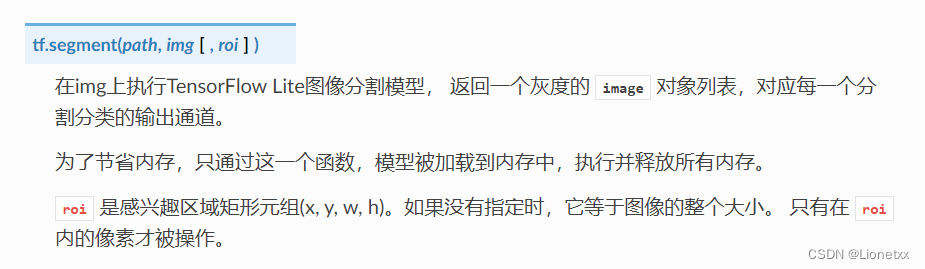
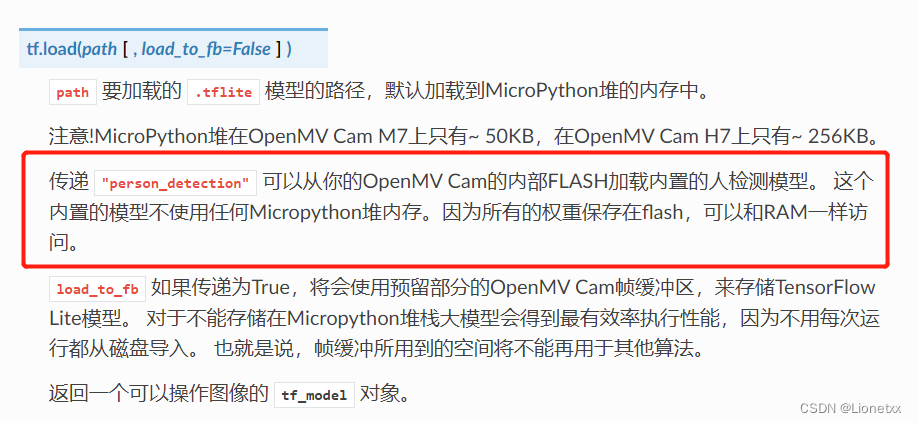
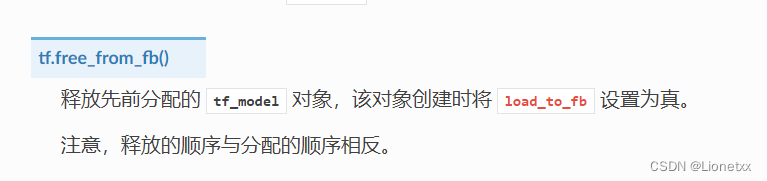
检测函数tf.classify() 返回值
如 for obj in tf.classify(mobilenet, img, min_scale=1.0, scale_mul=0.5, x_overlap=0.0, y_overlap=0.0): 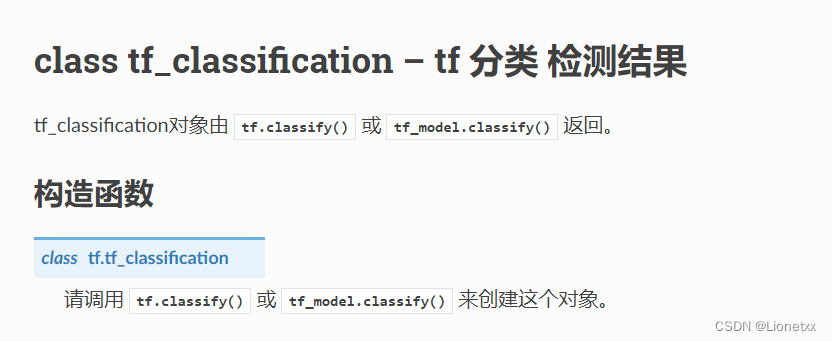 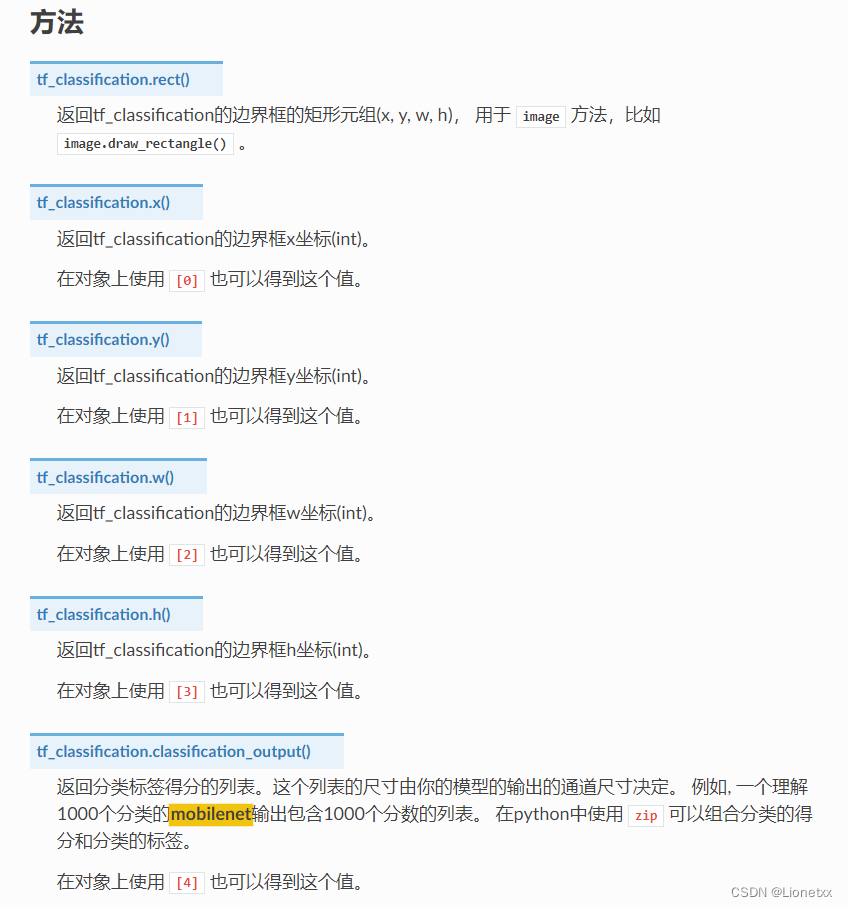
加载神经网络函数tf.load()返回值
如net = tf.load('person_detection') 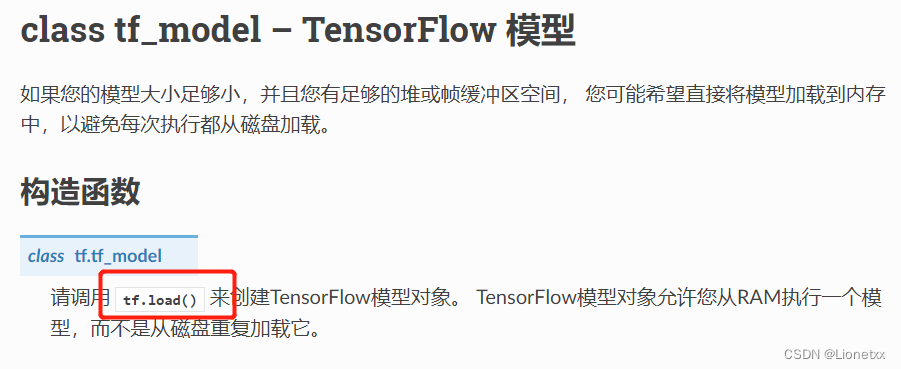 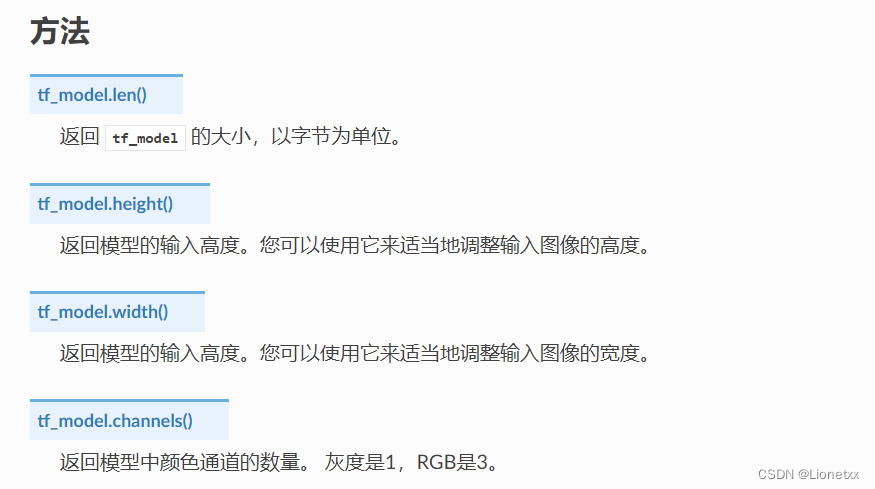
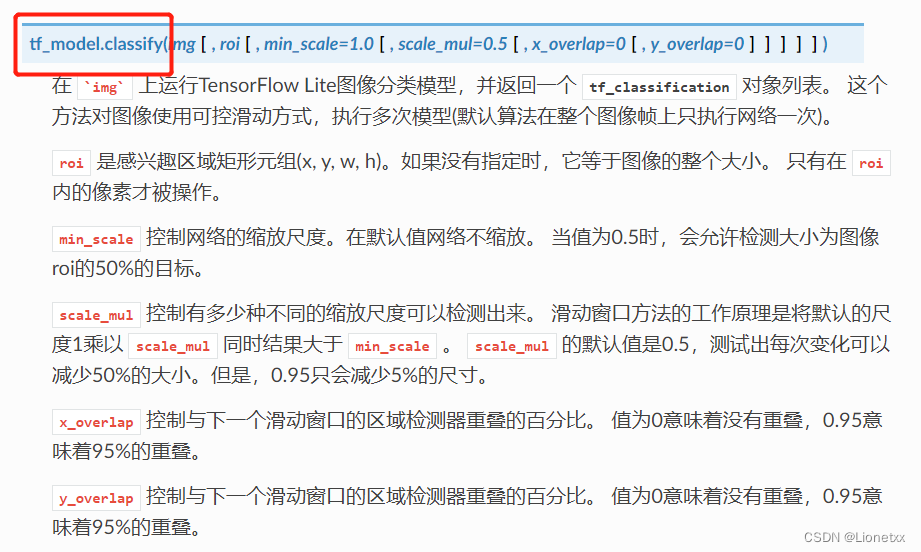
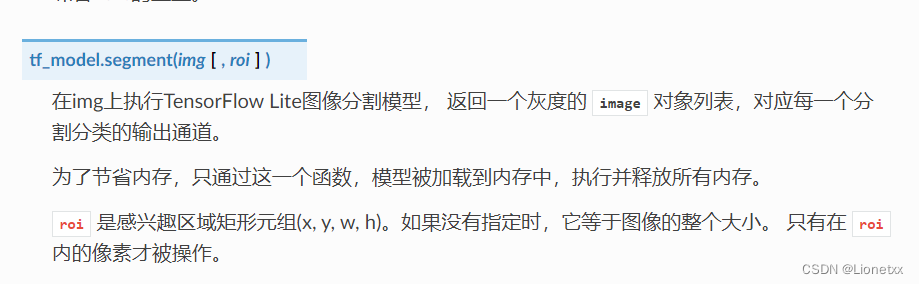
例程1.图像中央人检测
利用了内置的人检测神经网络模型person_detection (该网络位于OpenMV Cam的固件中)
例程tf_person_detection_search_just_center TensorFlow图像中央人检测
import sensor, image, time, os, tf
sensor.reset()
sensor.set_pixformat(sensor.GRAYSCALE)
sensor.set_framesize(sensor.QVGA)
sensor.set_windowing((240, 240))
sensor.skip_frames(time=2000)
net = tf.load('person_detection')
labels = ['unsure', 'person', 'no_person']
clock = time.clock()
while(True):
clock.tick()
img = sensor.snapshot()
for obj in net.classify(img, min_scale=0.5, scale_mul=0.5, x_overlap=-1, y_overlap=-1):
print("**********\nDetections at [x=%d,y=%d,w=%d,h=%d]" % obj.rect())
for i in range(len(obj.output())):
print("%s = %f" % (labels[i], obj.output()[i]))
img.draw_rectangle(obj.rect())
img.draw_string(obj.x()+3, obj.y()-1, labels[obj.output().index(max(obj.output()))], mono_space = False)
print(clock.fps(), "fps")
例程2.整幅图像人脸检测
利用了内置的人检测神经网络模型person_detection (该网络位于OpenMV Cam的固件中)
例程tf_person_detection_search_whole_window TensorFlow整幅图像人检测
import sensor, image, time, os, tf
sensor.reset()
sensor.set_pixformat(sensor.GRAYSCALE)
sensor.set_framesize(sensor.QVGA)
sensor.set_windowing((240, 240))
sensor.skip_frames(time=2000)
net = tf.load('person_detection')
labels = ['unsure', 'person', 'no_person']
clock = time.clock()
while(True):
clock.tick()
img = sensor.snapshot()
for obj in net.classify(img, min_scale=1.0, scale_mul=0.5, x_overlap=0.0, y_overlap=0.0):
print("**********\nDetections at [x=%d,y=%d,w=%d,h=%d]" % obj.rect())
for i in range(len(obj.output())):
print("%s = %f" % (labels[i], obj.output()[i]))
img.draw_rectangle(obj.rect())
img.draw_string(obj.x()+3, obj.y()-1, labels[obj.output().index(max(obj.output()))], mono_space = False)
print(clock.fps(), "fps")
|