前言
RNN的特点是拥有储存上一次节点的输出结果的能力,因此就算是同样的输入集合,只要改变其输入序列,输出结果就会完全不一样。在本次学习中,主要展示了RNN的正向和反向手动推导过程,用代码逐行实现单向和双向RNN,并与PyTorch API输出的结果进行验证正确。
一、RNN的手推过程
1. 如下图所示,这是我们要手推RNN模型的内部结构示意图。图中的t下标指当前时间节点,St由Whh矩阵和Whx矩阵竖着拼接,乘上横着拼接的ht-1和Xt得到;ht由tanh作为激活函数,St作为参数得到,在这里每一轮产生的ht将会更新到下一轮使用;Ot由Wyh乘上ht得到;最后用softmax得到概率分布。 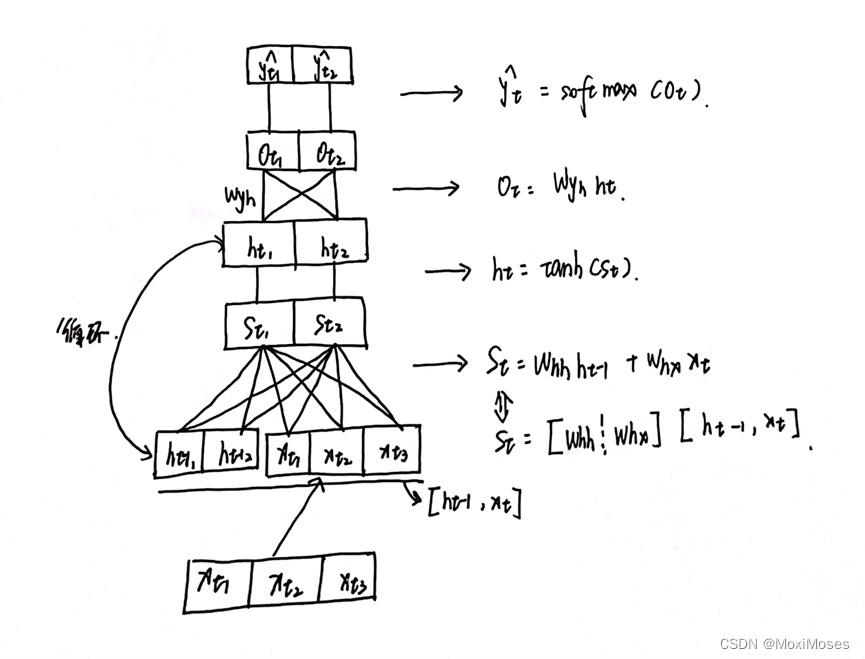 2. 如下图所示,这是我们制定的任务,训练数据用one-hot vector表示。图中的最下面是RNN的展开,因为我们只需要最后一次的输出,所以就无需计算出第一次和第二次的输出。 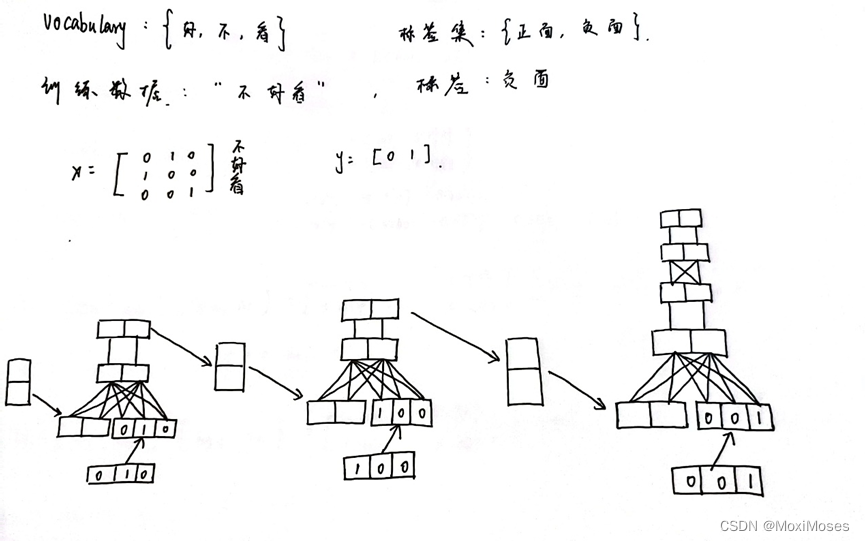 3. 如下图所示,初始化参数。 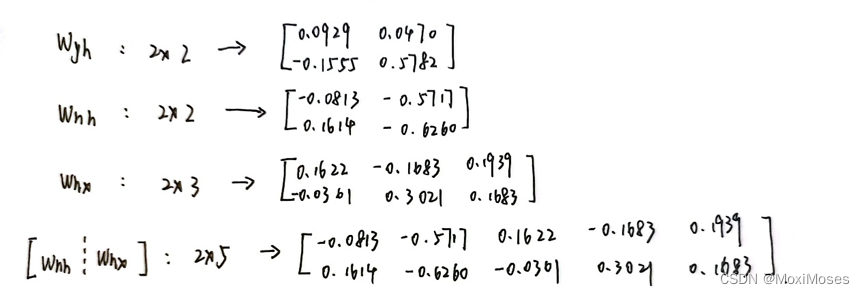 4. 正向运算 1)我们设定初始的h0为0,于是计算出t=1时的s1、h1,t=2时s2、h2。 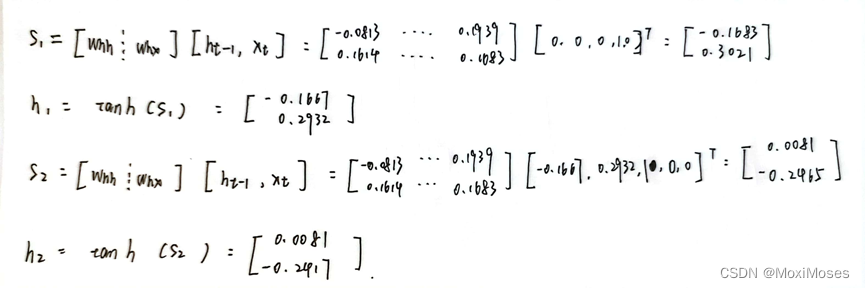 2)当t=3时,计算出s3、h3、O,最后把O放入softmax中得到概率分布。 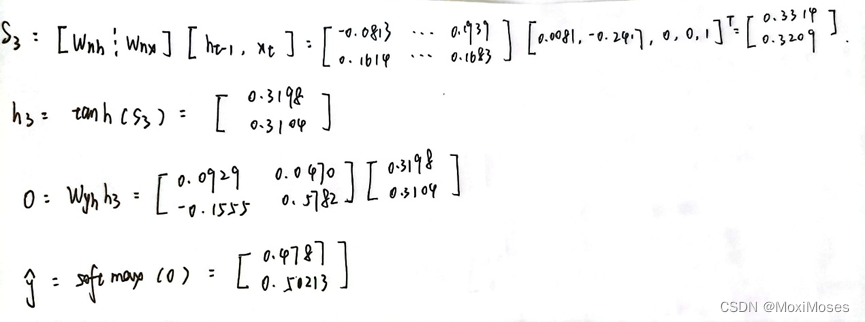 5. 反向运算 反向运算中比较复杂的是loss对Whh求导和loss对Whx求导,原因是Whh和Whx对Loss的影响有3种情况,一种是h3直接影响,另一种是h2、h3影响,最后一种是h1、h2与h3影响。 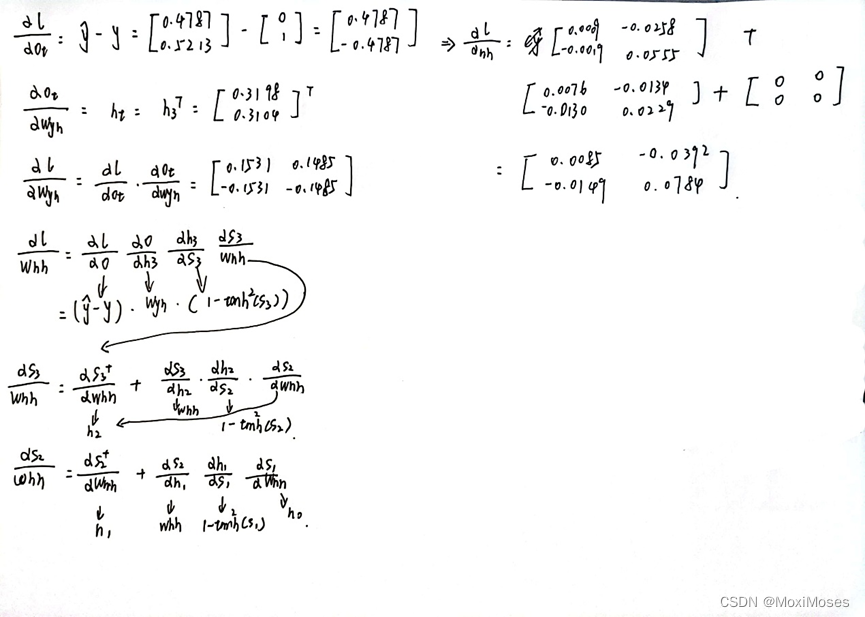 6. 通过反向运算得到的gradient,更新参数。 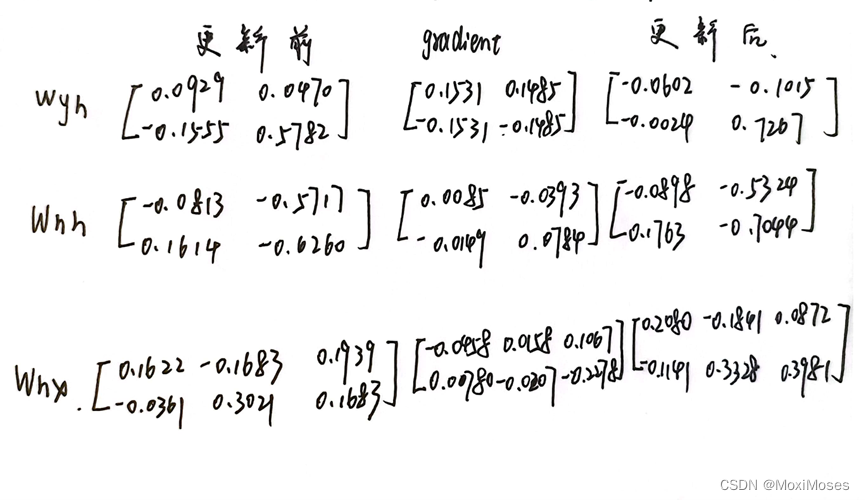
二、RNN代码实现
1、PyTorch API实现
1)实现单向、单层RNN 首先实例化对象single_rnn,然后调用正态分布随机函数torch.randn生成输入,最后将生成的输入作为single_rnn的输入,并且得到输出和最后时刻状态。
single_rnn = nn.RNN(4, 3, 1, batch_first=True)
input = torch.randn(1, 2, 4)
output, h_n = single_rnn(input)
print(output)
print(h_n)
查看输出结果。  2)实现双向、单层RNN 首先实例化对象bidirectional_rnn,在这里需要加上bidirectional=True即可实现双向RNN,然后给bidirectional_rnn输入,得到输出和最后时刻状态。
bidirectional_rnn = nn.RNN(4, 3, 1, batch_first=True, bidirectional=True)
bi_output, bi_h_n = bidirectional_rnn(input)
print(bi_output)
print(bi_h_n)
查看输出结果。 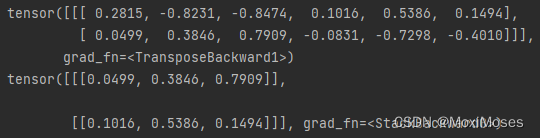 3)比较单向、单层RNN和双向、单层RNN
print(output.shape)
print(bi_output.shape)
print(h_n.shape)
print(bi_h_n.shape)
从输出上来看,单向的RNN维度是1 * 2 * 3,而双向的RNN维度是1 * 2 * 6,原因是双向RNN把forward和backward的结果拼在一起;从最后时刻状态来看,单向的RNN维度是1 * 1 * 3,而双向的RNN维度是2 * 1 * 3,原因是双向RNN在最后时刻有两个层,而单向RNN在最后时刻有一个层。 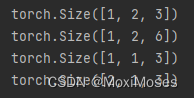
2、代码逐行实现RNN
1)首先初始一些张量,然后调用正态分布随机函数随机初始化一个输入特征序列,以及初始隐含状态(设为0)。
import torch
import torch.nn as nn
bs, T = 2, 3
input_size, hidden_size = 2, 3
input = torch.randn(bs, T, input_size)
h_prev = torch.zeros(bs, hidden_size)
2)手写一个rnn_forward函数,手动地模拟单向RNN的运算过程,并且与PyTorch RNN API进行比较,验证输出结果。
rnn = nn.RNN(input_size, hidden_size, batch_first=True)
rnn_output, state_final = rnn(input, h_prev.unsqueeze(0))
print("PyTorch API output:")
print(rnn_output)
print(state_final)
def rnn_forward(input, weight_ih, weight_hh, bias_ih, bias_hh, h_prev):
bs, T, input_size = input.shape
h_dim = weight_ih.shape[0]
h_out = torch.zeros(bs, T, h_dim)
for t in range(T):
x = input[:, t, :].unsqueeze(2)
w_ih_batch = weight_ih.unsqueeze(0).tile(bs, 1, 1)
w_hh_batch = weight_hh.unsqueeze(0).tile(bs, 1, 1)
w_times_x = torch.bmm(w_ih_batch, x).squeeze(-1)
w_times_h = torch.bmm(w_hh_batch, h_prev.unsqueeze(2)).squeeze(-1)
h_prev = torch.tanh(w_times_x + bias_ih + w_times_h + bias_hh)
h_out[:, t, :] = h_prev
return h_out, h_prev.unsqueeze(0)
custom_rnn_output, custom_state_final = rnn_forward(input, rnn.weight_ih_l0, rnn.weight_hh_l0,
rnn.bias_ih_l0, rnn.bias_hh_l0, h_prev)
print("rnn_forward function output:")
print(custom_rnn_output)
print(custom_state_final)
查看输出结果以及并用torch.allclose验证最后时刻的结果
print(torch.allclose(state_final, custom_state_final))
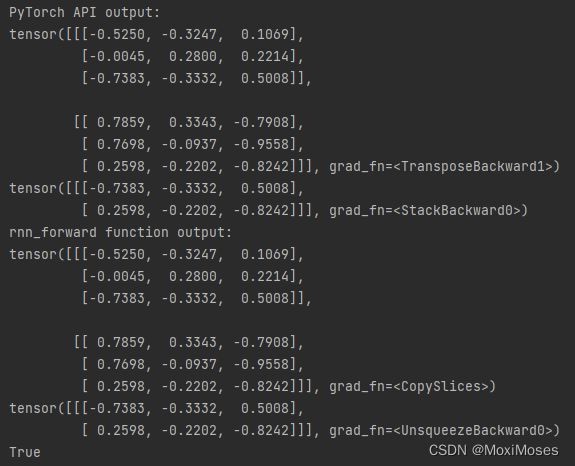 3)手写一个bidirectional_rnn_forward函数,手动地模拟双向RNN的运算过程,并且与PyTorch RNN API进行比较,验证输出结果。
def bidirectional_rnn_forward(input, weight_ih, weight_hh, bias_ih, bias_hh, h_prev,
weight_ih_reverse, weight_hh_reverse, bias_ih_reverse, bias_hh_reverse, h_prev_reverse):
bs, T, input_size = input.shape
h_dim = weight_ih.shape[0]
h_out = torch.zeros(bs, T, h_dim * 2)
forward_output = rnn_forward(input, weight_ih, weight_hh, bias_ih, bias_hh, h_prev)[0]
backward_output = rnn_forward(torch.flip(input, [1]), weight_ih_reverse, weight_hh_reverse, bias_ih_reverse,
bias_hh_reverse, h_prev_reverse)[0]
h_out[:, :, :h_dim] = forward_output
h_out[:, :, h_dim:] = backward_output
return h_out, h_out[:, -1, :].reshape((bs, 2, h_dim)).transpose(0, 1)
bi_rnn = nn.RNN(input_size, hidden_size, batch_first=True, bidirectional=True)
h_prev = torch.zeros(2, bs, hidden_size)
bi_rnn_output, bi_state_final = bi_rnn(input, h_prev)
custom_bi_rnn_output, custom_bi_state_final = bidirectional_rnn_forward(input, bi_rnn.weight_ih_l0, bi_rnn.weight_hh_l0,
bi_rnn.bias_ih_l0, bi_rnn.bias_hh_l0,
h_prev[0], bi_rnn.weight_ih_l0_reverse,
bi_rnn.weight_hh_l0_reverse,
bi_rnn.bias_ih_l0_reverse,
bi_rnn.bias_hh_l0_reverse, h_prev[1])
print("PyTorch API output:")
print(bi_rnn_output)
print(bi_state_final)
print("bidirectional_rnn_forward function output:")
print(custom_bi_rnn_output)
print(custom_bi_state_final)
查看输出结果以及并用torch.allclose验证最后时刻的结果
print(torch.allclose(bi_state_final, custom_bi_state_final))
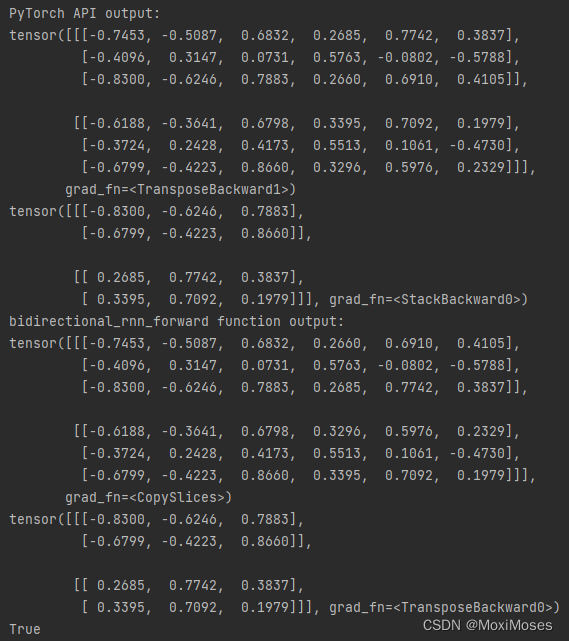
总结
在本次的学习中,通过对RNN的手动推导与代码逐行实现,加深了自己对RNN的理解与推导。RNN其实就是给模型一个记忆的功能,让之后每一步的输出对于前面的输入有关,因此随着时间变化的数据,使用RNN会比较好,但RNN也存在一些问题,随着时序长度变长,RNN的深度也会变深,这就会导致出现梯度爆炸和梯度消失的问题,于是出现了对RNN的改进,因此在下次的学习中我将继续学习LSTM。
|