【深度学习】-Imdb数据集情感分析之模型对比(4)- CNN-LSTM 集成模型
前言
【深度学习】-Imdb数据集情感分析之模型对比(3)- CNN 【深度学习】-Imdb数据集情感分析之模型对比(2)- LSTM 【深度学习】-Imdb数据集情感分析之模型对比(1)- RNN 对之前内容感兴趣的朋友可以参考上面这几篇文章,接下来我要给大家介绍本篇博客的内容。为了观察CNN提取文本结构化的优势和LSTM提取上下文关系性的优势,我们将两种模型进行集成,引入了CNN-LSTM集成模型进行实验。先经过CNN层来提取局部特征,再使用LSTM层提取这些局部特征的长距离特征,再经变换输入全连接层,进行情感分析并打分量化,最后将两种模型的分数平均,从而得到最终分数。
一,CNN是什么?
CNN网络适合于提取数据的结构化信息,因此在特征工程中得到了广泛的应用,而LSTM网络更适合于提取数据的时间相关性和文本片段中的依赖项,有按时间顺序扩张的特性,广泛应用于时间序列中。同时,这给我们在情感分析任务上带来一个新思路,可以将两者结合,构建新的集成模型CNN-LSTM的对文本进行分类。模型结构如图所示: 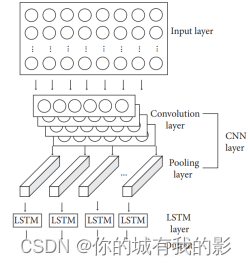
二、训练CNN-LSTM模型
1.数据预处理
与前文类似,详细请移步【深度学习】-Imdb数据集情感分析之模型对比(1)- RNN 数据预处理部分
2.构建及训练CNN-LSTM模型
模型结构
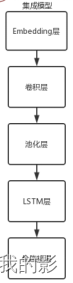
设定模型参数
filter_length = 5
pool_length = 4
max_features = 4000
maxlen = 400
embedding_size = 32
nb_filter = 32
filter_length = 3
hidden_dims = 256
构建网络模型
model = Sequential()
model.add(Embedding(max_features, embedding_size, input_length=400))
model.add(Dropout(0.2))
lstm_output_size =32
model.add(Convolution1D(nb_filter=nb_filter,
filter_length=filter_length,
border_mode='valid',
activation='relu',
subsample_length=1))
model.add(MaxPooling1D(pool_length=pool_length))
model.add(LSTM(lstm_output_size))
model.add(Dense(1))
model.add(Activation('sigmoid'))
model.summary()
训练模型
定义损失函数,优化器以及评估矩阵,并开始训练模型。
model.compile(loss='binary_crossentropy',
optimizer='adam',
metrics=['accuracy'])
train_history =model.fit(x_train, y_train,batch_size=32,
epochs=10,verbose=1,
validation_split=0.2)
三、可视化结果
import matplotlib.pyplot as plt
def show_train_history(train_history,train,validation):
plt.plot(train_history.history[train])
plt.plot(train_history.history[validation])
plt.title('Train History')
plt.ylabel(train)
plt.xlabel('Epoch')
plt.legend(['train', 'validation'], loc='upper left')
plt.show()
show_train_history(train_history,'accuracy','val_accuracy')
show_train_history(train_history,'loss','val_loss')
print('Test score:', score)
print('Test accuracy:', acc)
因为博主的数据集删除了就不放可视化图片了,下次一定。
?四、模型预测
请参照我这篇博客下的模型预测代码。
【深度学习】-Imdb数据集情感分析之模型对比(3)- CNN
五、评估模型并保存
模型评估
用测试集对模型的匹配精度进行评估
score, acc = model.evaluate(x_test, y_test,
batch_size=128)
保存模型
model_json = model.to_json()
with open("D:/final_all/1/1.json", "w") as json_file:
json_file.write(model_json)
model.save_weights("D:/final_all/1/2.h5")
print("Saved model to disk")
六、总结
CNN-LSTM模型训练总用时670s,训练集上的准确率为98.96%,在测试集上的准确率为90.02%,损失率在7.5%,是这几个模型中最优良的模型。
实验总结可以参照我的下一篇博客,有我的整个实验的对比分析以及总结。
参考资料
https://blog.csdn.net/keeppractice/article/details/106145451
|