官方API
RNN: 循环神经网络 short-term memory 只能记住比较短的时间序列的信息,时间长了会遗忘 GRU: 折中方案,相对于LSTM更加简单,计算成本更低 LSTM: 长短期记忆神经网络 long short-term memory 能够记住比较长的时间序列信息
RNN
$$ h'=tanh(W_{ih}x+b_{ih}+W_{hh}h+b_{hh})\\ \\ x:[batch\_size,input\_size]\\ h\:h':[batch\_size,hidden\_size]\\ W_{ih}:[input\_size,hidden\_size]\\ W_{hh}:[hidden\_size,hidden\_size]\\ b_{ih}\:b_{hh}:[hidden\_size] $$
CLASS torch.nn.RNNCell(input_size, hidden_size, bias=True, nonlinearity='tanh', device=None, dtype=None)
CLASS torch.nn.RNN(input_size, hidden_size, num_layers=1, nonlinearity='tanh', bias=True, batch_first=False, dropout=0, bidirectional=False, device=None, dtype=None)
GRU
$$ r=\sigma(W_{ir}x+b_{ir}+W_{hr}h+b_{hr})\\ z=\sigma(W_{iz}x+b_{iz}+W_{hz}h+b_{hz})\\ n=tanh(W_{in}x+b_{in}+r*(W_{hn}h+b_{hn}))\\ h'=(1-z)*h+z*n\\ \\ x:[batch\_size,input\_size]\\ h\:h':[batch\_size,hidden\_size]\\ W_{i?}:[input\_size,hidden\_size]\\ W_{h?}:[hidden\_size,hidden\_size]\\ b:[hidden\_size] $$
CLASS torch.nn.GRUCell(input_size, hidden_size, bias=True, device=None, dtype=None)
CLASStorch.nn.GRU(input_size, hidden_size, num_layers=1, bias=True, batch_first=False, dropout=0, bidirectional=False, device=None, dtype=None)
LSTM
$$ f=\sigma(W_{if}x+b_{if}+W_{hf}h+b_{hf})\\ i=\sigma(W_{ii}x+b_{ii}+W_{hi}h+b_{hi})\\ g=tanh(W_{ig}x+b_{ig}+W_{hg}h+b_{hg})\\ o=\sigma(W_{io}x+b_{io}+W_{ho}h+b_{ho})\\ c'=f*c+i*g\\ h'=o*tanh(c')\\ \\ x:[batch\_size,input\_size]\\ h\:h':[batch\_size,hidden\_size]\\ c\:c':[batch\_size,hidden\_size]\\ W_{i?}:[input\_size,hidden\_size]\\ W_{h?}:[hidden\_size,hidden\_size]\\ b:[hidden\_size] $$
CLASS torch.nn.LSTMCell(input_size, hidden_size, bias=True, device=None, dtype=None)
CLASStorch.nn.LSTM(input_size, hidden_size, num_layers=1, bias=True, batch_first=False, dropout=0, bidirectional=False, proj_size=0, device=None, dtype=None)
辅助函数
问题
一个batch中的3个样本,最长长度为5,用0填充,如下图1所示。将3个样本的数据按照时间步不断输入一个RNN、GRU、LSTM单元时,样本1和样本2有多次输入了padding的数据0。为了减少padding的影响,我们希望样本1输入1后即得到最后的hidden state(或加cell state)、样本2输入2、3、4后即得到最后的hidden state(或加cell state),如下图2所示。可以使用后面的PackedSequence实现。 在seq2seq应用中,编码器推荐用此方法,因为h_5是对待翻译句子的记忆,而解码器不必要
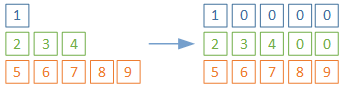
pad_sequence & unpad_sequence
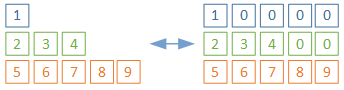
torch.nn.utils.rnn.pad_sequence(sequences, batch_first=False, padding_value=0.0)
参数:
sequences (list[Tensor]): list of variable length sequences
返回:
[max_seq_len, batch_size, *] (*表示剩余的多个维度)
torch.nn.utils.rnn.unpad_sequence(padded_sequences, lengths, batch_first=False)
参数:
padded_sequences (Tensor): [max_seq_len, batch_size, *] (*表示剩余的多个维度)
lengths (Tensor): length of original (unpadded) sequences.
返回:
list of variable length sequences
import torch
from torch.nn.utils.rnn import pad_sequence, unpad_sequence
a = torch.tensor([1])
b = torch.tensor([2, 3, 4])
c = torch.tensor([5, 6, 7, 8, 9])
test_data = [a, b, c]
lengths = torch.as_tensor([v.size(0) for v in test_data])
padded_sequences = pad_sequence(test_data)
print(padded_sequences)
sequences = unpad_sequence(padded_sequences, lengths)
print(sequences)
import torch
from torch.nn.utils.rnn import pad_sequence, unpad_sequence
a = torch.tensor([1])
b = torch.tensor([2, 3, 4])
c = torch.tensor([5, 6, 7, 8, 9])
test_data = [a, b, c]
lengths = torch.as_tensor([v.size(0) for v in test_data])
padded_sequences = pad_sequence(test_data)
print(padded_sequences)
sequences = unpad_sequence(padded_sequences, lengths)
print(sequences)
padded_sequences = pad_sequence(test_data)
print(padded_sequences)
sequences = unpad_sequence(padded_sequences, lengths)
print(padded_sequences)
padded_sequences = pad_sequence(test_data, batch_first=True)
print(padded_sequences)
sequences = unpad_sequence(padded_sequences, lengths, batch_first=True)
print(padded_sequences)
pack_padded_sequence & pad_packed_sequence
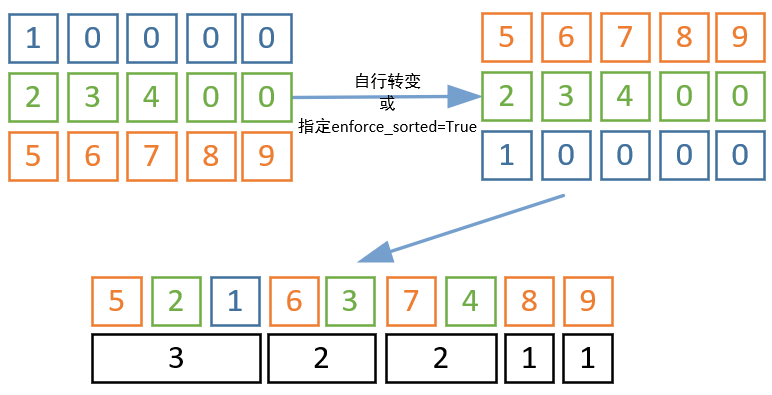
torch.nn.utils.rnn.pack_padded_sequence(input, lengths, batch_first=False, enforce_sorted=True)
参数:
input (Tensor): padded batch of variable length sequences.
lengths (Tensor or list(int)): list of sequence lengths of each batch element. (must be on the CPU if provided as a tensor).
enforce_sorted: if True, the input is expected to contain sequences sorted by length in a decreasing order. If False, the input will get sorted unconditionally. Default: True.
返回:
a PackedSequence object
torch.nn.utils.rnn.pad_packed_sequence(sequence, batch_first=False, padding_value=0.0, total_length=None)
参数:
sequence (PackedSequence): batch to pad
total_length (int, optional): if not None, the output will be padded to have length total_length. This method will throw ValueError if total_length is less than the max sequence length in sequence.
返回:
Tuple of Tensor containing the padded sequence, and a Tensor containing the list of lengths of each sequence in the batch. Batch elements will be re-ordered as they were ordered originally when the batch was passed to pack_padded_sequence or pack_sequence.
import torch
from torch.nn.utils.rnn import pad_sequence, pack_padded_sequence, pad_packed_sequence
a = torch.tensor([1])
b = torch.tensor([2, 3, 4])
c = torch.tensor([5, 6, 7, 8, 9])
test_data = [a, b, c]
lengths = torch.as_tensor([v.size(0) for v in test_data])
padded_sequences = pad_sequence(test_data)
print(padded_sequences)
pack_padded = pack_padded_sequence(padded_sequences, lengths, enforce_sorted=False)
print(pack_padded)
pad_packed = pad_packed_sequence(pack_padded)
print(padded_sequences)
pack_sequence & unpack_sequence
torch.nn.utils.rnn.pack_sequence(sequences, enforce_sorted=True)
参数:
sequences (list[Tensor]): A list of sequences of decreasing length.
enforce_sorted (bool, optional): if True, checks that the input contains sequences sorted by length in a decreasing order. If False, this condition is not checked. Default: True.
返回:
a PackedSequence object
torch.nn.utils.rnn.unpack_sequence(packed_sequences)
参数:
packed_sequences (PackedSequence): A PackedSequence object.
返回:
a list of :class:`Tensor` objects
import torch
from torch.nn.utils.rnn import pack_sequence, unpack_sequence
a = torch.tensor([1])
b = torch.tensor([2, 3, 4])
c = torch.tensor([5, 6, 7, 8, 9])
pack_seq = pack_sequence([a, b, c], enforce_sorted=False)
print(pack_seq)
unpack_seq = unpack_sequence(pack_seq)
print(unpack_seq)
语言翻译例
import torch
import torch.optim as optim
import torch.utils.data as Data
from torch import nn
from torch.nn import RNN
from torch.nn.utils.rnn import pad_sequence, pack_padded_sequence
if torch.cuda.is_available():
device = 'cuda'
else:
device = 'cpu'
epochs = 1000
input_size = 512
hidden_size = 512
sentences = [
['我 有 一 个 好 朋 友', 'S i have a good friend .', 'i have a good friend . E'],
['我 有 零 个 女 朋 友', 'S i have zero girl friend .', 'i have zero girl friend . E']
]
src_vocab = {'P': 0, '我': 1, '有': 2, '一': 3, '个': 4, '好': 5, '朋': 6, '友': 7, '零': 8, '女': 9}
src_idx2word = {w: i for i, w in src_vocab.items()}
src_vocab_size = len(src_vocab)
tgt_vocab = {'P': 0, 'i': 1, 'have': 2, 'a': 3, 'good': 4, 'friend': 5, 'zero': 6, 'girl': 7, 'S': 8, 'E': 9, '.': 10}
tgt_idx2word = {w: i for i, w in tgt_vocab.items()}
tgt_vocab_size = len(tgt_vocab)
class MyDataSet(Data.Dataset):
"""
自定义DataLoader,返回:
enc_input: [batch_size, len_src]
dec_input: [batch_size, len_tgt]
dec_output: [bath_size, len_tgt]
enc_input_len: [batch_size]
"""
def __init__(self, sentences, src_vocab, tgt_vocab):
super(MyDataSet, self).__init__()
self.len = len(sentences)
self.enc_input = []
self.dec_input = []
self.dec_output = []
self.enc_input_len = []
for i in range(self.len):
self.enc_input.append(torch.tensor([src_vocab[n] for n in sentences[i][0].split()]))
self.dec_input.append(torch.tensor([tgt_vocab[n] for n in sentences[i][1].split()]))
self.dec_output.append(torch.tensor([tgt_vocab[n] for n in sentences[i][2].split()]))
self.enc_input_len.append(len(self.enc_input[-1]))
self.enc_input = pad_sequence(self.enc_input, batch_first=True)
dec = pad_sequence(self.dec_input + self.dec_output, batch_first=True)
self.dec_input = dec[:self.len]
self.dec_output = dec[self.len:]
def __len__(self):
return self.len
def __getitem__(self, idx):
return self.enc_input[idx], self.dec_input[idx], self.dec_output[idx], self.enc_input_len[idx]
loader = Data.DataLoader(MyDataSet(sentences, src_vocab, tgt_vocab), 2, True)
class Model(nn.Module):
def __init__(self, input_size, hidden_size, src_vocab_size , tgt_vocab_size):
super(Model, self).__init__()
self.src_emb = nn.Embedding(src_vocab_size, input_size)
self.tgt_emb = nn.Embedding(tgt_vocab_size, input_size)
self.rnn = RNN(input_size, hidden_size)
self.projection = nn.Linear(hidden_size, tgt_vocab_size)
def forward(self, enc_input, dec_input, enc_input_len):
"""
:param enc_input: [batch_size, len_src]
:param dec_input: [batch_size, len_tgt]
:param enc_input_len: [batch_size]
:return:
"""
enc_input = self.src_emb(enc_input.t())
dec_input = self.tgt_emb(dec_input.t())
_, hidden_state = self.rnn(pack_padded_sequence(enc_input, enc_input_len, enforce_sorted=False))
output, _ = self.rnn(dec_input, hidden_state)
dec_logits = self.projection(output)
dec_logits = dec_logits.view(-1, dec_logits.size(-1))
return dec_logits
model = Model(input_size, hidden_size, src_vocab_size, tgt_vocab_size)
model = model.to(device)
criterion = nn.CrossEntropyLoss(ignore_index=tgt_vocab['P'])
optimizer = optim.SGD(model.parameters(), lr=1e-3, momentum=0.99)
for epoch in range(epochs):
for enc_input, dec_input, dec_output, enc_input_len in loader:
"""
enc_input: [batch_size, len_src]
dec_input: [batch_size, len_tgt]
dec_output: [batch_size, len_tgt]
enc_input_len: [batch_size]
"""
enc_input, dec_input, dec_output = enc_input.to(device), dec_input.to(device), dec_output.to(device)
output = model(enc_input, dec_input, enc_input_len)
loss = criterion(output, dec_output.t().flatten())
print('Epoch:', '%04d' % (epoch + 1), 'loss =', '{:.6f}'.format(loss))
optimizer.zero_grad()
loss.backward()
optimizer.step()
def greedy_decoder(model, enc_input, src_vocab, tgt_vocab, device):
"""
:param model: 模型
:param enc_input: str sequence
:param src_vocab: 源语言字典
:param tgt_vocab: 目标语言字典
:param device: 设备
:return:
"""
seq = torch.tensor([[src_vocab[n] for n in enc_input.split()]], device=device)
dec_input = torch.zeros(1, 0).to(device=device, dtype=torch.int64)
next_symbol = tgt_vocab['S']
terminal = False
while not terminal:
dec_input = torch.cat([dec_input, torch.tensor([[next_symbol]]).to(device)], -1)
dec_logits = model(seq, dec_input, [seq.shape[1]])
next_symbol = dec_logits[-1].argmax()
print(tgt_idx2word[next_symbol.item()])
if next_symbol == tgt_vocab["E"]:
terminal = True
greedy_decoder(model, '我 有 零 个 女 朋 友', src_vocab, tgt_vocab, device)
|