风格转换模型style_transformer项目实例 pytorch实现
有没有想过,利用机器学习来画画,今天,我将手把手带大家进入深度学习模型neural style的代码实战当中。 neural-style模型是一个风格迁移的模型,是GitHub上一个超棒的项目,那么什么是风格迁移,我们来举一个简单的例子: 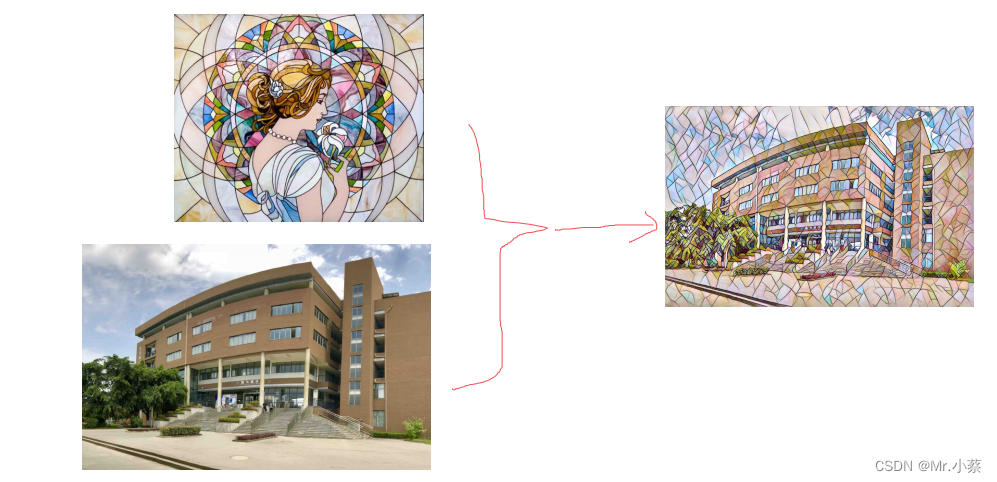 这个项目的理论指导来自论文:Perceptual Losses for Real-Time Style Transfer and Super-Resolution
一. 相关工作
相关神经网络架构: 本论文相关的前馈神经网络架构是基于“Deep residual learning for image recognition. ”以及 “Training and investigating residual nets.”两篇论文。 相关图像产生方法:本文中产生图像的方法是基于论文“Inverting visual representations with convolutional networks”,但并未使用其Pixel-Loss Function, 而是采用感知损失函数来代替求逐像素差距的损失函数。 此方法应用了前馈神经网络,与Gatys论文中使用的“Understanding deep image representations by inverting them”方法有相同效果但是速度更快。
二. 实现细节
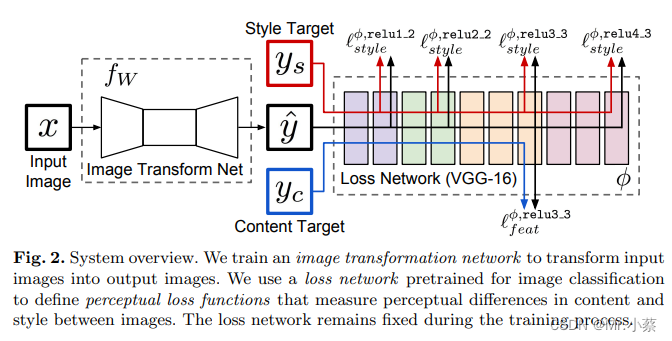 像图2中展示的那样,我们的系统由两部分组成:一个图片转换网络
f
w
fw
fw 和一个损失网络
?
\phi
?(用来定义一系列损失函数
l
1
,
l
2
,
l
3
l_1, l_2, l_3
l1?,l2?,l3?),图片转换网络是一个深度残差网络,参数是权重
W
W
W,它把输入的图片
x
x
x通过映射
y
^
=
f
w
(
x
)
\hat y=fw(x)
y^?=fw(x)转换成输出图片
y
^
\hat y
y^?,每一个损失函数计算一个标量值
l
i
(
y
^
,
y
i
)
l_i(\hat y,y_i)
li?(y^?,yi?), 衡量输出的
y
^
\hat y
y^?和目标图像
y
i
y_i
yi?之间的差距。图片转换网络是用SGD训练(代码实现时采用Adam),使得一系列损失函数的加权和保持下降。 图2:系统概览。左侧是Generator,右侧是预训练好的vgg16网络(一直固定).  损失网络
?
\phi
?是能定义一个特征(内容)损失
l
f
e
a
t
?
l_{feat}^{\phi}
lfeat??和一个风格损失
l
s
t
y
l
e
?
l_{style}^{\phi}
lstyle??,分别衡量内容和风格上的差距。对于每一张输入的图片
x
x
x我们有一个内容目标
y
c
y_c
yc?一个风格目标
y
s
y_s
ys?,对于风格转换,内容目标
y
c
y_c
yc?是输入图像
x
x
x,输出图像
y
y
y,应该把输出图像
y
s
y_s
ys?结合到内容
x
=
y
c
x=y_c
x=yc?上。我们为每一个目标风格训练一个网络。对于单图超分辨率重建,输入图像
x
x
x是一个低分辨率的输入,目标内容是一张真实的高分辨率图像,风格重建没有使用。我们为每一个超分辨率因子训练一个网络。
三. 图像转换网络
亮点: 使用残差网络下采样input image,然后再通过上采样插值产生新的output image. 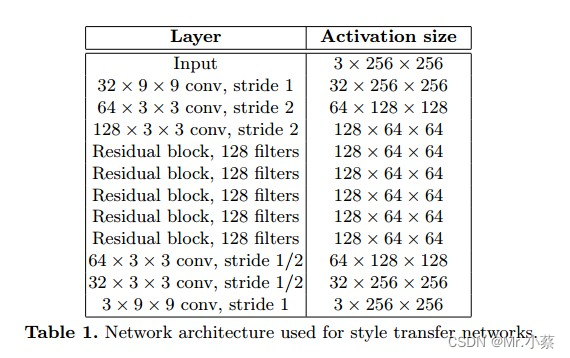
1. 使用残差网络如下所示:
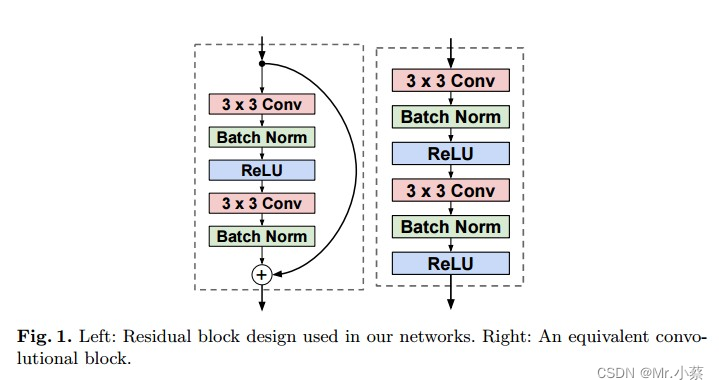
note:作者在论文中比较了使用residual block 和 normal convolutional network的表现,如Fig1所示,结果显示residual block会收敛的比较快一些,但最终结果两者差不多。作者推测可能残差网络在更深的网络中可能会表现更好。
四. Loss Functions 细节
1. Content Loss Function
我们不建议做逐像素对比,而是用VGG计算来高级特征(内容)表示,这个取法和那篇artistic style使用VGG-19提取风格特征是一样的,公式:  找到一个图像
y
^
\hat y
y^?使较低的层的特征损失最小,往往能产生在视觉上和
y
y
y不太能区分的图像,如果用高层来重建,内容和全局结构会被保留,但是颜色纹理和精确的形状不复存在。用一个特征损失来训练我们的图像转换网络能让输出非常接近目标图像y,但并不是让他们做到完全的匹配。
2. Style Reconstruction Loss
特征(内容)损失惩罚了输出的图像(当它偏离了目标
y
y
y时),所以我们也希望去惩罚风格上的偏离:颜色,纹理,共同的模式,等方面。为了达成这样的效果Gatys等人提出了以下风格重建的损失函数。
其中
?
j
(
x
)
\phi_j(x)
?j?(x)代表网络
?
\phi
?的第
j
j
j层,输入是
x
x
x。特征图谱的形状就是
C
j
×
H
j
×
W
j
C_j × H_j × W_j
Cj?×Hj?×Wj?、定义Gram矩阵
G
j
?
(
x
)
G^{\phi}_j(x)
Gj??(x)为
C
j
×
C
j
C_j × C_j
Cj?×Cj?矩阵(特征矩阵)其中的元素来自于:  如果我们把
?
j
(
x
)
\phi_j(x)
?j?(x)理解成一个
C
j
C_j
Cj?维度的特征,每个特征的尺寸是
H
j
×
W
j
H_j × W_j
Hj?×Wj?,那么上式左边
G
j
(
x
)
G_j(x)
Gj?(x)就是与
C
j
C_j
Cj?维的偏心的协方差成比例。每一个网格位置都可以当做一个独立的样本。这因此能抓住是哪个特征能带动其他的信息。 风格损失函数是输出图片
y
^
\hat y
y^? 和目标图片
y
y
y之间的gram矩阵: 
五. 代码实现
1. 风格转换网络:
class TransformerNet(nn.Module):
def __init__(self):
super(TransformerNet, self).__init__()
self.conv1 = ConvLayer(3, 32, kernel_size=9, stride=1)
self.in1 = nn.InstanceNorm2d(32, affine=True)
self.conv2 = ConvLayer(32, 64, kernel_size=3, stride=2)
self.in2 = nn.InstanceNorm2d(64, affine=True)
self.conv3 = ConvLayer(64, 128, kernel_size=3, stride=2)
self.in3 = nn.InstanceNorm2d(128, affine=True)
self.res1 = ResidualBlock(128)
self.res2 = ResidualBlock(128)
self.res3 = ResidualBlock(128)
self.res4 = ResidualBlock(128)
self.res5 = ResidualBlock(128)
self.deconv1 = UpsampleConvLayer(128, 64, kernel_size=3, stride=1, upsample=2)
self.in4 = nn.InstanceNorm2d(64, affine=True)
self.deconv2 = UpsampleConvLayer(64, 32, kernel_size=3, stride=1, upsample=2)
self.in5 = nn.InstanceNorm2d(32, affine=True)
self.deconv3 = ConvLayer(32, 3, kernel_size=9, stride=1)
self.relu = nn.ReLU()
def forward(self, x):
y = self.relu(self.in1(self.conv1(x)))
y = self.relu(self.in2(self.conv2(y)))
y = self.relu(self.in3(self.conv3(y)))
y = self.res1(y)
y = self.res2(y)
y = self.res3(y)
y = self.res4(y)
y = self.res5(y)
y = self.relu(self.in4(self.deconv1(y)))
y = self.relu(self.in5(self.deconv2(y)))
y = self.deconv3(y)
return y
2. 残差模块
class ResidualBlock(nn.Module):
"""ResidualBlock
introduced in: https://arxiv.org/abs/1512.03385
recommended architecture: http://torch.ch/blog/2016/02/04/resnets.html
"""
def __init__(self, channels):
super(ResidualBlock, self).__init__()
self.conv1 = ConvLayer(channels, channels, kernel_size=3, stride=1)
self.in1 = nn.InstanceNorm2d(channels, affine=True)
self.conv2 = ConvLayer(channels, channels, kernel_size=3, stride=1)
self.in2 = nn.InstanceNorm2d(channels, affine=True)
self.relu = nn.ReLU()
def forward(self, x):
residual = x
out = self.relu(self.in1(self.conv1(x)))
out = self.in2(self.conv2(out))
out = out + residual
return out
3. 上采样模块
class UpsampleConvLayer(nn.Module):
"""UpsampleConvLayer
Upsamples the input and then does a convolution. This method gives better results
compared to ConvTranspose2d.
ref: http://distill.pub/2016/deconv-checkerboard/
"""
def __init__(self, in_channels, out_channels, kernel_size, stride, upsample=None):
super(UpsampleConvLayer, self).__init__()
self.upsample = upsample
reflection_padding = kernel_size // 2
self.reflection_pad = nn.ReflectionPad2d(reflection_padding)
self.conv2d = nn.Conv2d(in_channels, out_channels, kernel_size, stride)
def forward(self, x):
x_in = x
if self.upsample:
x_in = nn.functional.interpolate(x_in, mode='nearest', scale_factor=self.upsample)
out = self.reflection_pad(x_in)
out = self.conv2d(out)
return out
4. 基础网络模块
class ConvLayer(nn.Module):
def __init__(self, in_channels, out_channels, kernel_size, stride):
super(ConvLayer, self).__init__()
reflection_padding = kernel_size // 2
self.reflection_pad = nn.ReflectionPad2d(reflection_padding)
self.conv2d = nn.Conv2d(in_channels, out_channels, kernel_size, stride)
def forward(self, x):
out = self.reflection_pad(x)
out = self.conv2d(out)
return out
class ResidualBlock(nn.Module):
"""ResidualBlock
introduced in: https://arxiv.org/abs/1512.03385
recommended architecture: http://torch.ch/blog/2016/02/04/resnets.html
"""
def __init__(self, channels):
super(ResidualBlock, self).__init__()
self.conv1 = ConvLayer(channels, channels, kernel_size=3, stride=1)
self.in1 = nn.InstanceNorm2d(channels, affine=True)
self.conv2 = ConvLayer(channels, channels, kernel_size=3, stride=1)
self.in2 = nn.InstanceNorm2d(channels, affine=True)
self.relu = nn.ReLU()
def forward(self, x):
residual = x
out = self.relu(self.in1(self.conv1(x)))
out = self.in2(self.conv2(out))
out = out + residual
return out
我的项目链接:Style_Transformer
|