🚩前言
- 🐳博客主页:😚睡晚不猿序程😚
- ?首发时间:2022.8.13
- ?最近更新时间:2022.8.13
- 🙆本文由 睡晚不猿序程 原创,首发于 CSDN
- 🤡作者是蒻蒟本蒟,如果文章里有任何错误或者表述不清,请 tt 我,万分感谢!orz
相关文章目录 :
1. 内容简介
上一次我们完成了基于 RNN 的图片描述网络,RNN 网络有些许的不足,比如长程依赖问题,在这里我们会完成基于 LSTM 的图片描述模型,将会很好的改善这一问题
2. LSTM
在开始做作业之前,先来回顾一下 LSTM 的内容吧
参考资料:LSTM 详解,作者:qian99
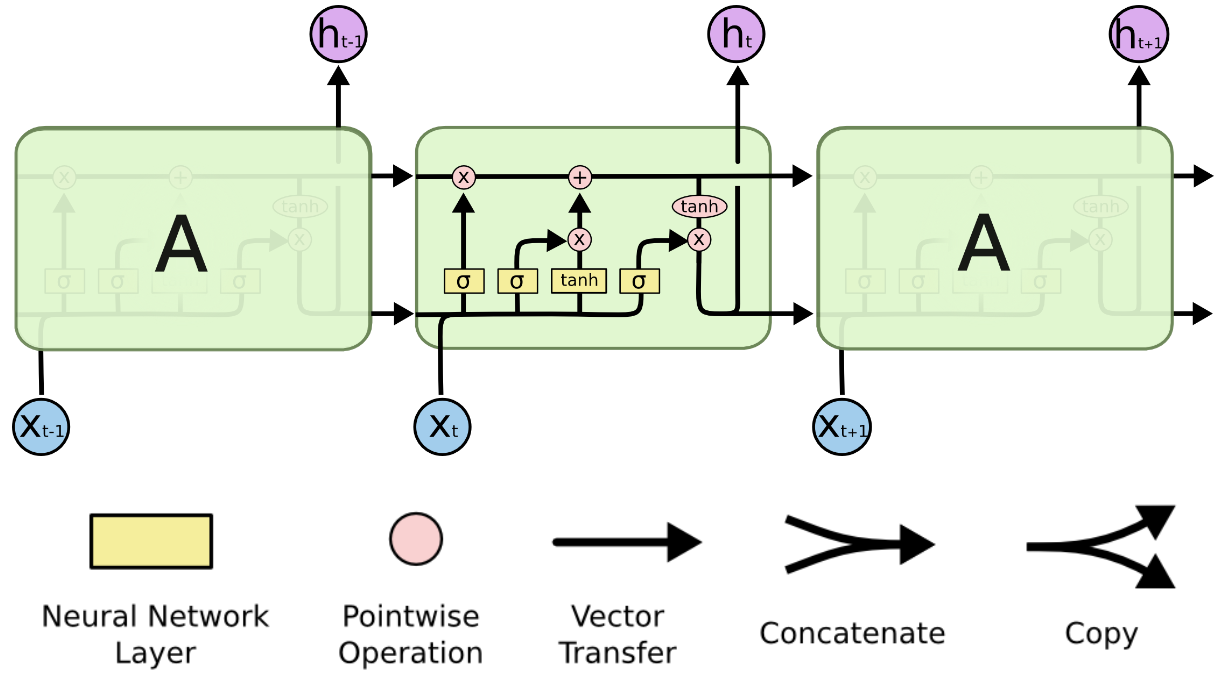
相比于 RNN,LSTM 增加了一个细胞状态(cell state)
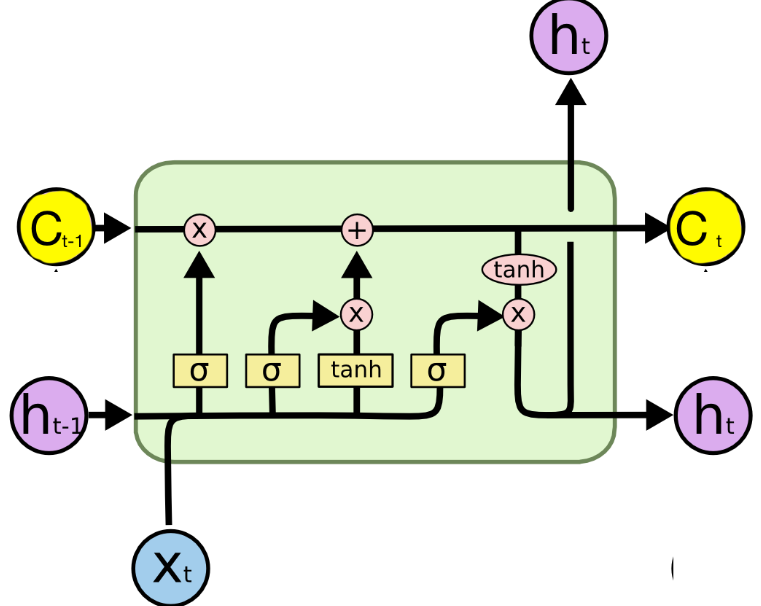
也就是说,对一个一个 LSTM 单元,输入有三个:
- 上一次的隐状态
h
t
?
1
h_{t-1}
ht?1?
- 上一次细胞状态
C
t
?
1
C_{t-1}
Ct?1?
- 当前输入
x
t
x_t
xt? ,
输出也有两个:
- 本单元的隐状态
h
t
h_t
ht?
- 本单元的细胞状态
C
t
C_t
Ct?
我们可以把 LSTM 的传播分为两条线,细胞状态的信息
C
C
C 一直只在上面的线上传播
隐状态一直在下面的线上传播,并且他们之间会做交互,LSTM 中包含有三个“门结构”
2.1 LSTM 的输入输出
LSTM 也是 RNN 的一种,所以我们要喂给她一个时序的数据
LSTM 有两个隐藏状态,也就是原先的隐状态和另一个细胞状态,一般初始化为0
2.2 LSTM 的门结构
门,就是被设计出来的一些计算步骤,经过这些计算,来调整输入和两个隐层的值
我们在看一下图,黄色的框框代表一个神经元,也就是一次
w
T
x
+
b
w^Tx+b
wTx+b 的操作,里面的符号是激活函数,分别是 sigmoid 和 tanh

而红色小圈圈代表着按元素计算
2.2.1 遗忘门
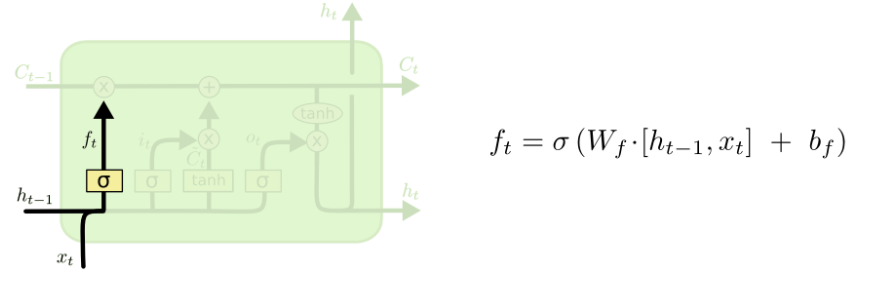
[
h
t
?
1
,
x
t
]
[h_{t-1},x_t]
[ht?1?,xt?]:意思是 h 和 x 两个张量进行链接
因为该门输出被限制为(0,1),接下来会和 C 进行按元素乘法,所以 C 中有些信息就会被忘记了(只记住一部分)
因为:只有在乘 1 的情况下,信息才会被完全保留
2.2.2 输入门
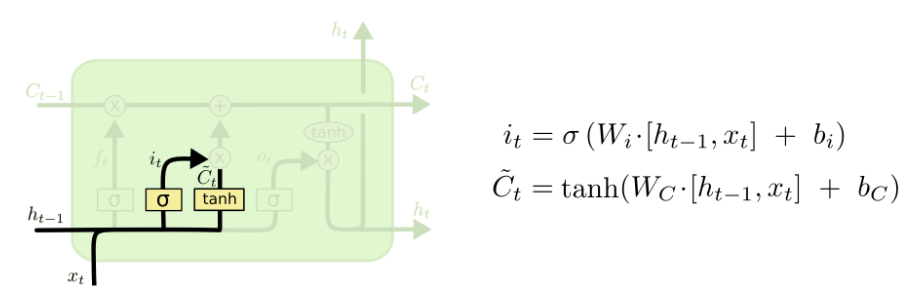
该门由两部分组成:
C
~
t
\tilde{C}_t
C~t? :可以看作是新的输入所带来的信息
i
t
i_t
it?:和遗忘门的结构一样,所以可以看作是我们要保留多少新的信息
2.2.3 细胞状态更新
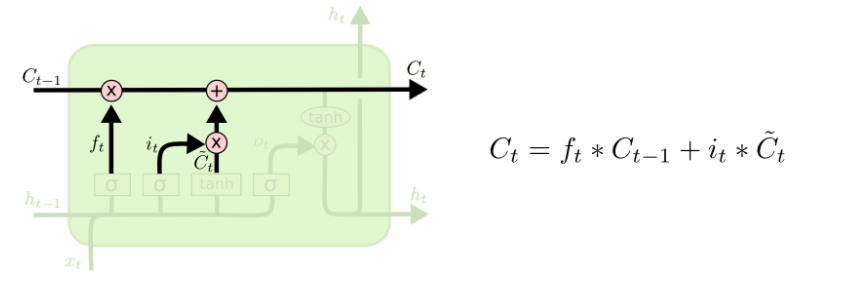
进行细胞状态的更新,也就是我们要忘掉多少以前的信息,记住多少新来的信息,接着两个合成一个当前的新状态,代表当前的全部信息
2.2.4 输出门
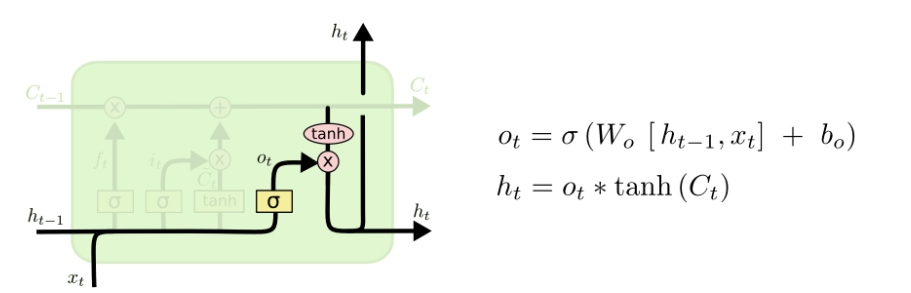
输出门得到的 LSTM 的输出,此时使用的是
C
t
C_t
Ct?
我们经过了一个 sigmoid 函数来进行处理,决定输出哪一些内容
C
t
C_t
Ct? 经过了 tanh 的处理,输出范围为(-1,1),然后乘上输出门的结果,就是当前的输出
h
t
h_t
ht?
2.2.5 小总结
所以这里总共有三套参数
- 遗忘门权重,偏置
- 输入门权重,偏置
-
C
~
t
\tilde{C}_t
C~t? 计算所需的权重,偏置
- 输出门权重,偏置
所以总共有四套可学习的参数~
3. LSTM for Image Caption
接下来我们要开始做作业了
3.1 LSTM
LSTM RNN 是简单 RNN 的一个常见变种。
简单 RNN 在长序列数据集上进行训练的时候容易出现梯度爆炸和梯度消失,这是多次的矩阵乘法导致的。LSTM 改善了这一问题,它使用“门机制”替代了 RNN 原本的简单更新规则
使用代码实现的时候,我们把上面四个门的权重 W 存储在一个矩阵里面来方便运算
3.1.1 变量
在每一个时间步:
输入:
x
t
∈
R
D
x_t \in \mathbb{R}^D
xt?∈RD
先前的隐状态:
h
t
?
1
∈
R
H
h_{t-1} \in \mathbb{R}^H
ht?1?∈RH
先前的细胞状态:
c
t
?
1
∈
R
H
c_{t-1}\in \mathbb{R}^H
ct?1?∈RH ,其维度和 h相同
可学习的参数:
- input-to-hidden 的矩阵:
W
x
∈
R
4
H
×
D
W_x \in \mathbb{R}^{4H \times D}
Wx?∈R4H×D
- hidden-to-hidden 的矩阵:
W
h
∈
R
4
H
×
H
W_h \in \mathbb{R}^{4H \times H}
Wh?∈R4H×H
- 偏置向量:
b
∈
R
4
H
b \in \mathbb{R}^{4H}
b∈R4H
3.1.2 步骤
在每一个时间步:
-
计算激活向量
a
∈
R
4
H
a\in\mathbb{R}^{4H}
a∈R4H:
a
=
W
x
x
t
+
W
h
h
t
?
1
+
b
a=W_xx_t + W_hh_{t-1}+b
a=Wx?xt?+Wh?ht?1?+b -
把得到的
a
a
a 划分为四个向量:
a
i
,
a
f
,
a
o
,
a
g
∈
R
H
a_i,a_f,a_o,a_g\in\mathbb{R}^H
ai?,af?,ao?,ag?∈RH -
分别计算四个门(就是经过激活函数处理)
- input gate:i
- forget gate:f
- output gate:o
- block gate:g
-
进行细胞状态 c 和隐藏状态 h 的更新
-
c
t
=
f
⊙
c
t
?
1
+
i
⊙
g
c_{t} = f\odot c_{t-1} + i\odot g \hspace{4pc}
ct?=f⊙ct?1?+i⊙g
-
h
t
=
o
⊙
tanh
?
(
c
t
)
h_t = o\odot\tanh(c_t)
ht?=o⊙tanh(ct?)
代码相关
接下来我们假设:
数据:
X
t
∈
R
N
×
D
X_t \in \mathbb{R}^{N\times D}
Xt?∈RN×D,
H
t
∈
R
N
×
H
H_t \in \mathbb{R}^{N \times H}
Ht?∈RN×H
参数:
W
x
∈
R
D
×
4
H
W_x \in \mathbb{R}^{D \times 4H}
Wx?∈RD×4H,
W
h
∈
R
H
×
4
H
W_h \in \mathbb{R}^{H\times 4H}
Wh?∈RH×4H
激活状态:
A
∈
R
N
×
4
H
A \in \mathbb{R}^{N\times 4H}
A∈RN×4H,它可以计算的非常快,公式:
A
=
X
t
W
x
+
H
t
?
1
W
h
A = X_t W_x + H_{t-1} W_h
A=Xt?Wx?+Ht?1?Wh?
到现在我们可以理清楚在当前作业我们需要做的内容了,现在我们准备开干
3.2 LSTM: Step Forward
我们要完成一个时间步的前向传播
完成文件cs231n/rnn_layers.py 中的lstm_step_forward 函数,它将会和rnn的相似
有了上面的铺垫,做前向传播应该很容易,我们直接来看代码
def lstm_step_forward(x, prev_h, prev_c, Wx, Wh, b):
"""Forward pass for a single timestep of an LSTM.
The input data has dimension D, the hidden state has dimension H, and we use
a minibatch size of N.
输入数据维度 D,隐状态维度 H,minibatch 大小为N
Note that a sigmoid() function has already been provided for you in this file.
Inputs:
- x: Input data, of shape (N, D)
- prev_h: Previous hidden state, of shape (N, H)
- prev_c: previous cell state, of shape (N, H)
- Wx: Input-to-hidden weights, of shape (D, 4H)
- Wh: Hidden-to-hidden weights, of shape (H, 4H)
- b: Biases, of shape (4H,)
Returns a tuple of:
- next_h: Next hidden state, of shape (N, H)
- next_c: Next cell state, of shape (N, H)
- cache: Tuple of values needed for backward pass.
"""
next_h, next_c, cache = None, None, None
H = prev_h.shape[1]
a = x.dot(Wx)+prev_h.dot(Wh)+b
input_gate = a[:, 0:H]
forget_gate = a[:, H:2*H]
output_gate = a[:, 2*H:3*H]
block_gate = a[:, 3*H:4*H]
i = sigmoid(input_gate)
f = sigmoid(forget_gate)
o = sigmoid(output_gate)
c = np.tanh(block_gate)
c_forgot = prev_c*f
c_input = c*i
next_c = c_forgot+c_input
next_h = o*np.tanh(next_c)
cache = (x, Wx, Wh, a, prev_c, prev_h, next_c)
return next_h, next_c, cache
代码详解
- 上面的讲解是将输入和隐藏状态拼接起来再进行矩阵运算,而这里选择的是分开计算,两个效果相同
3.3 LSTM: Step Backward
在这里我们要实现反向传播
根据上面的前向传播我们可以看出,这里的计算大部分都是矩阵的按元素运算,所以反向传播就是把梯度从后往前算一下就可以啦,也就是前向传播的逆过程。但是我们先注意一下 sigmoid 函数和 tanh 的求导公式:
?
σ
?
x
=
σ
(
x
)
(
1
?
σ
(
x
)
)
?
t
a
n
h
?
x
=
1
?
t
a
n
h
2
(
x
)
\frac{\partial \sigma}{\partial x}=\sigma(x)(1-\sigma(x)) \\ \frac{\partial tanh}{\partial x}=1-tanh^2(x)
?x?σ?=σ(x)(1?σ(x))?x?tanh?=1?tanh2(x) 了解了公式我们就可以进行反向传播了
def lstm_step_backward(dnext_h, dnext_c, cache):
"""Backward pass for a single timestep of an LSTM.
Inputs:
- dnext_h: Gradients of next hidden state, of shape (N, H)
- dnext_c: Gradients of next cell state, of shape (N, H)
- cache: Values from the forward pass
Returns a tuple of:
- dx: Gradient of input data, of shape (N, D)
- dprev_h: Gradient of previous hidden state, of shape (N, H)
- dprev_c: Gradient of previous cell state, of shape (N, H)
- dWx: Gradient of input-to-hidden weights, of shape (D, 4H)
- dWh: Gradient of hidden-to-hidden weights, of shape (H, 4H)
- db: Gradient of biases, of shape (4H,)
"""
dx, dprev_h, dprev_c, dWx, dWh, db = None, None, None, None, None, None
x, Wx, Wh, a, prev_c, prev_h, next_c = cache
H = prev_c.shape[1]
input_gate = a[:, 0:H]
forget_gate = a[:, H:2*H]
output_gate = a[:, 2*H:3*H]
block_gate = a[:, 3*H:4*H]
i = sigmoid(input_gate)
f = sigmoid(forget_gate)
o = sigmoid(output_gate)
c = np.tanh(block_gate)
do = dnext_h*np.tanh(next_c)
dnext_c += dnext_h * o * (1 - np.tanh(next_c)**2)
dc_forgot = dnext_c
dc_input = dnext_c
dc = i*dc_input
di = c*dc_input
dprev_c = f*dc_forgot
df = prev_c*dc_forgot
da = np.zeros_like(a)
da[:, 0:H] = i*(1-i)*di
da[:, H:2*H] = f*(1-f)*df
da[:, 2*H:3*H] = o*(1-o)*do
da[:, 3*H:4*H] = (1-c**2)*dc
dx = da.dot(Wx.T)
dprev_h = da.dot(Wh.T)
dWx = x.T.dot(da)
dWh = prev_h.T.dot(da)
db = np.sum(da, axis=0)
return dx, dprev_h, dprev_c, dWx, dWh, db
代码详解
- 这次的反向传播比较简单,我们从后往前计算梯度就可以了
- 注意
dnext_c 的问题,其梯度有两个输入,所以要进行 += 运算
3.4 LSTM: Forward
接下来我们会完成 LSTM 的前向传播步骤啦,我们会用到我们之前构造的 stepforward 函数
因为比较简单,我们直接来看代码
def lstm_forward(x, h0, Wx, Wh, b):
"""Forward pass for an LSTM over an entire sequence of data.
We assume an input sequence composed of T vectors, each of dimension D. The LSTM uses a hidden
size of H, and we work over a minibatch containing N sequences. After running the LSTM forward,
we return the hidden states for all timesteps.
一句话 T 个词语,
每个词维度为 D
隐状态维度 H
minibatch 大小为 N
经过 LSTM 处理,返回每个时间片的隐状态
Note that the initial cell state is passed as input, but the initial cell state is set to zero.
Also note that the cell state is not returned; it is an internal variable to the LSTM and is not
accessed from outside.
初始的细胞状态被置为0
细胞状态不会返回,细胞状态属于 LSTM 的内部变量,不是通过外部得到的
Inputs:
- x: Input data of shape (N, T, D)
- h0: Initial hidden state of shape (N, H)
- Wx: Weights for input-to-hidden connections, of shape (D, 4H)
- Wh: Weights for hidden-to-hidden connections, of shape (H, 4H)
- b: Biases of shape (4H,)
Returns a tuple of:
- h: Hidden states for all timesteps of all sequences, of shape (N, T, H)
- cache: Values needed for the backward pass.
"""
h, cache = None, None
N, T, D = x.shape
H = h0.shape[1]
h = np.zeros((N, T, H))
c = np.zeros((N, T, H))
c0 = np.zeros_like(h0)
cache = []
for i in range(T):
if i == 0:
h[:, i, :], c[:, i, :], forward_cache = lstm_step_forward(
x[:, i, :], h0, c0, Wx, Wh, b)
else:
h[:, i, :], c[:, i, :], forward_cache = lstm_step_forward(
x[:, i, :], h[:, i-1, :], c[:, i-1, :], Wx, Wh, b)
cache.append(forward_cache)
return h, cache
代码详解
- c 是一个内部状态(细胞状态),我们同样也要给他构造一个张量来进行保存
- c0 代表 c 的初始状态,初始化为全0
3.5 LSTM: backward
在这里我们会完成 LSTM 的反向传播算法,LSTM 的反向传播算法和 RNN 的反向传播算法类似,只需要把单步反向传播的函数更换一下就可以了,现在我们来看看代码
def lstm_backward(dh, cache):
"""Backward pass for an LSTM over an entire sequence of data.
Inputs:
- dh: Upstream gradients of hidden states, of shape (N, T, H)
- cache: Values from the forward pass
Returns a tuple of:
- dx: Gradient of input data of shape (N, T, D)
- dh0: Gradient of initial hidden state of shape (N, H)
- dWx: Gradient of input-to-hidden weight matrix of shape (D, 4H)
- dWh: Gradient of hidden-to-hidden weight matrix of shape (H, 4H)
- db: Gradient of biases, of shape (4H,)
"""
dx, dh0, dWx, dWh, db = None, None, None, None, None
N, T, H = dh.shape
D = cache[0][0].shape[1]
dx = np.zeros((N, T, D))
dh0 = np.zeros((N, H))
dh_i = np.zeros((N, H))
dWx = np.zeros((D, 4*H))
dWh = np.zeros((H, 4*H))
db = np.zeros((4*H))
dc_i = np.zeros_like(dh_i)
for i in range(T-1, -1, -1):
dh_i += dh[:, i, :]
dx_i, dh_i, dc_i, dWx_i, dWh_i, db_i = lstm_step_backward(
dh_i, dc_i, cache[i])
dx[:, i, :] = dx_i
dWx += dWx_i
dWh += dWh_i
db += db_i
dh0 = dh_i
return dx, dh0, dWx, dWh, db
代码详解
- 关于细胞状态的梯度 dc_i
- 反向传播是从后往前,因为细胞状态并没有输出,所以初始梯度应该是一个全零的向量
- dh_i 是 += 进行赋值,因为反向传播的梯度有两个方向,和 RNN 一样,一个是“上方”传递过来的梯度,另一个是从“右边”传递过来的梯度
- 同时 dh_i 初始也为0,因为刚开始只有“上方”传递过来的梯度,没有“右边”传递过来的梯度
3.6 LSTM Captioning Model
现在我们已经完成了 LSTM,接下来我们要在完善一下文件cs231n/classifiers/rrn.py 中CaptioningRNN 中的loss 函数,也就是把 LSTM 添加进去
只需要把 LSTM 的前向传播和反向传播添加进去就可以啦,添加的代码分别是:
if self.cell_type == "rnn":
hidden_state, hidden_cache = rnn_forward(
word_emb_in, ini_hidden_state, Wx, Wh, b)
else:
hidden_state, hidden_cache = lstm_forward(
word_emb_in, ini_hidden_state, Wx, Wh, b)
dout, grads["W_vocab"], grads["b_vocab"] = temporal_affine_backward(
dtemp_out, temp_cache)
if self.cell_type == "rnn":
demb, dini_hidden, grads["Wx"], grads["Wh"], grads["b"] = rnn_backward(
dout, hidden_cache)
else:
demb, dini_hidden, grads["Wx"], grads["Wh"], grads["b"] = lstm_backward(
dout, hidden_cache)
好了我们直接放出完整的代码,代码如下
def loss(self, features, captions):
"""
Compute training-time loss for the RNN. We input image features and
ground-truth captions for those images, and use an RNN (or LSTM) to compute
loss and gradients on all parameters.
Inputs:
- features: Input image features, of shape (N, D)
- captions: Ground-truth captions; an integer array of shape (N, T + 1) where
each element is in the range 0 <= y[i, t] < V
Returns a tuple of:
- loss: Scalar loss
- grads: Dictionary of gradients parallel to self.params
"""
captions_in = captions[:, :-1]
captions_out = captions[:, 1:]
mask = captions_out != self._null
W_proj, b_proj = self.params["W_proj"], self.params["b_proj"]
W_embed = self.params["W_embed"]
Wx, Wh, b = self.params["Wx"], self.params["Wh"], self.params["b"]
W_vocab, b_vocab = self.params["W_vocab"], self.params["b_vocab"]
loss, grads = 0.0, {}
ini_hidden_state, ini_cache = affine_forward(features, W_proj, b_proj)
word_emb_in, word_emb_cache = word_embedding_forward(
captions_in, W_embed)
if self.cell_type == "rnn":
hidden_state, hidden_cache = rnn_forward(
word_emb_in, ini_hidden_state, Wx, Wh, b)
else:
hidden_state, hidden_cache = lstm_forward(
word_emb_in, ini_hidden_state, Wx, Wh, b)
temp_out, temp_cache = temporal_affine_forward(
hidden_state, W_vocab, b_vocab)
loss, dtemp_out = temporal_softmax_loss(temp_out, captions_out, mask)
dout, grads["W_vocab"], grads["b_vocab"] = temporal_affine_backward(
dtemp_out, temp_cache)
if self.cell_type == "rnn":
demb, dini_hidden, grads["Wx"], grads["Wh"], grads["b"] = rnn_backward(
dout, hidden_cache)
else:
demb, dini_hidden, grads["Wx"], grads["Wh"], grads["b"] = lstm_backward(
dout, hidden_cache)
grads["W_embed"] = word_embedding_backward(demb, word_emb_cache)
dfeatures, grads["W_proj"], grads["b_proj"] = affine_backward(
dini_hidden, ini_cache)
return loss, grads
3.7 Overfit LSTM Captioning Model on Small Data
在这里我们会用一个小数据集来让 LSTM 过拟合,经过测试,总损失应该会低于0.5
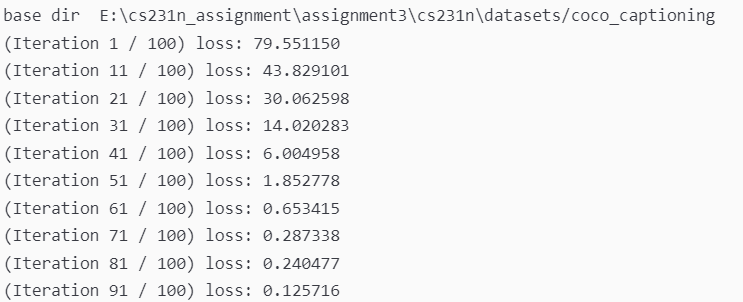
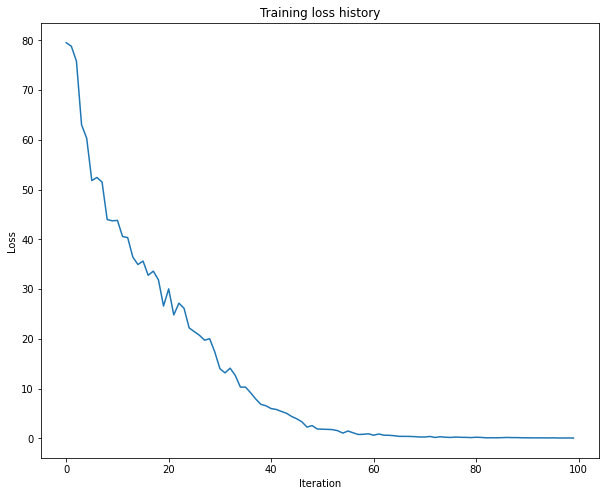
最后输出一下总损失:

总损失仅为 0.08,非常低!
3.8 LSTM Sampling at Test Time
接下来我们要用 LSTM 来进行图像描述了,我们需要稍微修改一下sample 函数来使用 LSTM,和上面的修改一样,只需要添加一个if就行了,我们直接来看完整代码
def sample(self, features, max_length=30):
"""
Run a test-time forward pass for the model, sampling captions for input
feature vectors.
At each timestep, we embed the current word, pass it and the previous hidden
state to the RNN to get the next hidden state, use the hidden state to get
scores for all vocab words, and choose the word with the highest score as
the next word. The initial hidden state is computed by applying an affine
transform to the input image features, and the initial word is the <START>
token.
每一个时间步,进行词嵌入,并进行前向传播的到当前的隐藏状态
用隐藏状态来得到单词的分数,选择分数最高的作为接下来的词
初始的隐藏状态使用输入图像做线性变换来得到
初始的词语为<START> token
For LSTMs you will also have to keep track of the cell state; in that case
the initial cell state should be zero.
Inputs:
- features: Array of input image features of shape (N, D).
- max_length: Maximum length T of generated captions.
Returns:
- captions: Array of shape (N, max_length) giving sampled captions,
where each element is an integer in the range [0, V). The first element
of captions should be the first sampled word, not the <START> token.
"""
N = features.shape[0]
captions = self._null * np.ones((N, max_length), dtype=np.int32)
W_proj, b_proj = self.params["W_proj"], self.params["b_proj"]
W_embed = self.params["W_embed"]
Wx, Wh, b = self.params["Wx"], self.params["Wh"], self.params["b"]
W_vocab, b_vocab = self.params["W_vocab"], self.params["b_vocab"]
hidden_state, _ = affine_forward(features, W_proj, b_proj)
word = self._start*np.ones(N, dtype=np.int32)
for i in range(max_length):
word_embed, _ = word_embedding_forward(word, W_embed)
if self.cell_type == "rnn":
hidden_state, _ = rnn_step_forward(
word_embed, hidden_state, Wx, Wh, b)
else:
hidden_state, _ = lstm_step_forward(
word_embed, hidden_state, Wx, Wh, b)
scores, _ = affine_forward(hidden_state, W_vocab, b_vocab)
word = np.argmax(scores, axis=1)
captions[:, i] = word
return captions
接下来我们就可以验证一下了
因为我是在windows下运行,所以对验证代码做如下修改:
for split in ['train', 'val']:
minibatch = sample_coco_minibatch(small_data, split=split, batch_size=2)
gt_captions, features, urls = minibatch
gt_captions = decode_captions(gt_captions, data['idx_to_word'])
sample_captions = small_lstm_model.sample(features)
sample_captions = decode_captions(sample_captions, data['idx_to_word'])
for gt_caption, sample_caption, url in zip(gt_captions, sample_captions, urls):
print(url)
plt.title('%s\n%s\nGT:%s' % (split, sample_caption, gt_caption))
plt.axis('off')
plt.show()
然后就可以进行验证了,但是看起来这个模型不是很聪明的样子
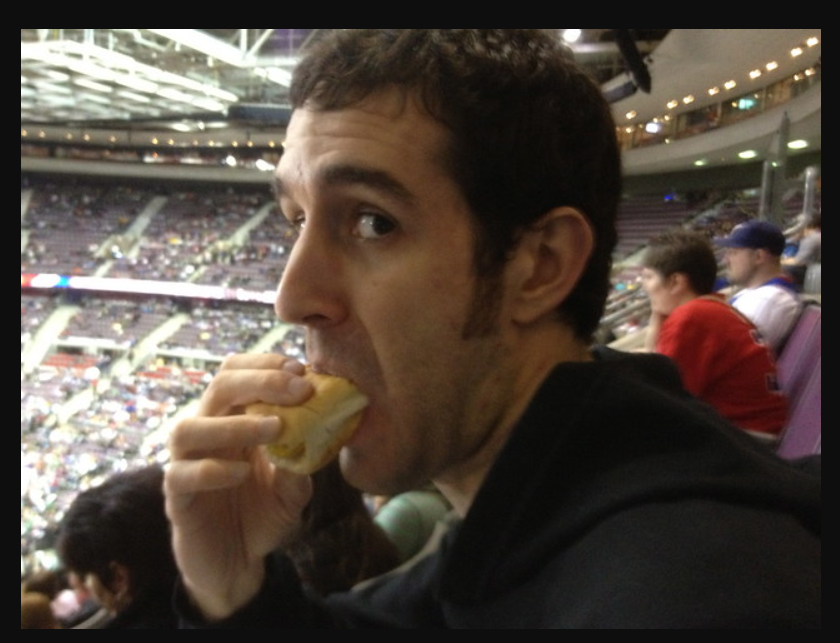

这描述的是一个东西吗?是不是少了个 hot 呀?
3. 总结、预告
在这次作业中,我们成功的使用了 numpy 实现了 LSTM
欸那有一个问题,之前作业二的剩余部分怎么就不见了呢?
呜呜呜拖拉博主马上会补上来的!对不起大家呜呜呜
|