一、环境
opencv4.5.1
Ubuntu 21.10
二、onnx转换
切换到yolov5工程中,打开终端运行指令
python export.py --weights yolov5s.pt --include onnx
onnx转onnx-sim
python -m onnxsim yolov5s.onnx yolov5s_sim.onnx
使用netron打开onnx文件
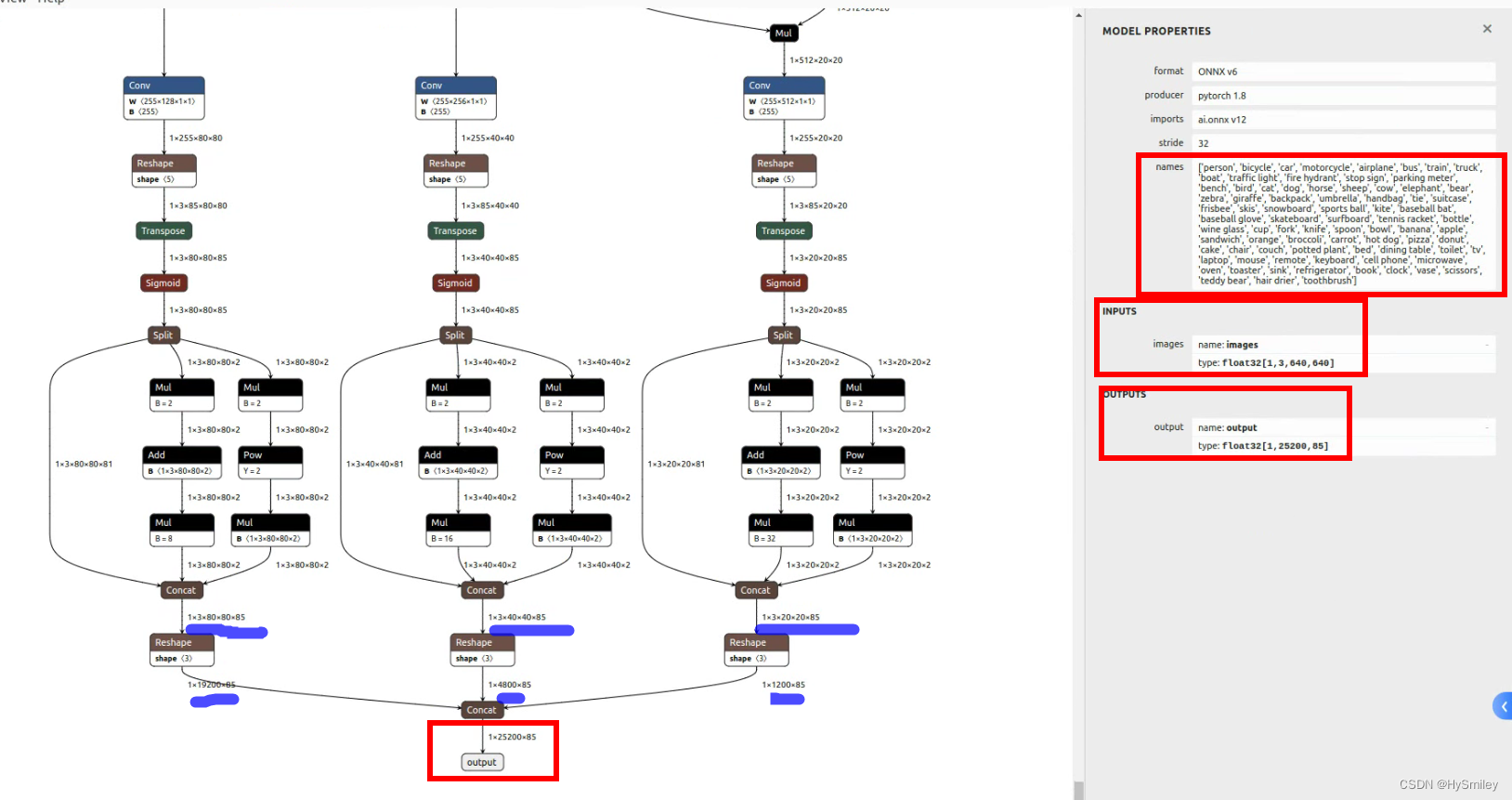
?最终的输出1*25200*85,将三个输出整合在一起即:
?25200=3*(20*20)+3*(40*40)+3*(80*80)个候选框
三、python版本推理
opecv DNN API: 1.读取模型 net = cv2.dnn.readNetFromONNX(model) 2.输入(类似Tensor) blob = cv2.dnn.blobFromImage(image, 1 / 255.0, (640, 640), swapRB=True, crop=False) ????????swapRB:BGR->RGB net.setInput(blob) 3.推理 preds = net.forward() 4.非极大值抑制 cv2.dnn.NMSBoxes(boxes,confidences,0.25,0.45)
import cv2
import numpy as np
classes=["person", "bicycle", "car", "motorcycle", "airplane", "bus", "train", "truck", "boat", "traffic light",
"fire hydrant", "stop sign", "parking meter", "bench", "bird", "cat", "dog", "horse", "sheep", "cow",
"elephant", "bear", "zebra", "giraffe", "backpack", "umbrella", "handbag", "tie", "suitcase", "frisbee",
"skis", "snowboard", "sports ball", "kite", "baseball bat", "baseball glove", "skateboard", "surfboard",
"tennis racket", "bottle", "wine glass", "cup", "fork", "knife", "spoon", "bowl", "banana", "apple",
"sandwich", "orange", "broccoli", "carrot", "hot dog", "pizza", "donut", "cake", "chair", "couch",
"potted plant", "bed", "dining table", "toilet", "tv", "laptop", "mouse", "remote", "keyboard", "cell phone",
"microwave", "oven", "toaster", "sink", "refrigerator", "book", "clock", "vase", "scissors", "teddy bear",
"hair drier", "toothbrush"]
def infer(img,pred,shape):
w_ratio=img.shape[1]/shape[0]
h_ratio=img.shape[0]/shape[1]
# print(w_ratio,h_ratio)
confidences=[]
boxes=[]
class_ids=[]
boxes_num=pred.shape[1]
data=pred[0]
# print("data_shape:",data.shape)
for i in range(boxes_num):
da=data[i]#[box,conf,cls]
confidence=da[4]
if confidence>0.6:
score=da[5:]*confidence
_,_,_,max_score_index=cv2.minMaxLoc(score)#
max_cls_id=max_score_index[1]
if score[max_cls_id]>0.25:
confidences.append(confidence)
class_ids.append(max_cls_id)
x,y,w,h=da[0].item(),da[1].item(),da[2].item(),da[3].item()
nx=int((x-w/2.0)*w_ratio)
ny=int((y-h/2.0)*h_ratio)
nw=int(w*w_ratio)
nh=int(h*h_ratio)
boxes.append(np.array([nx,ny,nw,nh]))
indexes=cv2.dnn.NMSBoxes(boxes,confidences,0.25,0.45)
res_ids=[]
res_confs=[]
res_boxes=[]
for i in indexes:
res_ids.append(class_ids[i])
res_confs.append(confidences[i])
res_boxes.append(boxes[i])
# print(res_ids)
# print(res_confs)
# print(res_boxes)
return res_ids,res_confs,res_boxes
def draw_rect(img,ids,confs,boxes):
for i in range(len(ids)):
cv2.rectangle(img, boxes[i], (0,0,255), 2)
cv2.rectangle(img, (boxes[i][0],boxes[i][1]-20),(boxes[i][0]+boxes[i][2],boxes[i][1]), (200, 200, 200), -1)
cv2.putText(img, classes[ids[i]], (boxes[i][0], boxes[i][1] - 10), cv2.FONT_HERSHEY_SIMPLEX, .5, (255, 0, 0))
cv2.putText(img, str(confs[i]), (boxes[i][0]+60, boxes[i][1] - 10), cv2.FONT_HERSHEY_SIMPLEX, .5, (255, 0, 0))
cv2.imwrite("res.jpg",img)
cv2.imshow('img',img)
cv2.waitKey()
if __name__=="__main__":
import time
st=time.time()
shape=(640,640)
src=cv2.imread("../data/bus.jpg")
img=src.copy()
net=cv2.dnn.readNet("../model_m1/yolov5s_sim.onnx")
blob=cv2.dnn.blobFromImage(img,1/255.,shape,swapRB=True,crop=False)
net.setInput(blob)
pred=net.forward()
print(pred.shape)
ids,confs,boxes=infer(img,pred,shape)
et=time.time()
print("run time:{:.2f}s/{:.2f}FPS".format(et-st,1/(et-st)))
draw_rect(src,ids,confs,boxes)
运行结果:
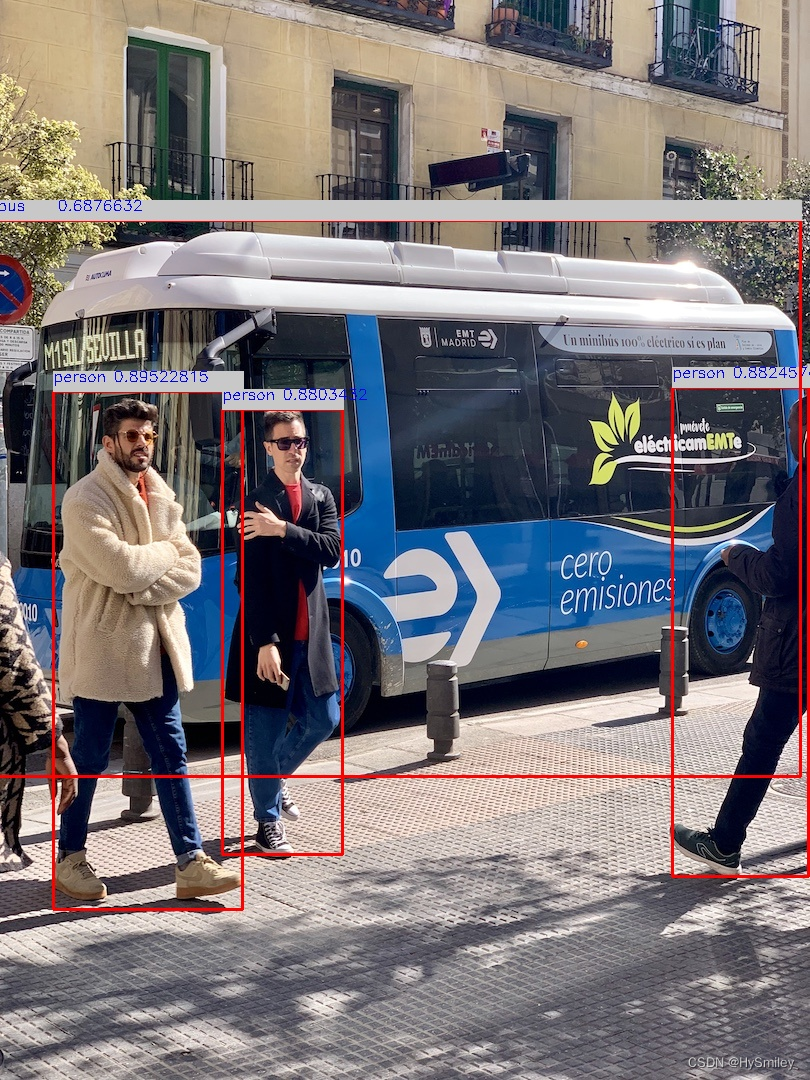
四、C++ 版本推理
cdnn.cpp
#include<iostream>
#include<opencv2/imgproc.hpp>
#include<opencv2/opencv.hpp>
void infer_res(cv::Mat& img,cv::Mat& preds,std::vector<cv::Rect> &boxes,std::vector<float>&confidences,std::vector<int> &classIds,std::vector<int>&indexs)
{
float w_ratio=img.cols/640.0;
float h_ratio=img.rows/640.0;
cv::Mat data(preds.size[1],preds.size[2],CV_32F,preds.ptr<float>());
for(int i=0;i<data.rows;i++)
{
float conf=data.at<float>(i,4);
if(conf<0.45)
{
continue;
}
cv::Mat clsP=data.row(i).colRange(5,85)*conf;
cv::Point IndexId;
double score;
minMaxLoc(clsP,0,&score,0,&IndexId);
if(score>0.25)
{
float x=data.at<float>(i,0);
float y=data.at<float>(i,1);
float w=data.at<float>(i,2);
float h=data.at<float>(i,3);
int nx=int((x-w/2.0)*w_ratio);
int ny=int((y-h/2.0)*h_ratio);
int nw=int(w*w_ratio);
int nh=int(h*h_ratio);
cv::Rect box;
box.x=nx;
box.y=ny;
box.width=nw;
box.height=nh;
boxes.push_back(box);
classIds.push_back(IndexId.x);
confidences.push_back(score);
}
}
// std::vector<int>indexs;
cv::dnn::NMSBoxes(boxes,confidences,0.25,0.45,indexs);
}
//void draw_label(cv::Mat& img,std::vector<cv::Rect> &boxes,std::vector<float>&confidences,std::vector<int> &classIds,std::vector<int>&indexs,std::string& classes[])
//{
// for(int i=0;i<boxes.size();i++)
// {
// cv::rectangle(img,boxes[i],(0,0,0),1);
//
// }
// cv::imshow("img",img);
// cv::waitKey();
//
//}
int main()
{
clock_t st=clock();
cv::Mat src=cv::imread("../../data/bus.jpg");
std::string classNames[]={ "person", "bicycle", "car", "motorcycle", "airplane", "bus", "train", "truck", "boat", "traffic light",
"fire hydrant", "stop sign", "parking meter", "bench", "bird", "cat", "dog", "horse", "sheep", "cow",
"elephant", "bear", "zebra", "giraffe", "backpack", "umbrella", "handbag", "tie", "suitcase", "frisbee",
"skis", "snowboard", "sports ball", "kite", "baseball bat", "baseball glove", "skateboard", "surfboard",
"tennis racket", "bottle", "wine glass", "cup", "fork", "knife", "spoon", "bowl", "banana", "apple",
"sandwich", "orange", "broccoli", "carrot", "hot dog", "pizza", "donut", "cake", "chair", "couch",
"potted plant", "bed", "dining table", "toilet", "tv", "laptop", "mouse", "remote", "keyboard", "cell phone",
"microwave", "oven", "toaster", "sink", "refrigerator", "book", "clock", "vase", "scissors", "teddy bear",
"hair drier", "toothbrush"};
cv::Mat img=src.clone();
cv::dnn::Net net=cv::dnn::readNet("../../model_m1/yolov5s_sim.onnx");
cv::Mat blob=cv::dnn::blobFromImage(img,1/255.0,cv::Size(640,640), cv::Scalar(0, 0, 0), true, false);
net.setInput(blob);
cv::Mat preds=net.forward();
std::vector<cv::Rect>boxes;
std::vector<float>confidences;
std::vector<int> classIds;
std::vector<int>indexs;
infer_res(src,preds,boxes,confidences,classIds,indexs);
clock_t et=clock();
std::cout<<"run time:"<<(double)(et-st)/CLOCKS_PER_SEC<<std::endl;
// draw_label(img,boxes,confidences,classIds,indexs,&classNames);
for(int i=0;i<indexs.size();i++)
{
cv::rectangle(src,boxes[indexs[i]],(0,0,255),2);
cv::rectangle(src,cv::Point(boxes[indexs[i]].tl().x,boxes[indexs[i]].tl().y-20),
cv::Point(boxes[indexs[i]].tl().x+boxes[indexs[i]].br().x,boxes[indexs[i]].tl().y),cv::Scalar(200,200,200),-1);
cv::putText(src,classNames[classIds[indexs[i]]], cv::Point(boxes[indexs[i]].tl().x+5, boxes[indexs[i]].tl().y - 10), cv::FONT_HERSHEY_SIMPLEX, .5, cv::Scalar(0, 0, 0));
std::ostringstream conf;
conf<<confidences[indexs[i]];
cv::putText(src,conf.str(), cv::Point(boxes[indexs[i]].tl().x+60, boxes[indexs[i]].tl().y - 10), cv::FONT_HERSHEY_SIMPLEX, .5, cv::Scalar(0, 0, 0));
}
cv::imwrite("res_.jpg",src);
cv::imshow("img",src);
cv::waitKey();
return 0;
}
CMakeLists.txt
cmake_minimum_required(VERSION 2.8)
project(cdnn)
find_package(OpenCV 4.5.1 REQUIRED)
include_directories(${OpenCV_INCLUDES_DIRS})
add_executable(cdnn cdnn.cpp)
target_link_libraries(cdnn ${OpenCV_LIBS})
执行:
mkdir build
cd build
cmake ..
make
./cdnn
运行结果:
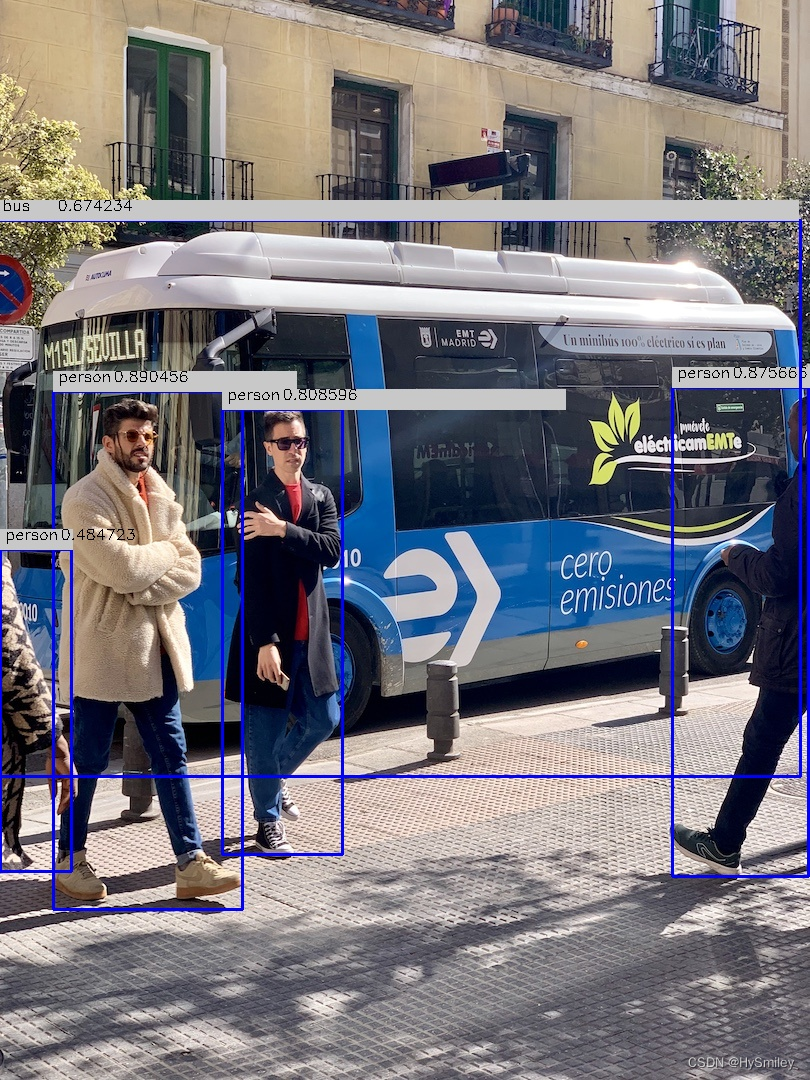
|